From 2004 to 2014, the International Federation of Red Cross and Red Crescent Societies estimated that, on average, more than 12 disasters occurred every week around the globe.Reference Hamza1 Some of these disasters had catastrophic consequences. In the United States, Hurricanes Katrina, Harvey, and Maria collectively killed at least 4500 people and caused $376 billion dollars in damages.Reference Brown, Beven and Franklin2-5 Unfortunately, these catastrophic events are becoming more common due to a convergence of anthropomorphic and climatological factors. Climate change is driving an increase in the frequency and severity of natural disasters, whether measured by number of events or economic damage,Reference Otto, Philip and Kew6-Reference Coronese, Lamperti and Keller8 while the recent rise in intra-state warfare and the interconnectedness of the global supply chain have increased vulnerability to man-made disasters.Reference Szayna, Watts and O’Mahony9,Reference Umar, Wilson and Heyl10 In addition, a global movement toward urbanization increases the potential impact of disasters as more individuals become exposed to the same hazards. Currently, 55% of the world’s population lives in urban areas, and that number is expected to rise to 68% by 2050.11 Already, 60% of cities with 500,000+ citizens are at a marked risk of a natural disaster,12 and urban settlements in low- and middle-income countries in Asia, South America, and Africa, the regions with the highest projected rates of urbanization, are located in areas with uniquely high risk to natural disasters.Reference Balk, Montgomery and McGranahan13 These heightened risks necessitate novel approaches to decrease morbidity and mortality driven by disasters.
The United States National Response Framework (NRF) defines the disaster lifecycle as comprising prevention, protection, mitigation, response, and recovery.14 Prevention, protection, and mitigation all take place before a disaster’s occurrence, while recovery occurs after the acute response has subsided. Response begins the moment a disaster affects an area. Disasters are fast moving, highly dynamic events, and the response can involve a wide range of actors, including state and local authorities, federal and international agencies, and the populations affected. Response is limited by time, capital, and human resources, which drive the need to quickly and efficiently mobilize limited supplies and personnel. Effective mobilization, particularly between agencies with disparate priorities and objectives, requires emergency managers to have a complete understanding of the situation in the field. This knowledge framework is frequently referred to as situational awareness (SA). In a disaster response, Emergency Operations Centers (EOCs) act as information hubs and are responsible for the attainment and sharing of SA vertically and horizontally across decision-makers and actors in the field.
SA is particularly challenging to obtain during a disaster due to logistical challenges in collecting and disseminating complete and high-quality information from first responders to EOCs, the constantly shifting needs and resources in the field, and organizations using different information sharing platforms. With incomplete SA, the decisions made within EOCs will be inefficient and potentially ineffective, as they target a situation different to the one at hand. The 2004 Indian Ocean Tsunami, which resulted in the death of over 227,000 people, was a somber illustration of the consequences of failing to achieve SA. The response was plagued by poor SA from the moment the tsunami was triggered, including the lack of a tsunami warning system, the inability of the Pacific Tsunami Warning Center to contact government officials in Indonesia, and the absence of a system to alert the public once the tsunami was identified.Reference Telford and Cosgrave15,Reference Ozer and de Longueville16 Domestically, this phenomenon was also demonstrated during the Deepwater Horizon response, when the EOC had incomplete and excessively technical data on the oil flow rate and well capacity, the locations of first responders, and the availability of resources, resulting in the inappropriate use of a well-sealing procedure that failed to halt the oil spill.17
Emerging technology, if appropriately applied, has the potential to revolutionize response operations. A prior review published by 2 authors involved in this study (J.R., J.F.) found that the use of information and communication technologies in disaster response is generally limited in geographical application, fails to identify the intended end-users, and does not address the challenges with implementing the technology in the field.Reference Freeman, Blacker and Hatt18 However, this prior study was limited by the lack of review of after-action reports (AARs). For example, the Deepwater Horizon AAR highlighted a lack of interoperability between technologies and information sharing platforms, challenges in interpreting and storing large amounts of gathered data, and time delays in processing data, resulting in unactionable information being delivered to decision-makers.17 This indicates that the technologies currently in use are not adequate to obtain SA, and may limit the speed, efficiency, and effectiveness of response efforts, but these specific challenges may not have been appreciated using the previous methodology, motivating this present study.
An inventory of existing SA technologies would allow response agencies to match their needs to available technologies. Likewise, identification of the technological capabilities that are currently unavailable would allow for strategic investment in these technologies. However, no systematic review of SA technologies relevant to disaster response was found in the academic literature. Therefore, the objectives of this review were to: identify technologies for gaining SA that are currently being applied to disaster response or are in development, classify these technologies based on their maturity level for fielding, and determine the SA needs of response agencies relative to the technologies currently available or emerging.
