Introduction
Of the many challenges to the successful conduct of clinical trials, a common disabling one is that a planned study cohort fails to materialize and enrollment falls short of expectations. One approach to addressing this has been to try to make accurate projections of available study cohorts at institutions by use of electronic health record (EHR) data.Reference Hripcsak and Albers1 EHR data warehouses can be searched for patients with the characteristics anticipated to meet enrollment criteria. This “cohort discovery” allows estimation of the likely numbers of patients at sites who could be available for a given trial.
However, technical and data quality issues can make this approach challenging. Cohort discovery using EHR data uses criteria for projected trial enrollment based on controlled terminologies, such as ICD-9/ICD-10 for medical conditions, CPT for medical procedures, RxNorm for medications, and LOINC for laboratory measurements. Hampering fidelity to the intended clinical criteria is that these EHR terms were developed for billing, prescription processing, and other purposes not directly related to a patient’s clinical or physiological state. Additional impediments to accurate case finding are complexity, inaccuracies, and incompleteness of data.Reference Hripcsak and Albers1 And while EHRs also contain data more closely related to patients’ physiological states, the prevailing approaches to cohort definitions do not often exploit these sources. A recent review of EHR-derived cohort definitions for acute myocardial infarction (AMI)Reference Rubbo2 found that of 33 studies examining the definition’s accuracy, only one used troponin levels (the standard biomarker for AMI) in combination with ICD diagnosis codes,Reference Gronski3 one used free-text related to symptoms in combination with ICD codes,Reference Coloma4 and none used electronic electrocardiographic data, the most common enrollment criteria for clinical trials of AMI.
Although EHR data warehouses have gained broad acceptance as a means of cohort discovery, to our knowledge, there have not yet been published studies of the success of EHR-based strategies in predicting enrollment in actual conducted trials. Given this void, and based on our previous experience using medical devices for clinical trial enrollment, we developed an alternative device-based strategy for projecting trial enrollment based on the point of care encounter in which a patient is evaluated and potentially enrolled. We compared its performance with data types typically used in EHR-based strategies, acknowledging that the need for the implementation of the device-based approach at the point of care does not permit direct comparisons of the two approaches.
The alternative approach we propose identifies potential study participants using data from medical devices used in real time for the clinical diagnosis that is the focus of trial enrollment. As an example, conventional computerized electrocardiographs can identify acute coronary syndromes (ACS), including acute cardiac ischemia time-insensitive predictive instrument (ACI-TIPI) predictions of ACS printed on the electrocardiogram (ECG) text header, and ST elevation myocardial infarction (STEMI), which can prompt clinicians to offer patients enrollment in a trial for these conditions, and has worked well for enrollment in hospital emergency department (ED) and emergency medical service (EMS) settings.Reference Selker5–Reference Selker10 Also, these electrocardiographic data can be used to monitor completeness of enrollment at trial sites. By checking the electrocardiograph management system’s database, the numbers of patients actually enrolled can be compared to the denominator of all those among stored ECGs that have the qualifying features (e.g., STEMI or high ACI-TIPI probability of ACS). We believe the ECG management database also could be used to project available patients for a clinical trial for which the electrocardiograph would be central to diagnosis, treatment, and enrollment. By searching ECG databases for patients with ECGs that qualify for enrollment, accurate projections of available cohorts should be possible. In this project, we aimed to demonstrate this approach for cohort discovery for a planned ACS clinical trial.
Methods
This project was done to accurately estimate enrollment at six candidate hospitals for participation in the planned IMMEDIATE-2 Trial. Based on our previous IMMEDIATE Trial,Reference Selker10 IMMEDIATE-2 will use the same enrollment criteria among patients age 30 or more presenting with symptoms suggestive of ACS: having 12-lead ECGs that reflect high likelihoods of ACS (ACI-TIPI prediction of ACS of > 75%) or STEMI. For device-based cohort discovery, we accessed the hospitals’ ECG management systems to apply the ECG-based enrollment criteria, and for comparison, we did analogous searches using the hospitals’ EHR data warehouses (Table 1).
