Policy Significance Statement
In an increasingly data-driven society, it is of utmost importance to develop and maintain reliable data management structures. Recently, we have witnessed the arrival of trust-based data management models, such as: information fiduciaries, data curators and stewards, and data trusts. It is crucial that policymakers understand the advantages as well as the pitfalls of these models in order to enable a responsible uptake. This article provides researchers and policymakers with in-depth knowledge on the functioning of these trust-based models. In particular, it will suggest several principles that should be taken into account when the data trust model, which this article foresees to have the most impact, is implemented. These insights will enable policymakers to make well-considered choices both on the policy as well as on the practical level.
1. Introduction
There exist two ideal-typical models of data regulation. The first one is to give control to individuals over their personal data. In its most extreme form, scholars have suggested that if individuals would gain property or other control rights over their data (Samuelson, Reference Samuelson2000), they would be able to adequately represent and protect their own interests against the multinationals and governmental organizations that intend to use their data (Mun et al., Reference Mun, Hao, Mishra, Shilton, Burke, Estrin, Hansen and Govindan2010). This model has clear advantages as it grants citizens autonomy over their personal data and steers away from any form of paternalism. In addition, it sets no absolute boundaries on what organizations can and cannot do with personal information but connects that question to each individual’s preferences, which may vary significantly per person (Lazaro and Metayer, Reference Lazaro and Metayer2015).
This model also has clear disadvantages. The capacity of citizens to make choices according to their best interests is limited in practice both because of the complexity of most contemporary data-driven processes involving biometric data, artificial intelligence, and profiling, because of the multitude of processes which contain the data of an average citizen, and because of the information-asymmetry between data-driven organizations and the average citizen (Cate and Mayer-Schönberger, Reference Cate and Mayer-Schönberger2013). Even if citizens would get all relevant information, removing the information asymmetry, and even if they would understand all the data technologies and potential consequences of the data processing initiatives just as well as the parties operating those techniques, which seems unlikely to say the least, citizens simply do not have the time to assess for each of the on average 5.000 organizations that process their data whether such is done correctly, legitimately and if not, to go through a complex, lengthy and often costly legal procedure. In addition, many of the data-driven processes affect large groups in society or the population in general; leaving it to each and every individual citizen to assess such processes and their potential flaws individually would mean a privatization of structural problems and would result in well-educated citizens protecting their personal data better than would already marginalized groups (Lanzing, Reference Lanzing2016).
A second model is to rely on legal standards and governmental enforcement of those standards. Just like there are minimum safety requirements for cars—a citizen can simply not legitimately buy a car that does not meet the legal safety standards—there are minimum requirements for legitimately processing personal data. It is not left to citizens to assess whether these rules are met, but to an independent governmental organization, who has the authority to both investigate data-driven organizations and set sanctions and fines when they violate the rules. This means that legal protection is provided to citizens, without them having to assess the validity, legality, and desirability of each individual data process that contains her data on her own.
However, this model too has its particular disadvantages. Companies stress that legal standards are oftentimes too restrictive, citizens may be limited in having their data processed against their will and legal standards are often too general, absolute, and inflexible and easily become outdated in the constantly developing data-driven environment (Zarsky, Reference Zarsky2016). In addition, it is practically impossible for one governmental organization to assess all data processing operations (Bennett, Reference Bennett2018) and difficult to ensure that parties based in other territories adhere to national standards. This means that supervisory organizations, such as Privacy Commissioners and the Data Protection Authorities in Europe, usually only focus on the bigger data processing operations, that have the biggest potential impact.
Obviously, these are two ideal models, which do not exist as such in legal practice. Still, it is evident that they have inspired regulatory approaches. Roughly speaking the American approach to privacy regulation, with its focus on notice and consent, is more akin to the first model. Still, there are several elements to be found of the second model in the American approach as well, such as can be exemplified by the recent bans on facial recognition (Conger et al., Reference Conger, Fausset and Kovaleski2019) and deepfakes (Sasse, Reference Sasse2018) and the restrictions set out by COPPA.Footnote 1 By and large, the European approach to privacy regulation is more akin to the second ideal model, although consent and individual rights have a significant bearing on both the General Data Protection RegulationFootnote 2 and the Convention 108.Footnote 3 On both sides of the Atlantic, regulators are struggling to address the rapidly evolving technological developments and increasing power of information intermediaries. Both approaches have so far proved to be imperfect matches.
That is why a third regulatory model has been proposed in recent years: a trust-based model. This approach can take various shapes. For example, organizations increasingly appoint a person within their organization that has an independent role and the task of safeguarding the interest of citizens affected, as well as general interests and to some extent the interest of other organizations that might want to reuse the data. In addition, large information intermediaries have been suggested to have fiduciary obligations or duties of care. Because they have such a broad power over citizens and because there is an information asymmetry between them, they not only have to stick by the choice of citizens or adhere to the prevailing legal norms, but also have to use their power to further the interests of citizens. To provide a final example, data trusts have been introduced. These are independent organizations to which citizens entrust their data and to which organizations that want to process these data can turn for partial access.
This third model holds several promises. First, an advantage over the first model, citizens no longer need to give their informed consent for specific processing activities; rather, the trusted partner can act on the citizen’s behalf. Second, as an advantage vis-à-vis the second model, it places a primary responsibility on organizations that have possession over the data instead of the data protection authority. Third, ethical duties of care and fiduciary obligations are broader and more general than both the legal standards and the informed consent given by the citizen. Thus, instead of having to update regulatory standards or the informed consent when new technologies emerge, the general ethical duties will cover these new opportunities. Fourth and finally, both the informed consent of the citizen and current regulatory regimes only concern citizens’ personal data, while many of the newer data processes run on group data, aggregated data, and nonpersonal data (Taylor et al., Reference Taylor, Floridi and Van der Sloot2016). Ethical duties do not center around the question whether an organization processes personal data or not, but around whether it has (data) power.
Hence, trust-based models may fill the gaps of currently existing regulatory models. Clearly, however, there are also various downsides and pitfalls with trust-based regulation. Are trust-based approaches used to strengthen existing regulatory strategies by providing additional duties of care or is it a way to steer around restrictive legal provisions? Who ensures that trusted parties do in fact act in a fiduciary way and who decides what falls under a duty of care and what not? Which interests should fiduciary organizations further: only those of specific data subjects or those of society in general? And what if these two interests clash?