METHODS
The authors conducted a systematic literature review using the Preferred Reporting Items for Systematic Review and Meta-Analysis (PRISMA) Guidelines to identify technologies currently being used to enable SA during disaster response.Reference Moher, Liberati and Tetzlaff19 This review included published journal articles, conference proceedings, and AARs describing SA technologies undergoing research, development, testing, and evaluation in real or simulated disaster responses.
Searches for journal articles and conference proceedings were conducted in May 2019 in the following databases: Web of Science, Embase, CINAHL, BIOSIS, PubMed, and Scopus. Searches included the terms listed in Table 1. English language, original research, and conference reports published between the years 2000 and 2019 were included if describing technology for SA in a real or simulated disaster. To facilitate the management of the systematic review, the Covidence platform20 was used to import citations and screen titles and abstracts. Covidence uploaded citations and removed duplicate entries among the databases, permitting more efficient screening and review of reports. All initial steps, including title and abstract screenings, were independently conducted by a random selection of 2 individuals (T.K., J.R., M.O., S.O., M.R., K.R.L.), with conflicts resolved by a third individual. All steps from assessment of full texts for inclusion onward were performed by a single individual, and data were extracted using a standardized Microsoft Access database.
TABLE 1 Search Terms and Exclusion Criteria for Abstract Review

Abbreviations: AAR, after action report; SA, situational awareness.
Searches for AARs were conducted in Columbia International Affairs Online,21 Policy File Index,22 Homeland Security Digital Library,23 the Defense Technical Information Center,24 National Technical Information Service,25 Transport Research International Documentation,26 Google,27 and Global Health Database.28 AARs were screened separately from journal articles and conference proceedings using the same inclusion and exclusion criteria (Table 1).
Each full text was reviewed and the following data were extracted from each record: type (Supplemental Tables S1 and S8), purpose (Supplemental Table S2), and maturity of the technology (Supplemental Table S3); organization potentially using the technology (Supplemental Table S4); intended technology end-user (Supplemental Table S5); type of disaster in which technologies were or could be applied (Supplemental Table S6); and gaps in obtaining adequate SA during disaster response (Supplemental Tables S7 and S9). These “gaps” were defined as inadequate or absent technological capabilities, processes, systems, or knowledge during disaster response that were explicitly described in an article or AAR. For each full text record, data were entered in a “choose all that apply” manner, in which each full text record could describe multiple types of technologies at various levels of maturity that could be used by multiple types of organizations. Details on the data types, categories, and definitions are available in the Supplemental Material.
As no quantitative or qualitative data were collected in this systematic review, quality assessment metrics were not applied to assess the methods of the journal articles. The statistical significance of the data was determined using Pearson’s Chi-square test of Independence for contingency tables containing only data from articles, or Fisher’s exact test for contingency tables that included data from only AARs or AARs and articles. Results were considered significant if the P-value was <0.05.
RESULTS
Before beginning the systematic review, an initial search of the academic literature, governmental, and intergovernmental agency documents showed that there are no consensus definitions for the key terms being used in this review, namely SA, technology, and disaster. In particular, for the term “disaster,” there are a wide range of definitions in the literature and used by federal and intergovernmental agencies (Supplemental Tables S10 and S11). The Federal Emergency Management Agency’s (FEMA’s) response is governed by the Stafford Act, which contains the legal definition of a “major disaster” and determines FEMA’s ability to provide federal funding and respond to a domestic disaster.29 A notable exclusion from the Stafford Act definition is a health-related disaster, such as an infectious disease epidemic. Another definition of interest was from the United Nations Office for Disaster Risk Reduction, which defines a disaster as “a serious disruption of the functioning of a community or society involving widespread human, material, economic or environmental losses and impacts, which exceeds the ability of the affected community or society to cope with using its own resources”.30 Unlike the Stafford Act, this definition includes health-related disasters; however, it also includes exclusively economic disasters such as the 2008 financial crisis, which would not be considered a disaster by most response agencies. Ultimately, no perfect definition existed that precisely mapped to the scope of this project, so an established definition was adapted. Not all agencies’ response activities are intractably linked to their definition of a disaster; however, it is important to define the scope of focus for this review.
For the purposes of this review, the term disaster was derived from the definition in the Stafford Act,29 in which “determination of the President” was removed to accommodate disasters taking place outside of the United States, while “epidemic or outbreak” was added to accommodate health-related disasters (shown in italics in Table 2). The definition of SA was taken directly from the First Edition of the NRF.31 Technology was defined ad hoc, as definitions identified in standard dictionaries (eg, “the practical application of knowledge especially in a particular area”32) were nonspecific. The exact definitions used in the present review served as inclusion criteria (Table 2).
