‘Cue reactivity’ has been discussed extensively in literature relating to drug use. It appears that even brief exposure to the sight of drugs or drug-taking paraphernalia (a ‘cue’) can elicit a physiological reaction and increased craving in addicts(Reference Carter and Tiffany1). Of course, only drug users experience drug-cue reactivity. By contrast, all of us eat and we all encounter food-related cues in our everyday lives. Importantly, targeted clinical comparisons, such as those involving binge eaters and bulimics, indicate that individual differences exist in food-cue reactivity(Reference Sobik, Hutchison and Craighead2, Reference Carter, Bulik, McIntosh and Joyce3). Despite this, our understanding of cue reactivity in non-clinical populations is poor. In particular, it remains unclear whether levels of food-cue reactivity represent a potential risk factor for overweight and obesity. In part, these unresolved issues arise because we have lacked basic research identifying the specific effects of food-cue exposure on food choice and meal size. This paper addresses aspects of this shortcoming.
Previously, researchers have focused on three different consequences of food-cue exposure. First, it influences subjective appetite for food(Reference Rogers and Blundell4–Reference Oakes and Slotterback6). Second, it initiates physiological changes, including a release of insulin(Reference Teff, Mattes and Engelman7) and changes in salivation, body temperature, heart rate, gastric activity and blood pressure(Reference Nederkoorn, Smulders and Jansen8, Reference Brunstrom, Yates and Witcomb9). These conditioned ‘cephalic phase responses’ are important because they prepare the body for the consumption, digestion and absorption of nutrients(Reference Nederkoorn, Smulders and Jansen8, Reference Mattes10, Reference Rodin11). The third effect of food-cue exposure relates to the amount of food that is consumed. Fedoroff et al. found that participants consumed significantly more pizza if they were exposed to the smell of baking pizza shortly before meal initiation(Reference Fedoroff, Polivy and Herman5). From a health perspective, this consequence is particularly important, because especially reactive individuals may be disposed to eat larger meals and, consequently, may be at greater risk of weight gain.
Previously, studies have focused on the effects of cue exposure on subsequent ad libitum food consumption (e.g. Fedoroff et al. (Reference Fedoroff, Polivy and Herman5)). In this context, participants are offered ‘limitless’ food and are instructed to eat until they no longer wish to continue. However, the extent to which individuals normally consume food in this way has recently been questioned. Instead, it has been suggested that meal size is often determined before a meal begins (or at least at discrete time points during a meal)(Reference Brunstrom, Shakeshaft and Scott-Samuel12). It remains to be determined whether cue exposure influences this kind of meal ‘planning’. More generally, this distinction between planned (active) and unplanned (passive) eating raises questions about the specific process by which cue exposure increases food consumption. In the present study we explored three possibilities.
Our first hypothesis was that cue exposure increases the amount of food that people want and plan to eat. Accordingly, we devised a methodology that enabled us to take precise measures of prospective intake, both before and after a period of cue exposure. Our second hypothesis was based around the concept of tolerated portion size. We reasoned that ‘ideal’ (planned) meal size might be decided before a meal begins. However, a mismatch will occur when an individual is offered a food portion that is larger than their prospective ideal. When the portion size is fixed and outside our control (e.g. when it is ordered from a restaurant or when it is pre-packaged) we may choose to tolerate or reject the larger portion outright. Accordingly, we explored the prospect that cue exposure increases intake because it increases our tolerance of larger portions.
Our final hypothesis was that cue exposure operates via a ‘passive’ process that arrests the development of satiety as a meal progresses. If this is the case, then the effects of exposure will be evident only after the meal begins and not in the decision-making that takes place before its onset. To test this idea, we exposed participants to either a food cue or no food cue and then gave participants a fixed portion of food (pizza) to consume. The participants were then asked to provide a measure of their hunger. We reasoned that if cue exposure delays the development of satiation, then participants should report relatively greater hunger in the food-cue condition.
