Introduction
There is a consensus that language experience contributes to changes in brain structure. Increasingly, researchers acknowledge that this restructuring is a dynamic and possibly cyclic process in which brain regions involved in language processing and control (de Bot, Reference De Bot2019; DeLuca et al., Reference DeLuca, Rothman and Pliatsikas2019; Pliatsikas & Luk, Reference Pliatsikas and Luk2016; Abutalebi & Green, Reference Abutalebi and Green2016; Grundy et al., Reference Grundy, Anderson and Bialystok2017; Green & Abutalebi, Reference Green and Abutalebi2013) may first expand as learners begin to acquire a second language, but with increasing proficiency, and/or increases in immersion, these regions may return to baseline values similar to those evidenced in monolingual speakers. Coincident with these changes are systematic alterations in the weighting and engagement of cortical and subcortical networks (i.e., Dynamic Restructuring Model (DRM), Pliatsikas, Reference Pliatsikas2020; Bilingual Anterior to Posterior and Subcortical Shift (BAPSS), Grundy et al., Reference Grundy, Anderson and Bialystok2017). However, these patterns of changes have been largely predicated on studies of bilinguals, speakers of one second language, compared to monolinguals. Neurolinguistic studies investigating fluent speakers of multiple languages (a.k.a., three or more) are far less common. The emphasis on studies of second language acquisition and use in bilingual speakers may provide only a partial picture of the effects of linguistic experience on brain organization. Additionally, while initial stages of second language acquisition are well documented in the literature through training (e.g., Della Rosa et al., Reference Della Rosa, Videsott, Borsa, Canini, Weekes, Franceschini and Abutalebi2013; Richardson et al., Reference Richardson, Thomas, Filippi, Harth and Price2010) and cross-sectional studies (Golestani, Reference Golestani2014; Klein et al., Reference Klein, Mok, Chen and Watkins2014; Olulade et al., Reference Olulade, Jamal, Koo, Perfetti, LaSasso and Eden2016) there are fewer comparative studies of brain structure in bi- or multiple languages in individuals who show high levels of proficiency (for reviews of this literature see Pliatsikas, Reference Pliatsikas2019, Reference Pliatsikas2020; Pliatsikas et al., Reference Pliatsikas, DeLuca and Voits2020; Pliatsikas & Luk, Reference Pliatsikas and Luk2016).
Recent models of brain restructuring related to second language experience have indicated a lawful progression from initial expansion, which occurs in the initial stages of 2nd language learning to decreases in grey matter (GM) for more proficient bilinguals (i.e., DRM – Pliatsikas, Reference Pliatsikas2020; BAPSS – Grundy et al., Reference Grundy, Anderson and Bialystok2017). Grundy et al. (Reference Grundy, Anderson and Bialystok2017) suggest that the relationship between the GM structure and second language expertise follows an inverted U shape. For example, compared to monolinguals, bilinguals at the initial stages of language learning show increases in anterior cortical brain regions, and regions of the inferior parietal cortex (Hosoda et al., Reference Hosoda, Tanaka, Nariai, Honda and Hanakawa2013; Mechelli et al., Reference Mechelli, Crinion, Noppeney, O'Doherty, Ashburner, Frackowiak and Price2004; Olulade et al., Reference Olulade, Jamal, Koo, Perfetti, LaSasso and Eden2016; Stein et al., Reference Stein, Federspiel, Koenig, Wirth, Strik, Wiest, Brandeis and Dierks2012). With increased proficiency, bilinguals rely less upon anterior brain regions and instead recruit subcortical and posterior brain regions to process language. This is taken as a reflection of more efficient language processing (Grundy et al., Reference Grundy, Anderson and Bialystok2017; Mårtensson et al., Reference Mårtensson, Eriksson, Bodammer, Lindgren, Johansson, Nyberg and Lövdén2012; Osterhout et al., Reference Osterhout, Poliakov, Inoue, McLaughlin, Valentine, Pitkanen, Frenck-Mestre and Hirschensohn2008).
Particularly pertinent to the present study are findings that have examined comparisons of highly proficient bilinguals to monolinguals. Researchers have reported changes in the volume of various subcortical regions including the putamen, thalamus, pallidum, and caudate nucleus (Burgaleta et al., Reference Burgaleta, Sanjuán, Ventura-Campos, Sebastian-Galles and Ávila2016; Ressel et al., Reference Ressel, Pallier, Ventura-Campos, Díaz, Roessler, Ávila and Sebastián-Gallés2012). However, it should be noted that both, GM structural increases (Burgaleta et al., Reference Burgaleta, Sanjuán, Ventura-Campos, Sebastian-Galles and Ávila2016; Pliatsikas et al., Reference Pliatsikas, DeLuca, Moschopoulou and Saddy2017) and decreases have been reported within these populations. For example, Pliatsikas et al. (Reference Pliatsikas, DeLuca, Moschopoulou and Saddy2017) showed increases in the GM surface of one group of highly immersed sequential bilinguals (of approximately seven years of immersion in a second language context) compared to monolinguals, in bilateral putamen, globus pallidus, and right thalamus. While for another group of immersed sequential bilinguals (immersed in a second language context for an average of 3.5 years), the same authors show expansions of the lateral posterior surface of the right caudate nucleus but contractions in the posterior surface of this region along with decreases in the bilateral thalamus and putamen, compared to monolingual controls. The authors did not report whether these structural changes were accompanied by volumetric changes. These mixed results were attributed to the amount of immersion that differed across the two bilingual groups in these two studies. One might note, this is one of the few studies that have tried to separate the effects of immersion from high bilingual proficiency.