TABLE 2 Definition of Terms

Note: The superscripts are references included in the bibliography of the manuscript.
A total of 1459 articles and 36 AARs were identified during literature searches. Following removal of 284 duplicate records, exclusion of 667 records during abstract screening, and exclusion of 242 records during full-text review, 302 records17,Reference Abdullah33–Reference Fang, Xu and Pei333 were included for data extraction (Figure 1). The most common reason for exclusion of a record during full-text review was due to it being a review study or opinion/editorial.

FIGURE 1 Inclusion and Exclusion of Reports During Screening and Full-Text Review.
The included articles and AARs skewed to recent years (Supplemental Figure S1), with 79% of the articles and 90% of the AARs being published in the last decade. The majority of technologies described in articles were related to responses to natural disasters (238 of 282 included articles, 84%) (Figure 2). The natural disasters to which the greatest proportions of technologies were applicable were hydrological (153 articles) and geological disasters (142 articles). In addition, a large number of technologies from the articles could be applied to accidental or deliberate disasters (163 articles). These categories were not exclusive, and technologies were sorted into 1 or more categories, depending on the use case described by the article’s authors, or use cases envisioned by the systematic review team.
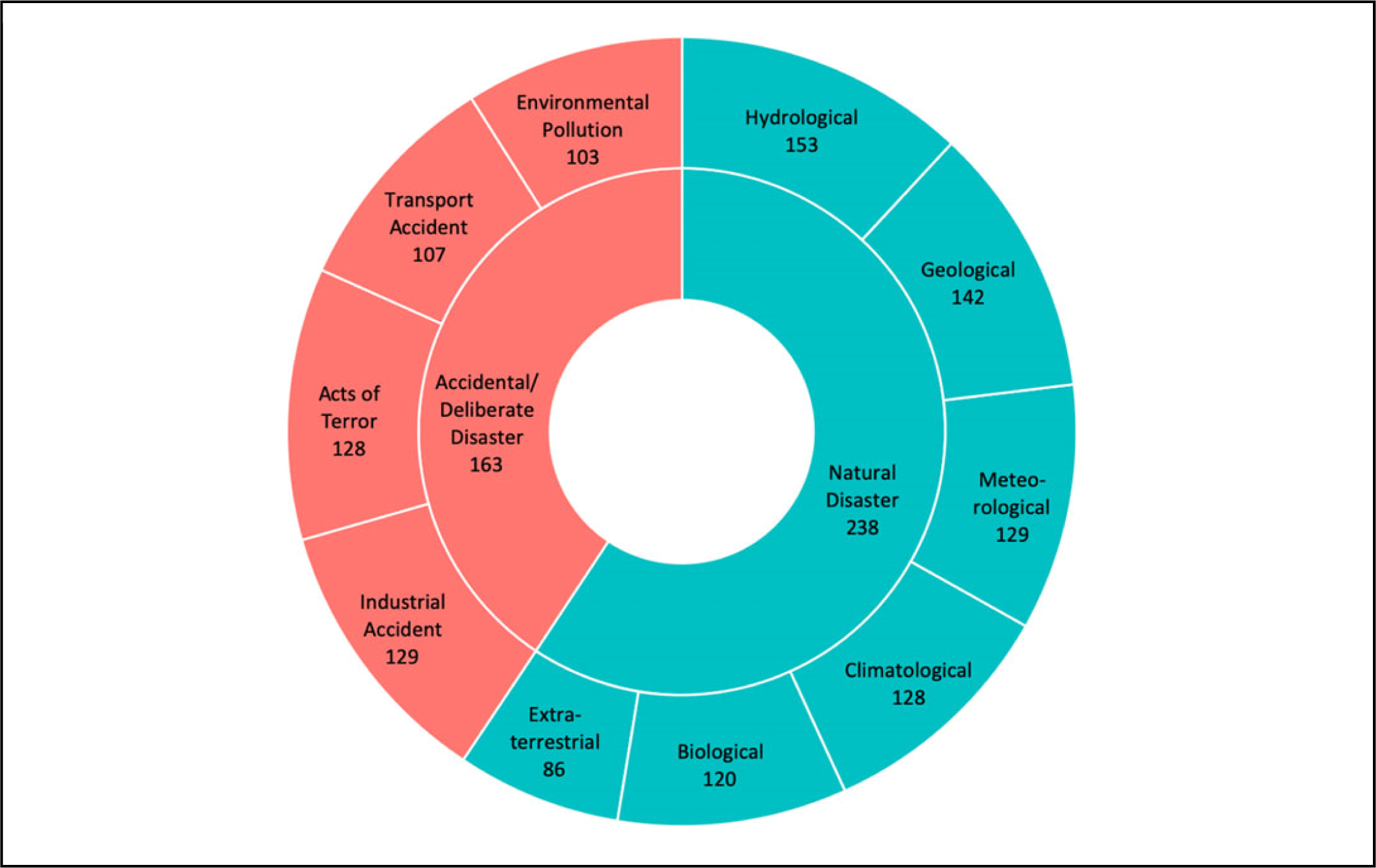
FIGURE 2 Disaster Type to Which Technology Was Applied (Number of Articles Only, n = 282 Articles).
