Impact statement
Humans operating in extreme environments have unique needs that are often not addressed by traditional medical methods. Moreover, precision medicine (PM) applied to humans in extreme environments has unique requirements beyond those of PM in general practice. Among the goals of PM in extreme environments are to (1) identify individual deficits in essential and conditionally essential nutrients, (2) identify elements of the exposome with health and performance implications, (3) optimize metabolic networks, (4) personalize drug therapeutics to improve safety and efficacy, (5) improve diagnostic accuracy, (6) reduce unnecessary variations in diagnosis, (7) improve predictive ability regarding outcomes, (8) personalize preventive measures, countermeasures, and therapies, (9) improve performance, (10) improve recovery from training, competition, operations, and injury, (11) better match countermeasures with the operating environment, (12) improve operator decision-making, (13) improve team cohesion, (14) provide direction on intervention in cases where research is limited, (15) optimize career longevity, and (16) optimize health upon retirement. This approach will be important for astronauts, warfighters (Special Forces, aviators, etc.), athletes (football, basketball, baseball, soccer, track and field, cycling, motorsports, divers, alpinists/mountaineers, etc.), first responders (police, firefighters, emergency medicine clinicians), explorers/expeditioners, wilderness guides and medics, field scientists, and anyone whose occupation or recreation involves extreme operating conditions.
Introduction
Extreme operating environments can be characterized in terms of two domains of the allostatic load: environmental (context) and personal. The environmental domain describes an operating environment where the surroundings or setting are characterized by harsh environmental conditions that, by virtue of their extremes, place a unique burden on human thriving. Examples are extremes of temperature, altitude, atmospheric pressure, wind, biological threats, chemical threats, radiation, microgravity, hypergravity, and others.
The personal domain describes an operating condition where the surroundings are not extreme, but the human is subjected to extreme performance demands in a nonhostile physical environment. Examples include but are not limited to extreme levels of training, competition, athletics, work, risk, psychosocial stressors, team demands, isolation, or combat that stretch the limits of human tolerance or performance.
Fundamentally, extreme environments are commonly characterized by exposures that may include physical and psychological pressures, including uncertainty, danger, hardship, interpersonal pressure, isolation, sleep deprivation, and monotony (Chapman, Reference Chapman2018; Table 1).
Table 1. Examples of environmental and personal conditions as extreme operating environments

a Adapted from the CSA Standard Z1010-2018 Management of work in extreme conditions. It defines extreme condition as “existing in a very high degree; exceeding the ordinary, usual, acclimatized to, or expected condition.”
Precision medicine definition
For the purpose of this discussion, precision medicine (PM) is defined as the science and practice of providing methods of treatment and prevention tailored to an individual’s molecular, physiological, morphological, and behavioral characteristics. A related requirement is that the assessment and countermeasures must be aligned to the specific environment in which the individual is operating. Each environment may carry specific exposures, risks to injury, or risks to illness to which the countermeasures must also be tailored.
It is important to identify two concepts within the application of PM in extreme environments (though these are nuanced when applied to extreme environments): stratification and personalization. Stratification applies to groups of individuals, whereas personalization applies to a single individual. In our view, both stratification and personalization are foundational to PM in extreme environments. Stratification applies when patterns of variance are identified within a group from which a shared suite of countermeasures can be developed. This may apply to crews, teams, units, or other types of small and large groups. Personalization applies when a careful evaluation reveals a unique pattern of variance whereby countermeasures are tailored to one individual.
The term countermeasure is commonly used in extreme environment applications because these are methods that are not typically centered on the treatment of disease. While there are several related definitions of the term countermeasure, a useful definition for our purpose is solutions to prevent the undesirable physiologic outcomes associated with an extreme environment (Ploutz-Snyder, Reference Ploutz-Snyder2016). In general, PM countermeasures are intended to optimize individual and team (group, crew) functionality in any extreme operating environment (Figure 1).

Figure 1. Conceptual view of precision medicine (PM) in extreme environments. PM in extreme environments is rooted in deep molecular profiling and pattern analysis for the purpose of detecting patterns of variance in molecular networks. Once biological meaning is established, one can proceed to develop countermeasures that members of a team, crew, or unit may have in common (stratification). Stratification may be needed, for instance, if a team, crew, or unit shares a drug-metabolizing genotype (e.g., CYP4502D6 ultra-rapid metabolizers), wherein guidance on the drug formulary can be applied to a subgroup. Individual variances typically exist that are most appropriately addressed by individualized countermeasures (personalization). Individuals operating in extreme environments cover a wide spectrum, including but not limited to athletes, soldiers, astronauts, first responders, expeditioners, and a wide range of occupations. Image credit: Sovaris Aerospace.
What distinguishes PM in extreme environments?
One key distinguishing feature of PM in extreme environments is the requirement for optimal performance, which is not inherent to PM in general practice. Optimal performance is defined as the degree to which individuals achieve a desired outcome when completing goal-oriented tasks. By the nature of its difficulty, an extreme environment commonly renders the completion of specific tasks much more challenging (Paulus et al., Reference Paulus, Potterat, Taylor, Van Orden, Bauman, Momen, Padilla and Swain2009). Therefore, a smaller number of individuals may be capable of optimally performing a given task. In practice, PM must always attend to raising the individual’s capability to operate in pursuit of executing such tasks.
Performance is not only a requirement for proper execution in extreme environments, but it is often tightly linked to operational safety and even survival. Thus, PM applied to extreme environments must consider incremental factors that can influence endurance, psychomotor speed, cognition, decision-making, execution of tasks, response time, mood, and numerous behavioral dynamics. In addition, a foundational premise of operating in extreme environments is not only that one thrives in standard (or nominal) conditions, but that one be able to respond to emergencies (off-nominal) with often limited access to emergency services or needed resources, for example, a disabled vessel at sea or an injured team member in a remote setting.
Further distinguishing PM in extreme environments is that there is often insufficient research to guide clinical decisions. This is typically due to several factors: (1) crews, teams, or groups are small, (2) study burdens to crews/teams/individuals working on execution, (3) many such missions are the first of their kind and are not research focused, (4) some are tactical with no place for research, (5) many are low resource environments where additional gear for research is impractical, (6) considerable heterogeneity of operations, (7) some are conducted in protective gear where sample acquisition is difficult, and (8) funding is limited.
This often presents clinicians who support extreme operators with the challenge of acting on insufficient data derived from controlled studies. As a partial solution, PM allows one to conduct a formal gap analysis of each individual (and group) at the molecular level. It allows the clinician to piece together a road map derived from a series of functionally characterized networks (FCN; see below). It allows the clinician to assign biological meaning to these discrete patterns of variance, which can then be used as clinical decision support in constructing individualized countermeasures, so that these gaps are not carried into the operating environment. Thus, a foundational premise of PM in extreme environments is that knowable, actionable metabolic network deficits be addressed, so that they are not carried into the operating environment.
The critical role of recovery
Those engaged in extreme environments must always regulate load. External load consists of the work the individual does in training, missions, operations, or competition. Internal load consists of the physical, physiological, and behavioral response to the external load. The internal and external load can vary considerably between and within individuals.
This load must always be balanced with recovery, so that there is sufficient adaptive capacity to maintain health, fitness, and performance. Short-term recovery addresses the interval between training sets. It can also address the day-to-day recovery between training, competition, or operations. Long-term recovery addresses recovery across months, a year (season), or a career.
Inattention to recovery can lead to overtraining, overreaching, or overuse syndromes. Therefore, recovery must be built into training and clinical care paradigms. Recovery must also be assessed on a regular basis using a series of subjective, molecular, and physiological measures. A brief sample is noted and adapted below (Load, Overload, and Recovery in the Athlete, 2019):
-
• Laboratory: blood work (e.g., cortisol, CK, hemoglobin, ferritin, iron, testosterone, lactate, vitamin D, white blood cell count); urinalysis (e.g., hydration status); targeted and untargeted metabolomics.
-
• Questionnaires: psychological (e.g., POMS, REST-Q Sport, Pittsburgh Sleep Quality Index, scales that measure stress), perceived exertion (e.g., RPE), wellness scales (e.g., muscle soreness scale), nutritional (e.g., food journal, food frequency questionnaire); see Table 8.
-
• Nonwearable technologies: force plates, VO2max, imaging (e.g., MRI, ultrasound, qEEG), isokinetic testing, equipment/devices to measure velocity and power (e.g., Wingate test), video analysis systems, internal/external measurements of core temperature (e.g., ingestive thermometer).
-
• Wearable technologies: Global Positioning System (GPS), heart rate monitors, HRV devices, velocity (accelerometers), wearable motion-capture devices (e.g., wrist-worn and clothing-based technology); hydration sensors.
Balancing performance with health and well-being
The nature of operating in extreme environments requires that individuals constantly push toward and then beyond the limits of their capability. Once a new limit is set, it is the nature of these individuals to push beyond this new boundary to set yet a higher level of capability. This perpetual state of seeking ever-higher performance places considerable physical and psychological demands on individuals. The pursuit of these limits naturally includes efforts at enhancement, some of which can be strongly beneficial and others that can be detrimental (such as overtraining or the use of performance-enhancing substances). Thus, clinicians must be alert for and incorporate means of monitoring for signals indicating that the rigorous efforts directed at performance do not conflict with objectives for health and well-being.
Pattern analysis versus diagnosis
Standard PM is traditionally focused on prevention and diagnosis of disease with greater specificity, so that the intervention and disease management can be applied to an individual with greater precision. While this principle applies to PM in extreme environments, individual operators in these environments are often not in need of a diagnosis. Rather, they are healthy, fit, highly conditioned individuals who are focused on elevating performance to meet the demands of a given operating environment with high external loads, high internal loads, or both.
Since small decrements in performance can have a notable adverse influence on success in these environments, PM applied to extreme environments is focused heavily on identifying patterns of variance (change) in molecular networks that incrementally add up to degrade performance. In general, a foundation of PM in extreme environments is to (1) query a range of molecular networks, (2) identify patterns of deficiency or excess, (3) identify fixed patterns of biological vulnerability (e.g., genetic variants), (4) describe the biological meaning of these molecular findings, (5) quantify important features of the operating environment, and (6) tailor an intervention or training program to incrementally address patterns found in the individual and the environment.
The pattern analysis approach can be vital when attempting to elucidate the nature of a problem such as unexplained fatigue in a submarine crew, astronaut crew, crew at sea, or almost any occupation. Often, traditional diagnosis has ruled out primary causes such as thyroid disease, infection, depression, neurological disease, cardiovascular disease, renal disease, cancer, and others. The pattern analysis approach rooted in the surveillance of metabolic networks is a well-tested method to identify signals that can lead to actionable countermeasures.
When diagnosis is required
Beyond pattern analysis, humans operating in extreme environments are frequently exposed to conditions that apply unique burdens in allostatic load leading to conditions or diseases in need of precise diagnosis.
For example, cancer is a leading cause of death among firefighters. In a recent study, cancer incidence and mortality were 9 and 14% higher in the firefighter and nonfirefighting public, respectively. This included increased mortality rates of greater than 30% for mesothelioma and cancers of the rectum, intestine, and esophagus. Increased cancer incidence has also been reported for skin, lung, prostate, kidney, bladder, testes, and stomach (Jeong et al., Reference Jeong, Zhou, Griffin, Jacobs, Dearmon-Moore, Zhai, Littau, Gulotta, Moore, Peate, Richt and Burgess2018).
Cardiovascular disease remains the most common chronic medical condition affecting active-duty members of the military. In 2011, 18% of active-duty soldiers had been diagnosed with high blood pressure, while 15% of soldiers were diagnosed with high cholesterol (Poon et al., Reference Poon, Por, Cho and Oliver2021).
Vision is an important factor in astronaut and pilot selection, accounting for more than 40% of disqualifications of astronaut candidates. However, there is evidence that astronauts are at greater risk to cataract formation when compared to aviators with lower exposure to radiation (Cucinotta et al., Reference Cucinotta, Manuel, Jones, Iszard, Murrey, Djojonegro and Wear2001; Richardson, Reference Richardson2022).
In practice, one must commonly align the elements of pattern analysis directed at performance with standard diagnostic approaches associated with known or emergent disease processes.
Where does one begin in addressing PM needs in extreme environments?
While PM is commonly viewed from the perspective of selecting the right drug, at the right dose, for the right patient, the approach herein addresses an important first step that precedes, or at minimum, parallels drug selection. This is focused on the identification of actionable targets within metabolic networks (gap analysis) that may be responsive to optimized regulation by providing substrates, precursors, intermediates, and cofactors. Many of these take the form of macronutrients and micronutrients.
In many cases, correction of these functional errors of metabolism may obviate the need for drugs or modify drug selection in important ways. When drugs are utilized, it is done so with careful consideration of their impact on metabolic networks via attention to drug–gene, drug–drug, drug–herb, drug–food, and drug–nutrient interactions. Thus, the full impact of drug selection can be gauged in the context of how they will impact performance of metabolic networks and, in turn, the human performance phenotype.
The systems approach begins from the premise that dysregulation across molecular networks can create incremental losses in network efficiency. Dysregulation typically reveals itself in biomarkers or analytes that are outside nominal (high or low). It sometimes also means identifying stable genomic elements (e.g., SNPs) that might influence risk or indicate a need to address the related pathway(s) by other means. For example, those homozygous (−/−) for methylentetrahydrofolate reductase (MTHFR 677TT) may have a considerable increase in the need for riboflavin as the FAD precursor (fold-level, supraphysiologic doses), due to changes in binding affinity of FAD for the modified (variant) MTHFR enzyme (McNulty et al., Reference McNulty, McKinley, Wilson, McPartlin, Strain, Weir and Scott2002).
Since almost any combination of molecular network variance can contribute to this dysregulation, we can reduce complexity by examining these networks in a constrained manner. To do this, we need to utilize analytes to which we can assign biological meaning, which then guides a level of precision in the intervention.
This can be viewed as a nonhypothesis-driven, data-driven, or exploratory approach. In some circles, this might be deemed a form of screening that could subject the participant to unnecessary testing. For the general population with limited resources or a national health service with financial constraints, this is certainly a concern worthy of discussion. However, in the extreme operating environment where performance, health, safety, and even survival are foundational, one could argue that the exploratory, data-driven method is the ideal, perhaps even optimum, approach. This approach is always enhanced when the clinician is attentive to the risk of false discoveries and random associations, while aligning results with the clinical phenotype.