A secondary aim was to consider the extent to which the effects of cue exposure are specific or general. In both animals(Reference Weingarten13) and human subjects(Reference Fedoroff, Polivy and Herman14, Reference Cornell, Rodin and Weingarten15), cue exposure is thought to promote eating of the cued food but to have little effect on motivation to consume non-cued foods(Reference Fedoroff, Polivy and Herman14). However, a potential problem with these studies is that very different kinds of foods have been used (e.g. cookies and pizza). Hence, it remains unclear whether evidence for specificity necessarily requires very dissimilar foods to be compared. In the present study we explored this issue by comparing the effects of cue exposure (desire to eat and prospective portion size) in both the cued food and a range of similar and dissimilar foods. We used hot pizza as the cued food and ‘cake’, ‘pasta and tomato sauce’ and ‘scrambled eggs, chips and baked beans’ as non-cued foods.
In addition to assessing the specific consequences of cueing, a final objective was to explore individual differences in reactivity. Previously, some researchers have found heightened reactivity in restrained eaters(Reference Fedoroff, Polivy and Herman5, Reference Fedoroff, Polivy and Herman14), while others have failed to observe this relationship(Reference Nederkoorn and Jansen16). These different outcomes might be attributed to the different measures of restraint that have been used. To clarify this issue we included two measures of dietary restraint, the Dutch Eating Behaviour Questionnaire (DEBQ)-restraint scale(Reference Van Strien, Frijters, Vanstaveren, Defares and Deurenberg17) and the Revised Restraint Scale(Reference Herman and Polivy18). Based on previous work, we also included a measure of trait-based impulsivity, dietary disinhibition(Reference Tetley19) and a measure of food craving that may be associated with externally induced eating(Reference Burton, Smit and Lightowler20).
Method
Overview
Each participant was tested on two separate days. On one day, they were exposed to the sight and smell of a freshly baked pizza (the ‘cue’ condition). On the other day, they took part in a simple cognitive task (the ‘no cue’ condition). The ordering of the cue and the no-cue condition was counterbalanced across participants. In both conditions, participants provided a measure of their ideal prospective pizza portion size and the maximum pizza portion that they would tolerate both before and after the cue/cognitive task. At the same time, measures were taken of prospective portion size and desire to eat, both for pizza and for three non-cued foods/meals: cake; ‘pasta and tomato sauce’; ‘scrambled eggs, chips and baked beans’. Non-cued foods were included to determine whether the effects of pizza exposure are specific to measures relating to pizza. In both conditions, the participants were then given a fixed portion of pizza to consume. Once they had eaten this portion, they were asked to complete ratings of hunger and fullness. Afterwards, they were instructed to consume pizza until they no longer wished to do so.
Participants
Fifty female participants aged between 18 and 62 (mean 28·30 (sd 10·86)) years were recruited from the staff and student populations of the University of Bristol (UK) using posters and email advertisements. Participants were excluded if they were vegetarian or vegan, had any food allergies or intolerances or if they reported a strong dislike for any of the four foods included in the study. Some students were awarded course credits and other participants were paid £20 (sterling) for their assistance. Our sample had a mean BMI of 22·9 (sd 3·4) kg/m2. Eight participants were overweight and forty-two were normal weight. Ethical approval was granted by the local Faculty of Science Human Research Ethics Committee.
Measures
Visual analogue scales
Desire to eat was measured using a 100-mm visual analogue rating scale with the title: ‘How strong is your desire to eat [food name inserted] right now?’ We also used similar scales to assess hunger, fullness and liking for the four foods. All of the scales were anchored with the phrases ‘not at all’ and ‘extremely’.