Technologies were categorized based on the technology type and purpose. Technology types varied, with the most common types in articles being data analysis (52%) and sensor technologies (42%), while the most common types in AARs were communications (90%) and user interface technologies (55%) (Figure 3). Communications and user interface technologies were significantly more likely to be mentioned in AARs, while data analysis technologies were significantly more likely to be mentioned in articles. In addition, technologies that performed data aggregation and data generation or collection were significantly more likely to be mentioned in AARs, and technologies that performed data interpretation were significantly more likely to be mentioned in articles (Supplemental Figure S2).

FIGURE 3 Technology and Gap Categories Mapped by Record Type (n = 282 Articles, 20 AARs).
Most technologies were not associated with certain disaster types, with the exception of robotics technology. Robotics technology was significantly more likely to be mentioned in relation to accidental or deliberate disasters in articles (Figure 4), such as unmanned vehicles that could remotely sense radiation in a nuclear disasterReference Towler, Krawiec and Kochersberger216 or identify an oil spill in the ocean.Reference Kroutil, Shen and Lewis120 There was no clear trend in the technology types mentioned over time.
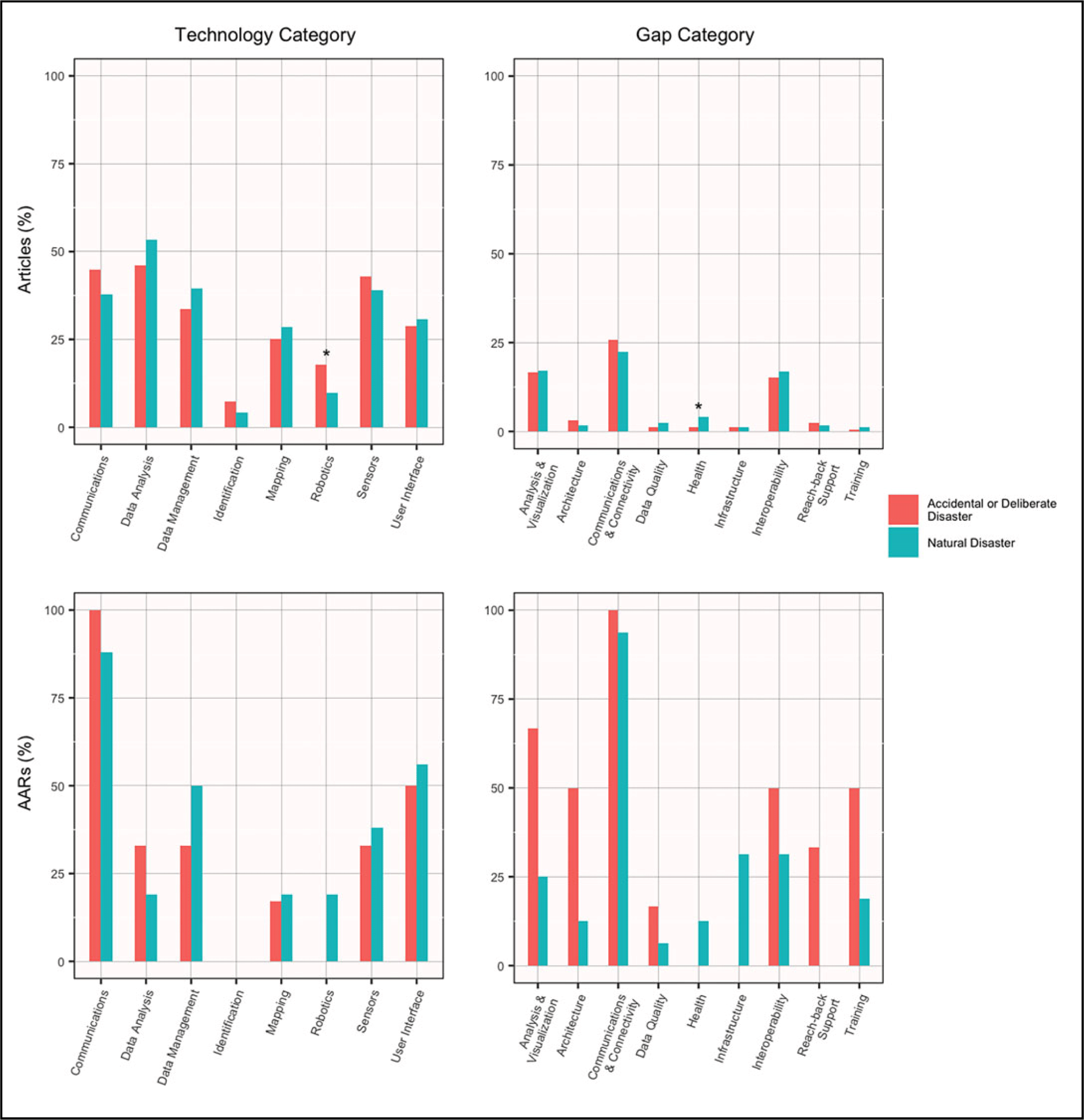
FIGURE 4 Technology and Gap Categories Mapped by Disaster Type (n =282 Articles, 20 AARs).