Table 1. EHR-based cohort discovery criteria
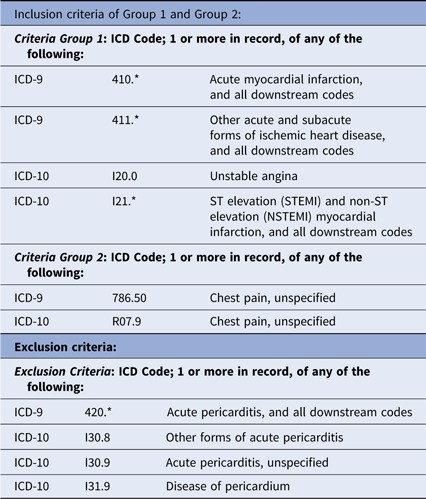
*Indicates the inclusion of all sub-ICD-9/ICD-10 codes under the top level ICD-9/ICD-10 code category
For device-based cohort discovery using data collected on ED electrocardiographs, we directly applied the IMMEDIATE-2 ECG inclusion criteria to data acquired by the hospitals’ native ED electrocardiographs (Philips PageWriter or GE Mac) stored in the hospitals’ ECG data management systems (Philips TraceMaster Vue, GE MUSE, or Epiphany Cardioserver). With institutional review board approval or exemption, this was done at each site to determine the number of patients meeting the criteria over a three-month period, and then the rates were annualized for enrollment projections.
To compute the ACI-TIPI probability of ACS, besides data provided by the electrocardiograph, one of the required variables is whether the patient has chest pain and whether it is the chief complaint. For enrollment in real time, this is easily obtained from the patient, as done in the original IMMEDIATE Trial. However, among the hospitals participating in this cohort projection, not all had collected this symptom report in the ED electrocardiographs when doing the first (or any) ECG. Thus, sources other than ED electrocardiographs were required for this variable. For this, we used ED patient logs or hospitals’ ED and/or EHR systems. However, from these sources, the ACI-TIPI chest pain variable levels (primary, secondary, or none) were difficult to reliably ascertain.
Based on this finding and because we sought to only use device-based data, we created a new version of ACI-TIPI, “e-ACI-TIPI,” that only used electrocardiographic data or data reliably acquired in obtaining an ECG (age and gender). After deleting the variables for chest pain, we recomputed the logistic regression coefficients of the original ACI-TIPI using only age, gender, and ECG waveform measurements as the only variables. To allow direct comparisons of the original and modified models, we generated the e-ACI-TIPI coefficients on the same database on which ACI-TIPI had been developed, and then tested it on the same data set on which the original ACI-TIPI was tested, using receiver-operating characteristic (ROC) curve area and calibration as metrics of performance.Reference Selker, Griffith and D’Agostino11 We also compared the full ACI-TIPI and e-ACI-TIPI using a database collected directly from ED electrocardiographs in a national trial of the use of ACI-TIPI.Reference Selker6 Additionally, the two models were compared as to the patients they identified when applying the IMMEDIATE-2 Trial inclusion criterion of > 75% probability of ACS.
As a reference for comparison of the electrocardiograph-based approach to EHR-based cohort discovery, we projected the likely IMMEDIATE-2 cohort using hospitals’ EHR data warehouses. Search criteria were based on codes derived through an Extraction/Transform/Load (ETL) process to provide demographics and ICD-9/ICD-10 codes that matched the target diagnoses, ACS, AMI, and STEMI (Table 1). The results were reviewed for fidelity to the intended diagnostic categories, but refined use of EHR data beyond these diagnostic codes, age, gender, and admission via the ED was beyond this project’s scope.
For the EHR-based approach, the criteria in Table 1 were transformed into an SQL query for searching EHR data warehouses (or operational EHR systems), the results of which were exported into a Microsoft Excel spreadsheet for counting patients meeting target inclusion criteria and age. These data were from the same three-month period as used for electrocardiograph-based cohort discovery, and were multiplied by four to generate annualized rates. At Hospital 1, as a check of the match of identification by EHRs and ED electrocardiographs, we checked a sample of EHR-identified patients to determine if they would qualify for enrollment using IMMEDIATE-2 ECG criteria.