PM return on investment
From a cost–benefit perspective, it is useful to consider the cost to train a fighter pilot, Navy SEAL, Delta Force operator, NFL (American football), NBA (basketball), soccer, baseball, or Olympic athlete. In the professional realm, the investment in the athlete is so high that the costs themselves begin to aggregate every time an individual is underperforming or is unavailable to engage in a respective activity. Further, there is an additional and substantial cost to training a replacement. The same is true if careers are shortened due to underperformance, illness, or injury.
For instance, the cost to train a Navy SEAL is roughly 2 million dollars (USD), while it costs roughly $850,000 to keep a soldier overseas for 1 year (Shaughnessy, Reference Shaughnessy2012). The cost of training a basic qualified fighter pilot ranges from $5.6 million for an F-16 pilot to $10.9 million for an F-22 pilot. Bomber pilot training ranges from $7.3 million for a B-1 pilot to $9.7 million for a B-52 pilot (all USD). And it is generally considered that retaining the successful pilot pool is more desirable than expanding it (Mattock et al., Reference Mattock, Asch, Hosek and Boito2019).
Professional athlete pay scales are even more dramatic. For example, the 50 highest-paid athletes in the world earned a total of $2.97 billion (USD) in 2022 (Knight, Reference Knightn.d.). The top 20 salaries in major league baseball ranged from $25 M to $43 M annually (Dunn, Reference Dunn2022). The top 10 salaries in professional football ranged from $25 M to $50 M annually (ESPN Staff, 2023). The top 10 salaries in professional soccer ranged from $29 M to $130 M annually (Birnbaum, Reference Birnbaum2022).
For example, in 2015–2016, we (Schmidt and Penfold) developed and led the molecular profiling and individualized countermeasures program for the Golden State Warriors NBA basketball team when they set the NBA record for wins in a season (73-9). During that season, the Warriors ranked among the best (lowest) in the league in salary lost to injury as a percentage of total team salary. While causality was not directly studied, the association raises interesting questions that could be addressed in future prospective studies to further explore how such approaches might benefit high-performing individuals or groups.
In general, the molecular profiling costs (thousands to tens of thousands of dollars per individual) required to implement routine serial testing of an individual often pale in comparison to the training and maintenance expenditures common in many high-performance operating environments. In short, there is a case to be made that PM based on comprehensive molecular profiling is an important means to support an optimum return on investment by addressing gaps in molecular networks that might impact individual operator/performer health, recovery, performance, and availability. Admittedly, there are those operating in extreme environments who are well below the pay scales just described. This simply argues for continued efforts to reduce the costs associated with PM wherever possible and work to develop a suite of standard measures, as we have done with operating environments such as spaceflight.
A systems approach to optimizing molecular networks in PM
Because many who operate in extreme environments interface with complex engineered mechanical systems, there is value in using a shared language of systems engineering in describing the human system in relation to those systems. There is also value in this approach because engineers must integrate humans into their engineered systems (human systems integration being a subdiscipline of its own in these environments). Thus, the better engineers can understand the medical applications via a common language, the better they will be able to integrate the human into such engineered systems (and vice versa).
Systems engineering has long viewed the design and assessment of complex systems from the vantage point of inputs, processes, and outputs. The Saturn V rocket, F-22 fighter jet, search-and-rescue helicopter, and Da Vinci Surgical System are examples of such complex systems. The systems engineer generally has a clear sense of how the systems are built, the nature of a system’s key inputs, the dynamics of its processes, and the desired outputs. In this framework, the engineer can develop explicit plans, act on them, and expect clear outcomes based on targeted performance metrics.
Applying this same framework to questions about human systems can also be a useful tool to direct a system toward a desired state of performance, with one important caveat. Human systems are also classified as complex adaptive systems (CAS), where another set of considerations is central in describing and adjusting behavior. CAS are based on relationships, network effects, connections, and evolution, though they share the same input-process-output construction (Figure 2). In the CAS paradigm, divergences/variances must be observed using multivariate molecular measures to act, learn, and plan simultaneously. The plan is then carried out by deploying a set of countermeasures using multiple (multimodal) leverage points and iteratively building on what emerges.
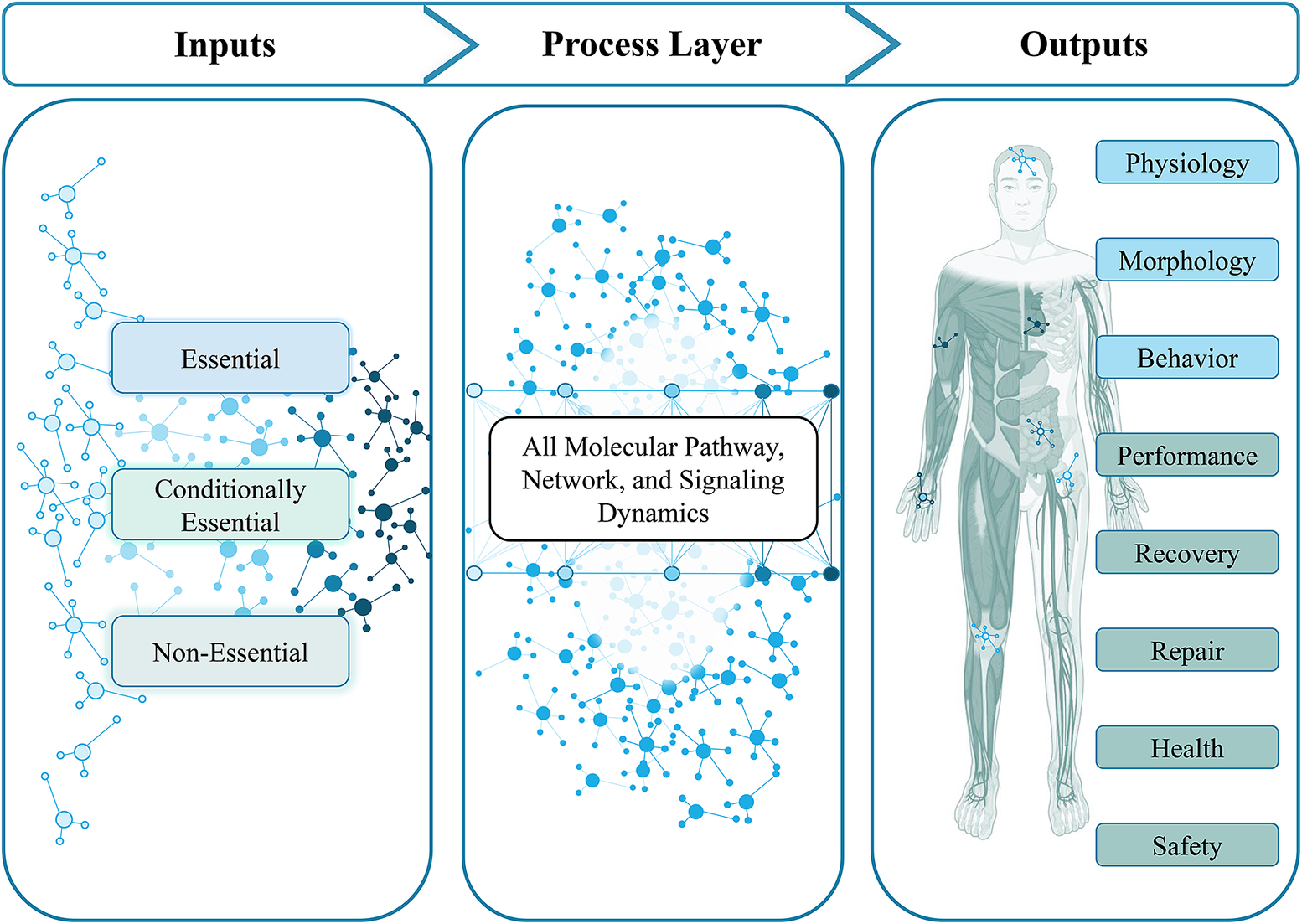
Figure 2. Basic input–output model for precision medicine. Three types of inputs enter the hidden layer (process layer): essential, conditionally essential, and nonessential. The process layer can be seen as the sum of the biological and biochemical processes that govern all human function. Driven by the varied inputs, this process layer is heavily modulated by the genome, epigenome, and feedback from other molecular forms (proteins, metabolites, gut microbiome, etc.), which influences the adaptive nature of the process. Single elements within the input layer, process layer, and output layer can be increasingly well characterized. This characterization adds precision to the stratified and personalized medicine application. Image credit: Sovaris Aerospace.
Figure 3 shows a simplified, neural network-based framework for dealing with complex systems with CAS characteristics. This framework does not attempt to recreate the dynamics of the entire system itself but rather presents a subset of large effector input nodes that account for much of the effect size in output. In practice, each of these layers can be formally assessed (as FCN) and are the basis for instrument monitoring and troubleshooting systems. Functionally, incremental gains or losses in the input layer or in the process layer may aggregate to produce significant changes in the output (and thereby, performance). However, depending upon the effector size, even a single input can have a considerable impact on the clinical or performance phenotype (e.g., water/hydration).
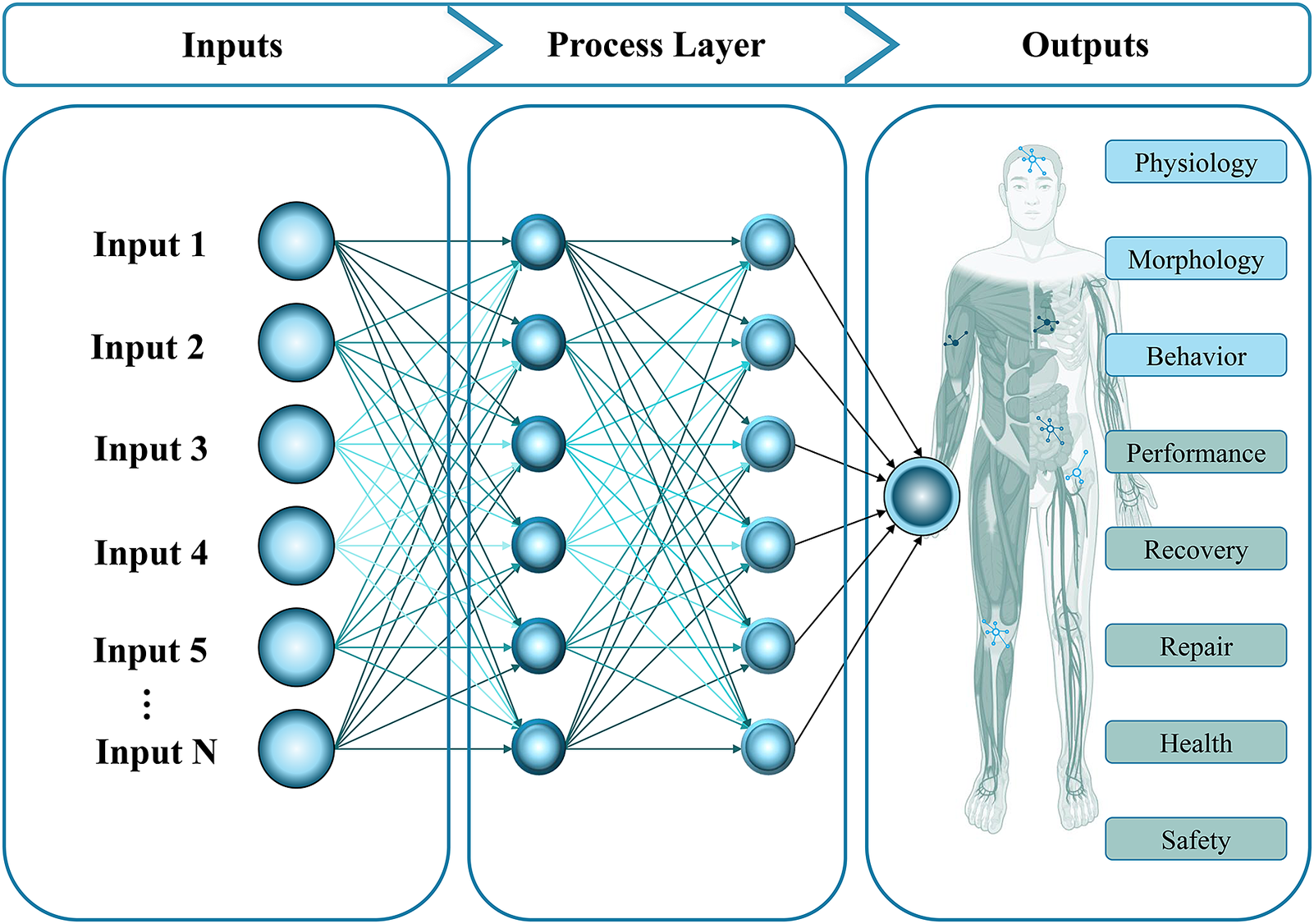
Figure 3. Artificial neural network (ANN) rendering to display the interaction between inputs, processes, and outputs. This feedforward ANN is used here merely to represent a set of relationships, rather than focusing on the traditional use of ANN for modeling, pattern analysis, training, and prediction. It is intended to show how multiple inputs (Input 1 to Input N) feed forward into the process layer to interact across multiple processes to arrive at a phenotypic output. The goal is to apply precision in characterizing inputs and networks within the process layer for the purpose of applying structure to the practice of precision medicine. Image credit: Sovaris Aerospace.
Characterizing input essentiality
Characterizing the types of inputs is useful because it creates a formal process for identifying molecular targets of interest to be measured in an effort to describe the system. This characterization also acts as guidance as to which inputs might be incorporated into the individualized countermeasure package and those inputs that may need to be reduced, remediated, or removed. These inputs can be divided into three categories (Table 2) (Schmidt and Goodwin, Reference Schmidt and Goodwin2013; Schmidt et al., Reference Schmidt, Meydan, Schmidt, Afshinnekoo and Mason2020a, Reference Schmidt, Meydan, Schmidt, Afshinnekoo and Mason2020b, Reference Schmidt, Meydan, Schmidt, Afshinnekoo and Mason2020c):
-
• Essential: Substances the body cannot make and must be obtained from the diet. Deficits lead to well-defined consequences.