Prospective portion-size task
Prospective portion size was assessed using a computerised task. For each food, forty-one images were taken and these were numbered 1 to 41. Each food was photographed on the same white plate (255 mm diameter). For each food, picture number 21 corresponded with a ‘standard’ (average portion size). Information about typical portion sizes was obtained from Gregory(Reference Gregory21) or from nutritional information on product packaging (see Table 1). For cake, picture 1 and picture 41 represented 0·25 and four times the weight of the standard, respectively. For the other foods this range was limited by the amount of food that could physically be placed on the plate. For these foods, picture number 1 represented 0·3 times the weight of the standard and picture 41 was three times the weight of the standard. Across the range of pictures the portion sizes increased in equally spaced logarithmic steps.
Table 1 Standard portion sizes for the cued food and non-cued foods*

* For details of subjects and procedures, see Method.
† Calculated from manufacturers' recommended serving size.
‡ Derived from Gregory(Reference Gregory21).
All of the pictures were taken using a high-quality digital camera that was mounted directly overhead with fixed lighting. Particular care was taken to ensure identical lighting and arrangement of the plate across foods and portion sizes. Images (210 mm × 285 mm) were displayed on a 19″ TFT-LCD monitor. A horizontal scroll bar was presented at the bottom of the screen. When the participant used the mouse to move the scroll bar, the computer loaded a new image that corresponded to the new position of the scroll bar. Moving the scroll bar to the left caused the portion size to decrease (a smaller picture number was displayed). Moving it to the right caused the converse. The pictures were loaded on to the screen with sufficient speed that steady movements on the scroll bar gave the appearance that the change in portion size was ‘animated’. Each trial started with the ‘standard’ portion size being displayed and with the scroll bar located in the middle of its range. Participants were instructed to select the portion size that they would like to eat at that moment in time. The code for this task was written in Visual Basic (version 6.0).
Tolerance of pizza portion size
A second computerised task was developed to calculate the maximum amount of pizza that participants would tolerate before they would opt for a smaller-than-ideal portion size. The task is based on a similar psychophysical procedure that has been developed in our laboratory to explore expectations about the satiating properties of different foods(Reference Brunstrom, Shakeshaft and Scott-Samuel12). Participants were presented with two pictures of pizza, one on the left of the screen and one on the right. The images were the same as those used in the ideal portion size task. Both were approximately 182 mm × 182 mm (actual displayed size) and they were presented immediately adjacent to one another (no gap between them), with a black border filling the rest of the screen. The screen resolution was 1280 × 1024. The picture on the left-hand side of the screen represented the ‘standard’ image. This was the photograph showing the closest correspondence to 40 % less than the ideal prospective pizza portion size that was selected by the participant in the prospective portion size task. The picture on the right-hand side of the screen was the ‘comparison’ image. The portion size in the comparison image changed across trials. The upper limit of this range was the largest portion size (picture 41) and the lower limit was set at 20 % below the ideal portion. Participants were told to imagine that they were going to be asked to consume all of one of the two portions (standard and comparison). They were instructed to press the right arrow on the keyboard if they would choose the comparison (right picture) and the left arrow if they would choose the standard (left picture).
We assumed that very large comparison portions would be rejected in favour of the smaller-than-ideal standard (i.e. they would not be tolerated). Conversely, in response to a small comparison portion, we anticipated that the comparison would be chosen much more often, especially when the comparison was similar to the ideal prospective portion size. Somewhere between these two extremes, the probability of choosing the standard or the comparison will be equal (both selected 50 % of the time). After a sufficient number of trials, it is possible to calculate this ‘point of subjective equality’ (PSE). Here, we used probit analysis to fit a sigmoid function to the associated probability density function. The PSE is important because it indicates the maximum amount of the comparison that would be tolerated before participants tend to choose the smaller standard. To significantly improve the efficiency of our procedure we chose to use the adaptive probit estimation algorithm(Reference Watt and Andrews22). With this approach, only a subset of the comparison range is tested. Adaptive probit estimation selects those stimulus levels that maximise the prospect of gaining information about the PSE. The selection of specific comparison values is updated as the participant proceeds through the trials and is based on an analysis of the recent history of responding. Using this approach we were able to derive a PSE within fifty-six trials. The adaptive probit estimation routine and the code for presenting the stimuli were both written in Matlab (version 12). The graphical interface was implemented using Cogent Graphics software (freeware).