This article will explore those questions and explore which benefits and pitfalls a trust-based model may have. First, a theoretical introduction in the notion of trust is provided (Section 2). Then, three trust-based structures will be explored in further detail: that of information fiduciaries (Section 3), data curators (Section 4), and data trusts (Section 5). Each will be described in further detail, examples will be provided of how they are deployed in practice and a trust-based analysis will shed light on the potential risks and benefits of these approaches. Finally, the concluding paragraph will shed further light on the specific model of data trusts, as this is the use case that can have the biggest impact on citizens and society at large. This article will suggest several principles that should be taken into account when the data trust models are implemented in practice and assess several challenges in further detail (Section 6). The full development of such a framework falls outside the scope of this article. Instead, the broad contours and relevant aspects such a framework should set out are mapped.
The conceptual distinctions drawn between information fiduciaries, data stewards, and data trusts are presented here as clearly distinguishable models, while in fact, the lines are often blurry and contested on the ground. This article does not engage with the unruliness of each term and the full extent of overlap between them on the ground. For instance, a number of works and public remarks on data trusts suggest that fiduciary law should be a vehicle to realize their vision (Delacroix and Lawrence, Reference Delacroix and Lawrence2019). Data trusts as posited in their bottom-up work argue for subject-initiated trusts, whereas in other models it is treated as a top-down regulatory form. This article will treat the three categories of trust-based regulatory models discussed in Sections 3–5 as ideal models, with clearly demarcated characteristics, in order to provide a normative analysis, while accepting that in practice, these models are not as clearcut as presented here.
2. Trust
Trust-based regulatory strategies have several basic components.Footnote 4 First, trust is deeply connected to our social disposition. Human beings are social beings. This “ontological togetherness” is first of all a matter of functional necessity. Everyone depends on others to some extent: providing food, a place to live, building roads, and so forth (Simpson, Reference Simpson2012). In addition, humans typically rely on reciprocal relationships to flourish in life; a life that is meaningful in the sense that it is driven by key values such as self-development, friendship, and compassion.
A key aspect of this multifaceted dependency is that we cannot control the actions of others, just as we cannot demand friendship or compassion. People have—to a certain extent—the freedom to behave in unexpected and even undesirable ways. They can prioritize their own gains above ours, they can pretend to care, they can lie, or they can simply make an honest mistake causing us grave harm. This fundamental uncertainty brought forth by the intentions and actions of others would be detrimental to everyday life if we were not able to neutralize it in some way. Trust can be seen as a strategy to deal with this uncertainty (Luhmann, Reference Luhmann and Davis1979).
When we trust someone, we have positive expectations of their actions. We assume that they will not take advantage of our vulnerability and that they will look after our interests. To trust is to embrace uncertainty, rather than diminishing it. It is acknowledging that one can get hurt, in the broadest sense of the word: physically, mentally, and financially, while simultaneously expecting that others will not abuse this weakness. In that sense, trust is a “risky business.” If there would be nothing at stake, nothing to lose, trust would be redundant.
On an epistemological level, one could say that trust predominantly is characterized by “not knowing,” where this “not knowing” does not prove to be a hindrance for action. Nevertheless, while trust might be about “not knowing” and therefore to a certain extent be blind, it is not completely sightless. We do look for cues to assess someone’s trustworthiness. We base ourselves on past experiences, testimonies of others, promises being made, and the expectations that come with a certain social role—it would be very disturbing if my GP suddenly starts advising me on my mortgage instead of my health—and we anticipate the possibility of shifting roles in the future; in the end, a trustee may well become a trustor one day. Reciprocity and “the shadow of the future” can be important incentives to act trustworthy (Axelrod, Reference Axelrod1984).
Trust can also be situated in impersonal systems: for example, the public transport, a bank, or a hospital (Luhmann, Reference Luhmann and Gambetta1988). These systems or organizations are way too complex for a lay person to overlook or understand. Generally, we know how to make use of them—we know what to do with our public transport card and how to wire money—but how these processes are exactly executed, we do not have a clue. The trust cues that accompany system trust are somewhat different from those guiding interpersonal trust. For instance, when interacting with big companies or institutions, we expect there are experts involved who check these complex processes on behalf of us. We assume that the education of the doctors working at the hospital is of an excellent standard. We rely on oversight boards and regular audits to keep an eye on expert systems (Luhmann, Reference Luhmann and Davis1979; Giddens, Reference Giddens1990; Giddens, Reference Giddens1991; Giddens and Pierson, Reference Giddens and Pierson1998).
The majority of the population lacks the expertise that is required to comprehend what is at stake in data-driven processes and to subsequently decide on how to look after their interests. Moreover, also the human representatives of the system, who often have the role to smoothen the interaction, have to make way for technical mediations (Keymolen and Voorwinden, Reference Keymolen and Voorwinden2019). From screens to voice assistants, these devices are increasingly becoming quasi-others with whom citizens have to interact. While these devices are often designed to be user-friendly, they simultaneously also hide that which is really at stake—how is privacy being safeguarded? How fair and accurate is the automated decision-making process? While trust is always blind to a certain extent, the data-driven environment hides many of these risks from view. Citizens become visible and therefore oftentimes vulnerable in a, to them, invisible way. This invisible visibility makes it hard for citizens to look after their own interests, if it were only because of the power imbalance this creates.
Current regulatory regimes address this problem. On the one hand, they empower data subjects, by providing them with data subject rights, such as a right to erasure and a right to data portability to gain more control over what happens with their data (model one of the introduction). On the other hand, by imposing all kinds of standards on data controllers, regulation limits the action space of those public and private actors that have the data-processing tools at their disposal (model two of the introduction). Both these regulatory strategies are not so much based on trust, as they are on control (van den Berg and Keymolen, Reference van den Berg and Keymolen2017).
In conclusion, to speak meaningfully of trust, we speak of trust relations, as there are at least two actors involved, referred to as a trustor and a trustee. In this relation, the former depends on the latter to do something, to perform an action which is of importance to the trustor. In a general sense, we could say that a trust relation takes the following shape: A (trustor) depends on B (trustee) to do x, where x is important to A. In this dependency relation, A has positive expectations of B’s actions. This trust relation is embedded in a social context, which currently is often globalized and data-driven. The trustee, therefore, is not necessarily a human being, but can also be a technology or organization. It is this tri-partite relationship (A trusts B to do X) that underpins all three trust-based data governance models. However, each model has its own specific relational arrangements and consequently also its own advantages and challenges. Looking at these data governance models through the lens of trust, enables us to unpack some of these inherent challenges and vulnerabilities and provide some suggestions on how to implement these models in practice.