-
• Conditionally essential: Substances that are ordinarily produced in sufficient amounts to meet the body’s basic requirements, though their synthesis can be compromised due to disease, genetics, essential input deficit, environmental exposures, or psychosocial stressors (Kendler, Reference Kendler2006). This can result in insufficient amounts available to support metabolic efficiency and require preformed exogenous sources.
-
• Nonessential inputs: Substances the body does not require, but to which the body is deliberately, incidentally, or accidentally exposed. These can modify metabolic network efficiency in complex ways.
Table 2. Representative essential, conditionally essential, and nonessential inputs

What is the origin of input deficits in generally healthy operators?
Before advancing to how one begins, it is useful to address selected factors that may contribute to deficits (or excesses) in substrates, precursors, intermediates, and cofactors (inputs) in generally healthy people operating in extreme environments. This also includes exposures to nonessential inputs that may impact performance. A brief summary of selected influences is considered below:
-
• Nutrient loss from extreme training or operations (e.g., magnesium loss through sweating via exertion in hot environments; Institute of Medicine (US) Committee on Military Nutrition Research Bernadette M. Marriott, 1993)
-
• Nutrient needs that increase due to the operating environment or operational demands (Longland et al., Reference Longland, Oikawa, Mitchell, DeVries and Phillips2016)
-
• Medication use that increases nutrient demands by consuming phase II conjugation agents or nutrient loss through excretion (Pickering et al., Reference Pickering, Macian, Papet, Dualé, Coudert and Pereira2019; Beger et al., Reference Beger, Schmidt and Kaddurah-Daouk2020; Kim et al., Reference Kim, Choi, Seo, Kwon, Yun and Jung2021)
-
• Medication use that impairs the synthesis of metabolically essential compounds (e.g., statins and ubiquinone), rendering them conditionally essential (Marcoff and Thompson, Reference Marcoff and Thompson2007)
-
• Exposure to environmental xenobiotics that place additional burden on metabolic networks, detoxification pathways, and nutrient cofactor pools (e.g., sulfur-bearing amino acids; Barros et al., Reference Barros, Oliveira and Morais2021)
-
• Reabsorption of metabolites derived from the gut microbiome that compete for critical nutrients, such as sulfhydryl compounds (e.g., p-cresol and glutathione; Schmidt et al., Reference Schmidt, Schmidt and Goodwin2022)
-
• Deficits in gut microbiome-derived critical nutrients (e.g., β-hydroxybutyrate needed to maintain colonic barrier integrity and brain epigenetic regulation; Silva et al., Reference Silva, Bernardi and Frozza2020)
-
• Exposure to environmental xenobiotics that poison enzyme systems (e.g., arsenic complexing with the alpha-lipoic acid residues on the pyruvate dehydrogenase complex, thus disrupting the TCA cycle and energy metabolism) (Schiller et al., Reference Schiller, Fowler and Woods1977; Nurchi et al., Reference Nurchi, Buha Djordjevic, Crisponi, Alexander, Bjørklund and Aaseth2020)
-
• Illicit drug use that modifies molecular networks (Patkar et al., Reference Patkar, Rozen, Mannelli, Matson, Pae, Krishnan and Kaddurah-Daouk2009)
-
• Specialized diets that lead to deficits unique to the diet (e.g., ketogenic, vegan, vegetarian, intermittent fasting, caloric restriction, caloric excess; Calton, Reference Calton2010; Thomas et al., Reference Thomas, Erdman and Burke2016; Churuangsuk et al., Reference Churuangsuk, Griffiths, Lean and Combet2019)
-
• Genetic variants (SNPs) that modify specific nutrient requirements (e.g., MTHFR and B12/folate/riboflavin; FAD1 and EPA/DHA; PEMT and choline; HFE and iron; Mathias et al., Reference Mathias, Pani and Chilton2014; Amenyah et al., Reference Amenyah, McMahon, Ward, Deane, McNulty, Hughes, Strain, Horigan, Purvis, Walsh and Lees-Murdock2020)
Why deficits and excesses scale across molecular networks: Essential inputs and the participation number
Essential inputs are frequently required in microgram or milligram amounts. Given these relatively small requirements, the impact of these inputs is often not given its due consideration. This is why it is important to view them from the vantage point of their participation number (PN) (Ames et al., Reference Ames, Elson-Schwab and Silver2002). The PN refers to the number of fundamental processes dependent upon a given input. In practice, this usually means the number of genes, enzymes, or signaling cascades in which a given input (often nutrient) is required. From this vantage point, it becomes evident that the effect of a single deficit can spread across 200, 300, or even 400 or more gene, transcription, signaling, or enzymatic processes comprising as many as 15% of the primary metabolic reactions in a human for a single input. As two, three, or more input deficits aggregate, their effects on molecular networks begin to scale. A glimpse at the participation number of selected inputs can be seen in Table 3.
Table 3. Participation number of selected essential inputs

Note: The numbers referenced in the table are referred to as “participation numbers,” owing to the number of fundamental processes dependent upon a given input. These can be reported as the number of enzymes or processes, or as the percentage of genes or enzymes in which the input is involved. The aggregation of two, three, or more input deficits (and the sum of their participation influence) can begin to scale significantly across molecular networks and human systems, which can have notable implications for health, safety, performance, and recovery.
Hydration status: An example of critical input quantification
Hydration status can be singled out as a predominant input since it is one of the single most influential modifiers of human performance in all extreme environments. Minor states of underhydration are well known to degrade performance. However, a loss of water greater than −2% of body mass (hypohydration) leads to substantial decreases in exercise performance, sport performance, work performance, cognitive function, decision-making, and mood. It also increases the risk of poor exercise tolerance, exertional heat illness, or exertional heatstroke for individuals exerting in hot and humid environments (Sawka et al., Reference Sawka, Cheuvront and Kenefick2015). This is amplified in careers where protective suits must be worn in extreme heat/humidity environments (firefighters, military). However, hypohydration can also occur due to diuresis from both cold and altitude exposure. Concomitant relative hypovolemia and absolute hypovolemia appear to be a prerequisite for hypohydration that impairs aerobic performance.
Watson et al. have demonstrated that mildly dehydrated subjects in a driving simulator made as many errors (like crossing over a rumble strip, late braking, and lane drifting) as those who operated with a blood alcohol concentration of 0.08%. The brain EEG data showed that impairment in mood, decision-making, and vigilance were likely to translate into greater actual driving errors in the real world.
Moreover, the error rate in driving in this mildly dehydrated state was similar to what they found in their previous studies of those consuming 2.5 oz of vodka (Watson et al., Reference Watson, Whale, Mears, Reyner and Maughan2015). In a study of dehydration and decision-making, those who ingested only 6 oz of water in one day (compared to a norm closer to 125 oz) committed 12% more errors in a cognitively loaded game scenario (Stachenfeld et al., Reference Stachenfeld, Leone, Mitchell, Freese and Harkness2018).
Deliberate attention to hydration status and hydration strategies is essential in all extreme operations. It bears noting that all measures of hydration status have limitations and should be viewed accordingly (Barley et al., Reference Barley, Chapman and Abbiss2020).
Beyond inputs: The process layer and performance
While this overview has highlighted the importance of essential, conditionally essential, and nonessential inputs, a critical feature in understanding molecular networks is also to query the extensive and complex process layer. The process layer essentially encompasses all fixed genomic elements, along with all other molecular dynamics that are fundamental to metabolism. In daily practice, it is impractical to query all molecular features within the process layer. However, there is value in measuring markers that have a known influence on performance and recovery, ideally coupled with those that can lead to actionable countermeasures.
One example of how process-associated molecular measures can impact performance regards inflammation. For instance, elevated IL-6, IL-1β, TNFα, INFγ, and in some cases, hsCRP are associated with attributes critical to performing (and safely so) in extreme environments such as altered decision-making and diminished psychomotor speed (Rawls et al., Reference Rawls, Dougherty, Blais, Stancliffe, Kolling, Vinnakota, Pannala, Wallqvist and Papin2019). For instance, in one study, psychomotor speed for older adults was shown on average to be 169 ms slower in reaction time tests than for young adults. However, when the level of IL-6 was used to divide the group into high- and low-inflammation classes, the high inflammation group showed a reaction time 90 milliseconds slower than the low inflammation group, even though the high inflammation group now had younger people as a part of it (Balter et al., Reference Balter, Higgs, Aldred, Bosch and Raymond2019). This suggests that inflammation is an important modulator of diminished psychomotor speed, as opposed to mere aging.
This is consistent with another study of 93 people between the ages of 21 and 65 who suffered from depression. IL-6 was associated with impaired reaction times in all seven measures used to detect psychomotor slowing. MCP-1 was associated with two of the measures of slowing (Goldsmith et al., Reference Goldsmith, Haroon, Woolwine, Jung, Wommack, Harvey, Treadway, Felger and Miller2016).
Baseline levels of hsCRP have also been used to assess whether warfighters were at greater risk of reporting symptoms of post-traumatic stress upon returning from combat. Blood CRP levels, physiological, and psychological parameters were determined pre-deployment and at approximately 3 and 6 months following a seven-month deployment. hsCRP predominantly influenced the likelihood of subjects reporting the presence versus absence of PTS symptoms (rather than the extent of symptoms when present). The authors suggested that high baseline hsCRP levels may mark a “state of vulnerability” (Eraly et al., Reference Eraly, Nievergelt, Maihofer, Barkauskas, Biswas, Agorastos, O’Connor and Baker2014). Similar findings of potential increased “vulnerability” have been reported for concussion in young American football players with elevated IL-6 and IL-1RA (Nitta et al., Reference Nitta, Savitz, Nelson, Teague, Hoelzle, McCrea and Meier2019).
Though some of the above studies would benefit from greater subject numbers, such findings suggest that identifying and correcting such inflammatory states might become a foundation of PM for such operations. These findings also raise the prospect of using inflammatory markers as one predictive tool for optimizing health and performance in those entering extreme environments. In certain operators such as fighter pilots traveling 1,500 mph, race car drivers traveling 200 mph, baseball hitters evading a 98-mph fastball, or air traffic controllers, decrements in psychomotor speed may also impact safety.
A set of measures commonly used to assess inflammatory status include MCP-1, sICAM-1, sCD40L, IL-1β, IL-6, IL-10, IL-8, IL-12p40, INFγ, and hsCRP. Below, the concept of FCN will be explored as one means to analyze the process layer more deeply.
Diet and nutrition
Diet and nutrition are among the most influential inputs on human performance since they shape almost all areas of metabolism. Because the subject matter is beyond the scope of this overview, only select examples are used to illustrate specific points of emphasis. In keeping with the concept of the previous examples of psychomotor response and performance, psychomotor vigilance and diet are explored below.
NASA uses the Human Exploration Research Analog (HERA) in Houston to study controlled habitation on Earth as an analog for spaceflight. The HERA Campaign 4 contained a 45-day mission examining the effect of standard spaceflight diet as used on the International Space Station (ISS) and compared that with an enhanced diet (ED) in 16 subjects.
The enhanced diet provided > 6 servings fruits and vegetables per day, 2–3 servings (8–12 oz) of fish per week, > 5 tomato-based (lycopene-rich foods) per week, and >2 flavonoid-rich foods per day. Protein intake was maintained between 1.2 and 1.7 g/kg/day, vitamin D at 800 IU/day, iron around 10 mg/day, calcium between 1,000 and 1,200 mg/day, and sodium around 2,300 mg/day.
Vigilant attention was evaluated using the Psychomotor Vigilance Test (PVT), which was administered twice at baseline (pre-mission) and three times weekly over the 45-day mission. Those on the enhanced diet showed improved reaction speed (p = 0.014), improved accuracy (p = 0.022), and fewer attention lapses (p = 0.0047) than those consuming the standard diet during the mission (Douglas et al., Reference Douglas, DeKerlegand, Dlouhy, Dumont-Leblond, Fields, Heer, Krieger, Mehta, Rooney, Torralba, Whiting, Crucian, Lorenzi, Smith, Young and Zwart2022). While PVT is not a direct measure of performance, it has been shown to be predictive of simulated spacecraft docking performance (Basner et al., Reference Basner, Moore, Hermosillo, Nasrini, Dinges, Gur and Johannes2020) and is correlated with rates of serious medical errors made by resident physicians (Rahman et al., Reference Rahman, Sullivan, Barger, St Hilaire, O’Brien, Stone, Phillips, Klerman, Qadri, Wright, Halbower, Segar, McGuire, Vitiello, de la Iglesia, Poynter, Yu, Sanderson, Zee, Landrigan, Czeisler and Lockley2021). In general, this study demonstrated the potential impact of a basic dietary enhancement on an important measure associated with success in an extreme environment.
We recently examined for molecular deficits in 30 American collegiate football players entering the NFL (professional) draft who were from different university programs. We identified several deficits in common across the cohort such as vitamin D, omega-3 fatty acids, magnesium, AA/EPA ratio, and elevated homocysteine. These patterns were detected in roughly 80% of the cohort. Of further note was the finding that 70% of those university programs had full-time dieticians or nutritionists (Kunces et al., Reference Kunces, Keenan, Schmidt and Schmidt2021). This suggests that dietary recommendations alone are often not sufficient to prevent molecular deficits and that molecular profiling for the purpose of detecting deficits is an important adjunct to professional dietary advice that can be used to guide individualized intervention.
In general, PM application of dietary and nutritional support can be facilitated by food frequency questionnaires, coupled with laboratory testing aimed at characterizing essential and conditionally essential dietary inputs. Intervention often requires a combination of dietary changes and nutritional supplementation.
The gut microbiome: An engine of local and systemic metabolic activity
The gut microbiome is a complex system consisting of bacteria, viruses, archaea, fungi, and protozoans. This community of organisms metabolizes and modifies dietary substrates to produce a vast array of compounds that modulate host immunity, vitamin synthesis, maintenance of gut barrier integrity, the microbial community itself, and numerous systemic processes. While strongly shaped by diet, other influences such as drugs, exercise, and sleep strongly impact its composition. Some of the gut microbiome molecular products act enterically, while others are absorbed and distributed systemically for more distant action (such as through exosomes or distributed through the bloodstream).