Technologies were also categorized by their maturity, their intended end-user within a disaster response agency, and by the organizations likely to use the technology, none of which were mutually exclusive. End users and organizations included those explicitly reported in the article, as well as those who could potentially leverage the technology. The majority of technologies were intended for use by EOC staff (84% in articles and 90% in AARs). In addition, technologies intended for use by first responders were significantly more likely to be mentioned in AARs (Supplemental Figure S3). The most common organization in which technologies could be applied was the Department of Homeland Security (DHS) (72%) (Figure S4). The majority of technologies in articles were still immature, being at the pilot/proof of concept stage or earlier (79%) (Supplemental Figure S5). The early technology maturity level was similar across all technology categories (Figure 5).
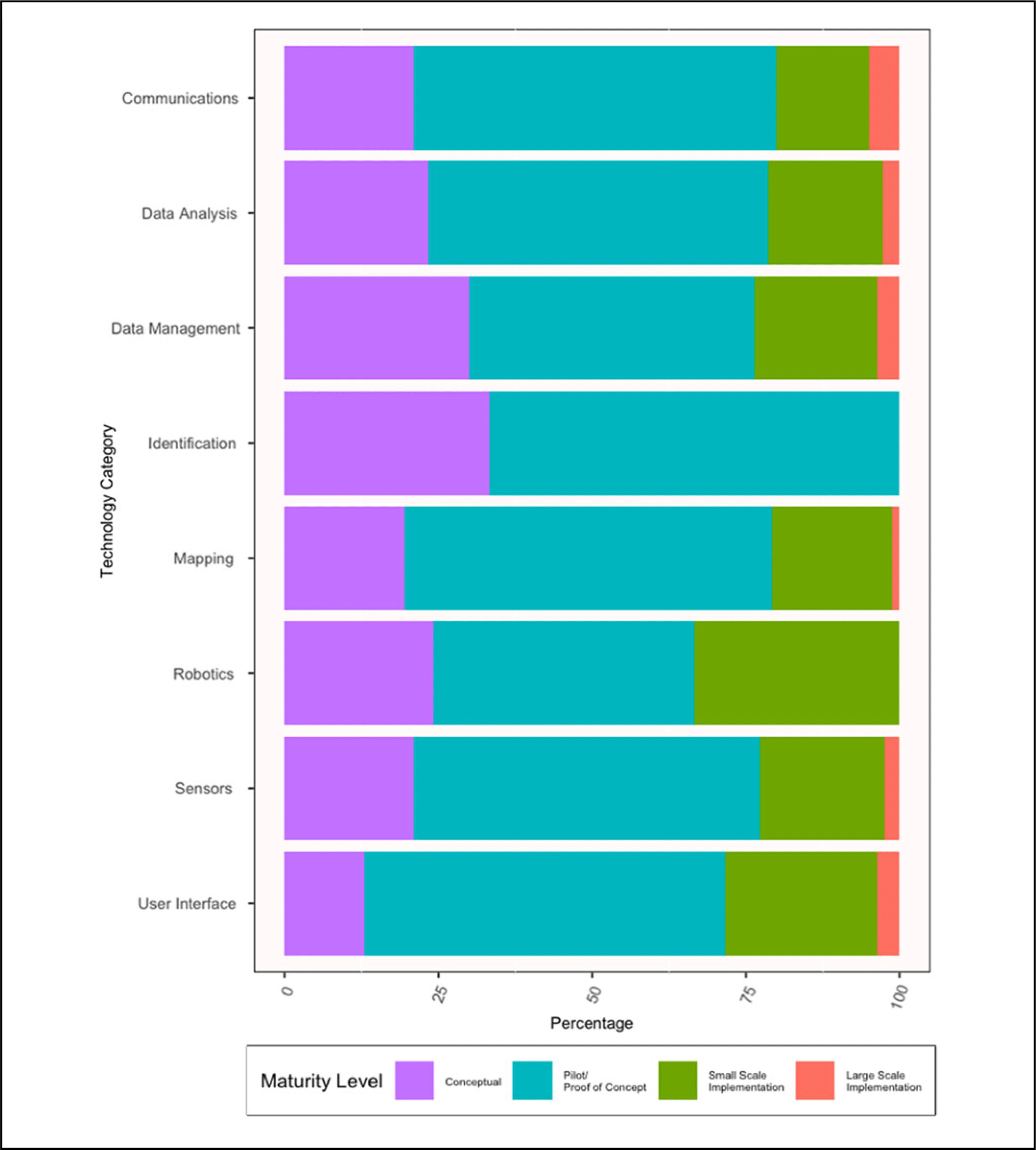
FIGURE 5 Technology Categories Versus Technology Maturity Levels (Articles Only, n = 282 Articles).
Gaps explicitly mentioned by the authors that might limit the use of a technology were mapped to 10 categories. These gap categories were composed of numerous individual gaps (Supplemental Table S9). The 3 most common gap categories were inadequacies in communications and connectivity (95% of AARs, 21% of articles), analysis and visualization (35% of AARs, 17% of articles), and interoperability and sensor capabilities (35% of AARs, 16% of articles) (Figure 3). Gaps in architecture, communications and connectivity, infrastructure, and training were significantly more likely to be mentioned in AARs.
There was no clear trend in the gap categories over time. Most gaps were not clearly associated with certain types of disasters, with the exception of health gaps, which were significantly more likely to be mentioned in relation to natural disasters in articles (Figure 4).
DISCUSSION
This systematic review defined disasters similarly to the Stafford Act, with the addition of health-related disasters. For the purposes of AARs and potential applicability of these findings, disasters located anywhere in the world were included. The included records skewed to more recent years, likely due to the increasing volume of publications over time, consistent with the trends seen in the initial 1495 reports identified in the literature searches.