Individual differences
Participants completed a study-specific, health-screening questionnaire, which was used to record their age, height, weight, smoking history, alcohol consumption and current medication. They also completed the impulsivity questionnaire from Eysenck's Personality Questionnaire(Reference Eysenck and Eysenck23), the trait food-craving questionnaire(Reference Cepeda-Benito, Gleaves, Williams and Erath24), the DEBQ(Reference Van Strien, Frijters, Vanstaveren, Defares and Deurenberg17), the Revised Restraint Scale(Reference Herman and Polivy18) and the disinhibition subscale of the Three Factor Eating Questionnaire(Reference Stunkard and Messick25).
Demand awareness
Participants were asked to complete an awareness questionnaire, which measured the extent to which they had acquired an understanding of the aims of the present study. Specifically, they were asked three questions: (1) ‘What was the aim of this experiment?’; (2) ‘Why did we test you on two separate days?’; (3) ‘We expected you to be hungrier and to eat more on one day, which one was it (day 1/day 2)?’.
Procedure
Participants were told that the purpose of the current study was to investigate the effects of mood on appetite for food. All attended two 60-min sessions, held between 11.30 and 14.30 hours. Sessions were scheduled for the same time of day and they took place no more than 1 week apart. Participants were asked to abstain from eating for a 3 h period before arriving for a test session. In so doing, our aim was to promote similar baseline measures of hunger, fullness and desire to eat across conditions. On arrival, participants were asked whether they had consumed food in the previous 3 h, provided written consent and then completed the visual analogue scales. Consistent with the cover story, they were then asked to fill out a questionnaire designed to measure mood (PANAS)(Reference Watson, Clark and Tellegen26) in both conditions. Participants then performed the ideal portion size task for the four foods. For each participant, the order of the foods was selected randomly. After the prospective portion size task, the participants completed the tolerance task and then rated their desire-to-eat the four foods.
In the cue condition, the participants were exposed to the sight and smell of a freshly cooked ‘Goodfellas deeply delicious loaded cheese pizza’ for 1 min (supplied by Green Isle Foods Limited, Naas, Co. Kildare, Republic of Ireland). In the no-cue condition, they completed a basic cognitive task in which they played a simulation of the game ‘snap’ on a computer. In this task, two pictures (non food) appeared on the screen simultaneously. Participants were asked to press the spacebar if the images were identical. After this 60-s period the participants were again asked to complete the prospective portion size task, the tolerance task and the desire-to-eat ratings.
Participants were then given a fixed portion of pizza to consume. This was equivalent to a quarter of a single cooked pizza (91 g; 951·9 kJ) and was the same kind that was used as a cue in the cue condition. After consuming the pizza, they were asked to complete hunger and fullness ratings and were then given free access to a further one and a quarter pizza (a standard cooked pizza weighs approximately 364 g; 3811·6 kJ). In both cases, the pizza was presented in bite-size pieces rather than in slices. This reduced the extent to which participants were able to remember the amount of pizza they had consumed, both within and across conditions.
At the end of the second session, the participants completed the remaining diet and personality questionnaires and a measure of height and weight was taken. Finally, the participants completed the awareness questionnaire.
Data analysis
All data were analysed using SPSS version 12.0.1 (SPSS Inc, Chicago, IL, USA) and Minitab version 13.32 (Minitab Inc., State College, PA, USA). Paired-samples t tests were used to explore whether there were any significant differences between baseline measures of hunger, fullness, liking and desire to eat across the cue and no-cue conditions.