Understanding these complex dynamics for clinical application can be challenging. Therefore, it is useful to consider several basic principles before entertaining actionable steps. First, untargeted microbiome analysis can yield patterns of interest, though using targeted analyses to confirm such findings (e.g., strain vs. species) adds needed refinement. Second, patterns derived from untargeted analyses are not typically used in diagnosis, as diagnosis is best left to standard fully quantitative methods. Third, since there is variability between labs conducting untargeted microbiome analyses (sampling, analytical methods, statistical processing, and interpretation), it is important to keep this variability in mind when drawing conclusions.
Given this, untargeted microbiome analysis is used extensively as a research tool, though commercial laboratories have emerged that provide microbiome services to consumers. These often use a combination of their own reference populations, coupled with populations derived from public databases. Some of them also provide species-level resolution.
In extreme environments, there is a minimal set of microbiome-associated questions that can often generate useful information for clinical follow-up. While the following list is in no way comprehensive, it permits a basic entry into the domain of gut microbiome surveillance that may lead to actionable targets. Questions include (but are not limited to):
-
• What are the positive and negative impacts of exercise on the gut microbiome (Gill et al., Reference Gill, Hankey, Wright, Marczak, Hemming, Allerton, Ansley-Robson and Costa2015; Mohr et al., Reference Mohr, Jäger, Carpenter, Kerksick, Purpura, Townsend, West, Black, Gleeson, Pyne, Wells, Arent, Kreider, Campbell, Bannock, Scheiman, Wissent, Pane, Kalman, Pugh, Ortega-Santos, Ter Haar, Arciero and Antonio2020)?
-
• What is the status of selected gut-derived systemic metabolites that confer benefit (e.g., butyrate, SCFA; Den Besten et al., Reference Den Besten, Van Eunen, Groen, Venema, Reijngoud and Bakker2013; Scheiman et al., Reference Scheiman, Luber, Chavkin, MacDonald, Tung, Pham, Wibowo, Wurth, Punthambaker, Tierney, Yang, Hattab, Avila-Pacheco, Clish, Lessard, Church and Kostic2019; Agus et al., Reference Agus, Clément and Sokol2021)?
-
• What is the status of selected gut-derived systemic metabolites that confer harm or facilitate metabolic dysregulation (e.g., secondary bile acids (cognition), p-cresol; (Schmidt et al., Reference Schmidt, Meydan, Schmidt, Afshinnekoo and Mason2020a; Varma et al., Reference Varma, Wang, An, Varma, Bilgel, Doshi, Legido-Quigley, Delgado, Oommen, Roberts, Wong, Davatzikos, Resnick, Troncoso, Pletnikova, O’Brien, Hak, Baak, Pfeiffer, Baloni, Mohmoudiandehkordi, Nho, Kaddurah-Daouk, Bennett, Gadalla and Thambisetty2021; Ahmed et al., Reference Ahmed, Leyrolle, Koistinen, Kärkkäinen, Layé, Delzenne and Hanhineva2022)?
-
• What is the individual gut microbial community structure and diversity profile? How does this compare to different populations (general public, fit individuals, IBD/IBS; Vijay and Valdes, Reference Vijay and Valdes2022)?
-
• What is the status of selected keystone species with known biological action (e.g., Akkermansia muciniphila, Faecalibacterium prausnitzii; Azad et al., Reference Azad, Sarker, Li and Yin2018; Effendi et al., Reference Effendi, Anshory, Kalim, Dwiyana, Suwarsa, Pardo, Nijsten and Thio2022)?
-
• What is the status of pathogenic organisms (e.g., fungi, protozoans, clostridial species; Burgess et al., Reference Burgess, Gilchrist, Lynn and Petri2017; Libertucci and Young, Reference Libertucci and Young2019; Zhang et al., Reference Zhang, Aschenbrenner, Yoo and Zuo2022)?
-
• Are there pathogenic organisms in the environment that may influence the host response (Yoo et al., Reference Yoo, Groer, Dutra, Sarkar and McSkimming2020; Panthee et al., Reference Panthee, Gyawali, Panthee and Techato2022)?
-
• What is the status of selected measures of gut barrier integrity (e.g., zonulin, calprotectin)? What is the impact on health and performance of disordered gut barrier integrity (Chelakkot et al., Reference Chelakkot, Ghim and Ryu2018; Ribeiro et al., Reference Ribeiro, Petriz, Marques, Kamilla and Franco2021)?
-
• What is the daily intake of dietary substrates that shape gut microbiome composition (e.g., dietary fiber types, dietary fiber total daily intake; Singh et al., Reference Singh, Chang, Yan, Lee, Ucmak, Wong, Abrouk, Farahnik, Nakamura, Zhu, Bhutani and Liao2017; Reynolds et al., Reference Reynolds, Mann, Cummings, Winter, Mete and Te Morenga2019; Miketinas et al., Reference Miketinas, Shankar, Maiya and Patterson2020)?
-
• What is the daily dietary intake of micronutrients that influence mucosal barrier integrity (e.g., glutamine, omega-3 fatty acids; Zuhl et al., Reference Zuhl, Dokladny, Mermier, Schneider, Salgado and Moseley2015; Costantini et al., Reference Costantini, Molinari, Farinon and Merendino2017)?
-
• What is the status of gastrointestinal symptoms (Wang et al., Reference Wang, Guo and Yang2015; McKenna et al., Reference McKenna, Fennel, Berkemeier, Nava, Amorim, Deyhle and Mermier2022)?
Translating the gut microbiome literature to individuals can be challenging. Understanding an individual’s untargeted gut microbiome report can also be challenging, but it can yield data one step closer to providing useful, actionable clinical decision support or in generating new hypotheses. This is especially true when the analysis adds targeted measures based on standard methods and calibration curves for individual analytes.
Gastrointestinal problems and persistent clinical symptoms are common, and often debilitating in humans operating in extreme environments (Wang et al., Reference Wang, Guo and Yang2015). They can impact everything from individual performance to team cohesion. Characterizing the dynamics of the gut microbiome and its associated metabolic fingerprint will increasingly become necessary in order to optimize individualized countermeasures. At this stage of our understanding, a combination of untargeted and targeted measures will be essential to unravel the connection between the microbiome, the clinical phenotype, and the operating environment, as well as the long-term host response.
The exposome: A source of nonessential inputs
One of the most underutilized tools in PM in extreme environments is exploration of the exposome. The exposome is the sum of external factors to which a person is exposed during a defined epoch or over a lifetime. Because the subject is extensive, the focus herein is limited by illustration to concepts of drug prescribing and selected examples of environmental xenobiotics as nonessential inputs that might be encountered in extreme environments.
The exposome: Drug exposure and principles of prescribing
The drug exposome is one component of the exposome that is fully within the control of individuals and can be seen as being most amenable to regulation. The need for enhancing efficacy and minimizing adverse events (AE) in drug prescribing for extreme operations is heightened because of the additional emphasis on performance and safety of operations. Therefore, a foundation of best practices should generally consider analysis of and correction for the following:
-
• Drug–gene interactions
-
• Drug–drug interactions
-
• Drug–herb interactions
-
• Drug–food interactions
-
• Drug–nutrient interactions
Drug–gene interactions
Drug–gene interactions fall within the domain of pharmacogenomics. Pharmacogenomics is the study of gene variants that influence the regulation of drug metabolism. It broadly considers single nucleotide polymorphisms, insertions, deletions, copy number variants, and other modifications to the genes involved in drug metabolism. One example can be used to explore the relevance of pharmacogenomics to people operating in extreme environments.
Codeine is among the opioid analgesics that is used to relieve mild to moderately severe pain. The enzyme CYP4502D6 (cytochrome P450 2D6) converts codeine to the active metabolite morphine, which is responsible for the analgesic effects of codeine. In those who carry two inactive copies of the 2D6 allele (poor metabolizers; ~10% conversion to morphine), there will be inadequate pain relief, due to inadequate morphine formation.
Conversely, those who carry more than two functional 2D6 copies more rapidly and completely convert codeine to morphine. These are the ultrarapid metabolizers (UM; 40–50% conversion to morphine) wherein even normal doses of codeine may lead to symptoms of morphine overdose. Included are shallow breathing, sleepiness, confusion, sweating, nausea, vomiting, constipation, dizziness, drowsiness, laryngospasm, and others (Dean and Kane, Reference Dean and Kane2012). Of note, morphine, oxycodone, and tramadol are among other analgesics whose metabolism is impacted by the 2D6 UM genotype (Smith, Reference Smith2009).
This knowledge is useful in operational settings because morphine overdose symptoms can threaten performance, health, safety, and even survival. In practice, one can single out CYP4502D6 ultrarapid metabolizers using pharmacogenomics and assure that codeine is not used in these individuals for any purpose. For perspective, roughly 3% of African Americans, 1–10% of Caucasians, and 16–28% of North Africans and Ethiopians are CYP2D6 UMs (Bertilsson et al., Reference Bertilsson, Dahl, Dalén and Al-Shurbaji2002; Gasche et al., Reference Gasche, Daali, Fathi, Chiappe, Cottini, Dayer and Desmeules2004; U.S. Food and Drug Administration, 2019). For CYP4502D6 poor metabolizers, codeine is not indicated because it is not effective.
The potential impact of genetic variants on drug metabolism in those operating in extreme environments can be further contextualized by an investigation of a large military veteran cohort. Out of 7,769,356 veterans who took part in the study, nearly 55% took at least one drug with strong evidence of altered metabolism associated with a specific gene mutation over the 6-year study period. More than 33% were given a new prescription for one of these drugs. Notably, of that 33%, 38% were prescribed more than one drug for which pharmacogenomics data could guide dosage, usage, and predict outcomes.
Of particular concern, of the 533,928 of those who received new prescriptions of simvastatin, 25.6% were projected to have a SNP variant in SLC01B1 (solute carrier organic anion transporter family member 1B1), which may put them at risk for myopathy no matter the dose. Twenty-five percent of those were prescribed an 80 mg dose, putting those individuals at further risk (Chanfreau-Coffinier et al., Reference Chanfreau-Coffinier, Hull, Lynch, DuVall, Damrauer, Cunningham, Voight, Matheny, Oslin, Icardi and Tuteja2019).
The potential value in pharmacogenomic profiling can be illustrated in one of the first large-scale studies of its kind in 6,944 patients conducted by the Ubiquitous Pharmacogenomics Consortium. Of the total cohort, 93.5% carried at least one actionable genetic variant based on an analysis of 12 genes (CYP: 2B6, 2C9, 2C19, 2D6, 3A5; DPYD; F5; HLA-B; SLCO1B1; TPMT; UGT1A1; VKORC1). Of these carriers, 30.5% carried two and 26.0% carried three actionable variants.
In patients with an actionable test result for the index drug (n = 1,558), a clinically relevant adverse drug reaction occurred in 21.0% of patients who underwent pharmacogenomic screening to guide prescribing. In contrast, 27.7% in the control group experienced clinically relevant adverse events. For the index drugs, the highest numbers of patients with an actionable variant were observed for atorvastatin (28.5%), tramadol (48.3%), and clopidogrel (27.8%). For drugs overall (taken by >25 patients and with actionability >20%), the highest extent of actionability was seen for venlafaxine, metoprolol, tamoxifen, codeine, oxycodone, amitriptyline, warfarin, simvastatin, sertraline, citalopram, and escitalopram (Swen et al., Reference Swen, van der Wouden, Manson, Abdullah-Koolmees, Blagec, Blagus, Böhringer, Cambon-Thomsen, Cecchin, Cheung, Deneer, Dupui, Ingelman-Sundberg, Jonsson, Joefield-Roka, Just, Karlsson, Konta, Koopmann, Kriek, Lehr, Mitropoulou, Rial-Sebbag, Rollinson, Roncato, Samwald, Schaeffeler, Skokou, Schwab, Steinberger, Stingl, Tremmel, Turner, van Rhenen, Dávila Fajardo, Dolžan, Patrinos, Pirmohamed, Sunder-Plassmann, Toffoli and Guchelaar2023).
Many of these drugs would be found in the clinical regimen of general military, military Special Forces, SWAT, spaceflight, athlete, first responder, search and rescue, or other high-performing individuals. In the clinical care of those operating in extreme environments, it is these small incremental gains and losses that aggregate to influence operational performance and safety. Schmidt et al. have published a pharmacogenomic strategy for astronauts that can be extrapolated for use in any extreme operating environment. In this application, a table of preferred and rejected drugs can be built for each individual on a team, which can guide physician prescribing (Schmidt et al., Reference Schmidt, Schmidt and Goodwin2022).
Drug–drug interactions
Drug–drug interactions may occur when two or more drugs are given concomitantly and one drug either induces or inhibits the metabolism of the other drug. The principle of drug–drug interactions can be represented by examining CYP4503A4, one of the cytochromes responsible for the metabolism of roughly 50% of prescription drugs (Low et al., Reference Low, Setia and Lima2018). CYP450 3A4 is the enzyme required for the metabolism of zolpidem (Ambien), a drug commonly used for insomnia in high-performance environments like spaceflight and military. Zolpidem is a substrate for CYP4503A4 metabolism. If circumstances also require the concomitant administration of a drug like erythromycin (a known inhibitor of CYP4503A4), the erythromycin will slow down zolpidem metabolism and extend zolpidem’s effect over time.
This may cause the effect of zolpidem to carry over into daytime operations with potential adverse effects on operational execution or safety. Drugs like sertraline (Zoloft), escitalopram (Lexapro), and fluoxetine (Prozac) used in depression will have a similar effect on zolpidem metabolism (Low et al., Reference Low, Setia and Lima2018). These types of interactions are widespread and often not considered for drug therapeutics in operating environments. The clinical attention to these does not require testing, but rather evaluation of the extensive published tables of drug–drug interactions. The Flockhart Table developed at the University of Indiana is widely used for this purpose (Flockhart et al., Reference Flockhart, Thacker, McDonald and Desta2021).