The finding that the majority of technologies were intended to be used by EOCs is likely reflective of the scoping of this systematic review, which focused on technologies providing SA to disaster responders, and excluded technologies aimed for use by the general public. In addition, AARs were significantly more likely to mention technologies for first responders, which may be because AARs provide detailed analyses of all participants in a response, whereas articles do not always describe all potential end-users or use cases of their technologies. It is also possible that this discrepancy exists because of inadequate research on technologies for first responders, such as safe and timely recall and evacuation of first responders,217,254 tracking the locations and status of fellow first responders and required supplies,17,39,217,248,249 and reliable communication of data to and from the EOC.17,35,247,254,255 The most frequently mentioned sector in which technologies could be implemented was DHS, the parent organization to FEMA and the US Coast Guard, which are among the federal agencies most frequently involved in disaster response activities in the United States. SA technologies identified in this review were relevant to all types of disasters, with all types of disasters being well-represented.
Similar types of gaps were described over time, suggesting chronic issues in disaster response technologies. However, there was inadequate data to determine whether these gaps were resolved over time. The most prevalent gap in articles and AARs was communications and connectivity, followed by analysis and visualization, and interoperability and sensor capabilities. Communications, data generation/collection, user interface, and data aggregation technologies were significantly more frequently mentioned in AARs, suggesting that the research community may not be prioritizing the areas of greatest need by the operations community. While the majority of AARs described using the first 3 technologies, the technologies were inadequate; thus, the gap persists.
Meanwhile, research on data aggregation technologies was limited, which may reflect a perception among researchers that high-quality data aggregation technologies already exist. Data analysis, interpretation, and identification technologies were mentioned by very few or no AARs, likely because AARs were inadequately detailed. In addition, gaps in communications and connectivity, infrastructure, training, and architecture were mentioned more often in AARs than in articles. Given the scope of the systematic review, which focused on technologies, it is possible that not all articles mentioning gaps in infrastructure, training, and architecture would be located by the search terms used. However, the search terms did identify articles focusing on communications and connectivity gaps. In other words, disaster response agencies were frequently reporting gaps in communications and connectivity, but academics and researchers were not, suggesting a disconnect between the state of the science and the technologies being used by disaster response agencies.