3. Information Fiduciaries
3.1. Introduction
One way of formulating ethical responsibilities for parties processing personal data is to attribute to them fiduciary responsibility (Schwartz and Cohn, Reference Schwartz and Cohn2018). Fiduciaries are persons or organizations with which a citizen has a trust relationship; a number of ethical duties derive from such relationships, that can be understood as duties of care. Because our relationship with companies like Google, Facebook, and Apple is characterized by vulnerability and dependency, they should be seen as information fiduciaries.Footnote 5 Hence, special, fiduciary obligations of loyalty and trustworthiness should be bestowed on these companies (Waldman, Reference Waldman2018).
The concept has been used for legislative reforms, among others in California’s Consumer Privacy law,Footnote 6 the failed New York Privacy Bill (Lapowsky, Reference Lapowsky2019) and India’s Data Privacy Bill (Greenleaf, Reference Greenleaf2020). Although so far, the concept has been used in Common Law systems, in the European context, duties of care have been proposed to impose on information intermediaries obligations to go beyond the specific legal requirements. In the European context, much in a similar vein, the introduction of virtue rules for large data organizations has been advocated. “Big Data processes have an effect on a more general and societal level, which rights-based models cannot adequately address but a virtue-based regulatory model can. Open norms may also have the additional benefit that they are not directed at one particular player, but rather at data controllers in general. One of the problems faced under the current regulatory regime is that it is often unclear where the responsibilities lie, because data are shared between many different organizations, and subsequently combined, harvested and used” (Van der Sloot, Reference Van der Sloot2017).
3.2. Promises
One key promise of fiduciary relations is that they enable citizens to navigate a complex data-driven society. Fiduciary relationships are a clearcut instance of a trust relation and although the introduction of fiduciary relationships in the data-driven context seems new, the suggestion is as old as the very existence of information markets. For example, already in 1996, Laudon argued that because “most people would not have the time or interest to participate directly in information markets, a role would emerge for information fiduciaries, or agents acting on their behalf who assume certain legal responsibilities. [] Like banks, they [the information fiduciaries] would accept deposits of information from depositors and seek to maximize the return on sales of that information” (Laudon, Reference Laudon1996, as cited in Brooks, Reference Brooks2015). Fiduciary principles entail that trustees only act in the interests of the beneficiary that has entrusted them with their power and resources (Catanzariti, Reference Catanzariti2019). A patient (A) goes to a doctor (B) and shares personal data with the doctor because she believes the doctor will use that knowledge to her benefit and not to its own (x). So too, an information intermediary should act, the argument goes.
The reason for suggesting this regulatory model is that it might overcome several limitations of current regulatory regimes (Richards and Hartzog, Reference Richards and Hartzog2015). Legal rules are per se general and cannot follow every new technological development instantly; there is a large data asymmetry when data subjects are requested to give consent, so that the basic presumption of the market—namely that when provided adequate information and control rights, customers will be able to further their own interest—might not work in the data context (Balkin and Zittrain, Reference Balkin and Zittrain2016). That is when information intermediaries should be held to have violated their fiduciary duty when they abuse users’ trust by: “(a) using their data to manipulate them; (b) using their data to discriminate against them; (c) sharing their data with third parties without consent; or (d) violating their own privacy policies” (Dobkin, Reference Dobkin2018). In addition, in the US, where the notion of information fiduciary has gained momentum, one of the underlying reasons for introducing the notion of information fiduciaries is the third-party doctrine. This doctrine roughly suggests that people have no reasonable expectation of privacy when data are shared with third parties, while people share data with information companies and expect them to keep their data private and not share them with others. “If I am right that new digital online service providers may be new kinds of information fiduciaries, then we should have reasonable expectations of privacy in at least some of the information about ourselves that we share with them. [I]f we give information to an information fiduciary, the third-party doctrine should ordinarily not apply, and the information should not fall outside of the protections of the Fourth Amendment” (Balkin, Reference Balkin2016).
3.3. Challenges
There is, however, also a number of challenges with the notion of information fiduciary. First, how does one determine whether an organization is in fact a fiduciary organization? Baily suggest that generally speaking, a fiduciary relationship, as a specific instance of a trust relation, exists where (a) the beneficiary has a need to achieve certain ends that society considers valuable, (b) the fiduciary holds herself out as able to achieve these ends, (c) the beneficiary has no or limited ability to monitor the fiduciary, and (d) the fiduciary is in a position to unilaterally act to the detriment of the beneficiary (Bailey and Goyal, Reference Bailey and Goyal2019). Alternatively, Demuro and Petersen suggest that a number of questions should be used to determine whether an organization should be considered an information fiduciary such as: Does the individual whose data is generated have an expectation of privacy and confidentiality with respect to that data? How sensitive might the individual believe her data is? Was the form in which the data was transmitted such that an individual would reasonably expect that it would only be received and used by the party intended? Will the data be used to provide better care for the individual whose data it is, or be for the common or public good, or will it be commercialized for the good of the holder of the data? Would the individual whose data are concerned have consented to the eventual use, if she knew what that use was? What is the value of the data? Can the data truly be deidentified and not reidentified (Demuro and Petersen, Reference Demuro and Petersen2019)?
Answering these kinds of questions is far from an exact science, which may make it difficult to use and implement such models in legal practice (Frankel, Reference Frankel1983). Second, and much related, how does one determine what a fiduciary organization should do; what are then the duties of care that are attributed to large data-driven organizations? And perhaps more importantly: who determines this? If it is left to citizens, companies might be imposed with unreasonable obligations, if it is left to organizations themselves, nothing may come from it. If the ultimate decision is left up to a regulator of the court of law, the question will be how this model is different from the normal rules contained in the various privacy laws.
Third, Arora (Reference Arora2019) suggests that fiduciary principles have traditionally served to fill the gaps in laws and contracts, they serve as an ethical backstop. Other authors also point out that fiduciary principles should be seen primarily as a meaningful response to contractual or regulatory incompleteness (Brooks, Reference Brooks2015).Footnote 7 As such, fiduciary models can have a significant added value when used to fill inevitable gaps that are also intrinsic to contracts and laws but can be detrimental when serving as a legitimation not to fill those gaps, while this may well be the effect in practice.