Although very limited, the literature on sequential multilinguals (individuals that have learned an L3 after acquiring an L2) seems to suggest a similar cycle of adaptations to the ones observed in sequential bilingualism, in which the same brain regions are undergoing similar structural changes (Grogan et al., Reference Grogan, Parker Jones, Ali, Crinion, Orabona, Mechias, Ramsden, Green and Price2012; Kaiser et al., Reference Kaiser, Eppenberger, Smieskova, Borgwardt, Kuenzli, Radue, Nitsch and Bendfeldt2015; Pliatsikas, Reference Pliatsikas2020). To date, there have been relatively few assessments of brain structure differences in multilinguals with high levels of language proficiency. Abutalebi et al. (Reference Abutalebi, Della Rosa, Gonzaga, Keim, Costa and Perani2013) compared multilingual speakers to monolinguals and reported increased GM volume in the putamen of multilinguals compared to monolinguals, along with higher activation (measured with functional MRI) in the putamen of only less proficient multilinguals. Note, however, that the multilinguals in the Abutalebi et al. (Reference Abutalebi, Della Rosa, Gonzaga, Keim, Costa and Perani2013) study had medium proficiency in the third language (L3). Studies by Kaiser et al. (Reference Kaiser, Eppenberger, Smieskova, Borgwardt, Kuenzli, Radue, Nitsch and Bendfeldt2015) and Hämäläinen et al. (Reference Hämäläinen, Joutsa, Sihvonen, Leminen and Lehtonen2018) examined structural adaptations in multilinguals – however, they aimed to evaluate whether there are differential structural patterns associated with simultaneous versus sequential second and third language acquisition. These studies did not directly address comparisons between highly proficient multilinguals and monolinguals.
Models such as the DRM predict further GM normalization of subcortical structure, along with cortical normalization at peak efficiency stages of second language expertise (Pliatsikas, Reference Pliatsikas2020). However, there are several issues with this characterization. First, the construct of the “peak efficiency” stage of second language expertise is not well defined.
Similarly, the manifestation of normalization (and/or renormalization) in brain GM as a result of high proficiency and immersion is still poorly understood. For example, statements regarding patterns of change (or expected change) in subcortical structures are mixed. In the context of a discussion of peak efficiency Pliatsikas (Reference Pliatsikas2020) suggests that in highly experienced bilinguals one might see a “full renormalization of the caudate and fairly stable volumes in the putamen and globus pallidus” for bilinguals relative to monolinguals. While evidence from DeLuca et al. (Reference DeLuca, Rothman and Pliatsikas2019) suggests that subcortical structures, specifically the caudate nucleus, show reductions in volume as a function of language immersion and age of acquisition (Pliatsikas, Reference Pliatsikas2020) relative to monolingual baseline (see also Elmer et al., Reference Elmer, Hänggi and Jäncke2014 for evidence coming from simultaneous interpreters). With respect to multilinguals, in the extreme, the DRM model would seem to suggest that renormalization will be found regardless of the number of languages one knows as long as a speaker holds some high degree of proficiency in the languages known and continues their second language exposure (Pliatsikas, Reference Pliatsikas2020); but, to our knowledge, this has not been tested.
In this study, we investigate brain structure in a group of highly proficient multilinguals, speakers of four or more languages, with extensive immersion in multiple language environments. There are two important characteristics of this group: a) all multilingual participants are highly proficient in at least three languages, as assessed through a battery of language tests; b) they have a very similar linguistic background, with an extensive amount of language use and language immersion in multi-linguistic environments. We compare quantitative measures of brain structure in these multilinguals to a group of age-matched monolinguals. Monolingual brains establish one possible end-state which may serve as a benchmark against a myriad of more complicated language profiles. This same approach has been used previously by Abutalebi et al. (Reference Abutalebi, Della Rosa, Gonzaga, Keim, Costa and Perani2013), who compared restructuring patterns of multilinguals compared to monolinguals.
Since we recruited a highly proficient, highly immersed, life-long multilingual group, we reasoned that the brain changes observed would be most similar to those described for highly proficient bilinguals. We examine whether there are volumetric differences within a-priori-defined regions of interest (ROIs) and first evaluate whether such changes are regionally specific; second, we report the direction of changes observed. These particular ROIs have been consistently documented in neurolinguistic literature for their involvement in language processing and control (i.e., Abutalebi & Green, Reference Abutalebi and Green2016; Fedorenko et al., Reference Fedorenko, Hsieh, Nieto-Castañón, Whitfield-Gabrieli and Kanwisher2010; Fedorenko & Thompson-Schill, Reference Fedorenko and Thompson-Schill2014; Green & Abutalebi, Reference Green and Abutalebi2013). We predict that proficient multilinguals will show no differences in GM thickness in anterior brain regions compared to monolinguals, specifically in the inferior frontal gyrus (IFG) and middle temporal gyri (MTG; including the Heschl's Gyrus), the anterior cingulate cortex (ACC) and the anterior insula (AI). While differences should be observed in posterior brain regions – namely, the superior temporal gyri (STG), inferior parietal lobe (IPL – namely, the angular gyrus (AG)) and superior parietal lobe (i.e., precuneus), along with differences in selected subcortical regions, including the caudate, putamen, and thalamus. This prediction follows from consideration of the BAPSS theory, which argues that increasing multilingual proficiency and language expertise is associated with greater recruitment of posterior/subcortical regions, and weaker reliance on the frontal regions (Grundy et al., Reference Grundy, Anderson and Bialystok2017). To the extent that increased energetic demands lead to volumetric neural expansion, one may predict that highly proficient multilinguals may show robust changes in these regions relative to monolingual controls. However, we acknowledge that, in some cases, linguistic efficiency in bilinguals may be reflected as regional GM decreases compared to monolinguals (e.g., Pliatsikas et al., Reference Pliatsikas, DeLuca, Moschopoulou and Saddy2017).