Communications and connectivity challenges, such as damaged or absent infrastructure,35,162,247,255 inadequate bandwidth for data to be transmitted from first responders in the field to the EOC,35,255 and high call and email volumes,245,247,254 are extremely common during disasters. Articles in this review highlighted promising potential solutions to these challenges, such as delay-tolerant networks,Reference Abrajano, Favila and Luo144,Reference Fajardo, Yasumoto and Ito330,Reference Fall, Iannaccone and Kannan331 mobile ad-hoc networksReference Bader and Alouini178,Reference Brown, Salmanian and Li273,Reference Chandra-Sekaran, Nwokafor and Johansson284,Reference Chen, Peña-Mora and Mehta287-Reference Cheng, Meiss and Park289 including those using drones,Reference Kim, Nam and Mahmud110,Reference Abrajano, Favila and Luo144 ultra-wideband technology,Reference Gorlatova, Kinget and Kymissis63,Reference Nekoogar and Dowla152 and more. However, they are largely still immature. Additional development of these technologies to reach a higher maturity level and additional investment into communications infrastructure, such as redundant systems, are needed to improve SA during disaster response.
Data analysis was identified as another significant challenge during disaster response. For example, responses to 2 of the most salient recent disasters, the Deepwater Horizon incident and the Fukushima nuclear disaster, experienced difficulty with processing, modeling, and understanding highly technical data17,243 ; inadvertent omission of certain sensor data in models that might have predicted the Tōhoku tsunamiReference Hasegawa252; and an overwhelming volume of data to be processed. These issues required substantial investments of time, energy, and resources.17,243 The AAR focusing on the 2017 wildfires in Sonoma, California, specifically noted that the Geographic Information Systems technology being used during the response was outdated.247 These findings suggest limited penetrance of novel data analysis technologies among disaster response agencies, and present an opportunity for disaster response agencies to increase the efficiency and effectiveness of their handling and interpretation of data through adoption of these technologies.
For example, while a minority (15%) of AARs reported using data analysis technologies during disaster response, newer types of these technologies now exist, such as those using artificial intelligence and machine learning (AI/ML), which have the potential to autonomously ingest, analyze, generate anomaly alerts, and make inferences and conclusions about large volumes of data in real time. Examples included ML analysis of social media posts to detect and localize an incidentReference Giridhar, Lee and Abdelzaher59,Reference Avvenuti, Del Vigna and Cresci145,Reference Preece, Roberts and Rogers172 and machine vision-based detection of anomalies, such as fire, and prediction about the severity of disaster damage.Reference Qureshi, Ekpanyapong and Dailey175,Reference Ramchurn, Huynh and Wu177 If implemented, AI/ML has the potential to revolutionize SA during disaster response operations. Articles also mentioned data analysis architectures, such as fog and edge computing,Reference Gargees, Morago and Pelapur55,Reference Greco, Ritrovato and Xhafa65 that enable data processing and analysis (including sensor data and video footage) close to the field collection site, rather than requiring transmission to a central server in the EOC for integration and analysis and transmission back to first responders, thus saving valuable time during a disaster response.
While sensor capabilities were identified as another gap during response activities, many articles described technologies to overcome these limitations. Examples included remote sensors, such as satellites and drones, to detect conditions on the ground in difficult-to-access regionsReference Foroushani and Damadi41,Reference Howden and Brendley82,Reference Jain, Sibley and Stryhn87,Reference Lundberg, Sevil and Das138,Reference Oh, Joyce and Gallagher158,Reference Towler, Krawiec and Kochersberger216,Reference Bhanumurthy and Behera261,Reference Brunke, Aubé and Legaré274,Reference Burman, Hespanha and Madhow276,Reference Di Lazzaro, Angino and Piemontese316 ; infrared sensors that enable image detection in low-visibility conditions, such as nighttime, smoke, or bad weatherReference Francisco, Roberts and Hanna43,Reference Vandecasteele, Merci and Verstockt220,Reference Young and Martin231,Reference Burnett and Wing277 ; and Radio Frequency Identification (RFID), which is a low-power device that can track the location of disaster supplies and victims,Reference Ganz, Schafer and Tang49,Reference Jokela, Rådestad and Gryth90,Reference Chandra-Sekaran, Flaig and Kunze285 as a replacement for spray-painted Building Marking SystemsReference Chen, Peña-Mora and Mehta287,Reference Chen, Peña-Mora and Plans288 ; and more. Other articles described architectures for integrating sensor data from different networks in real time,Reference Gray, Sadler and Kit64,Reference Alamdar, Kalantari and Rajabifard301 thus providing timely and common SA to all response agencies.