Fourth, it may be counterintuitive to adopt trust-based models when it is known that citizens do not actually trust data companies. Recent survey research indicates that trust in the tech industry has declined, while it grew or remained steady in other sectors (Edelman Trust Barometer, 2020). Considering the regular scandals (Cambridge Analytica, fake news, data leaks, etc.) that make it to the headlines, data subjects do not have “positive expectations” of these companies.Footnote 8 Although there is “vulnerability” and “reliance,” and companies could have the means to take the interests of end users at heart, these companies are not motivated by actual trust vested in them.
Fifth, there is a mismatch between the level of interaction which is connected to the traditional fiduciary model and the proposed fiduciary model for internet companies. Whereas traditional fiduciaries are generally persons (a person’s lawyer, doctor, or accountant) or organizations with facework commitments with whom trustees have a direct and sometimes even interpersonal interaction, this sort of interaction is absent with information fiduciaries such as Facebook or Google. This entails that trust cues which normally help people to invest trust wisely, are missing. For instance, testimonial evidence such as promises made by a trustee or statements on past accomplishments are absent.
Finally, tech companies face the problem of conflicting interests; it is hard to encapsulate the interests of data subjects as they do not necessarily align with the company’s interest. Indeed, tech companies do not only have a duty of loyalty vis-a-vis their customers, but also vis-à-vis their shareholders. The latter expect their return on investment. Consequently, there is a strong push for companies to monetize personal data, even if this might harm data subjects. Whereas in traditional fiduciary relations (lawyers, doctors) information is processed to reach a goal that is predominantly of importance to the trustor (a convincing defense in court or successful treatment), for internet companies the data processing itself is their bread and butter; their sine qua non (Khan and Pozen, Reference Khan and Pozen2019).
4. Data Curators, Data Custodians, and Data Stewards
4.1. Introduction
A second trust model is to have a data company appoint a data curator, data custodian, or data steward. The idea of having a person within an organization not only concerned with the direct interest of that organization, but also of the citizens whose personal information is processed and society at large is not new. An early but important example is the European Union’s data protection official or data protection officer, an independent employee or consultant, that must ensure that all rules contained in the Data Protection Directive 1995 and the subsequent General Data Protection Regulation 2016 are respected.Footnote 9 This person is paid by the organization processing data, but her primary role is ensuring that the data subjects’ rights are respected and the other data protection principles are adhered to, such as those concerning data security, confidentiality, and data quality. Organizations that appoint such an officer must ensure that person “does not receive any instructions regarding the exercise of those tasks. He or she shall not be dismissed or penalised” for performing her tasks.Footnote 10 Thus, such a figure resembles an occupational physician, who is paid by an organization, but still functions independently and is bound by both her hypocritic oath and professional secrecy.
4.2. Promises
The data protection officer has many advantages. It reliefs the data protection authority from part of its tasks of control and oversight, while laying the costs with the organization processing personal data; it ensures that the person executing the oversight has direct contact with and knowledge of the concrete contexts in which data processing operations occur; and it ensures that there is an independent authority that can respond to data subject request swiftly.
In Common Law jurisdictions, similar models have been proposed and implemented; such persons have been coined data curators, data custodians, or data stewards (Mon, Reference Mon2007; Choudhury, Reference Choudhury2008; Witt, Reference Witt2008; Walters, Reference Walters2009). Although there are small differences between data curators, data custodians, and data stewards, their perceived tasks are largely similar (Delserone, Reference Delserone2008). Because their role is bigger than that of a typical data protection officer, these figures are also increasingly appointed within the European context. They not only have the obligation to ensure that the data subjects’ interests are safeguarded, but also to see to it that general interests are furthered.Footnote 11
One of the main tasks of data curators, custodians, and stewards is ensuring that the quality of the data process is exemplary (Yakel, Reference Yakel2007; Yakel et al., Reference Yakel, Conway, Hedstrom and Wallace2011). This is an independent value, applicable not only to personal data, but also to meta-data and aggregated data. When gathering data, metadata has to be retained, data have to be categorized transparently (so-called end-to-end data stewardship) (Knapp, Reference Knapp2008) and data have to be archived in a way that the data can be used by future generations (Downs et al., Reference Downs, Duerr, Hills and Ramapriyan2015). As such, this obligation goes beyond serving the interests of the data subject. The underlying idea is that data are a public good, so that they must not only be stored and prepared in a way that is useful for the organizations that have the data, but in a way that they may be used by other organizations.
The Committee on Science, Engineering, and Public defines data stewardship as “the long-term preservation of data so as to ensure their continued value, sometimes for unanticipated uses” and notes that stewardship “embodies a conception of research in which data are both an end product of research and a vital component of the research infrastructure” (Rolando et al., Reference Rolando, Doty, Hagenmaier, Valk and Parham2013). Doing so, organizations ensure that other organizations can also use the data and that future generations can assess and evaluate the data that have been used, for example, for scientific research (Knapp et al., Reference Knapp, Bates and Barkstrom2007). Good data curatorship may entail publishing the data and may involve “efforts to make the data accessible, including the provision of data documents, the linking of data to a digital literature library, the preparation of ready-to-use model data sets and reanalysis data sets, and the provision of a number of Web services” (Li et al., Reference Li, Nan, Cheng, Ding, Wu, Wang, Wang, Ran, Li, Pan and Zhu2011).
Data curators, custodians, or stewards have initially been promoted by governmental agencies that award research funding (Wilkinson et al., Reference Wilkinson, Dumontier, Aalbersberg, Appleton, Axton, Baak, Blomberg, Boiten, da Silva Santos, Bourne, Bouwman, Brookes, Clark, Crosas, Dillo, Dumon, Edmunds, Evelo, Finkers, Gonzalez-Beltran, Gray, Groth, Goble, Grethe, Heringa, ’t Hoen, Hooft, Kuhn, Kok, Kok, Lusher, Martone, Mons, Packer, Persson, Rocca-Serra, Roos, van Schaik, Sansone, Schultes, Sengstag, Slater, Strawn, Swertz, Thompson, van der Lei, van Mulligen, Velterop, Waagmeester, Wittenburg, Wolstencroft, Zhao and Mons2016), and have subsequently also been appointed in public institutions, such as libraries, archives, and universities (Strasser et al., Reference Strasser, Cook, Michener, Budden and Koskela2011; Scaramozzino et al., Reference Scaramozzino, Ramírez and McGaughey2012; Steeleworthy, Reference Steeleworthy2014), and private sector organizations (Treloar et al., Reference Treloar, Groenewegen and Harboe-Ree2007; Heidorn, Reference Heidorn2011; Hartter et al., Reference Hartter, Ryan, MacKenzie, Parker and Strasser2013; Papadatos et al., Reference Papadatos, Gaulton, Hersey and Overington2015; Fourches et al., Reference Fourches, Muratov and Tropsha2016).