Methods
Participants
Thirty (15 monolingual and 15 multilingual) volunteers participated in this study, following written informed consent, approved by the Institutional Review Board at the University of California, Davis. All participants had no previous history of neurological or developmental disorders. Participants were between 19 and 33 years of age, (mean 24.8 years, SD 1.7 years for the monolingual group and mean 27.6 years, SD 3.2 years for the multilinguals) and were consistent right-handers, as measured by the Edinburgh Handedness Inventory (Oldfield, Reference Oldfield1971), laterality index: mean of 85.8% for the monolinguals and a mean of 81.63% for the multilingual group, the scores ranging from 0 (no hand preference) to +100m (always using the right hand) for all participants. All multilinguals were born and grew up in Moldova, a bilingual country with most of the population speaking Romanian and Russian fluently. They acquired Romanian as their native language (L1) and started learning Russian (L2) in elementary/middle school (mean age of 5.8 years), but also used it in everyday conversations, as well as while watching Russian television. All participants learned an additional second language (e.g., French, Italian, Belarusian, German, or Spanish) sometime between birth and middle school. At a mean of 15.2 years, all multilingual participants moved to the United States, where they started learning and using English 50% of the time on average (14 out of 15 participants went to high school and/or college in the U.S.).
All multilingual participants reported speaking 4 or 5 languages; however, they reported higher proficiency in three of these, commonly spoken by all multilinguals in the group – namely, Romanian (native language), Russian (an early learned second language that we will refer to as L2), and English (a later learned second language, that we will refer to as the L3). All monolingual participants have indicated to only have ever used English to communicate, and despite learning a foreign language in school (for six months on average), they are not able to communicate in any other language.
Behavioral data collection
Participants completed several language proficiency tests and a background questionnaire. Since all multilingual participants spoke Romanian, Russian, and English alike, we adapted/translated the set of proficiency tests used in these three languages. While proficiency was not assessed in the other second languages, the participants self-rated their proficiency in all languages they reported speaking. The set of tests used in this study: included a Self-Rating Questionnaire, a Language Production, and Vocabulary Assessment, a standardized test originally developed to assess semantic and vocabulary knowledge widely used in other studies – namely, the Shipley Vocabulary Test (Shipley, Reference Shipley1940), adapted/translated to Romanian and Russian; a Semantic and Vocabulary Knowledge Test – based on a previously validated production/vocabulary knowledge test (MINT; Ivanova et al., Reference Ivanova, Salmon and Gollan2013); and a Grammatical Assessment Test, developed for this study based on Linebarger et al. (Reference Linebarger, Schwartz and Saffran1983). Monolingual participants completed the tests in writing, on one occasion, before or after the scanning session. The multilinguals completed the tests in two sessions. They completed the language proficiency tests in the first session (the order of the language tests was randomized across participants) while the background information and the self-rating questionnaire were completed in a second session before or after the scanning session.
Behavioral data analysis
Welch's two-sample t-tests were performed to assess differences in native language proficiency across groups. Paired sample t-tests were performed to assess differences in proficiency across languages within the multilingual group. The behavioral data were manually digitized for ease of processing. The test averages and statistical analysis were performed in R software (R Core Team, 2020; www.R-project.org ). Other summaries and descriptive analyses were performed in Excel. Tables and figures were also created in Excel and R.
MRI data acquisition
The participants were scanned with a Siemens 64-channel 3-Tesla “Skyra” MRI System (Siemens Healthcare, Erlangen, Germany) at the Imaging Research Center of the University of California, Davis. For the structural data, a T1-weighted imaging sequence was used, with an MR-RAGE (TfL) sequence with a voxel size = 0.9 × 0.9 × 0.9 mm3, FOV = 243 × 243 mm, 208 sagittal slices, 7-degree flip angle, TR = 2500msec, TI = 1100 msec, and TE = 4.44 msec, Bandwith 160 Hz/Px; GRAPPA = 2, 32 reference lines.