Drug–herb interactions
Hypericum perforatum (commonly known as St. John’s wort) is frequently used in depression and is an inducer of CYP450 3A4. This is a classic example of a drug–herb interaction, in which the induction of CYP3A4 causes it to metabolize an active drug more rapidly. Mechanistically, hypericum increases the expression of both CYP3A4 and P-glycoprotein (P-gp), which leads to increased drug metabolism and reduced cellular uptake, respectively (Rombolà et al., Reference Rombolà, Scuteri, Marilisa, Watanabe, Morrone, Bagetta and Corasaniti2020).
One example of a drug that is metabolized by CYP3A4 is the antipsychotic medication, clozapine. In a 2012 clinical case, a female patient, who had been taking a 500 mg daily dose of clozapine, began experiencing a reduction in the efficacy of her medication. Blood tests revealed a sudden decrease in plasma concentrations of clozapine, which coincided with the worsening of her clinical symptoms. Clinicians discovered that she had begun to self-medicate with 900 mg daily of St. John’s wort. Following her discontinuation of St. John’s wort, the patient’s clozapine plasma concentrations increased and her psychiatric condition improved (Van Strater and Bogers, Reference Van Strater and Bogers2012).
Kratom (Mitragyna speciosa) is a botanical now in wide use by athletes, soldiers, and others operating in extreme environments for its opioid-like effects. Kratom alkaloids, such as mitragynine (MTG), may be of clinical relevance as they have been shown to be inhibitors of CYP450 2D6 and may interfere with the metabolism of drugs such as haloperidol, chlorpromazine, thioridazine, perphenazine, risperidone nortriptyline, paroxetine, fluoxetine, venlafaxine, desipramine, propranolol, metoprolol, timolol, and alperolol (Ayano, Reference Ayano2016; Kamble et al., Reference Kamble, Sharma, King, Berthold, León, Meyer, Kanumuri, McMahon, McCurdy and Avery2020).
Drug–food interactions
Cabbage (Brassica oleracea) is a commonly ingested cruciferous vegetable that contains high levels of glucosinolates that are metabolized in the liver to produce bioactive compounds like isothiocyanates, indoles, and dithiolethiones (Esteve, Reference Esteve2020). This class of compounds interacts with drug metabolism through different mechanisms. Firstly, they can induce cytochrome P450 (CYP) enzymes and increase their activity in the liver. For instance, indole-3-carbinol (I3C) found in cabbage may induce CYP1A1, CYP1A2, and CYP1B1, leading to enhanced metabolism, such as caffeine, melatonin, olanzapine, and theophylline. As a result, the efficacy of the drugs may be reduced, and their clearance from the body increased (Abdull Razis et al., Reference Abdull Razis, Bagatta, De Nicola, Iori and Ioannides2010). Alternatively, cabbage and its bioactive compounds may also inhibit drug metabolism by down-regulating CYP enzymes or inhibiting drug transporters. For example, sulforaphane, another bioactive compound in cabbage, has been shown to inhibit CYP2C9 and CYP3A4 enzymes that are involved in the metabolism of many drugs, including sertraline, codeine, fentanyl, and sildenafil, among others (Zhou et al., Reference Zhou, Poulton, Grün, Bammler, Blumberg, Thummel and Eaton2007).
More generally, cruciferous vegetables, such as broccoli, Brussels sprout, cabbage, cauliflower, radish, and watercress, have been found to have significant, quantifiable effects on drug-metabolizing enzymes. While the data is still scarce and isolating the effects from specific Cruciferae species can be challenging, one study found that the ingestion of cruciferous vegetables increased CYP1A2 activity by 20–40% (Eagles et al., Reference Eagles, Gross and McLachlan2020). Taken together, the induction or inhibition of drug-metabolizing enzymes such as CYP1A1, CYP1A2, CYP1B1, CYP2C9, and CYP3A4, can lead to the altered metabolism of a wide range of drugs, such as acetaminophen, warfarin, and metformin (Platel and Srinivasan, Reference Platel and Srinivasan1997; Steinkellner et al., Reference Steinkellner, Rabot, Freywald, Nobis, Scharf, Chabicovsky, Knasmüller and Kassie2001; Hladun et al., Reference Hladun, Papaseit, Martín, Barriocanal, Poyatos, Farré and Pérez-Mañá2021).
Drug–nutrient interactions
There exists an extensive body of literature on drug–nutrient interactions that lead to nutrient deficits that negatively impact the clinical phenotype (Péter et al., Reference Péter, Navis, de Borst, von Schacky, Van Orten-Luiten, Zhernakova, Witkamp, Janse, Weber, Bakker and Eggersdorfer2017; Mohn et al., Reference Mohn, Kern, Saltzman, Mitmesser and McKay2018). For example, metformin can be used to illustrate one such dynamic as a demonstration of principle. It is well-established that metformin leads to vitamin B12 deficiency on a dose-dependent basis. This correlates with a predicted elevation of homocysteine (Hcy) in a high percentage of users over time. The resultant decrease in B12 and the rise in Hcy have also been associated with a worsening of the clinical phenotype, such as neuropathy.
In one analysis of those who took metformin for at least 6 months, vitamin B12 deficiency was observed in 22.2% of patients. This argues for providing supplemental B12, while also monitoring B12, methylmalonic acid, and Hcy status in metformin users (Beger et al., Reference Beger, Schmidt and Kaddurah-Daouk2020). Beyond the specific example, it also highlights the importance of addressing drug–nutrient interactions in all drug prescribing for those in extreme environments.
Careful prescribing to avoid banned drugs
Since many in extreme environments (sports, some occupations) are prohibited from using banned substances, clinicians must always remain cognizant of the individual drugs that have been banned by various organizations. Otherwise, it is possible to inadvertently prescribe a banned drug that places the individual’s participation or even career in jeopardy.
For example, glucocorticoids that are administered by certain routes are prohibited by the World Anti-Doping Agency (WADA). In one survey of 603 sports medicine physicians (30 countries), less than 25% accurately identified which routes of glucocorticoid administration are prohibited in competition by WADA. There was also considerable variation in the length of time before competition that the use of glucocorticoids would compel the physician to consider applying for a therapeutic use exemption (Hughes et al., Reference Hughes, Vlahovich, Welvaert, Tee, Harcourt, White, Vernec, Fitch and Waddington2020).
Concerns about drug overuse in extreme environments
Because of the extensive demands of heavy training, competition, or operations, the use of performance-enhancing drugs, illicit drugs, and/or unapproved drugs must be considered in operators in extreme environments. For example, US Navy SEALs have tested positive for cocaine, methamphetamine, heroin, marijuana, and ecstasy. They sometimes use Viagra to avoid lung fluid buildup in extreme water training. This has resulted in injury and death (Clean Sport, 2023).
One significant area of concern for those operating in extreme environments is the excessive use of analgesics. For example, it has been observed that athletes use up to four times more analgesics than their age-matched counterparts (Holgado et al., Reference Holgado, Hopker, Sanabria and Zabala2018; Zandonai et al., Reference Zandonai, Escorial and Peiró2021).
Codeine is among the analgesics widely used among athletes. Its pharmacogenomic profile and links to symptoms of morphine overdose have been described above. Tramadol is another analgesic widely used by athletes (Baltazar‐Martins et al., Reference Baltazar‐Martins, Plata, del, Muñoz‐Guerra, Muñoz, Carreras and Del Coso2019). Like codeine, it is also metabolized via CYP4502D6. Ultrarapid 2D6 metabolizer phenotypes are more likely to convert tramadol to morphine and place the user at risk of morphine overdose symptoms. Moreover, tramadol carries a greater risk of addiction, due to its euphoric properties. Knowledge of genetic variants could help optimize treatment with tramadol to achieve better pain control (Peiró, Reference Peiró2018). It is important to understand that when the pharmacogenomic profile of a high-performing individual is known, many adverse drug reactions can be avoided because they are predictable and repeatable.
Dietary supplement contaminants and adulterants
The precision nutrition approach based on essential and conditionally essential inputs often leads to countermeasure approaches that require intervention by dietary changes or dietary supplement use. Dietary supplement use is widespread among those operating in extreme environments (e.g., athletes) because they are generally perceived as providing an advantage. The most frequently reported reasons for such use are improving athletic performance, improving health, and accelerating recovery. Depending upon the population, supplement use varies between 11 and 100% of athletes polled (Daher et al., Reference Daher, Mallick and El Khoury2022). When guided by laboratory testing, such supplementation can be done with even greater precision and is often crucial to restoring metabolic network efficiency.
However, a foundation of this practice should also consider attention to contaminants and adulterants, which represent another hidden element of the exposome. Contaminants are those agents sometimes present in a product that was either inadvertently present or accrue via poor supply chain management and poor manufacturing practices. Adulterants are those agents (e.g., drugs) that have been deliberately added to a supplement in order to enhance its effect (Mathews, Reference Mathews2018).
Among the most relevant classes of contaminants are metals, microbes, toxins, mycotoxins, pesticides, herbicides, dioxins, and PCBs, as well as pharmacologically active ingredients (Costa et al., Reference Costa, Vidovic, Saraiva, do Céu Costa, Del Favero, Marko, Oliveira and Fernandes2019). In one report of adulterants, four experimental stimulants were found in sports and weight loss supplements, including 2-amino-6-methylheptane (octodrine), 1,4-dimethylamylamine (1,4-DMAA), 1,3-dimethylamylamine (1,3-DMAA) and 1,3-dimethylbutylamine (1,3-DMBA) (Cohen et al., Reference Cohen, Travis, Keizers, Deuster and Venhuis2018).
An analysis of the US Food and Drug Administration (FDA) warnings from 2007 to 2016 revealed that unapproved pharmaceutical ingredients were identified in 776 dietary supplements. These products were most commonly marketed for muscle building, sexual enhancement, or weight loss. The most common adulterants were synthetic steroids or steroid-like ingredients for muscle-building supplements, sibutramine for weight loss supplements, and sildenafil for sexual enhancement supplements. Notably, 157 products (20.2%) contained more than one unapproved drug ingredient (Tucker et al., Reference Tucker, Fischer, Upjohn, Mazzera and Kumar2018).
Organizations, individuals, and clinicians have addressed this issue by seeking products from companies that submit their products to third-party testing for contaminants and adulterants (Vento and Wardenaar, Reference Vento and Wardenaar2020). One such global organization based in the United States is NSF. NSF is an independent organization that currently screens supplements for 280 substances banned by most major athletic organizations (NSF Certified for Sport). This includes undeclared ingredients like stimulants, narcotics, steroids, diuretics, beta-2-agonists, masking agents, and other substances. In the US, major league baseball, hockey, and football (Canada) are permitted to only provide and recommend supplement products that are Certified for Sport. Other leagues are beginning to follow suit. Clinicians who adhere closely to these same NSF standards will offer a valuable service to their patients who operate in extreme environments (Tucker et al., Reference Tucker, Fischer, Upjohn, Mazzera and Kumar2018; Crawford et al., Reference Crawford, Walter, Avula, Lindsey, Hunter, Ikhlas and Deuster2022).
The exposome: Environmental exposures
Environmental exposures in extreme operating environments can occur during training, deployment, or competition. Three examples are noted below to highlight this point. The HunterSeven Foundation recently commissioned a study of over 3,000 military respondents who had deployed to Afghanistan at least once between 2001 and 2018 (Dow, Reference Dow2021). The study revealed the following:
-
• 36% of respondents reported being directly involved with an improvised explosive device (IED) blast.
-
• 75% of respondents reported having some IED-related exposure.
-
• 51% of respondents reported unexploded ordnance exposures.
-
• 41% of respondents reported active chemical exposures (mostly to the skin).
-
• 19% of respondents reported being exposed to Depleted Uranium.
-
• 18% of respondents reported exposure to asbestos.
-
• 13% of respondents reported exposure to radiation.
-
• 79% of respondents reported burn pit exposures on base.
After a series of respiratory complaints were presented by a group of Berlin police officers, a study was undertaken to assess duration of exposure to the indoor firing range, blood metal content (lead, antimony, manganese), and respiratory measures (plethysmography). Witt et al. observed increased blood lead concentrations depending on the subject’s age (+1.2% per year) and cumulative gunshot exposure (+0.34% per 100,000 shots). This was accompanied by differences in forced expiratory volume between the groups (Witt et al., Reference Witt, Kienast, Bölke, Hoffmann, Roehle, Bender, Nowak, Tauber, Gunga, Hoffmann, AJS and Liebers2023).
These findings are consistent with a meta-analysis wherein 31 studies showed blood lead levels (BLLs) > 10 μg/dL in some shooters, 18 studies with BLLs >20 μg/dL, 17 studies >30 μg/d, and 15 studies with BLLs >40 μg/dL (Laidlaw et al., Reference Laidlaw, Filippelli, Mielke, Gulson and Ball2017). The literature suggests that BLLs in shooters are associated with the number of bullets discharged and the caliber of weapon fired, which is associated with lead aerosol discharge from guns and air lead at the firing ranges. Previous studies have shown that elevated lead (10.76 μg/dl) in recreational pistol shooters was associated with impaired verbal memory (Asa-Mäkitaipale et al., Reference Asa-Mäkitaipale, Jehkonen, Uitti and Vilkki2009).
The uptake and elimination of targeted volatile organic compounds have been investigated in firefighters by collecting the exhaled breath on sorbent tubes before and after controlled structure burns. When data were assessed at the group level, benzene concentrations were found to be elevated post-exposure in victim search, fire attack, and outside ventilation firefighting positions. However, examination of data at the individual level demonstrated that some firefighters may be more susceptible to post-exposure volatile organic compounds increases than others (Wallace et al., Reference Wallace, Pleil, Oliver, Whitaker, Mentese, Fent and Horn2019).
Assessment of the exposome, the clinical phenotype of variable exposures over time, and understanding measures of individual susceptibility is a complex dynamic in the extreme environment setting. However, it is justifiable that clinicians give appropriate attention to the history of potential exposure and pursue the relevant targeted molecular analytics in order to formulate a precision clinical management strategy. In alignment with the foundational premise outlined herein, knowable, actionable xenobiotic loads should be addressed, so that they are not carried unattended into the operating environment.