Finally, AARs identified data aggregation technologies, such as WebEOC and other shared platforms, as a major area for improvement. These shared platforms were not portable into the field, meaning that first responders used paper to collect data,243 and they often required a high degree of customization before use.243,245 Other challenges with shared platforms were related to gaps in communications and connectivity, training, and interoperability. For example, AARs reported numerous users whose accounts had not been authorized to access a shared platform,245,253 inadequate training of staff to effectively use the shared platform, resulting in paper-based data aggregation in EOCs,243 poor interoperability between computing infrastructure,17 and variable data security requirements at different agencies,17 resulting in ineffective data sharing.
In some cases, a shared platform was not available during the response.162 It may be valuable to conduct additional research and development to make such data aggregation platforms more user-friendly to limit the amount of training required for their effective use, and to enable their use in the field. For disaster response agencies and all levels of government, it would be important to establish data use agreements proactively, and to either ensure interoperability between their platforms or switch to a common platform, well in advance of the onset of a disaster. Other key enablers of effective shared platforms include addressing gaps in communications and connectivity, training of staff, and interoperability between sensors and data aggregation platforms.
These handful of examples suggest that there are numerous technologies that can fill gaps in disaster response operations. Introducing newer versions of all types of technologies into disaster response activities has the potential to substantially improve the ability of disaster response agencies to acquire real-time information, efficiently analyze the large volumes of data they receive, share information with one another on a common platform, and quickly request and deploy relevant resources during a disaster. In other words, more rapidly transitioning new technologies from researchers to disaster response agencies has the potential to transform disaster response agencies’ ability to gain SA, and thus to respond efficiently and effectively during a disaster.
While some technologies mentioned in articles were at the implementation stage, the majority of technologies across all technology categories were immature, which suggests an ongoing challenge in transitioning technologies from research and development to the field. Additionally, this highlights a gap in the systematic evaluation of technologies that have been implemented at large scales. Assessments of mature technologies would aid agencies that seek technologies to expand their capabilities. An important limitation of this systematic review is that the majority of data sources were published research articles or conference proceedings, which are skewed toward reporting immature technologies. Technologies that are beyond early research and development phases, but have yet to be commercialized, were likely excluded by virtue of not having been published. It is also possible that there was variability in the extraction of data. Because the search terms were intended to capture technologies, this systematic review was unable to capture gaps related to policy, training, and other non-technology issues. An important area of further research may include assessing whether a technology gap actually exists among disaster response agencies, or if gaps in policy or training prevent responders from knowing about or properly using available technology. As only English-language articles and AARs were captured in this review, it is likely that both the breadth and maturity of technologies that exist in reality is greater than is indicated by this systematic review. Importantly, the newest, most cutting-edge research will not be captured by a systematic review, particularly in technology fields, due to time lags in writing and publishing of journal articles.
CONCLUSIONS
Timely, accurate, and complete SA is a key enabler of successful disaster response, where the situation is changing rapidly, resources are limited, and different agencies must coordinate their activities. While policy and governance are the foundation of effective disaster response, technologies have the potential to provide rapid and shared SA for response agencies. This systematic review aimed to identify existing technologies that can be used to obtain SA during a disaster response, classify them based upon maturity level, and compare existing technologies with identified technological gaps in disaster response activities. This review identified a substantial divide between what research shows is the state of the science and the technologies that disaster response agencies are currently using. In addition, while the number of AARs was small, many technological gaps experienced by disaster response agencies seemed to be chronic issues. Further research should investigate whether these gaps are persisting over time.
Moreover, many of these challenges could be partially or fully addressed by implementing existing technologies, particularly in the areas of communications, data analysis, interoperability, and user interfaces. For example, communications and connectivity was by far the most commonly-reported gap in AARs, and was significantly less frequently reported as a gap in articles. Investing in research and maturation of, and implementing existing mature communications technologies, would profoundly impact the ability of EOCs and first responders to share information reliably and rapidly in settings with damaged or absent infrastructure. There is also a need for more research evaluating the large-scale implementation of technologies, which could aid in the uptake of mature technologies by agencies. More efficient acquisition and implementation of relevant novel technologies by disaster response agencies are recommended to improve the speed, quality, and coordination of SA in disaster response.
Acknowledgments
The team acknowledges helpful discussion with several individuals, particularly including Christine Fox, Matt Schaffer, and Jen Dailey.
Funding
This study was internally funded by the Johns Hopkins University Applied Physics Laboratory.
Supplementary material
To view supplementary material for this article, please visit https://doi.org/10.1017/dmp.2020.196