From a trust perspective, it might be tempting to think of the curators, custodians, and stewards as being the trustee. However, different from data protection officers, these professionals are not the actors which citizens interact with. The trustee remains the data-processing organization. Still, curators, custodians, and stewards can take on the crucial role of checking and controlling the organization, making the data-processing organization as a trustee more reliable and accountable. Trust may play an important role in the cost-benefit analysis of organizations processing personal data, as institutions might aim at developing and maintaining long-lasting relations with data subjects because that is valuable to them (e.g., data subjects as returning customers). In addition, there is an incentive for maintaining a good reputation (Hardin, Reference Hardin2006). By encapsulating the interests of data subjects, data curators, custodians, and stewards can contribute to fostering trust to the public at large and the data subjects whose data are processed in the institutions that use their data (Lord et al., Reference Lord, Macdonald, Lyon and Giaretta2004). Besides the trust relationship between the citizen and the organization, there is, especially for governmental organizations or private organizations that obtain public funding, the trust relationship between the government (and by extension the general public) and the organization. The data curator model plays an important role in this trust relationship as well.
4.3. Challenges
First, data curators, custodians, and stewards are not merely serving the interests of data subjects, but have to safeguard additional interests, such as the general interest and the interests of the organization that appointed them. There are clear tensions between these. For example, reuse of data may be in the public interest, but not in that of the data subject and the organization in question. Or, some data subjects may want their data reused, while others may not, or one funding organization may have another idea of what data management in the public interest entails than another. This means that the type of safeguards that are provided and the type of interests that are furthered in practice depends highly on the data curator, custodian, or stewards in question.
A second challenge is the limited focus of their responsibility (Rosenbaum, Reference Rosenbaum2010). Data curators, custodians, and stewards particularly aim their attention at the quality and governance of the data processing (Larson et al., Reference Larson, Magnus, Lungren, Shah and Langlotz2020). They ensure that the data repository is well-kept (Dankar and El Emam, Reference Dankar and El Emam2012), so that at least the data that are in them are correct (http://www.dcc.ac.uk/digital-curation/what-digital-curation) and that the analysis that is performed on that data is sound (Baker, Reference Baker2009) and trustworthy (McLure et al., Reference McLure, Level, Cranston, Oehlerts and Culbertson2014). While it might be true that “[b]y improving these quality dimensions, data curation can increase the credibility and trust of data that passes through the curation process” (Curry et al., Reference Curry, Freitas and O’Riáin2010), these interventions do not take care of all the vulnerabilities that citizens face when their data is being processed. For instance, these professionals do not necessarily ensure that citizens are provided with a meaningful explanation when they want to know what happens with their data, nor do they make sure that the collection of data takes place in a lawful way (Abrams et al., Reference Abrams, Abrams, Cullen and Goldstein2019). Thus they cannot replace a figure such as the data protection officer (Karasti et al., Reference Karasti, Baker and Halkola2006), which might mean that conflicts arise between data protection officers and data curators, custodians, or stewards.
A third challenge is the apparent gap between the responsibilities of the data curators, custodians, and stewards, and their competences. On the one hand, these persons operate on a number of highly complex and interdependent domains, requiring, inter alia, technical expertise, an understanding of data management and curation, legal expertise, the organization’s core operations, and interpersonal skills (Kouper, Reference Kouper2013). It is clear that it is difficult to combine all these aspects in one person, while courses and trainings for data curators, custodians, and stewards often only focus on one or two of these aspects (Wildgaard et al., Reference Wildgaard, Vlachos, Nondal, Larsen and Svendsen2020). In addition, it is clear that these persons often lack the required competences and clearances to perform their duties as well as the required budget, staff, and time (Tammaro et al., Reference Tammaro, Ross and Casarosa2014).
The multifaceted work of the data curators, custodians, and stewards ties into a fourth obstacle, and that is the lack of a clear definition and understanding of the precise role of these figures within an organization. This is, in general, the advantage of trust-based regulatory models, but at the same time their disadvantage. Because of the lack of fixed definitions or delineations of the role of these figures, organizations can tailor their tasks to what is required in their specific context (Tammaro and Casarosa, Reference Tammaro and Casarosa2018). Yet, this means that the added value of the data curators, custodians, and stewards is yet again depended on the benevolence of the organization processing the data. This means that also appointing such a figure within an organization may give the aura of trustworthiness, while in reality, the position of these figures may be limited. In addition, this means the exact tasks and responsibilities of these figures will vary significantly per organizations and context (Tammaro et al., Reference Tammaro, Matusiak, Sposito and Casarosa2019).
A final challenge is that there are no standards or common targets for data curators, custodians, and stewards. This means that when they, for example, want to ensure that data is reusable and interoperable, there is no standard format. This is complicated by the fact that many organizations, both in the public and the private sector, duplicate each other’s datasets. Data stewards themselves see this as one of the primary obstacles in their functioning: “As one respondent stated, data is scattered all across the government. Whereas the national registers (e.g., with citizen data, company data, income data, building data, and so on) were meant to be singular data sources, many organizations still download a duplicate of the entire data set every day in order to work with the data. [] The forms of data proliferation can be seen in the silo’s which have grown within the government. ‘I see silos everywhere, when talking about data sharing. There is no common infrastructure. Within the government, there is a system of registration, which on its own is a nice system. Only, how do you connect with other systems? Then again you end up with silos.’” (Van Donge et al., Reference Van Donge, Bharosa and Janssen2020).
5. Data Trusts
5.1. Introduction
The philosophy behind data trusts presents a third model of ethical data management that combines elements of the two previous models, with important variations (Ciuriak, Reference Ciuriak2019). A trust is an institution specifically designed and set up to govern data on behalf of those that have shared them (Hall and Pesenti, Reference Hall and Pesenti2017). A data trust has a clear set of aims. First, it is not based on a model of rights and claims, but on compromises and shared interests. Second, it leaves the model of well-intended but often useless transparency; instead, a data subject is assisted by the professional or expert running the trust. Third, data trusts could do ex ante auditing of requests and ex post monitoring of data usages. Fourth, data trusts would have to account for their choices and their consequences to the data subjects. Finally, a data trust would assess whether data processes accord with the prevailing legal standards (O’hara, Reference O’hara2019).