Results
Table 2 provides the participating hospitals’ annual numbers of ED visits and numbers of patients on whom ECGs were performed in the ED.
Table 2. Institution characteristics

The logistic regression coefficients for the original ACI-TIPI and e-ACI-TIPI are in Table 3. The ROC areas for ACI-TIPI and e-ACI-TIPI, on development and test data sets, are in Table 4; their calibration graphs are in Fig. 1. The ROC areas were slightly less in the e-ACI-TIPI compared to the ACI-TIPI, both in the development and test data sets, but being at or above 0.8 in all cases reflected excellent diagnostic performance. When applied to data collected from electrocardiographs in EDs nationally, the ROC for e-ACI-TIPI was lower, at 0.69, but still reflecting very good performance.

Fig. 1. Calibration plots – Observed vs estimate proportions of patients with ACI (synonymous with ACS) across deciles of predicted values: Original ACI-TIPI vs e-ACI-TIPI models in this figure. Original ACI-TIPI development data (top figure) and two validation data sets (bottom figures).
Table 3. ACI-TIPI and e-ACI-TIPI model coefficients (estimated from ACI-TIPI development database of n = 3453 subjects, of whom 1251 had ACSReference Selker, Griffith and D’Agostino11)

Table 4. ROC Areas for original ACI-TIPI and e-ACI-TIPI

The comparisons of results of applying the IMMEDIATE inclusion criterion of > 75% using the original ACI-TIPI and e-ACI-TIPI are shown in Table 5. Although statistically significantly different, the proportions identified by each approach were very close. The e-ACI-TIPI detected about 20% fewer patients, and thus provides conservative estimates of patient numbers that the full ACI-TIPI would detect in real-time care.
Table 5. Evaluation of application of > 75% probability of ACS threshold

The cohort discovery results, based on three-month assessments and expressed as one-year estimates to project potential accrual over one year, are in Table 6. The EHR-based cohort projections for patients with ACS had wide differences in total counts across different hospitals, and the estimates across hospitals made by the e-ACI-TIPI were more consistent. We compared patient discovery using the ECG- and EHR-based methods at the two hospitals at which sufficiently detailed data were available. We found little overlap between the ECG- and EHR-based cohorts. A suggestion of the cause of the discrepancy was obtained from clinical reviews done at Hospital 1, where among 16 EHR-identified patients, 14 had ECGs done in the ED, of which only one met IMMEDIATE-2 ECG enrollment criteria.
Also reflected in Table 6, we compared the cohort estimates based on the ACI-TIPI compared to the e-ACI-TIPI, looking for the influence of variation in the chest pain variable, which is present in ACI-TIPI but not in e-ACT-TIPI. No hospital had uniform presence of electronic data on this variable, which were derived at each site using the best available data, which had limitations. At Hospital 1, the ED Director considered the chief complaint recorded on the electrocardiograph as unreliable, and so as a default, we made the most conservative assumption that would lower the estimate of the ACS cohort, that is, that the patients did not have chest pain. Hospital 2 did have data from their ED records of chief complaints and reasons for ED visits, from which we could infer the three-level variable, chest pain as a primary complaint, a secondary complaint, or not present. For Hospital 3, the presence of chest pain, not differentiated as primary or secondary, was based on the ED chief complaint. For Hospital 4, the variable was derived from the reason the ECG was ordered, simply chest pain present or absent. Also for Hospital 5, the chest pain variable was the reason the ECG was ordered, but from a short list of potential reasons: six different chest pain-related reasons, compared to more than 70 chest pain-related reasons at Hospital 4. At Hospital 6, there were no chest pain data, and so, in part to illustrate the range that this variable could induce, we simulated the ACI-TIPI each as if patients had a chief complaint of chest pain, a secondary complaint of chest pain, or no chest pain, shown in the last three rows of Table 6.
Table 6. Cohort discovery findings (numbers of patients identified, annualized) among patients > age 30 admitted via EDs using EHR- and electrocardiograph-based projections

aUsing “no chest pain” for ACI-TIPI calculation. bUsing “primary chest pain” status for ACI-TIPI calculation. cUsing “secondary chest pain” status for ACI-TIPI calculation.