MRI data processing
The raw MPRAGE images (.dcm) were converted to NIFTI format using the “dcm2nii” function obtained from www.nitrc.org. We used a Debian-based Ubuntu distribution to run the different command tools in BASH (Bourne-Again Shell) command language interpreter. Data preprocessing, image reconstruction, and volumetric and cortical parcellation were carried out with the “recon-all” command from the Freesurfer image analysis suite, version 7.2.0 (http://surfer.nmr.mgh.harvard.edu/). The preprocessing steps performed were non-brain removal, referred to as “skullstripping”, using a hybrid watershed/surface deformation procedure (Ségonne et al., Reference Ségonne, Dale, Busa, Glessner, Salat, Hahn and Fischl2004) automated Talairach transformation, and segmentation of the subcortical white matter and deep gray matter volumetric structures (Fischl et al., Reference Fischl, Salat, Busa, Albert, Dieterich, Haselgrove, Van Der Kouwe, Killiany, Kennedy and Klaveness2002, Reference Fischl, Salat, Van Der Kouwe, Makris, Ségonne, Quinn and Dale2004a) Intensity normalization was performed, as described in (Sled et al., Reference Sled, Zijdenbos and Evans1998). An estimation of the delimitation between white and grey matter and subcortical structures was then performed, followed by automated topology correction (Fischl et al., Reference Fischl, Liu and Dale2001; Ségonne et al., Reference Ségonne, Pacheco and Fischl2007). From these estimates, the pial surface of the brain is estimated. Surface deformation following intensity gradients was estimated (Dale et al., Reference Dale, Fischl and Sereno1999; Dale & Sereno, Reference Dale and Sereno1993; Fischl & Dale, Reference Fischl and Dale2000). This procedure produces optimal placement of the border between the gray-white and gray-cerebrospinal fluid where there is a shift in the intensity, defining the transitions to another type of tissue. After the computation of cortical models, deformable procedures are performed, including two steps of surface inflation and flattening (Fischl et al., Reference Fischl, Sereno, Tootell and Dale1999) and registration to a spherical atlas based on individual cortical folding patterns to match cortical geometry across subjects (Fischl et al., Reference Fischl, Sereno, Tootell and Dale1999). Cortical parcellations into units based on gyral and sulcal structure are performed, based on two atlases, the Desikan-Killiany atlas and the Destrieux atlas (Desikan et al., Reference Desikan, Ségonne, Fischl, Quinn, Dickerson, Blacker, Buckner, Dale, Maguire and Hyman2006; Fischl et al., Reference Fischl, Salat, Van Der Kouwe, Makris, Ségonne, Quinn and Dale2004a). The parcellations from the template are matched back to the individual subjects and adjusted for small variations depending on the individual's anatomy. These steps output several surface-based maps of curvature and sulcal depth, by using the spatial intensity gradients across tissue classes. Cortical thickness is calculated as the closest distance from the gray-white boundary to the gray-CSF boundary at each vertex on the tessellated surface (Fischl & Dale, Reference Fischl and Dale2000). The algorithms are not restricted by voxel resolution (i.e., from the raw original data) and can detect submillimeter differences between groups. Procedures for the measurement of cortical thickness have been validated against histological analysis (Rosas et al., Reference Rosas, Liu, Hersch, Glessner, Ferrante, Salat, van Der Kouwe, Jenkins, Dale and Fischl2002) and manual measurements (Fischl et al., Reference Fischl, Van Der Kouwe, Destrieux, Halgren, Ségonne, Salat, Busa, Seidman, Goldstein and Kennedy2004b; Kuperberg et al., Reference Kuperberg, Broome, McGuire, David, Eddy, Ozawa, Goff, West, Williams and van der Kouwe2003). FreeSurfer morphometric procedures have been demonstrated to show good test-retest reliability across scanner manufacturers and different field strengths (Reuter et al., Reference Reuter, Schmansky, Rosas and Fischl2012).
MRI data analysis
FreeSurfer offers a rich set of data outputs that can be further analyzed for comparisons across groups. The outputs include an average number of the metrics calculated (i.e., thickness, area, and volume) for each region in which the brain is segmented and parcellated. Three metrics are provided for each cortical ROI average cortical thickness (the average of the entire region), the total area for the ROI (based on the surface mesh model, in which each triangle is assigned to a brain area), and the total volume (which is a measure of thickness multiplied by the area). For this analysis, the volume information for the ROI was extracted from the subcortical segmentation and white matter parcellation outputs. We restricted the measures of cortical structural changes to the “thickness” measure for the cortical ROIs. Thickness is one of the most sensitive metrics to certain clinical conditions and group differences (Fischl & Dale, Reference Fischl and Dale2000; Lerch & Evans, Reference Lerch and Evans2005). It is measured as the distance between white and pial surfaces (1-5mm). The data used for the analysis were provided by parcellations on the Destrieux Atlas (Fischl et al., Reference Fischl, Van Der Kouwe, Destrieux, Halgren, Ségonne, Salat, Busa, Seidman, Goldstein and Kennedy2004b). Figures 1 and 2 show the ROIs extracted for the analysis based on the subcortical segmentation and cortical parcellations, overlayed on the study template.

Figure 1. Subcortical regions of interest.