There are two primary examples where trusts have been proposed and used.
First is the medical domain, where medical patient data are used and reused for scientific research. Here, a trust can help in distributing the data to the right research team and determine preconditions for them to access the data. Having the data in trusts also helps establish trust with patients, who might otherwise be hesitant to share their data for research purposes. An early example is the UK biobank. “UK Biobank is a national and international health resource with unparalleled research opportunities, open to all bona fide health researchers. UK Biobank aims to improve the prevention, diagnosis, and treatment of a wide range of serious and life-threatening illnesses—including cancer, heart diseases, stroke, diabetes, arthritis, osteoporosis, eye disorders, depression, and forms of dementia. It is following the health and well-being of 500,000 volunteer participants and provides health information, which does not identify them, to approved researchers in the UK and overseas, from academia and industry. Scientists, please ensure you read the background materials before registering. To our participants, we say thank you for supporting this important resource to improve health. Without you, none of the research featured on this website would be possible” (Biobank Homepage, 2022).
A second prominent example where trusts are used is in smart cities. Here, the idea is that data are gathered in the public domain about public behavior and that that data should remain public and be used only in the public interest. Among others, it was introduced by Google when it faced privacy criticism for one of its smart city projects, the Sidewalk Labs project in Toronto, Canada. It then proposed alternatives to its initial plan with four key components, namely responsible data, open standards, responsible data impact assessment, and a civic data trust. The trust is defined as a “model for stewardship and management of data and digital infrastructure that approves and controls the collection and use of data for the benefit of society and individuals” (https://waterfrontoronto.ca/nbe/wcm/connect/waterfront/41979265-8044-442a-9351-e28ef6c76d70/18.10.15_SWT_Draft+Proposals+Regarding+Data+Use+and+Governance.pdf?MOD=AJPERE).
5.2. Promises
The main benefit of having an independent organization govern the data is that the data are never in the hands of the private parties, such as Amazon, Google, Facebook, or Microsoft, in the first place. Rather, the data are placed in the hands of a trust and it is for that trust to make decisions on who can access the data and for what purposes. The trust itself is governed by a person, group, or institution that is neutral and has the public interest at heart (Hardinges, Reference Hardinges2018)—a data trust is consequently not aimed at promoting the interests of the private parties that wish to get access to the data (Alsaad et al., Reference Alsaad, O’Hara and Carr2019).
The problem underlying the conceptualization of data trusts is both that public data are currently privatized and thus not optimally used and that there are privacy and intellectual property rights that block the sharing of data to multiple parties. In addition, the data subject is believed to favor her data being used for “the good,” while being hesitant to share data because she distrusts large information intermediaries. The advantage of a data trust is that the data are not privatized by one party and also not open in the public domain, like open data, that can be used and abused by any party with an internet connection (Stalla-Bourdillon et al., Reference Stalla-Bourdillon, Thuermer, Walker, Carmichael and Simperl2020).
In addition, data trusts could be used to provide a tailor-made approach, either because there are multiple trusts which data subjects can choose from, opting for the trust that best fits her own privacy preferences, or because citizens within a trust can still indicate their privacy preferences and can opt-in or opt-out to certain data uses and/or parties having access to their data (Thuermer et al., Reference Thuermer, Walker and Simperl2019). Trusts can negotiate the terms under which parties may access data, relieving individuals from having to discuss (or rather agree to) terms with each party individually (Ruhaak, Reference Ruhaak2019). Data trusts also allow for chained and traceable data provenance. “Transportation data from any number of sources are used to develop models, but encoding the data as trained weights in a model ends up ‘laundering’ it—that is, it is no longer transparent to trace the source of the data through to the decisions reached by the model. A trust mitigates the lack of algorithmic accountability in part by emphasizing the use of synthetic datasets whenever possible during research and development; once a proof of concept is established, and access to the raw data is requested, ongoing data-sharing relationships mediated through data-sharing agreements present an opportunity to enforce provenance” (Young et al., Reference Young, Rodriguez, Keller, Sun, Sa, Whittington and Howe2019).
The concept of data trusts provides an alternative to the idea of information fiduciaries and is presented as an improvement of that model. For example, while Balkin acknowledges the potential for conflict of interest by parties that want to maximize growth and profit and at the same time need to respect certain fiduciary principles, “he fails to draw the only logical conclusion: a fiduciary obligation toward data subjects is incompatible with the data controllers” responsibility toward shareholders (Delacroix and Lawrence, Reference Delacroix and Lawrence2019).Footnote 12
As a final advantage, a data trust model can be best understood as a form of third-party trust (Lau et al., Reference Lau, Penner and Wong2020). A, the data subject, does not necessarily trust the internet company (let us say C), but does trusts B, the data trust, to interact with C. The data trust (B) therefore bridges the trust gap between A and C. In contrast to the two other trust-based regulation models, the data trust does not suffer—or at least to a much lesser degree—from conflicting interests. They first and foremost represent the data subjects and their wishes.
5.3. Challenges
As with all trust-based strategies, vulnerability and risk remain. For instance, even with clear agreements underlying the functioning of the data trust, the data-sharing with the interested data-processing parties can still go wrong. For instance, even with data trusts’ ex ante checks and ex post monitoring, third parties may not live up to the agreement and use data for other goals than decided upon. Also, unintended consequences—unforeseeable discrimination, faulty decision-making based on the data—may harm the data subjects. In addition, by bringing together data in a data trust, it becomes much more attractive for cybercriminals to try to hack and steal these data (Mills, Reference Mills2019).
A second challenge that the data trust model might face is the problem of scalability and granularity. The strength of a data trust lies in pooling the interests of a specific subset of data subjects (citizens of a smart city, people suffering from a specific illness). However, this bringing together of data subjects is heavily context-dependent. It is not clear if and how a data trust can function in supra-context situations, where the data subjects are more heterogeneous and the use cases do not necessarily align. Data trusts can impossibly negotiate individual terms per trustor. For a data trust to effectively govern the entrusted data, it will have to define group profiles reflecting the interests of the data subjects. To make this manageable, compromises will need to be made and data subjects might not see all their interests and wishes being reflected in the categorizations made by the data trust.