This uncertainty and variety in the ACI-TIPI chest pain variable were reflected in the differences between the cohorts identified by it and the e-ACI-TIPI. In the case of Hospital 2, where two independent EHR documentation fields were available and used (chief complaint and reason for visit), cohort discovery was more consistent between the ACI-TIPI (284 patients) and e-ACI-TIPI (316 patients), compared to the other hospitals at which only a single EHR field (chief complaint) was available (ACI-TIPI vs e-ACI-TIPI being, respectively, 8 vs 28 patients; 400 vs 448 patients; 136 vs 256 patients; and 124 vs 216 patients). This finding illustrates the variability in local EHR documentation and shows the e-ACI-TIPI to be a more consistent instrument for cohort discovery across varied ED environments and EHR documentation practices. At Hospital 6, where the full ACI-TIPI could not be computed because of the absence of data for the chest pain variable, the range of projections by simulating by use of the three levels of the chest pain variable (primary, secondary, or none) were, respectively, 1980 patients, 636 patients, and 224 patients. This illustrates the impact of removing the chest pain variable from the ACI-TIPI model for cohort projection.
Discussion
Medical devices can be used for diagnosing, treating, and identifying patients for enrollment into clinical trials, such as electrocardiographs for ACS. In this investigation, at six hospitals, using electrocardiograph databases of ECGs done in their EDs, we generated cohort estimates for potential enrollment into the IMMEDIATE-2 Trial. Having used the electrocardiograph-based ACI-TIPI predictions of ACI to diagnose, treat, and enroll patients in the original IMMEDIATE Trial, these cohort projections for IMMEDIATE-2 used the same inclusion criteria as in the original trial.
Because the original electrocardiograph-based ACI-TIPI will be used to support enrollment decision-making in the IMMEDIATE-2 Trial, we started cohort discovery using the ACI-TIPI to search the hospitals’ ECG databases. However, a patient’s chest pain status, which is required by ACI-TIPI, typically obtained in real-time care, was not reliably recorded along with ECGs in routine ED care. Thus, the original ACI-TIPI was impractical to use for solely device-based cohort identification. To allow reliable retrospective device-based calculations for this purpose, we created the completely ECG-based e-ACI-TIPI that did not require the chest pain variable. When tested on the same independent database on which the original ACI-TIPI was tested, the e-ACI-TIPI’s performance was very similar to the original version. Moreover, the new e-ACI-TIPI was not hampered by the uncertainty of the chest pain variable assessed retrospectively at hospitals as was the case with the full ACI-TIPI, which resulted in more consistent predictions across EDs. This finding supports the importance of limiting device-based cohort discovery methods to only data directly available from the diagnostic tool. It also supports the notion that a single source of key data needed for identification of the study cohort may be better than using multiple sources with less predictive value in an aggregated fashion. This may be especially true when looking at EHR data across institutions where heterogeneity is likely in information coding and completeness, as well as organizational differences that affect data collection. Nonetheless, given that the full ACI-TIPI has been extensively tested in clinical careReference Selker6 and is available in conventional electrocardiographs, we intend that this version be used in clinical care and studies.
As reference, we compared the device-based estimates with an EHR-based cohort discovery approach for the IMMEDIATE-2 Trial. The EHR-based cohort counts differed significantly among hospitals and were inconsistent with the ECG-based counts. At the two hospitals for which comparison was possible, the overlap in cases detected by the EHR-based cohort and ECG-based cohort was negligible. Also, a small sample from one of the hospitals showed that many EHR-identified cases did not have an ED ECG that showed changes of ACS (one of 16 reviewed), as would be required for enrollment into the IMMEDIATE-2 Trial. Given that an ED ECG showing a high probability of ACS will be central to IMMEDIATE-2 enrollment, the electrocardiograph-based cohort projection, and in particular, using e-ACI-TIPI, seems to most accurately predict available patients for the trial.