Use of laboratory analytics to query molecular networks
The general approach to pattern-focused assessment is not unlike the neonatal examination for inborne errors of metabolism, wherein a suite of metabolic markers is assessed to determine the potential origins of a specific functional or structural deficit (Tebani et al., Reference Tebani, Afonso, Marret and Bekri2016). Inborn error assessment is also performed so that any potential inherited errors of metabolism are detected early enough, whereby early intervention can be applied and adverse clinical outcomes can be prevented (or minimized).
In the case of human performance, one can query a suite of small molecules (<1,500 amu) for what might be appropriately deemed functional errors of metabolism. This may also include genetic variants that do not severely impact the phenotype though they may consistently and repeatedly lower metabolic network efficiency. The latter case may require specific intervention to enhance those networks (or pathways) or limit the adverse impact of an intermediary metabolite (e.g., homocysteine, adipate, suberate, superoxide).
Laboratory testing: Longitudinal and serial measures
Single time-point measures can be misleading if there is not a stable baseline developed. Moreover, the demands of long-term training and even short-term training can modify the levels of a range of molecular species. For instance, in our multi-omic work with the NASA Twins Study of One Year in Space (Garrett-Bakelman et al., Reference Garrett-Bakelman, Darshi, Green, Gur, Lin, Macias, McKenna, Meydan, Mishra, Nasrini, Piening, Rizzardi, Sharma, Siamwala, Taylor, Vitaterna, Afkarian, Afshinnekoo, Ahadi, Ambati, Arya, Bezdan, Callahan, Chen, AMK, Chlipala, Contrepois, Covington, Crucian, De Vivo, Dinges, Ebert, Feinberg, Gandara, George, Goutsias, Grills, Hargens, Heer, Hillary, Hoofnagle, VYH, Jenkinson, Jiang, Keshavarzian, Laurie, Lee-McMullen, Lumpkins, MacKay, Maienschein-Cline, Melnick, Moore, Nakahira, Patel, Pietrzyk, Rao, Saito, Salins, Schilling, Sears, Sheridan, Stenger, Tryggvadottir, Urban, Vaisar, Van Espen, Zhang, Ziegler, Zwart, Charles, Kundrot, GBI, Bailey, Basner, Feinberg, SMC, Mason, Mignot, Rana, Smith, Snyder and Turek2019), the SpaceX Inspiration4 mission (Overbey et al., Reference Overbey, Ryon, Kim, Schmidt, Schmidt, Narayanan and Mason2023) and the SpaceX Polaris Dawn mission, three to six baseline samples were analyzed. This approach obviates the need to rely on only one baseline measure to interpret the pre-mission status of a given individual. It also permits the clinician the ability to monitor the trajectory of changes that may occur in the run-up to a stressful deployment by comparing several baseline measures with one another.
Fundamentally, collecting serial measures over time (e.g., four to six times per year) creates the ability to describe molecular changes over the course of a training program, competitive season, mission in the field, or career. Importantly, serial testing also allows the clinician to identify patterns of variance post-mission, post-deployment, or post-season, so that corrective measures can be implemented to enhance recovery and optimize physiology in advance of the next operating deployment (mission, season; Pedlar et al., Reference Pedlar, Newell and Lewis2019).
Laboratory testing: Preanalytical factors
To assure the reliability and repeatability of analytical values, one must address pre-analytical factors that introduce the chance for false discoveries. Preanalytical factors are those factors involved in the procurement, storage, and transport of specimens before they are analyzed. It is the goal of PM to capture true biological variance to the greatest extent possible. This means removing as much experimental variance as possible. Experimental variance is comprised of those things that clinicians, technicians, couriers, and investigators do in order to capture the human biological specimens before they are analyzed. One of the most common pre-analytical errors regards improper specimen temperature or the introduction of freeze–thaw cycles (Kirwan et al., Reference Kirwan, Brennan, Broadhurst, Fiehn, Cascante, Dunn, Schmidt and Velagapudi2018).
An example of modeling experimental variance is batch effects. This might occur in a circumstance where team or crew samples are collected for analysis but are analyzed individually rather than in a single batch. This is a greater problem in untargeted multi-scale omics investigations use for research (high dimensionality), as opposed to the targeted analyses usually conducted in PM (Rasnic et al., Reference Rasnic, Brandes, Zuk and Linial2019; Stopsack et al., Reference Stopsack, Tyekucheva, Wang, Gerke, Vaselkiv, Penney, Kantoff, Finn, Fiorentino, Loda, Lotan, Parmigiani and Mucci2021). Another example is time of day collection. Time of collection should be kept consistent unless one intends to study the time-variance of individual analytes. Otherwise, one might model time rather than the analytes in question.
In general, one should take great care to create as much homogeneity across every step of the preanalytical process as possible. This is done by exerting tight process controls. Where processes cannot be controlled, careful annotation should be applied for use in post hoc analysis.
Assessing clinical and performance outcomes
A critical feature of PM in extreme environments is that the intervention be tied to measurable health and performance outcomes. This initially requires that one not be satisfied with the mere return of abnormal analytical values to the normal or optimal range. It is fundamentally important that objective and subjective measures of health, fitness, and performance be part of the foundation of this application of PM (see Tables 7 and 8). Since each unique operating environment will have its own conditions and requirements, the definition of what constitutes a suitable outcome will vary and must be customized to meet performance demands in those conditions.
Molecular class assessment in PM
Foundational to the practice of PM in extreme environments is the capture of a suite of analytes that provides a suitable picture across metabolic networks that is relevant to the environment or the task. While it is beyond the scope of this overview to outline the full extent of these measures, a minimal list of targeted analytes to consider is outlined in Table 4. In many cases, the mission or purpose may determine which tissue, fluid, or compartment is optimal for a given analyte. For instance, the authors prefer the reliability of red blood cells when assaying for fatty acid status. However, given the proper controls, plasma may be suitable. In general, plasma reflects day-to-day status, while RBC reflects long-term status over several months.
Table 4. Candidate molecular class analyses for functionally characterized networks
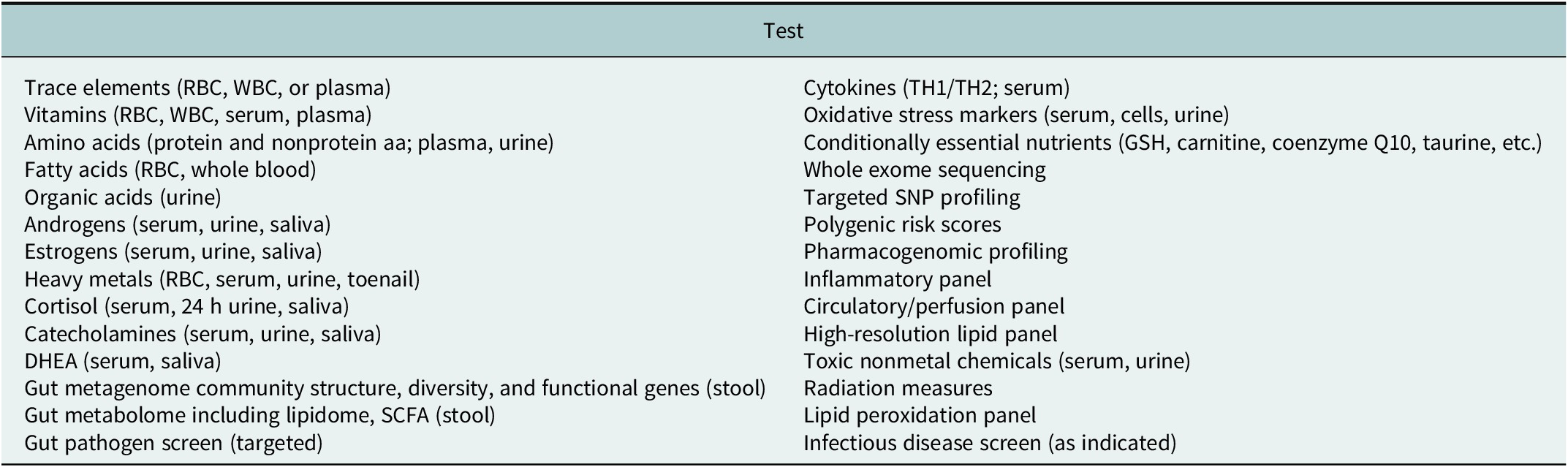
Abbreviaion: SCFA, short chain fatty acids.
Using functionally characterized networks: An example of PM concepts in practice
One approach to reducing complexity in PM is to evaluate FCN, wherein discrete boundaries are established around elements of a given pathway or network known to have a substantial impact on the clinical phenotype. FCN can be used with a number of constraint-based reconstruction and analysis methods to make predictions or provide insights into how a system changes in the face of variable influences (Rawls et al., Reference Rawls, Dougherty, Blais, Stancliffe, Kolling, Vinnakota, Pannala, Wallqvist and Papin2019). Specifically, constraints (boundaries) can be applied to individual metabolites, metabolic reactions, proteins, and genes to generate context-specific descriptions and predictions. While much of the FCN and reconstruction work lies within the field of computational biology, there is relevance in approaching PM and human performance by using such methods in clinical decision support.
The tryptophan–serotonin–kynurenine network: Mechanistic considerations
Below is one such FCN for the purpose of elucidating the thought process that can be considered for any metabolic network of clinical importance. This case example is the tryptophan–serotonin–kynurenine network. The tryptophan–serotonin–kynurenine network is a clinically actionable FCN that can be described using the systems model comprised of inputs, processes, and outputs. In principle, the network contains a primary essential input (tryptophan) that is directed down one of two pathways (Figure 4).
-
• Serotonin pathway: Tryptophan to serotonin to melatonin
-
• Kynurenine pathway: Tryptophan to kynurenine to quinolinic acid
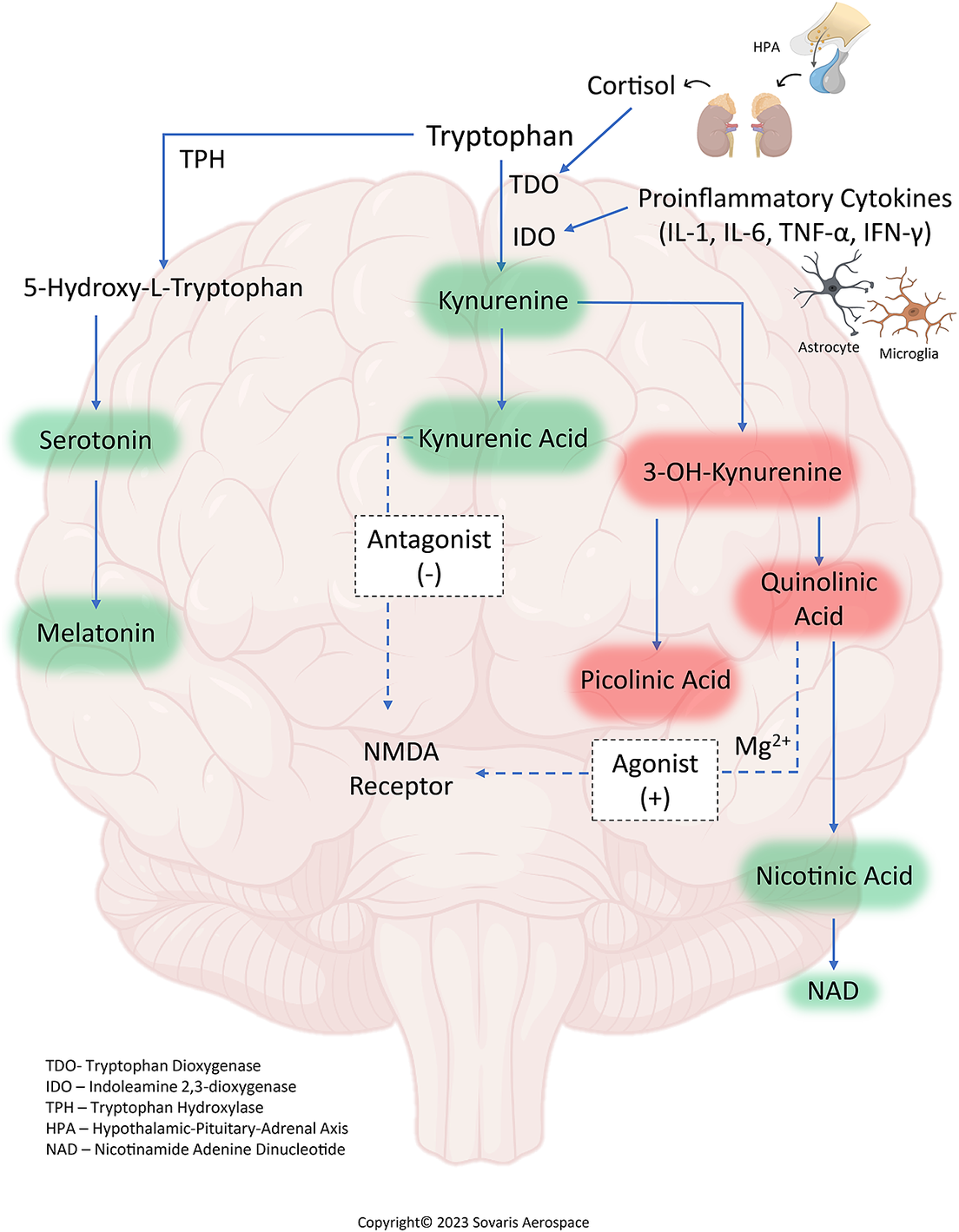
Figure 4. The tryptophan–serotonin–kynurenine molecular network in precision medicine (simplified). A key feature of this FCN is the competition for the tryptophan precursor (input) between the two pathways. Low availability of tryptophan feeding into the serotonin pathway results in low serotonin and low melatonin synthesis, which have notable effects on human physiology and behavior. This can occur via activation of the IDO or TDO enzymes, which diverts greater amounts of tryptophan toward a series of end products, notably kynurenic acid and quinolinic acid. These have the opposing effects of NMDA receptor antagonism and agonism, respectively. Note, however, that maintaining adequate Mg++ acts as a buffer against NMDA receptor activation and excitotoxicity, thus reflecting the importance of another essential input. IDO and TDO enzyme activation are driven by cytokines and corticosteroids, respectively. Compounds shaded in green are metabolically beneficial in most circumstances (neuroprotective). Those shaded in red are considered to be cytotoxic (neurotoxic) if they persist in elevated or high levels. Note that some QUIN can be subsequently converted into nicotinic acid (and then NAD+), a step that is evolutionarily conserved to play an important role in cellular energy metabolism (especially immune cell upregulation).