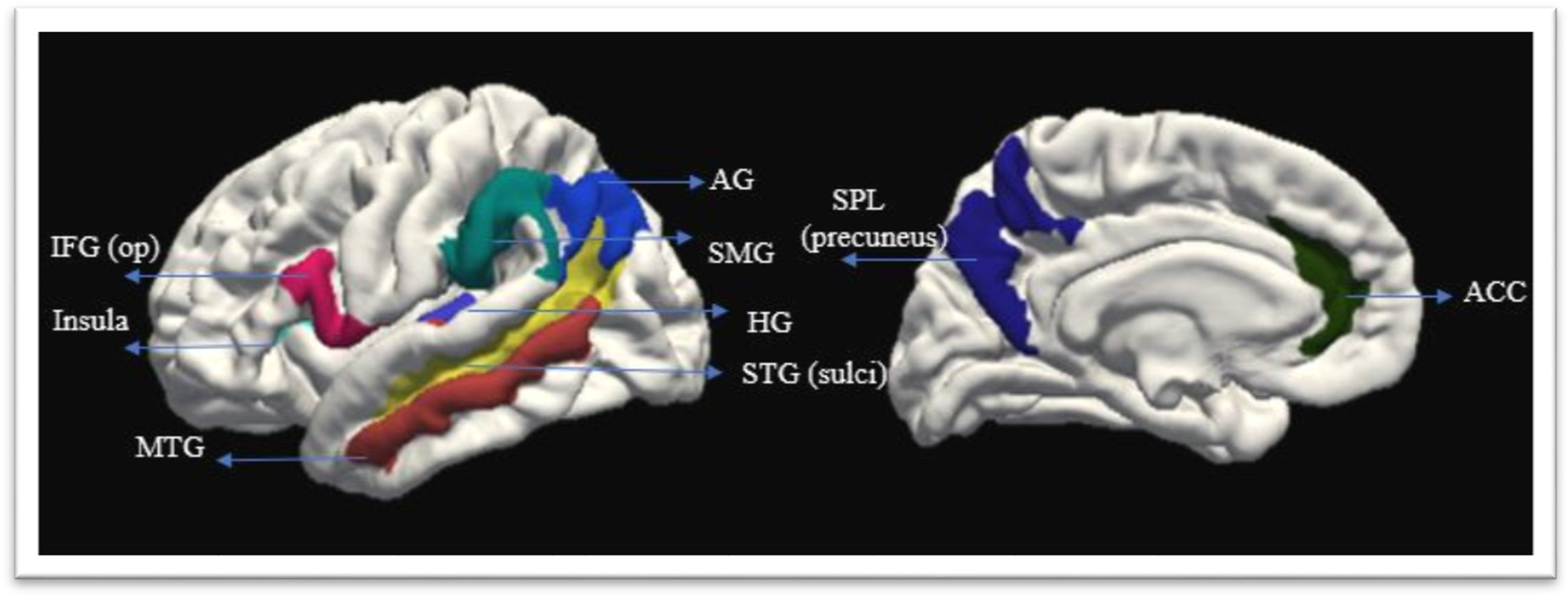
Figure 2. Cortical regions of interest.
Welch's two-sample t-tests across the two groups were performed for all areas of interest. We provide the uncorrected p-values, as well as the FDR (false discovery rate), and corrected p-values by the number of tests performed. We performed additional correlation and regression analysis to evaluate the potential effects of other covariates (namely, Age, Biological Sex, Years of Education, Handedness, and total Intracranial Volume (ICV) on the results. We report the beta and p-values for regression analysis controlling for the effect of total ICV in Appendix 1. We first computed correlation analysis between covariates of age, biological sex, years of education, and standardized values for total ICV and Handedness with the GM thickness in the precuneus and AG. The total ICV was computed by FreeSurfer. The handedness variable was calculated as a laterality quotient LQ=(R-L)/(R+L)*100. Lastly, regression analyses were performed including the covariates that showed statistically significant association at a p < .1, with the GM thickness in the regions that survived multiple comparison corrections. The test averages and statistical analysis were performed in R software (R Core Team, 2020; www.R-project.org).
Results
Behavioral data and language proficiency
All monolingual participants rated their English proficiency at 100%; multilingual participants rated their L1 proficiency at 100%, L2 at 72% (SD = 0.94), and L3 at 78% (SD = 0.91). Table 1 shows a summary of the background and self-rating assessments. In addition to the measures of the age of acquisition (AoA) and self-rated fluency. The multilingual participants have had several years of high L2 immersion (with a mean of 11.3 years of using it every day) and L3 (with a mean of 12.5 years of daily use).
Table 1. Participants’ Background Summary

Description: P: participant number; Years of Ed: years of education; Bio. Sex: biological sex; Engl: English, Rom: Romanian, Russ: Russian; AoA: age of acquisition; SRP: Self-rated proficiency; Mono: monolingual; Multi: multilingual; Fr: French, Span: Spanish, Ger: German; Blr: Belarus, It: Italian.
The L2 language was used from a mean of 5.5 years of age to a mean of approximately 15 years, for a total of 9.5 years every day, and have used it less since. After the age of 15 (group average), the participants started using only L3 daily (with a mean of 12.5 years), while L2 only on occasion. Thus, while participants have had similar exposure and immersion in both L3 and L2, they differ at different times in life. The languages that were used on an everyday basis at the time of the scanning were only L1 (at home, with family and friends) and L3 (at school, work, church, store, etc.) while L2 was used differently by participants and less than L3 and L1. The overall percentage errors for the three tests were 11.84% (SD = 5.3%) for the monolingual group L1. For the multilingual group, the percentage error was 10.02% (SD = 5.3%) for L1, 19.39% (SD = 8.5%) for L2, and 18.04% (SD = 9.6%) for L3 respectively. A two-sample t-test was carried out for comparing proficiency between the groups in the native language. The difference between the L1 monolinguals and L1 multilinguals (t(28) = 1.05, p = .3; 95% CI, 2.56 to 0.83) was not significant. Paired sample t-tests were computed to investigate differences in proficiency within the multilingual group between the languages. The differences between L1 and L2 scores (t(14) = 4.4; p < .001; 95% CI, 2.3 to 6.63) and L1 and L3 naming (t(14) = 4.2; p< .001; 95% CI, 1.87 to 5.76) were significant. There was not a statistical difference in scores between L2 and L3 (t(14) = .5, p = .5; 95% CI, 3.3 to 2.01). Table 2 shows the results of the statistical proficiency tests performed.