To determine whether cueing had a significant effect on prospective portion size, for each food, we calculated two change scores. One of these change scores represented the difference between the responses made immediately before and after exposure to the food cue. The other change score represented the difference between the responses made immediately before and after exposure to the cognitive task. In the first instance, we conducted a four (food type: pizza; cake; ‘pasta and tomato sauce’; ‘scrambled egg, chips and beans’) × two (condition: cue; no cue) repeated measures analysis of covariance (ANCOVA) to determine whether there was a significant effect of condition on prospective intake. For each food, we then conducted a separate repeated measures ANCOVA. In so doing, our aim was to assess whether cueing specifically increases prospective intake of pizza. Since the dependent variables were change scores, the appropriate baseline estimate of prospective portion size was included as a covariate in each analysis. Pre-exposure hunger and pre-exposure liking were included as covariates when they significantly correlated with the dependent variable. There was no multicollinearity associated with the covariates that were entered into our ANCOVA, with the exception of an analysis of the effects of cueing on ideal (prospective) portion size for scrambled eggs, chips and baked beans. In this analysis, pre-exposure liking was significantly associated with the baseline estimate of prospective intake (r 0·455, P < 0·001). Therefore, the results from this test should be treated with some caution. In all of our ANCOVA, the homogeneity of regression slopes assumption was met.
In addition to prospective portion size, participants also provided a measure of their desire to eat. To analyse these desire-to-eat ratings, change scores were derived using the same procedure outlined earlier and these were submitted to a similar set of ANCOVA.
Data from the tolerance task can be analysed in two ways. In the first instance, we compared ‘absolute’ difference scores across conditions. These scores represent the difference between the amount of pizza that was tolerated before and after the exposure phase. Separate change scores were calculated for the cue and the no-cue condition. A potential complication here is that absolute difference scores will also reflect changes in ideal portion size, making it difficult to determine the independent effects of cueing on tolerance. As predicted, pilot testing indicated a consistent relationship between absolute difference scores and ideal prospective pizza portion size. Essentially, participants who chose larger ideal portions were generally willing to tolerate a larger deviation (in absolute terms) from their ideal. For this reason, we also chose to standardise all comparisons by deriving a measure of tolerance relative to ideal. This ‘relative’ measure was based on the ratio of tolerated to ideal portion size. Relative change scores were then calculated as follows:
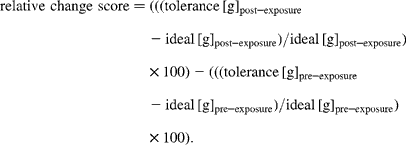
Absolute and relative change scores were submitted to separate repeated measures ANCOVA. The appropriate pre-exposure tolerance value was entered as a covariate in these analyses. Pre-exposure hunger and pre-exposure liking were included as covariates when they significantly correlated with the dependent variable.
Separate ANCOVA were also used to explore the effects of condition on hunger and fullness ratings after the participants consumed the fixed portion of pizza. Similarly, an ANCOVA was used to compare participants' ad libitum consumption of pizza. In each case, initial hunger and liking for pizza were entered as a covariate when they significantly correlated with the dependent variable.
To explore individual differences in cue reactivity, following previous work(Reference Fedoroff, Polivy and Herman5, Reference Fedoroff, Polivy and Herman14, Reference Tetley19), we allocated participants to ‘high’ or ‘low’ scoring groups based on a median split of scores on Eysenck's Personality Questionnaire(Reference Eysenck and Eysenck23), the trait food-craving questionnaire(Reference Cepeda-Benito, Gleaves, Williams and Erath24), the Revised Restraint Scale(Reference Herman and Polivy18), the disinhibition subscale of the Three Factor Eating Questionnaire(Reference Stunkard and Messick25) and the three subscales of the DEBQ(Reference Van Strien, Frijters, Vanstaveren, Defares and Deurenberg17). Participants were also allocated as being high (>25) or low ( ≤ 25) BMI. Thus, participants were categorised as high or low scoring on eight different measures. For each of these dichotomised measures we repeated the set of ANCOVA outlined earlier. Each time, a different measure was entered as a between-subjects factor. To reduce the likelihood of a type I error we decided to only consider change scores relating to pizza (i.e. the cued food only) and applied a Bonferroni correction (the adjusted α level was 0·0021).