In this process, tryptophan is an essential amino acid precursor that can follow the tryptophan hydroxylase path to produce serotonin, which is critical for mood and influences the behavioral phenotype. This pathway accounts for less than 5% of available tryptophan in the network (Badawy, Reference Badawy2017). The next step in this path is the conversion of serotonin to melatonin, which is central to diurnal rhythms, mitochondrial function, immune function, behavior, and sleep in high-performance environments (Cipolla-Neto and Do Amaral, Reference Cipolla-Neto and Do Amaral2018).
A divergent path is the conversion of tryptophan to kynurenine via the indole dioxygenase (IDO) and tryptophan dioxygenase (TDO) enzymes (accounting for roughly 95% of tryptophan consumption in this network; Platten et al., Reference Platten, Nollen, Röhrig, Fallarino and Opitz2019). Here, tryptophan is converted to kynurenine by one of three rate-limiting enzymes: (1) indoleamine-2,3-dioxygenase-1 (IDO1), (2) indoleamine-2,3-dioxygenase-2 like protein (IDO2), or (3) tryptophan 2,3-dioxygenase (TDO). IDO enzymes are activated in response to inflammatory and immune signals, such as IFN-γ, TNFα, IL-1β, and IL-6. This activation pathway has been confirmed in the well-defined response to therapeutic IFN-α given for the treatment of hepatitis C, whereby serotonin levels fall, while KYN and QUIN levels increase in accordance with the emergence of depressive symptoms (Baranyi et al., Reference Baranyi, Meinitzer, Breitenecker, Amouzadeh-Ghadikolai, Stauber and Rothenhäusler2015; Pawlowski et al., Reference Pawlowski, Malyszczak, Inglot, Zalewska, Radkowski, Laskus and Pawlak2018). In contrast to IDO stimulation, TDO is activated through an increase in cortisol via the hypothalamic–pituitary–adrenal axis (Bhat et al., Reference Bhat, Pires, Tan, Babu Chidambaram and Guillemin2020) and is responsive to psychological stressors.
The tryptophan–serotonin–kynurenine network also contains other inputs that can be limiting. These consist of essential vitamin and mineral cofactors (riboflavin, niacin, pyridoxine, folate, ascorbic acid, zinc, iron, copper, magnesium, S-adenosylmethionine, etc.) that can only be derived from the diet and, when deficient, also influence downstream reactions in a limiting fashion (Majewski et al., Reference Majewski, Kozlowska, Thoene, Lepiarczyk and Grzegorzewski2016). This can render certain intermediates (5-hydroxytryptophan) and selected end products (melatonin) as conditionally essential. As a result, compounds such as 5HTP and melatonin become therapeutic candidates for consideration in regulating the network. The dynamics of this network are also heavily influenced or governed by nonessential inputs, such as infection, trauma, psychological stressors, sleep deprivation, and other mediators.
An illustration of the tryptophan–serotonin–kynurenine network dynamic in sport
To examine the utility of assessing kynurenine pathway metabolites in one group of people active in extreme training/competition environments, it is instructive to review concussion in American football players (Savitz et al., Reference Savitz, Dantzer, Wurfel, Victor, Ford, Bodurka, Bellgowan, Teague and Drevets2015a, Reference Savitz, Drevets, Smith, Victor, Wurfel, Bellgowan, Bodurka, Teague and Dantzer2015b; Meier et al., Reference Meier, Lancaster, Mayer, Teague and Savitz2017). For instance, football players with and without a history of prior concussion have demonstrated either a trend or significantly lower KYN when compared with controls. Meanwhile, plasma QUIN concentrations were elevated in American football players with prior concussion compared with those without concussion. Notably, an inverse association was observed between the neurotoxic metabolite 3HK and bilateral hippocampal volume in football players with prior concussion. Moreover, a positive association has been observed between the KYNA/QUIN ratio (an index of neuroprotection) and the left hippocampal volume in football players without prior concussion. This mirrors the hippocampal volume-kynurenine pathway relationships found in some psychiatric disorders (Meier et al., Reference Meier, Nitta, Teague, Nelson, McCrea and Savitz2020; Meier et al., Reference Meier, España, Nitta, Kent Teague, Brett, Nelson, McCrea and Savitz2021). In general, elevated QUIN is associated with a worse outcome (i.e., magnetic resonance imaging abnormalities, psychiatric symptoms, longer return to play, and death) in human studies of both mild and severe TBI (Meier and Savitz, Reference Meier and Savitz2022). Functions of individual metabolites in this network are summarized in Table 5.
Table 5. Metabolite functions in the tryptophan–serotonin–kynurenine molecular network

Therapeutic considerations in the tryptophan–serotonin–kynurenine network
This mechanistic knowledge is of clinical value because it allows one to describe the network with the specific goal of determining the suite of countermeasures needed to reestablish network regulation. Intuitively, for example, one seeking to raise serotonin levels would consider the use of oral tryptophan for the purpose of raising the tryptophan pool with the aim of upregulating endogenous serotonin and melatonin synthesis. However, the bulk of the tryptophan used in this manner (>95%) is likely to be diverted through the active IDO/TDO pathway, creating additional kynurenine metabolites with modest to variable impact on serotonin or melatonin production. Moreover, if the IDO/TDO enzymes are in an upregulated state, even more tryptophan can be expected to be shunted down the kynurenine pathway.
This is potentially problematic because, as noted, kynurenine metabolites such as quinolinic acid have neurotoxic properties in excess (Kadriu et al., Reference Kadriu, Farmer, Yuan, Park, Deng, Moaddel, Henter, Shovestul, Ballard, Kraus, Gold, Machado-Vieira and Zarate2021) and can be further elevated by increasing the tryptophan load. A clinical alternative to tryptophan in this instance is 5-hydroxytryptophan (5HTP) since this is not a substrate for IDO/TDO and would assure that therapeutic tryptophan (as 5HTP) is preferentially metabolized via the serotonin/melatonin pathway (initially via 5HTP decarboxylase). A brief conceptual overview of candidate interventions in this network can be seen in Table 6.
Table 6. Clinical targets of the tryptophan–serotonin–kynurenine network

The general approach to the TRP-SER-KYN network described herein can be applied to any FCN relevant to human health and performance. Thus, one can envision a suite of analytes that are used to build a separate set of FCNs that describe, for instance, one-carbon metabolism, fatty acid metabolism, acylcarnitine metabolism, amino acid metabolism, and many others. These FCNs can then be used to understand how network perturbation might be impacting performance and serve as a guide to countermeasure development.
The physiologic phenotype in PM
While this overview has focused on molecular applications in PM for the purpose of brevity, the importance of the physiologic phenotype warrants brief mention since these dynamics are not easily detectable by molecular profiling. Among the physiologic metrics most often used in extreme environment operations is heart rate variability (HRV). HRV is the variance in time between RR intervals of successive heartbeats, with the R wave reflecting depolarization of the main mass of the ventricles (Shaffer and Ginsberg, Reference Shaffer and Ginsberg2017). HRV is driven by nonlinear autonomic nervous system (ANS) processes and reflects a balance between the sympathetic (flight or fight responses) and parasympathetic (“rest and digest” or “growth and repair”) inputs.
HRV is highly individualized, which is one reason why it is a necessary foundation of PM in extreme environments. In general, the normal heart rate at rest for human adults is between 50 and 100 beats per minute, depending on the level of conditioning. Resting heart rate itself can provide long-term health prognostic information, while HRV changes from baseline can provide a more short-term health assessment (Arnold et al., Reference Arnold, Fitchett, Howlett, Lonn and Tardif2008; Jensen et al., Reference Jensen, Suadicani, Hein and Gyntelberg2013). The average HRV for a reasonably fit adult with a resting heart rate of 60 beats per minute would typically be 1 second, that is, a HRV score of 60, but actual HRV values could have a broader fluctuation depending on age and fitness.
Typically, higher HRV scores (80–90) are seen in younger adults, especially in elite athletes, while lower scores are found in the elderly and untrained. High sympathetic tone decreases HRV and eventually leads to fatigue and reduced performance, while parasympathetic activity increases the HRV, indicating recovery or readiness, and will predict increased performance (Fatisson et al., Reference Fatisson, Oswald and Lalonde2016). However, as Le Meur and colleagues found, HRV does not consistently change acutely as the phase of training and load evolves, and the duration of recovery period varies (Le Meur et al., Reference Le Meur, Pichon, Schaal, Schmitt, Louis, Gueneron, Vidal and Hausswirth2013). Higher HRV can be a sign that the body adapts well to changes in the environment and different levels of stress. Higher HRV measurements are also expected to occur when one’s heart rate is increased during physical activities like running. However, higher HRV can also be a sign of certain medical conditions, such as atrial fibrillation (AFib, an abnormal heart rhythm).
The categories of factors that can affect HRV include lifestyle, inflammation, medical conditions, age, fitness level, and pharmaceuticals. Deviations in HRV from an established baseline may be the most useful for health tracking in extreme environment operations. Importantly, such deviations, along with other tracked physiological parameters, could indicate an acute change in overall health or insight into the reasons for a drop in performance level.
While HRV is a measure of ANS function, it can also be explored for a linkage to metabolic networks. For instance, in a cohort of college students with depression, low HRV was associated with the disruption of coenzyme Q10 biosynthesis, glycine–serine–threonine metabolism, tyrosine metabolism, pyrimidine metabolism, and steroid metabolism, based on untargeted urinary metabolome (Zhao et al., Reference Zhao, Chi, Yan and Yao2020). Thus, HRV can be correlated with other PM measures and be fine-tuned to assess emotional status and readiness indicators. Other physiologic measures useful in personalizing to the physiologic phenotype are summarized in Table 7.
Table 7. Examples of physiological measures useful in extreme operations

Note: Herein is a selected list of tests that can be informative about attributes associated with an individual’s physiological phenotype. The list should be seen as a rudimentary example of the types of inventories that can be collected at baseline on all those operating in extreme environments and periodically during a season or post-mission. They can also be deployed (and compared with baseline) when there is a suspected deterioration in health or performance, or when there is an illness or incident. Importantly, assessment of the physiological domain is highly complex and there are many such measures that should be considered, so that the right assessment is chosen for the physiological indication, cohort, and the operating environment. Thus, the measures above can be seen as merely a basic starting point for general consideration.
Current efforts under development to further advance the field of (real-time) physiologic monitoring and countermeasure development in extreme environments include: (1) technological enhancement of performance by providing individual status information to optimize self-regulation, workload distribution, and enhanced team sensing/situational awareness; (2) detection of impending failure from stress load (physical, psychological, and environmental); (3) earliest possible detection of threat agent exposure that includes the “human sensor”; (4) casualty detection, triage, and early clinical management; (5) optimization of individual health and fitness readiness habits; and (6) long term health risk-associated exposure monitoring and dosimetry (Friedl, Reference Friedl2018).
The behavioral phenotype in PM
Extreme environments harbor the complex dynamic of conjunctive versus disjunctive tasks. Conjunctive tasks are those where teamwork and collaboration are needed in order to complete a successful effort (mission, operation, game). Success may be determined by how the weakest link performs and is rooted in a kind of collectivism where individual expertise is often subservient to the group. Disjunctive tasks are those where individual survival is at stake or where one team member with expertise must lead the group to safety or to success. Expertise must be highlighted, rather than erased.
Summiting a mountain on a well-established path benefits from conjunctive behavior, while an expert choosing the correct route to the safest descent (requiring unique expertise) is an example of disjunctive behavior. In practice, groups that tend to score higher in collectivism (e.g., Guatemalan’s score among the highest) have shown greater success at summiting but demonstrate reduced safety when diversity in expertise is high and enabling that expertise is low (Low et al., Reference Low, Setia and Lima2018).
Similar results have been found in a study where oxygen resources were low in a simulated crash landing on the moon. Oxygen was limited and there were two available escape routes (with one being shorter but riskier). The collectivists were good at dividing up the oxygen, though that approach harmed the groups in selection of the best escape route, as it encouraged people to ignore expertise on their team.
These are examples of nuances in the behavioral phenotype and how the sophisticated balancing of behavioral aptitudes (or training) must be refined in pursuit of success. While PM as commonly practiced adheres strongly to the pursuit of clarifying the molecular dynamics of an individual (and sometimes group), the behavioral phenotype is of such importance that it can never be ignored or taken for granted.
In fact, of all the phenotypic dynamics described in PM, behavior remains the only one over which an individual, group, team, or institution has complete control. Behavior affects everything from the choice of dietary resources, nutritional supplement adherence, physical activity, conformance to protocols, adherence to training, medication use, hydration, alcohol consumption, illicit drug use, self-care, getting social support, treatment of others, getting medical care, and a host of other activity. In practice, this really means that behavior significantly drives the inputs, processes, and outputs described above. In addition, behavior is used to observe and analyze the circumstances, then make adjustments and take appropriate action when outputs are off-nominal.
Notably, in extreme training and qualifying environments, it is commonly the behavioral dynamic that determines success versus failure. This is evidenced by the finding that 70–85% of enlisted men attempting to complete the BUD/S (Navy SEAL) program fail the basic underwater demolition screening, often because of the grueling phase called Hell Week. Similarly, only 31% of first-round draft picks selected between 2010 and 2017 in the National Football League draft signed a second contract with and the team that drafted them (33rd Team, 2023). Much of this is attributed to the mental grind and the psychological difficulty of competing at this level (though injury surely comes into play).
While the behavioral phenotype influences almost all activity in an extreme operating environment, it must also be acknowledged that the underlying molecular dynamic also shapes the behavioral phenotype. One needs only recall the dynamics of the tryptophan–serotonin–kynurenine molecular network to see how perturbations at the molecular level may influence the behavioral phenotype. Table 8 explores a limited set of measures of the behavioral phenotype that can be used to monitor behavioral dynamics over the trajectory of short- and long-time series for those engaged in PM applications.