Table 2. Proficiency Measures Statistic Testing Results

Description: P-values were FDR-corrected for the number of tests. Note: mono: monolingual; multi: multilingual; GAT: Grammar test, MINT: Multilingual Naming Tests; SHIPLEY: Self-Administering Scale for Measuring Intellectual Impairment and Deterioration.
Morphometry results
Compared to the monolinguals, the multilingual group showed decreased thickness in several cortical areas, two of these – namely the left precuneus of the superior parietal lobe (SPL), t(26.5) = 3.37, p < .01 (95% CI, 0.4 to 0.18), and the right angular gyrus (AG), t(26.2) = 3.24, p < .01 (95% CI, 0.08 to 0.35) – remained significant at p < .05 (FDR; see Table 3).
Table 3. Morphometry Test Results.

Description: The p-values were corrected using a factor of 22 (number of ROIs) with the false discovery rate method (FDR). Cortical Regions as named in the Destrieux Atlas (Fischl, Reference Fischl, Sereno, Tootell and Dale2004b).
Decreases in GM for the multilingual groups within the cortical language areas were limited to the left Transversotemporal Gyrus (Hesch's gyrus), t(28) = 2.13, p = .04 (95% CI, 0.006 to 0.34) and right superior temporal sulcus (rSTS), t(28) = 2.1, p < .05 (95% CI, 0.0005 to 0.25). Additional decreases were observed in the left anterior insula, t(26.7) = 2.77, p < .01 (95% CI, 0.04 to 0.25), and the volume of the left putamen t(26) = 2.3, p < .05 (95% CI, 34.3 to 766.3). However, these did not survive multiple comparisons. Lastly, the only region that showed a greater GM thickness for the multilinguals compared to the monolinguals was the right anterior cingulate cortex (ACC), t(27.5) = −2.1, p < .05 (95% CI, -0.17 to -0.002), although this difference did not survive multiple comparison correction.
Additional analysis for the AG thickness
We computed a point biserial correlation analysis to evaluate an association between the biological sex and GM thickness in the AG. The two variables were not significantly correlated, rpb(28) = 1.4, p = .16. Similarly, there was no significant correlation between GM thickness in the AG and variables of years of education (r(28) = .022, p = .9), ITCV (r (28) = - .02, p = .9), and handedness (r(28) =.29, p = .1). We obtained a marginally significant negative point-biserial correlation between AG and age, r(28) = −.37, p <.05, suggesting a decrease in GM thickness in the right AG with age.
We then computed a multiple linear regression analysis predicting GM thickness in the AG with age and group membership. A significant regression equation was found (F(2, 27) = 5.34, p < .01), with an R2 of .28. Participant's predicted AG GM thickness is equal to 2.95 − 0.182 (group membership) − 0.01(age), where group membership is coded as 1 = multilinguals, 0 = monolingual, and age is measured in years. Participants’ AG GM was reduced on average by .182mm for multilinguals compared to monolinguals and decreased by .01 for each year of age. While group membership was a significant predictor of grey matter thickness (β= −.182 SE = .07, p < .03), age was not a significant predictor ((β = −.01, SE = .01, p < .4).
Additional analysis for the precuneus thickness
There was no significant correlation between GM in the precuneus and variables of years of education (r(28) = .16, p = .4), total ICV (r(28) =.19, p = .3), age (r(28) =−0.6, p = .5) and handedness (r(28) = .25, p = .2), hence no further regressions were performed. A non-significant point biserial correlation was also obtained between biological sex and left hemisphere precuneus GM thickness (rpb(28) = -.09, p = .6).
Discussion
Behavioral data
In the present paper, we provide quantitative assessments of language proficiency across three languages, English (L1 for monolinguals and L3 for multilinguals) Romanian (L1 for multilinguals), and Russian (L2 for multilinguals), and examine language skills at three structural levels, phonological, lexico-semantic, and grammatical. In addition, a background questionnaire evaluated the age of acquisition, educational background, language use, and experience measures for each participant. First, as expected, both groups showed excellent performance in their native languages. Second, the multilingual group scored 80% or better on tests of phonology, lexico-semantics, and grammatical knowledge, showing close to native-like proficiency in both (L2) and (L3). Multilinguals’ self-reported proficiency across languages was very high and each participant reported prolonged immersion in at least two second-language environments. By our accounts, these multilingual subjects exhibit a high degree of second and third language expertise and evidence significant immersion in their respective language environments. Based on the multilingual profiles obtained in this study, we may expect neural changes to be commensurate with those reported for highly proficient bilinguals when compared to monolingual controls.
Morphometry Data
Our study yielded several interesting results. Compared to their monolingual peers, highly proficient speakers of multiple languages exhibit select volumetric differences in posterior brain regions and no evidence of differences in the frontal regions of interest. These results are consistent with our hypothesis, following predictions of the BAPSS theory, which argues that increasing second language proficiency and language expertise is associated with greater recruitment of posterior regions and weaker reliance on the frontal regions. As established by our behavioral measures these multilingual participants show evidence of high proficiency in L2 and L3. However, contrary to what we hypothesized, there were no statistical group differences observed in subcortical ROIs. Moreover, as discussed below, the posterior volumetric changes indicate reductions in volume compared to monolinguals.