Results
Baseline measures
Initially, we were interested to determine whether there were any significant differences between baseline hunger, fullness, liking and desire-to-eat ratings across the cue and no-cue conditions. Table 2 shows the means and standard deviations for these variables. Values are provided for the cue and no-cue conditions, separately. Paired-samples t tests revealed no reliable differences between the two conditions.
Table 2 Baseline ratings of hunger, fullness, liking and desire to eat, in each condition*
(Mean values and standard deviations)

* For details of subjects and procedures, see Method.
Effects of cueing on desire to eat
Fig. 1 shows the mean and standard error of the mean change in the desire-to-eat ratings in the cue and no-cue conditions. Participants experienced a significantly greater increase in their desire to eat pizza in the cue condition relative to the no-cue condition (F 1,48 14·28, P < 0·001). There was no significant effect of condition on the change in desire to eat pasta and tomato sauce (F 1,48 0·42, P = 0·518) or scrambled egg, chips and beans (F 1,48 1·04, P = 0·314). However, participants experienced a significant decrease in their desire to eat cake in the cue condition relative to the no-cue condition (F 1,48 7·38, P = 0·009).

Fig. 1 Mean and standard error of the mean change in desire to eat (mm) in the cue condition (■) and in the no-cue condition (□).
Effects of cueing on ideal (prospective) portion size
Fig. 2 shows the mean and standard error of the mean change in prospective portion for the cue and no-cue conditions. Change in prospective portion size was significantly greater in the cue condition than in the no-cue condition (F 1,143 11·01, P = 0·002). However, this main effect was qualified by an interaction with food type (F 3,143 3·39, P = 0·020). To explore this interaction further, we considered the effect of condition in each food separately. Participants reported a significantly greater change in prospective pizza portion size in the cue condition relative to the no-cue condition (F 1,47 8·33, P = 0·006). However, the same was also the case for scrambled egg, chips and beans (F 1,46 4·73, P = 0·035). There were no significant differences between the change in prospective portion sizes for cake (F 1,48 2·10, P = 0·154) or for pasta and tomato sauce (F 1,48 0·99, P = 0·324).

Fig. 2 Mean and standard error of the mean change in prospective portion size (g) in the cue condition (■) and in the no-cue condition (□).
Effects of cueing on tolerance of larger pizza portion sizes
Table 3 shows the unadjusted means and standard deviations for participants' estimate of their tolerance of pizza portion sizes. Across conditions, we found no significant differences between absolute (F 1,38 2·82, P = 0·101) or relative (F 1,38 1·04, P = 0·314) tolerance values.
Table 3 Ratings of absolute (g) and relative (% above the ideal) tolerance scores in each condition*
(Mean values and standard deviations)

* For details of subjects and procedures, see Method.
Effects of cueing on pizza intake and on hunger and fullness after consuming a fixed portion of pizza
Table 4 shows the mean and standard error of the mean hunger and fullness rating after the participants consumed a fixed portion of pizza and the mean amount of pizza that was subsequently consumed in the ad libitum meal. Separate values are given for the cue and no-cue condition. Across conditions, there were no significant differences in hunger (F 1,48 0·07, P = 0·793) or fullness (F 1,48 0·72, P = 0·401). However, participants did consume significantly more pizza (on average 25 g more; 261·5 kJ) in the cue condition than in the no-cue condition (F 1,48 6·08, P = 0·017).
Table 4 Pizza intake, hunger and fullness ratings (0–100), (after the fixed portion size) in each condition separately*
(Mean values with their standard errors)

* For details of subjects and procedures, see Method.