Table 8. Examples of behavioral measures useful in extreme operations

Note: Herein is a selected list of tests that can be informative about attributes associated with an individual’s behavioral phenotype. The list should be seen as a rudimentary example of the types of inventories that can be collected at baseline on all those operating in extreme environments and periodically during a season or post-mission. They can also be deployed (and compared with baseline) when there is a suspected deterioration in health or performance, or when there is an illness or incident. Importantly, assessment of the behavioral domain is highly complex and there are many such inventories that should be considered, so that the right instrument is chosen for the cohort and the operating environment. Thus, the tools above can be seen as merely a basic starting point for general consideration.
It bears noting that, while the behavioral phenotype strongly shapes one’s capability to thrive in an extreme environment, the extreme environment can profoundly shape the behavioral phenotype. This was shown, for example, when Stahn et al. (Stahn et al. Reference Stahn, Gunga, Gallinat and Kuhn2014; Stahn and Kühn Reference Stahn, Gunga, Kohlberg, Gallinat, Dinges and Kühn2019) followed nine polar expeditioners during 14 months at the German Neumayer III station in Antarctica. Antarctica is considered an essential analog condition for studying several parameters of human spaceflight, as well as isolation on Earth.
High-resolution MRI showed that volume in the dentate gyrus of the hippocampus was reduced by 7.2% in expeditioners versus controls. These reductions in dentate gyrus volume were also strongly correlated with lower cognitive performance in tests of spatial processing and selective attention. This suggests that the changes in dentate gyrus volume did not impair general cognitive performance but did affect response conflict and spatial short-term memory processing of object location.
Of note, changes in hippocampal volume also correlated with decreased serum brain-derived neurotrophic factor (BDNF). Whole-brain imaging in expeditioners further showed a mean decrease in gray-matter volume in the left orbitofrontal cortex, right dorsolateral prefrontal cortex, and left parahippocampal gyrus.
The NASA Twins study of One Year in Space followed one astronaut in space for 340 consecutive days, while his monozygotic astronaut twin was followed on Earth using the same multi-scale omics, physiologic, morphologic, cognitive, and behavioral measures. For the space-bound astronaut, cognitive accuracy and speed scores were measured for the different phases of the mission (pre-flight, in-flight months 1–6 (early flight), in-flight months 7–12 (late flight), and post-flight) (and mirrored on Earth).
In the cognitive domain, speed decreased in space for all tests except for the digital span substitution test, and accuracy decreased for all domains except for spatial orientation post-flight. In space, cognitive efficiency (a combination of speed and accuracy across cognitive domains) was similar pre- and in-flight relative to the Earth control but was significantly lower post-flight (Garrett-Bakelman et al., Reference Garrett-Bakelman, Darshi, Green, Gur, Lin, Macias, McKenna, Meydan, Mishra, Nasrini, Piening, Rizzardi, Sharma, Siamwala, Taylor, Vitaterna, Afkarian, Afshinnekoo, Ahadi, Ambati, Arya, Bezdan, Callahan, Chen, AMK, Chlipala, Contrepois, Covington, Crucian, De Vivo, Dinges, Ebert, Feinberg, Gandara, George, Goutsias, Grills, Hargens, Heer, Hillary, Hoofnagle, VYH, Jenkinson, Jiang, Keshavarzian, Laurie, Lee-McMullen, Lumpkins, MacKay, Maienschein-Cline, Melnick, Moore, Nakahira, Patel, Pietrzyk, Rao, Saito, Salins, Schilling, Sears, Sheridan, Stenger, Tryggvadottir, Urban, Vaisar, Van Espen, Zhang, Ziegler, Zwart, Charles, Kundrot, GBI, Bailey, Basner, Feinberg, SMC, Mason, Mignot, Rana, Smith, Snyder and Turek2019) and persisted for several months thereafter. The general conclusion from these and other cognitive findings in space is that continuous long-duration operations can have detectable effects on cognition and shape the behavioral phenotype (Stahn and Kühn Reference Stahn and Kühn2021).
Multi-omics research enables PM
The future of PM in extreme environments will depend on better understanding the biological response of humans in these conditions, generating new hypotheses, and developing precision countermeasures. One means to accelerate this process of discovery is the incorporation of multi-omics assessment across the many extreme environment domains. But this must be undertaken with the knowledge that subject numbers are frequently small, while variable (analyte) numbers are high. Such multivariable studies involving high dimensional data sets require extensive pre-analytical controls, as well as disciplined multivariate statistical methods in order to avoid false discoveries.
In the NASA Twins Study of One Year in Space (N = 2), we were involved in the first multi-omics study deployed in the spaceflight environment, (Garrett-Bakelman et al., Reference Garrett-Bakelman, Darshi, Green, Gur, Lin, Macias, McKenna, Meydan, Mishra, Nasrini, Piening, Rizzardi, Sharma, Siamwala, Taylor, Vitaterna, Afkarian, Afshinnekoo, Ahadi, Ambati, Arya, Bezdan, Callahan, Chen, AMK, Chlipala, Contrepois, Covington, Crucian, De Vivo, Dinges, Ebert, Feinberg, Gandara, George, Goutsias, Grills, Hargens, Heer, Hillary, Hoofnagle, VYH, Jenkinson, Jiang, Keshavarzian, Laurie, Lee-McMullen, Lumpkins, MacKay, Maienschein-Cline, Melnick, Moore, Nakahira, Patel, Pietrzyk, Rao, Saito, Salins, Schilling, Sears, Sheridan, Stenger, Tryggvadottir, Urban, Vaisar, Van Espen, Zhang, Ziegler, Zwart, Charles, Kundrot, GBI, Bailey, Basner, Feinberg, SMC, Mason, Mignot, Rana, Smith, Snyder and Turek2019; Schmidt, Reference Schmidt2019; Schmidt et al., Reference Schmidt, Meydan, Schmidt, Afshinnekoo and Mason2020a, Reference Schmidt, Meydan, Schmidt, Afshinnekoo and Mason2020b, Reference Schmidt, Meydan, Schmidt, Afshinnekoo and Mason2020c). It was notable in providing insight about the molecular, physiologic, and behavioral phenotypes in spaceflight due to its long duration of 340 days and its extensive serial measures (19). It also provided insight into the spectrum of methods needed to properly conduct omics experiments in this environment.
This study was followed by the Everest Twins Study (N = 4), which used similar multi-omics measures to the NASA Twins Study (Luxton et al., Reference Luxton, McKenna, Lewis, Taylor, George, Dixit, Moniz, Benegas, Mackay, Mozsary, Butler, Bezdan, Meydan, Crucian, Zwart, Smith, Mason and Bailey2020) but studied two twin pairs on a Mt. Everest Summit versus sea level. This provided another opportunity to study the biology and the methods, with the harmonization of methods affording the ability to compare two extreme environments (albeit one without the impact of microgravity).
More recently, our team was involved in the multi-omics component of the all-civilian SpaceX Inspiration4 mission (September 2021). This mission differed from the Twins study in that it was only a three-day orbital flight involving four people. It utilized many of the same multi-omics measures as the NASA Twins Study (excepting for specific in-mission analytics), though lessons from the Twins Study informed new design elements that rendered the Inspiration4 mission the most extensive multi-omics spaceflight investigation performed at that time (Overbey et al., Reference Overbey, Ryon, Kim, Schmidt, Schmidt, Narayanan and Mason2023).
A similar multi-omics approach has been taken by our team in the five-day SpaceX Polaris Dawn Mission (N = 4) and the nine-day SpaceX Axiom-2 Mission (N = 4). Polaris Dawn includes the first civilian spacewalk and the highest altitude (1,400 km) reached by an astronaut since the Apollo Moon missions. The Axiom-2 mission is characterized by four civilian astronauts reaching and working on the ISS.
It is important to note that retained specimens from each of these missions are archived in a biobank, which serves as a resource for future discovery. In addition, data from these studies is being archived (to the greatest extent permitted by informed consent), so that it may be used by investigators in the future. The ultimate goal of these efforts is translational. We hope to better understand the molecular dynamics of humans operating in extreme environments with the focused aim of translating that knowledge into actionable tools of PM.
Conclusion
This overview presents one conception of how PM can be approached, based on a systems biology or systems engineering perspective. PM applied to humans in extreme environments is centered on understanding the molecular dynamics of an individual, while also considering the physiological and behavioral phenotypes. In parallel, close attention must be given to the unique environmental conditions and personal exposures encountered in the operating environment itself.
In general, PM in extreme environments is focused on pattern analysis rather than diagnosis, though the diagnosis is appropriate wherever indicated. A foundation of the molecular approach is to first assure that knowable, actionable molecular dynamics (deficiency or excess) are identified as central to the development of methods of intervention. This frequently takes the form of addressing substrates, precursors, intermediates, and cofactors commonly in the form of macro and micronutrients. In many cases, correction of these functional errors of metabolism may obviate the need for drugs or modify drug selection in important ways.
When drugs are utilized, it is done so with careful consideration of their impact on metabolic networks via attention to drug–gene, drug–drug, drug–herb, drug–food, and drug–nutrient interactions. Proceeding with a drug intervention in the absence of querying these networks elevates the likelihood that a chosen drug intervention may merely target the epiphenomenon of these deficits or excesses.
The assessment of molecular dynamics should encompass the measurement of inputs, processes, and outputs by examining a range of FCN. Input analysis should consider essential, conditionally essential, and nonessential inputs. The exposome (e.g., drugs, xenobiotics) warrants examination, since these nonessential inputs can exert notable and, often, predictable effects upon molecular networks.
In parallel, examination of the physiological and behavioral phenotypes using sensitive measures affords one the ability to assess these endophenotypes in ways that molecular analytics cannot. This is done with the knowledge that it is the molecular dynamics that frequently underlie these phenotypes. Conversely, aspects of the physiological and behavioral phenotype can also act as drivers of the molecular phenotype and, thus, performance in extreme environments. This has been demonstrated, for instance, by the considerable rates of failure for individuals entering selection programs to become elite performers in extreme environments (e.g., military special forces) which is often attributed to issues of behavioral resiliency.
In conclusion, we advance the premise herein that knowable, actionable metabolic network deficits should always be addressed for those who function in extreme environments, so that those deficits are not carried into the operating environment in a manner that adversely impacts performance, recovery, health, and safety. While advancements in the field are clearly needed, it is reasonable to assert a basic order of operations guiding the manner in which PM applications can be viewed in the present. This includes (1) those that are actionable today, (2) those that are expected to be actionable in the near term, pending additional research, and (3) those expected to be actionable in the distant future, thus requiring more extensive research. Finally, though this PM overview is heavily weighted toward clinician concerns, we must always give priority to that which matters most to the individual who chooses to operate in extreme environments.
Abbreviations
- AA
-
arachidonic acid
- BUD/S
-
basic underwater demolition SEAL training
- DHA
-
docosahexaenoic acid
- EPA
-
eicosapentaenoic acid
- FAD
-
flavin adenine dinucleotide
- FAD1
-
fatty acid desaturase 1
- HFE
-
hemochromatosis gene test for the genes C282Y or H63D
- hsCRP
-
high-sensitivity C-reactive protein
- IL-1β
-
interleukin 1 beta
- IL-1RA
-
interleukin 1 receptor antagonist protein
- IL-6
-
interleukin 6
- IL-8
-
interleukin 8
- IL-10
-
interleukin 10
- IL-12p40
-
interleukin 12p40
- INFγ
-
interferon gamma
- IU
-
international unit
- MCP-1
-
monocyte chemotactic protein 1
- NAD
-
nicotinamide adenine dinucleotide
- NADP
-
nicotinamide adenine dinucleotide phosphate
- NBA
-
National Basketball Association
- NFL
-
National Football League
- NMDA
-
N-methyl-d-aspartate
- PEMT
-
phosphatidylethanolamine methyl transferase
- sCD40L
-
soluble CD40L
- SEAL
-
United States Navy sea, air, and land teams
- sICAM-1
-
soluble intercellular adhesion molecule 1
- SNP
-
single nucleotide polymorphism
- SWAT
-
special weapons and tactics police unit
- Targeted
-
a method of molecular analysis where the analytes are pre-determined and calibration curves are generated for each individual analyte. Generally used for a smaller number of analytes
- TCA
-
tricarboxylic acid cycle
- TNFα
-
tumor necrosis factor alpha
- Untargeted
-
a method of molecular analysis where the analytes are not pre-determined and calibration curves are not generated for each individual analyte. It is often referred to as a nonhypothesis-driven method, wherein hundreds to thousands of analytes may be assessed at one time
Open peer review
To view the open peer review materials for this article, please visit http://doi.org/10.1017/pcm.2023.16.
Acknowledgments
A special thanks to Marc A. Shepanek, Ph.D., NASA Office of the Chief Health and Medical Officer, Washington DC, USA, for his contribution as an editor and research advisor in the overall project, and for his support in writing and reviewing the manuscript. Thanks also to Caleb M. Schmidt, M.S., Julian C. Schmidt, M.S., and Robert Hubbard, Ed.D. of Sovaris Aerospace for their contributions to manuscript support. Selected elements of Figures 1–4 were obtained from BioRender.com. Figures 1–4 were designed and produced by Sovaris Aerospace.
Author contribution
M.A.Sc. led the conception of the work, interpretation of data for the work, drafting of the work and conclusions. M.A.Sc. and J.A.J. led the development of the section on the physiologic phenotype. M.A.Sc. led and M.A.Sh. contributed to the development of the section on the behavioral phenotype. M.A.Sc. and C.E.M. led the sections on multi-scale omics and microbiome. M.A.Sc., J.A.J., M.A.Sh., and C.E.M. reviewed the manuscript for content, accuracy and approval of the final version. All authors are accountable for all aspects of the work in ensuring that questions related to the accuracy or integrity of any part of the work are appropriately investigated and resolved.
Financial support
This research received no specific grant from any funding agency, commercial, or not-for-profit sectors.
Competing interest
M.A.Sc. is the founder of Sovaris Aerospace. J.A.J. has no conflicts of interest to declare. C.E.M. is a cofounder of Onegevity Health.
Comments
No accompanying comment.