The two cortical brain regions that survived correction for multiple comparisons were the right AG in the inferior parietal lobe and the precuneus of the left SPL. Both the SPL (precuneus) and the AG have been largely implicated in non-linguistic, goal-directed behaviors (e.g., Duncan, Reference Duncan2010; Fedorenko, Reference Fedorenko2014; Goulden et al., Reference Goulden, Khusnulina, Davis, Bracewell, Bokde, McNulty and Mullins2014) but show consistent co-activation during different linguistic tasks (Fedorenko & Thompson-Schill, Reference Fedorenko and Thompson-Schill2014).
Parietal brain regions have been consistently shown to be involved in lexico-semantic processing and processing of the phonological features of newly acquired lexical items (e.g., Pliatsikas, Reference Pliatsikas2019; Richardson et al., Reference Richardson, Thomas, Filippi, Harth and Price2010) as well as processes involved in task maintenance control (Green & Abutalebi, Reference Green and Abutalebi2013). While the left AG has been implicated in a wide range of linguistic processes, especially those involving semantics (Obleser & Kotz, Reference Obleser and Kotz2010; Vigneau et al., Reference Vigneau, Beaucousin, Hervé, Duffau, Crivello, Houdé, Mazoyer and Tzourio-Mazoyer2006), reports of right AG in language use are less frequent. More recently, the bilateral AG has been shown to be involved in focusing attention on memory when information in memory has to be evaluated internally and has associated this region with the default mode network (Cabeza et al., Reference Cabeza, Mazuz, Stokes, Kragel, Woldorff, Ciaramelli, Olson and Moscovitch2011; Sormaz et al., Reference Sormaz, Murphy, Wang, Hymers, Karapanagiotidis, Poerio, Margulies, Jefferies and Smallwood2018a, Reference Sormaz, Murphy, Wang, Hymers, Karapanagiotidis, Poerio, Margulies, Jefferies and Smallwood2018b).
One of the most comprehensive models describing the neural correlates of the processes involved in bilingual language control – namely the Adaptive Control Hypothesis (Abutalebi & Green, Reference Abutalebi and Green2016; Green & Abutalebi, Reference Green and Abutalebi2013) – implicated the parietal cortices (including the AG) in goal maintenance tasks. A multilingual speaker must maintain a task goal of speaking in one of the known languages rather than another in any interactional context. Interactional contexts may be very complex for speakers of multiple languages. All multilingual participants use their native language at home, with some family members, and interchangeably the native language and the later learned L2 (English) with other family members. They also use the later-learned L2 (English) in other social contexts, such as school, work, etc. The early L2 (Russian) and other early learned languages are used with friends, or in specific work environments. Consequently, the demands for each of these contexts may vary greatly for multilinguals compared to bilinguals, requiring some switching and a mixture of two or three of these languages with friends that come from the same background. Maintaining a task goal requires control processes that control interference from different cues in conversations that may activate the goal of speaking in another language. Hence, processes of task goal maintenance may factor significantly in multilingual speakers and may lead to the restructuring of the parietal regions implicated in this function.
The precuneus has been previously reported to be associated with semantic and lexico-phonological processing (Burgaleta et al., Reference Burgaleta, Baus, Díaz and Sebastián-Gallés2014). Activity in the precuneus in lexical tasks was sensitive to word imageability (Bedny & Thompson-Schill, Reference Bedny and Thompson-Schill2006) as well. Halsband (Reference Halsband2006) showed bilateral precuneus activation for a memory retrieval task, in which participants had to retrieve high imagery word pairs in two languages – i.e., Finnish (native) and English (second language) – spoken by the participants. Left precuneus activation was specifically shown for the non-native language during the retrieval of low imagery content. The naming of low imagery stimuli may necessitate a greater reliance on abstract semantic attributes. The involvement of the precuneus in verbal memory retrieval was further corroborated by Bhattasali et al. (Reference Bhattasali, Fabre, Luh, Al Saied, Constant, Pallier, Brennan, Spreng and Hale2019), that showed precuneus activation during the retrieval of multi-word expressions (a.k.a., word sequences that are treated as a unit by the speaker). The retrieval of non-compositional multi-word expressions may tax semantic memory systems. Compared to monolinguals, multilingual abilities establish a greater number of lexical-semantic associations. Demands for the retrieval of this dense information may engender cortical changes. The morphometric changes within the precuneus may reflect increased recruitment of this area to support semantic retrieval processes across multiple languages.
Directions of structural GM changes
We have additionally examined the direction of expected changes within posterior and subcortical regions. The manifestation of normalization in brain GM related to high proficiency and immersion in a second language is poorly understood in the literature. Investigations of highly experienced sequential bilinguals have reported both increases (Burgaleta et al., Reference Burgaleta, Sanjuán, Ventura-Campos, Sebastian-Galles and Ávila2016) and decreases (Pliatsikas et al., Reference Pliatsikas, DeLuca, Moschopoulou and Saddy2017) in GM for bilinguals compared to monolinguals.
The limited available evidence coming from high proficiency bilinguals through time, with increases in immersion and proficiency (namely, DeLuca et al., Reference DeLuca, Rothman and Pliatsikas2019) showed decreases in the volume of subcortical structures at three-year follow-up visit scans, compared to the initial scan. The authors interpret these effects to indicate increased efficiency in second language use and control. Our present results suggest that the normalization processes associated with high proficiency and immersion in second language expertise may manifest in values that fall below levels observed in monolingual speakers. Current models of brain restructuring that result from language experience may need to be expanded to account for these possibilities.