Individual differences
There was an interaction between DEBQ-restraint status (restrained/unrestrained) and condition for change in prospective pizza portion size, which was significant even after applying the Bonferroni correction (F 1,44 10·60, P = 0·002). Unrestrained eaters reported a greater change in prospective pizza portion size in the cue condition relative to the no-cue condition. By contrast, cueing had little effect on ideal pizza portion size in restrained eaters (see Fig. 3). We found a similar pattern of results using the Revised Restraint Scale. However, in this case, the interaction term was not reliable (F 1,45 3·13, P = 0·084). All other interaction terms failed to reach significance.

Fig. 3 Mean and standard error of the mean change in prospective pizza portion size in unrestrained (□) and restrained (■) eaters (based on median split of the Dutch Eating Behaviour Questionnaire restraint scores). Separate values are given for data from the cue and the no-cue condition.
Post hoc, we compared ad libitum intake of pizza in restrained and unrestrained eaters and found that unrestrained eaters ate significantly more pizza than restrained eaters, both in the cue condition (F 1,46 5·72, P = 0·021) and the no-cue condition (F 1,46 4·79, P = 0·034). Further analyses revealed that our measure of prospective intake was a highly reliable predictor of actual pizza consumption in unrestrained eaters in the cue condition (r 0·65, P = 0·001). However, the same comparison failed to reach significance in restrained eaters (r 0·31, P = 0·14).
Demand awareness
Responses to the awareness questionnaire are summarised in Table 5. These suggest that the majority of participants were unaware of the overall purpose of the experiment (question 1: 90 % unaware) and why they were tested on two separate days (question 2: 94 % unaware). Those who were ‘unaware’ tended to believe that the purpose of the experiment was to explore the effect of mood on appetite for food. We included a third question to assess whether participants knew that we expected them to be hungrier and to eat more on the cue day. Analysis of the frequencies associated with Table 5 suggest that this is unlikely to be the case (, P = 0·23).
Table 5 Summary of responses to the awareness questionnaire*

* For details of subjects and procedures, see Method.
Discussion
Our primary aim was to explore the process by which food cue exposure promotes overeating. We proposed three different mechanisms: (1) exposure increases the amount of food people plan to consume; (2) exposure increases tolerance of larger portion sizes; (3) exposure delays the normal development of satiation. Cueing had little effect on tolerance of larger portions (hypothesis 2), indicating that the effects of the cue are not dependent on the presence of portion sizes that are necessarily larger than ideal.
Relative to the no-cue condition, our participants reported a significantly greater increase in their ideal prospective pizza portion after the exposure period. Therefore, the current findings appear to share greatest consistency with our first hypothesis, that cueing increases the amount of food that people plan to consume. This is the first study to show that cue exposure influences a measure of prospective intake. However, a potential weakness in our approach is that we are unable to claim with certainty that participants actually executed their plans about ideal portion size and that the effects of cueing on ideal portion size are instrumental in the differences in food intake that were observed. However, there are good reasons to believe that planning played a causal role. First, we found no evidence that our participants were more hungry and less full in the cue condition after consuming a fixed portion of pizza (hypothesis 3). Therefore, it would seem unlikely that differences in intake should otherwise be attributed to the relative effects of the exposure period on the development of satiation in each condition. Second, consistent with the notion that planned intake influenced actual intake, we found a close correspondence between our measure of ideal portion size and actual intake, albeit to a greater extent in unrestrained eaters. Finally, other sources indicate that satiation signals offer relatively weak control over meal size. For example, amnesic patients will tend to consume very large amounts of food when they fail to encode recently consumed meals(Reference Higgs27). Similarly, participants with self-refilling soup bowls will tend to consume much larger meals(Reference Wansink, Painter and North28), indicating a relatively weak role for internal signals in the determination of meal size and, by implication, an important role for decision making and planning.
In future, it may be possible to address this shortcoming by exploring the relative contribution of meal size planning and the ‘passive’ effects of cueing on satiation using a paradigm that disrupts an individual's capacity to execute a planned meal. For example, in the context of Wansink et al.'s (2005) self-refilling bowl, we would predict that the effects of cueing would be diminished, because participants will experience a degraded capacity to ingest their planned meal size.