The difficulty in using the EHR approach in this case may encompass issues affecting both specificity and sensitivity. Given that the EHR-based method seemed to miss ED patients with ECGs showing ACS, it seems unlikely to have high sensitivity for detecting potential candidates for the planned trial. However, it also appear not to have good specificity either, as it did not appears to distinguish ED patients whose ECGs do not show ACS. In contrast, the electrocardiograph-based approach appears to have both high sensitivity and specificity to identify patients presenting with ACS in the ED, the target for the planned IMMEDIATE-2 Trial. We understand that this is not a fair direct comparison of the two methods. This project had the specific objective of demonstrating an approach for a trial that will use that device-based discovery for real-time enrollment; EHR-based discovery may be appropriate for other trials. With both approaches, cohort projections should be tested by comparisons to the numbers of enrolled participants at the same centers.
We conclude that when there is an opportunity to use a device-based approach for cohort discovery, it may be a preferable method. In our example, the ECG-based approach appeared to better identify patients who would be appropriate candidates for the proposed study of ACS in the ED. This approach also could be used with other diagnostic devices, such as CT scans used for detecting intracranial bleeds, oximetry for identifying hypoxia, wearable devices recording physiologic parameters, clinical diagnostic instruments, such as those used for biochemistry testing, and potentially combinations of device-based diagnostic information.
Device-based trial enrollment also may have the advantage of enrolling as many patients as possible, in an unbiased way, to optimally acquire substantial generalizable study samples (potentially with randomization incorporated into the device). Then, the ECG database provides a record of all eligible patients seen at the point of evaluation, rather than requiring clinicians to trigger enrollment and study coordinators to detect all potentially eligible patients. For studies of ACS and STEMI, such as the IMMEDIATE Trials, all patients presenting with symptoms consistent with ACS for whom an ECG demonstrates ischemia are eligible for enrollment. Therefore, using the electrocardiograph as the key diagnostic device, the denominator of eligible patients is available and unbiased, and enrollment rates can be used as a metric for improvement. For example, in the original IMMEDIATE Trial, using this approach in community-based care with EMS-based defibrillator-electrocardiographs, 52% of all eligible patients were enrolled.
This ability to identify the denominator of all eligible patients for enrollment is what enables the pre-study projection of an available cohort, as illustrated in this study. It has the advantage over EHR-based approaches in that the denominator is clinically based on the specific enrollment criteria as will be used in the trial, at the point of care and where enrollment will take place. Additionally, the assessment is much more straightforward – downloading ECGs and scanning for qualifying abnormalities rather than trying to map various diagnostic codes to categories of likely enrollees.
The question arises, both for device-based trial enrollment and for cohort discovery, how might this approach be expanded to devices other than the electrocardiograph? As alluded to above, analogous opportunities should be considered for other devices that are central to diagnosing patient conditions, such as CT scanners for patients with acute neurological problems, oximetry for those with respiratory problems, wearable devices that reveal conditions needing intervention, and many others. Indeed, this approach also could be based on the use of predictive instrument decision support for shared decision-making for patients and clinicians using clinical trial enrollment based on “mathematical equipoise.”Reference Selker12 All of these approaches deserve investigation in our efforts to improve clinical trial cohort projection, ultimately to assist the effective and efficient conduct of trials.
We believe this approach has promise, but verification will require comparisons of cohort projections to the actual numbers enrolled in trials. Although not typically done systematically and published, such verification is necessary for trialists to have confidence in the projections. This is the work we intend to do in the IMMEDIATE-2 Trial.
Acknowledgment
The authors thank Maggie Towne, MSc, for support of this project and expert manuscript preparation.
Conflict of Interest
The authors do not have any conflicts to declare.
Funding
Research in this report was funded through Tufts University Clinical and Translational Science Award (CTSA), NIH NCATS grants 3UL1TR001064 and UL1TR002544, Johns Hopkins-Tufts CTSA Network Trial Innovation Center, NIH NCATS grant 3U24TR001609, Vanderbilt University Recruitment Innovation Center grant 5U24TR001579-03, and RUS at University of Utah is supported by a National Heart, Lung, and Blood Institute grant K08HL136850.