Several studies have indicated that decreases in cortical thickness may be a signature of more efficient processing. Porter et al. (Reference Porter, Collins, Muetzel, Lim and Luciana2011), reported correlations between task fMRI (for the Controlled Oral Word Association Test) and decreased cortical thickness in regions involved in verbal fluency. These regions included the left inferior parietal gyrus (i.e., the supramarginal (SMG) and AG), and the superior temporal regions. Studies that performed combined structural and functional neuroimaging have reported higher functional activation in thinner cortices in supratemporal areas on the right auditory core/belt during basic auditory perception (Liem et al., Reference Liem, Zaehle, Burkhard, Jäncke and Meyer2012). Similarly, GM morphology decreases (i.e., in the left STG and bilateral fronto-parietal regions) have been also reported as a result of non-linguistic cognitive training, such as intensive working memory (Takeuchi et al., Reference Takeuchi, Taki, Sassa, Hashizume, Sekiguchi, Fukushima and Kawashima2011). Lastly, synaptic pruning resulting in decreases in GM has been proposed as a potential mechanism associated with brain maturation and tuning of brain regions’ communication (Giorgio et al., Reference Giorgio, Watkins, Chadwick, James, Winmill, Douaud, De Stefano, Matthews, Smith, Johansen-Berg and James2010). One further notes that simultaneous interpreters have been reported to have decreased GM volume and thickness in several different areas, including the left IPL, ACC, IFG, and bilateral caudate nucleus (Elmer et al., Reference Elmer, Hänggi, Meyer and Jäncke2011). These authors interpret the results to suggest improved functional specialization, leading to more efficient processing, as a result of neuronal pruning.Footnote i
Correlational analysis with additional demographic variables indicated that differences in cortical thickness on the AG were not correlated with biological sex or education while a weak correlation was noted with age. However chronological age did not differentiate between subject groups. Similarly, no demographic variables of interest (education, biological sex, or age) were associated with left hemisphere precuneus GM thickness. Note, the multilingual group discussed in this study has acquired multiple languages to almost native-like proficiencies and has used these across their lifespan. Being highly immersed in high code-switching multilingual environments may have led to continuous adaptations within regions involved in language processing. The reductions in GM thickness observed in these highly proficient multilinguals may indicate that the normalization of brain regions’ GM does not only revert to similar values seen in the monolingual brain but instead falls below regional values measured in monolingual speakers.
Limitations
One of the main limitations of our study was the lack of a bilingual group, for additional comparisons with the multilingual group. While the presence of a bilingual group would have allowed us to understand the neuroplastic effects that multilingual experience may induce beyond bilingualism, we considered it important to look at differences across multilinguals and the traditionally used monolingual speakers. While most of the bilingual literature, as well as neurolinguistic models of bilingualism, are predicated on comparisons between bilinguals and monolinguals, to our knowledge, there is only one study looking at multilingual adults compared to monolinguals (i.e., Abutalebi et al., Reference Abutalebi, Della Rosa, Gonzaga, Keim, Costa and Perani2013).
Another limitation of this study is the number of participants. Limitation in power in MRI studies often leads to inconsistencies in findings (Munson & Hernandez, Reference Munson and Hernandez2019), as well as limitations in identifying effects of interest. Hence, further research with groups comparable to this study's participants is needed for a better understanding of grey matter changes associated with high proficiency in multiple languages.
Differences in brain structure may be affected by environmental, genetic, and cultural factors. Indeed studies reported WM and GM differences for participants of different cultures (e.g., Tang et al., Reference Tang, Zhao, Lou, Shi, Fang, Lin, Liu and Toga2018); however, the intracranial volume did not differ across groups in our study. Nonetheless, more research is needed to identify the contribution of the specific factors contributing to brain morphometry differences across groups.
Lastly, although generally interpreted as such, a lack of observed changes in certain brain areas may not be interpreted as a lack of change. Due to the limitation of the MRI techniques, combined methods (i.e., structural, functional, network-based, etc.) should be used for a more comprehensive account of experience-based effects on brain function and structure.
Conclusion
This study provides unique evidence of brain plasticity associated with expertise in multiple languages. The group of multilinguals investigated in this study provided unique characteristics that allowed specific predictions testing of recent neurolinguistic models of brain restructuring in multilinguals. Participants have a life-long experience with multiple second languages and have demonstrated high proficiency in at least three languages. We identify two main areas that show robust decreases in grey matter morphometry for the multilinguals compared to the monolinguals – namely, the AG and the precuneus. The results of the present study indicated that individuals with high proficiency in multiple languages, and immersed in highly complex linguistic contexts compared to baseline monolinguals, may show evidence of decreased GM thickness in regions supporting goal maintenance and memory retrieval.
Supplementary material
The supplementary material for this article can be found at https://doi.org/10.1017/S1366728923000445
Acknowledgements
We would like to thank Dr. Costin Tanase for his help in suggesting imaging protocols and data analysis practices, and Sharon Coffey Corina for helping throughout the length of the project. Thanks to Diana Malancea-Malac for helping with the behavioral and MRI data collection and organization and to Cristina Sewell for helping with coding and software installation. Additional thanks to Charles D. Arnold for advising with statistical analysis. This research was supported by the UC Davis Imaging Research Center Pilot Program.
Competing interests
The authors declare none.
Data availability
The data that support the findings of this study are available in the OpenNeuro repository, at the link: doi:10.18112/openneuro.ds004581.v1.0.0