No CrossRef data available.
Article contents
INVESTIGATING ATTRIBUTE RISKS AND CONSTRUCTING LINKAGE ERROR MODELS FOR PROBABILISTICALLY-LINKED DATA
Part of:
Stochastic analysis
Published online by Cambridge University Press: 19 April 2021
Abstract
An abstract is not available for this content so a preview has been provided. As you have access to this content, a full PDF is available via the ‘Save PDF’ action button.
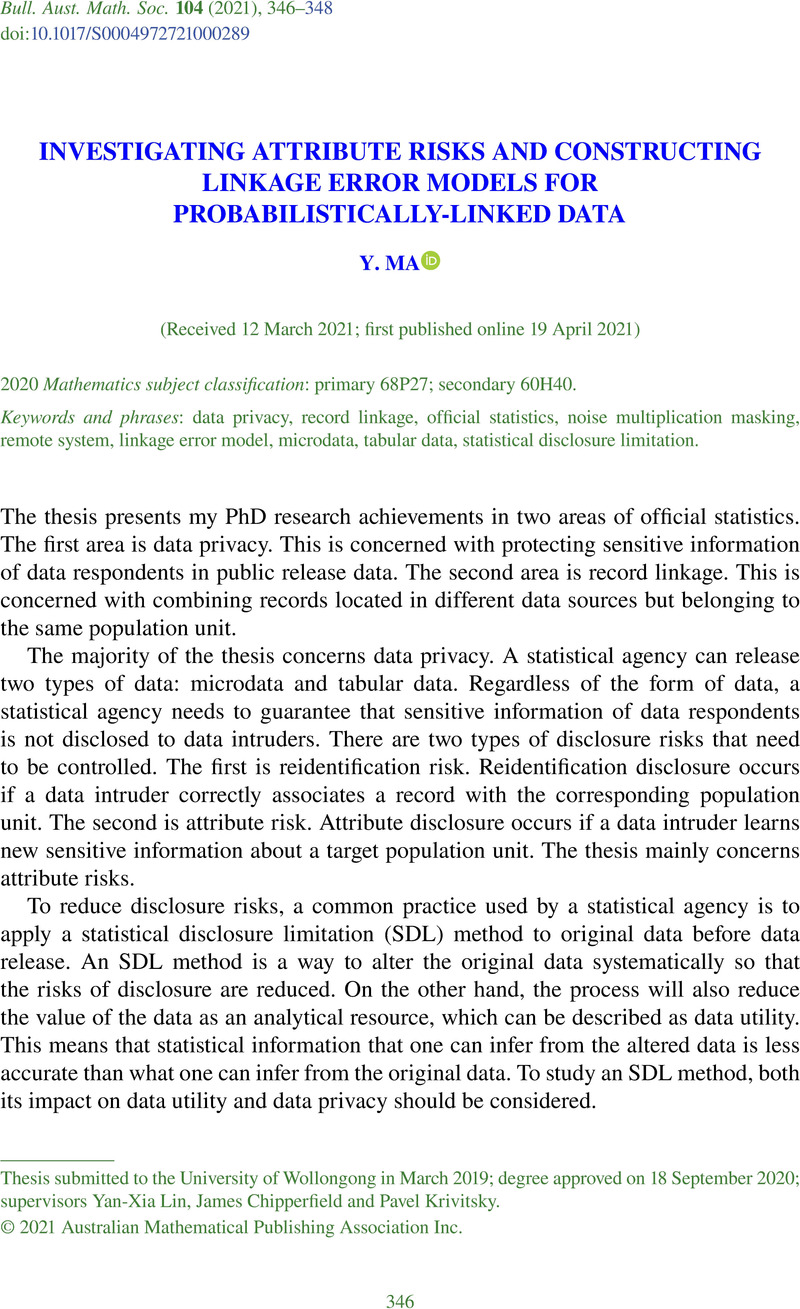
Keywords
MSC classification
Secondary:
60H40: White noise theory
- Type
- Abstracts of Australasian PhD Theses
- Information
- Bulletin of the Australian Mathematical Society , Volume 104 , Issue 2 , October 2021 , pp. 346 - 348
- Copyright
- © 2021 Australian Mathematical Publishing Association Inc.
Footnotes
Thesis submitted to the University of Wollongong in March 2019; degree approved on 18 September 2020; supervisors Yan-Xia Lin, James Chipperfield and Pavel Krivitsky.
References
Chambers, R., ‘Regression analysis of probability-linked data’, Statisphere Off. Stat. Res. 4 (2009).Google Scholar
Chipperfield, J. O. and Chambers, R. L., ‘Using the bootstrap to account for linkage errors when analysing probabilistically linked categorical data’, J. Off. Stat. 31(3) (2015), 397–414.CrossRefGoogle Scholar
Lin, Y. X., ‘Density approximant based on noise multiplied data’, in: Privacy in Statistical Databases, Lecture Notes in Computer Science, 8744 (Springer,
Cham, 2014), 89–104.CrossRefGoogle Scholar
Lin, Y. X. and Wise, P., ‘Estimation of regression parameters from noise multiplied data’, J. Priv. Confid. 4 (2012), 61–94.Google Scholar
Ma, Y., Lin, Y. X., Chipperfield, J., Newman, J. and Leaver, V., ‘A new algorithm for protecting aggregate business microdata via a remote system’, in: Privacy in Statistical Databases 2016, Lecture Notes in Computer Science, 9867 (eds. Domingo-Ferrer, J. and Pejić-Bach, M.) (Springer,
Cham 2016), 210–221.CrossRefGoogle Scholar
Ma, Y., Lin, Y. X., Krivitsky, P. N. and Wakefield, B., ‘Quantifying the protection level of a noise candidate for noise multiplication masking scheme’, in: Privacy in Statistical Databases 2018, Lecture Notes in Computer Science, 11126 (eds. Domingo-Ferrer, J. and Montes, F.) (Springer,
Cham, 2018), 279–293.CrossRefGoogle Scholar
Ma, Y., Lin, Y. X. and Sarathy, R., ‘The vulnerability of multiplicative noise protection to correlation- attacks on continuous microdata’, Sankhyā Ser. B 82 (2020), 305–327.CrossRefGoogle Scholar
Thompson, G., Broadfoot, S. and Elazar, D., ‘Methodology for the automatic confidentialisation of statistical outputs from remote servers at the Australian Bureau of Statistics’, in: Joint UNECE/Eurostat Work Session on Statistical Data Confidentiality (UNECE,
Ottawa, 2013).Google Scholar