Third, as with all trust-based regulatory strategies, the question remains: how to ensure that trust is not completely blind? Or in other words, how can data subjects assess the trustworthiness of the data trusts? Here again, some control-based strategies might come to the rescue. Some of the uncertainty will be reduced by the contracts underpinning the relation of data subjects and data trusts. Also, the possibility to be actively involved in the operation of the data trust can be a way to reduce uncertainty. Finally, one can also think of certification schemes for data trusts. This would entail another trusted, independent party checking if the data trust is bona fide (Reed and Ng, Reference Reed and Ng2019).
Fourth, more so than the other trust-based strategies discussed in this article, data trusts may operate in a way that they allow for more data to be processed than is currently the case, rather than less. This means that data companies as well as (governmental) organizations have a clear interest in these types of trusts. They may want to try to have paws in the board of these trusts or through spending on marketing, promote certain data trusts. This means, for example, that there might be a risk that board members have a double or secret agenda, as was feared when Google proposed to set up a data trust when the Sidewalks project was in danger. Would Google have influence over who served on the board and if so, what added benefit would a trust then have? (Toronto Star, 2019).
Fifth and finally, the question is whether there should be legal limits to what data trusts can and cannot do with the data of the trustors and within which framework they should operate. Can data trusts ask money for sharing data with data-driven organizations and if so, should that money flow directly to the trustors or can it also be for making profit? Can data trusts themselves participate in data-driven processes or even initiate them? What legal boundaries are there to the purposes the data received from trustors can be put to use for? What are the transparency obligations for data trusts; should they make annual reports public, should they only inform trustors or can they wait until they receive specific requests? Such legal frameworks are lacking so far.
6. A Regulatory Model for Data Trusts
Regulators around the world are struggling with emerging technologies, disruptive data-driven applications, and the power of large information intermediaries. Most countries use a combination of two regulatory models: informed consent and governmental scrutiny. Both have their specific merits, both have their specific limits, but what they share is that many feel they have been unable to satisfactorily regulate and limit large data-driven organizations. That is why a third model has been suggested, that is not based either on the right to control by the data subject or the desire to assert control over the information market by governmental authorities, but on trust. This article has shown that every relationship has trust characteristics and that organizations can play an important role in ensuring trust in an increasingly complex environment (Section 2). Subsequently, this article scrutinized three specific models of trust-based relationships that have been proposed in the literature and implemented in practice: that of information fiduciaries (Section 3), data curators/stewards/custodians (Section 4), and data trusts (Section 5). Each of those has their specific merits and their potential dangers.
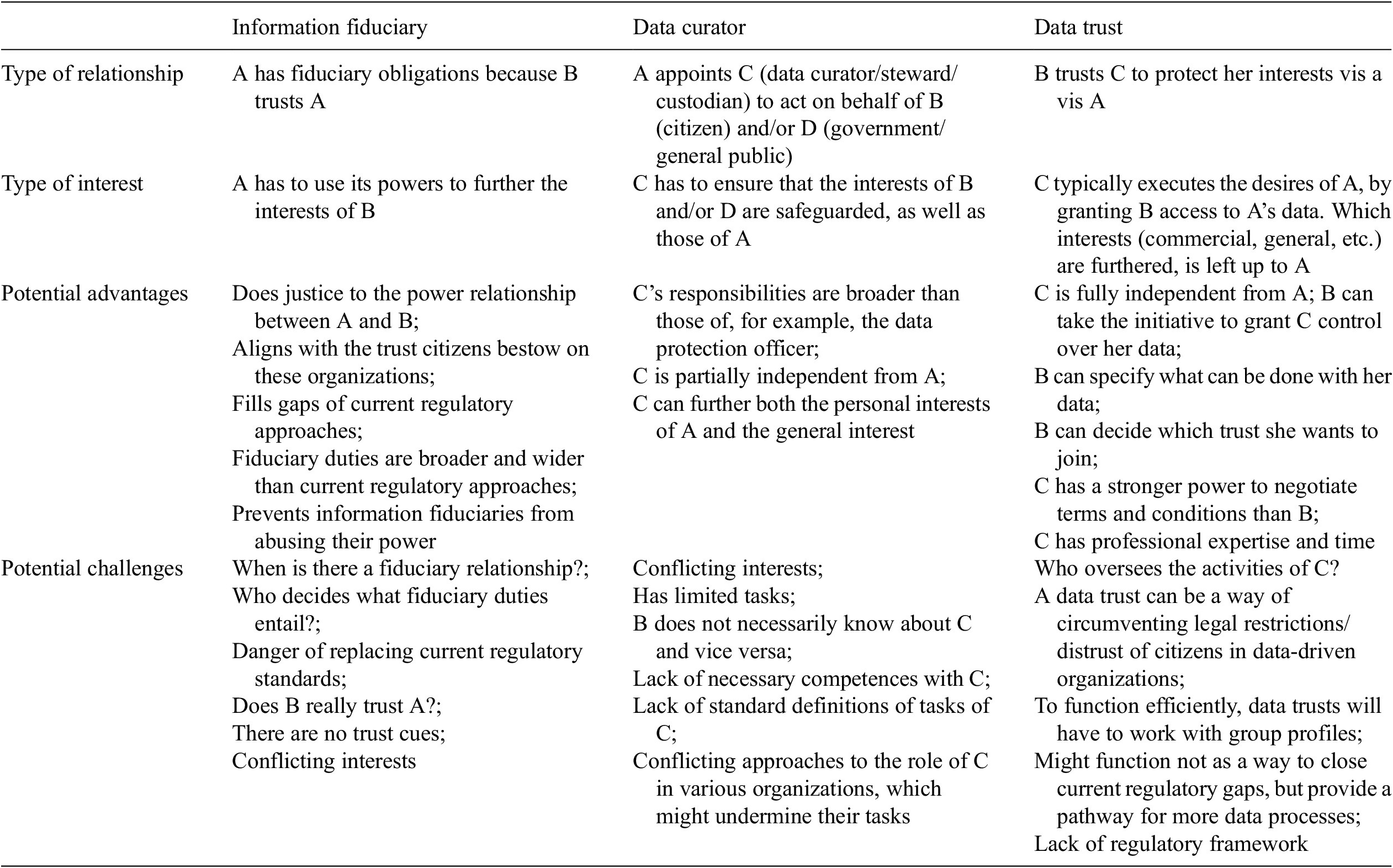
It is clear that of the three, the last one is the most far-reaching and the one that can have a big impact on the data-driven environment. While the first two models ensure that the current regulatory regimes are complemented with additional duties of care, either born by the information fiduciary or a data custodian, curator, or steward appointed by the organizations, the third model proposes to set up an entirely new institution. While the first two models set out to fill the gaps in the current regulatory model, the third one sets up an entirely different approach and while the first two models aim at further restricting or setting additional frameworks for data processing, the third one opens ways for processing more data. And while the first two fit in standard approaches in law, such as duties of care or the rules that apply to an occupational physician, such are absent with data trusts. Because data trusts can have a huge impact on the data-driven environment, a standard framework should be set up.