As noted earlier, we found no effects of cueing on tolerance of larger pizza portion sizes. Nevertheless, the data from this measure are noteworthy. To our knowledge, this is the first study to attempt to quantify portion size tolerance. In particular, it is striking that our participants were prepared to tolerate portion sizes that were much larger than their ideal. Specifically, when confronted with the prospect of consuming a portion 40 % less than ideal, participants indicated that they would be prepared to consume a portion that was 27–38 % larger than their ideal. Further research is needed to explore the extent to which foods are routinely ‘over consumed’ when they are served in portions that are tolerable yet greater than ideal. It would also be interesting to explore the extent to which individuals differ in their degree of tolerance and whether this promotes overeating, leading to a positive energy balance and a higher BMI.
A secondary aim of the study was to explore the specificity of the effects of cueing. Weingarten argued that cueing specifically increases motivation to seek out the particular food that is cued (in this case pizza) rather than any other food(Reference Weingarten13). He demonstrated that rats will wait for the specific food that is signalled by a cue even if other food is available. The present desire-to-eat ratings are consistent with this observation since desire to eat pizza increased in the cue condition and desire to eat the non-cued foods remained very similar or decreased. By contrast, in the no-cue condition, the ratings of desire to eat remained very similar, both in the cued food and the non-cued foods. However, we found less evidence for cue specificity in the prospective portion size estimates. Specifically, cueing increased prospective portion size of both the cued food (pizza) and also a combination of non-cued foods, ‘scrambled egg, chips and baked beans’. Perhaps this result is not unsurprising given that generalisation is commonplace in other forms of associative learning(Reference Wickens29). Individuals are commonly exposed to many different varieties of a food. In this context, generalisation is potentially more economical because it obviates the need to form specific associations with each brand, variety and so on. Indeed, one possibility is that exposure to one food brings about an increase in ideal portion size for all foods that share common super-ordinate characteristics such as savoury, sweet, hot and so on. This idea resonates with findings in the literature on sensory-specific satiety. In particular, studies suggest that after consuming a sweet food, other sweet foods also decline in pleasantness, whereas savoury foods remain unaffected(Reference Rolls, Vanduijvenvoorde and Rolls30). More generally, the prospect of cue generalisation would appear at odds with the work of Fedoroff et al. (Reference Fedoroff, Polivy and Herman14). However, as noted earlier, Fedoroff et al. compared very dissimilar types of foods (cookies and pizza) and so it is perhaps unsurprising that generalisation was not observed in their study.
Finally, the present study revealed evidence that individuals differ in their reactivity to food cues. Based on DEBQ-restraint scores, we found that cueing increased prospective intake in unrestrained eaters but had very little effect on the prospective appetite of restrained eaters. Our finding that restrained eaters have reduced reactivity contradicts the work of Fedoroff and colleagues(Reference Fedoroff, Polivy and Herman5, Reference Fedoroff, Polivy and Herman14) but is consistent with the work of Nederkoorn & Jansen, who recorded greater physiological responsivity in unrestrained eaters(Reference Nederkoorn and Jansen16). These researchers speculated that restrained eaters control their intake using cognitive suppression to constrain cue-induced physiological reactivity. However, this hypothesis remains to be tested empirically.
In conclusion, this is the first study to assess the effects of food-cue exposure on both prospective and actual intake. The current data show that cueing influences prospective intake of both the cued food and other non-cued foods that share similar characteristics. Although a causal relationship would seem likely, further research is needed to establish a direct effect of prospective meal planning on actual intake. In future it would also be interesting to explore the various effects of cueing in a larger sample. Research of this kind might reveal individuals who are at risk of weight gain because they experience high levels of reactivity and consequently consume larger meals.
Acknowledgements
This research was supported by a grant from the Economic and Social Research Council (RES-000-22-1745) on individual differences and food-cue reactivity, awarded to J. M. B. None of the authors has any conflict of interest.