Currently, there seems to be no direct prohibition for anyone to set up a data trust, even in the world’s strictest data protection regime: the GDPR. If a person or institution contacts data subjects or advertises its services, it will obtain consent from the data subject if she agrees with the terms and services of the data trust, which will presumably be laid down in a contractual agreement. Thus, there would be a processing ground for processing both “ordinary”Footnote 13 and “special” or “sensitive” personal data.Footnote 14 It might be argued that giving broad consent for using personal data for various kinds of purposes, where the data subject only specifies the general type of data processes that its data can be used for or the parties that can access its data will not be in conformity of the GDPR. Although ever broader forms of consent are deemed legally permissible (Hallinan, Reference Hallinan2020), this potential obstacle can be remedied by asking concrete consent every time a party wants to gain access to a data subject’s data, while she does not receive the access requests by parties or projects that fall outside her pre-set preferences.
By staying in direct contact with the data subject, the data trust would also adhere to the transparency requirement. When assessing parties and data processing initiatives that want to have access to the data in the data trust on behalf of the data subjects that joined the trust, it would have to do a general test of legitimacy, reliability, and accountability. Thus, it would have to ask detailed questions about the entity wanting to gain access to the data, the purposes for which it wants to gain access and other relevant information, which could be passed onto potentially interested data subjects.Footnote 15
By having data subjects give consent to each individual entity or for each individual processing operation, there would be no problem with respect to the prohibition of reuse of personal data.Footnote 16 The data trust would process, primarily meaning storing and managing the data, on the basis of consent and/or contract; the purpose of managing personal data on behalf of trustors would be deemed legitimate and fair.Footnote 17 And the data subject would provide a new processing ground for a new processing operation by a third party wanting to gain access to her data if she would give her consent.Footnote 18
Obviously, the parties wanting to gain access to the data as well as the trust would need to adhere to the formal, technical, and organizational requirements contained in the GDPR, such as having a data protection officer,Footnote 19 doing a data protection impact assessment for risk-intense data operations,Footnote 20 setting up an internal data protection policy,Footnote 21 having a register with all data processing operations and their details,Footnote 22 and implementing technical and organizational measurements to ensure data protection by design and/or default.Footnote 23 In addition, there would have to be adequate technical and organizational standards to ensure the safety and confidentiality of the data.Footnote 24 With respect to the latter requirement, as well as the storage limitation principle,Footnote 25 the question will be how the data trust will be organized. Will third parties gain a copy of the data, will such a copy be under a license which will automatically expire after a certain date or will the data trust maintain the sole copy of the data, only giving third parties temporary access to the data?
Another question is whether third parties will gain access to the data on a personal level or that they will only be allowed to have access to aggregated data. For most profiling and research purposes, aggregated data will be sufficient. Thus, data trust would deliver or give access to aggregated data according to the specific needs of the third party wanting to have access to the data. Though there are questions as to what extent aggregated data should be considered as nonpersonal data (Fluitt et al., Reference Fluitt, Cohen, Altman, Nissim, Viljoen and Wood2019), it seems clear that at some point, aggregated data will fall outside the scope of the GDPR. This might be seen as a prudent approach, as it ensures that third parties do not have access to direct or indirect identifiable information, but the pitfall is that trustors can no longer rely on their data subject rights.
This means that by and large, even the strictest data protection regime in the world does not pose any substantial barriers to the data trust model. Given the huge impact data trusts can have on the data-driven environment and data subject rights, a regulatory framework might be warranted. Setting out such a framework falls outside the scope of this article, but it is possible to highlight a number of important questions and situations that such a framework would need to tackle. The basic question to be answered first is, do we want data trusts? The answer may well be no, because it may prove to be Pandora’s data processing box and because data companies might seize the opportunity to set up new ways of gathering even more data than they already have. If the answer is, in principle, yes, then a legal framework should at least address the following elements:
1. Internal structure:
a. Initiator: can anyone initiate and found a data trust, including, for example, Google or Facebook? Can foreign actors set up data trusts (e.g., a Russian company setting up a trust where data subjects can submit their political preferences and other relevant information for elections?)
b. Board: can anyone be on the board of the data trust or should there be rules on conflict of interest or competence? Should there be rules on the maximum salary for board members?
c. Transparency: should there be transparency about which types of third parties want to have access and why and what are the outcomes of the selection process?
2. Data management:
a. Access: rules on whether third parties may copy, license or only have temporary access to the data stored at the data trust and if the first, how would the trust ensure that the data protection principles are subsequently respected by the third party?
b. Aggregation: rules on whether third parties gain access to the data in individual or in aggregated form and if the latter, what level of aggregation (which may depend on the sensitivity of the data processing operations of the third party).
c. Data quality: how does the data trust ensure that the data provided by the data subjects and processed by third parties is correct?
3. Activities:
a. Prohibitions: should there be prohibitions on types of actors or types of activities for which data trusts may grant access to the data?
b. Public interests: should there be a rule that data trusts can only grant access to data when third parties aim at furthering public interests and if so, how should those public interests be delineated?
c. Revenue: can the trust ask money in return for access and if so, can a trust make revenue and/or should the revenue flow directly to the data subjects? Or can data trusts pay data subjects a lump sum for their data when they join the trust?
4. Data subjects’ rights:
a. Data: should there be types of data that are excluded from these data trusts (e.g., medical information) or that are reserved for special trusts?
b. Information: how is it ensured that data subjects can obtain relevant information about data processing operations executed by third parties?
c. Influence: should a data subject representative be part of the committee deciding on the admissibility of third-party requests?
Funding Statement
No funding was received for this work.
Competing Interests
The authors declare no competing interests exist.
Author Contributions
Both authors did the research for this article and have co-written the article.
Data Availability Statement
This study is based on a literature review. The list of sources used is provided in the bibliography below. These sources are publicly available.
Comments
No Comments have been published for this article.