Introduction: A Current Opportunity
Digital Health technologies (DHTs) are IT applications dedicated to improving healthcare delivery and its support processes. This broad category includes technologies such as mobile Health/mHealth (such as mobile apps), wearables (for example, Fitbit), and telehealth, including telemedicine and clinical decision support systems (CDSS). Digital Health technologies offer an answer to healthcare challenges on many levels: On an international level, the WHO deems them to systematically support “health system needs” as part of the Sustainable Development Goals (SDGs). This also includes improved access to care for patients living in remote areas, populations particularly present in low- and middle-income countries. On a national level, an increasing number of governments and healthcare system payors see DHTs as an opportunity to reign in escalating expenditures while increasing population health overall. Finally, on the level of individual patients, the consumerization of healthcare technologies in digital formats allows patients to play a larger role in their own health with the border line between healthcare, health and well-being becoming increasingly blurry. DHTs have been attracting a great deal of attention also by businesses. Both traditional Pharma and MedTech as well as additional players, such as venture capitalists and IT Companies, are pouring in resources as they recognize the market potential of DHTs in replacing and/or changing existing healthcare markets.Reference DeSilva and Zweig 1
Despite the potential, the problem of regulation lagging behind technological development remains unresolved. New technologies come with new and old risks. While there are clear and specific rules for drug development and use as well as improving rules on medical devices — digital health technologies remain largely uncharted territory from a regulatory perspective.Reference Iqbal and Biller-Andorno 2 In the sphere of digital health, the current debate features conflicts of the new technologies with ethical principles, including the “Big-4”: autonomy, benevolence, fairness and justice, and non-malevolence. These classical bioethical issues are joined by concerns over privacy, responsibility and accountability, solidarity, and transparency, among others. Unfortunately, the debate on ethical principles, appropriate regulation and testing and validation procedures is lacking a few years behind the state of technical development as well as adoption of DHTs.
A new technology frontier is currently opening — offering a chance for bioethics to think ahead of technological development and anticipate ethical challenges. Digital Twins are live or near-live representations of physical entities, either living or non-livingReference El Saddik 3 and were first proposed as an Engineering paradigm in the early 2000s as the “Mirrored Spaces Model.”Reference Grieves 4 The concept is gaining increasing interest and adoption in various Engineering fields, e.g. aircraft engines, industrial plants and power grids.Reference Tao, Zhang, Liu and Nee 5 These application fields representing capital-intensive and complex systems share their high cost (time, money) in both R&D and deployment technology life cycles (activities like prototyping and testing) as well as high cost of lost functionality during operations.
This paper aims to introduce the reader to the concept of digital twins, its technological foundations, application areas and identify potential ethical challenges in context of the debate on related technologies and a potentially changed future practice of medicine.
In a digital twin, a physical twin with its characteristics is mirrored through a flow of data on its status from the physical twin to the digital twin. Its usefulness stems from the feedback loop of recommendations on actions to be taken, such as adjustments and preventive maintenance measures.
Take the simple but limited example of a collaborative robot used in a production factory environment picking and placing parts: The robot is experiencing wear and tear throughout its use — somewhat similar to a human, this wear and tear particularly affects its joints after repetitive movements. Failure of a robot in a factory environment is costly as it may slow or halt production and render many other resources idle. Torque sensors in all of the joints of the robot continuously measure force data as well as operating hours that are fed to the digital twin of the robot in its simulation software. The digital twin may then recommend — both on changing force characteristics as well as expected operating lifetime hours — a preventive maintenance action (joint replacement) and/or changing its operating speed in one joint and takeover of range movement by another joint.
Digital Twins of humans may draw on actual patient data from the large spectrum of traditional medical data (blood work, imaging, etc.) and non-medical data (e.g., social media data) as well as the various shades of grey in between (e.g., step counts and sound volume data from mobile devices). They represent a technological evolution step beyond just digitalization (the process of converting data, information and knowledge from non-digital to digital formats and their subsequent applications) but into virtualization (the representation of some physical asset in a digital format). Their development and use in healthcare are in their infancy, therefore offering an opportunity to foresee and react to ethical challenges that may arise. This paper aims to introduce the reader to the concept of digital twins, its technological foundations, application areas and identify potential ethical challenges in context of the debate on related technologies and a potentially changed future practice of medicine.
Digital Twins: The Technology
A digital twin may be implemented in many technical ways. While an implementation based completely on deep learning may be feasible in the future,Reference Pang, Pillai and Nguyen 7 a digital twin modeling approach typically consists of two components: a mechanistic model and a machine learning model. Through the combination of these model components drawing upon multiple sources of data, they reach their patient-specific level of personalization.
Mechanistic Models
Mechanistic models rely on the assumption that any complex physical system can be represented by modeling its individual components and interactions thereof. This requires a prior explicit understanding of the constituent parts and laws of interactions. In the medical example of the heart, such a model would encompass the electrophysiology, mechanics of the muscle and dynamics of the heart as well as their connection via electro-mechanical coupling and fluid-solid interaction. What sounds simple, encompasses highly complex modeling efforts from the cellular level up to the macro level on supercomputers.Reference Santiago, Aguado-Sierra and Zavala-Aké 8 Other more traditional examples include bone mechanics, including adaptation, remodeling and healing.Reference Bhattachary and Viceconti9
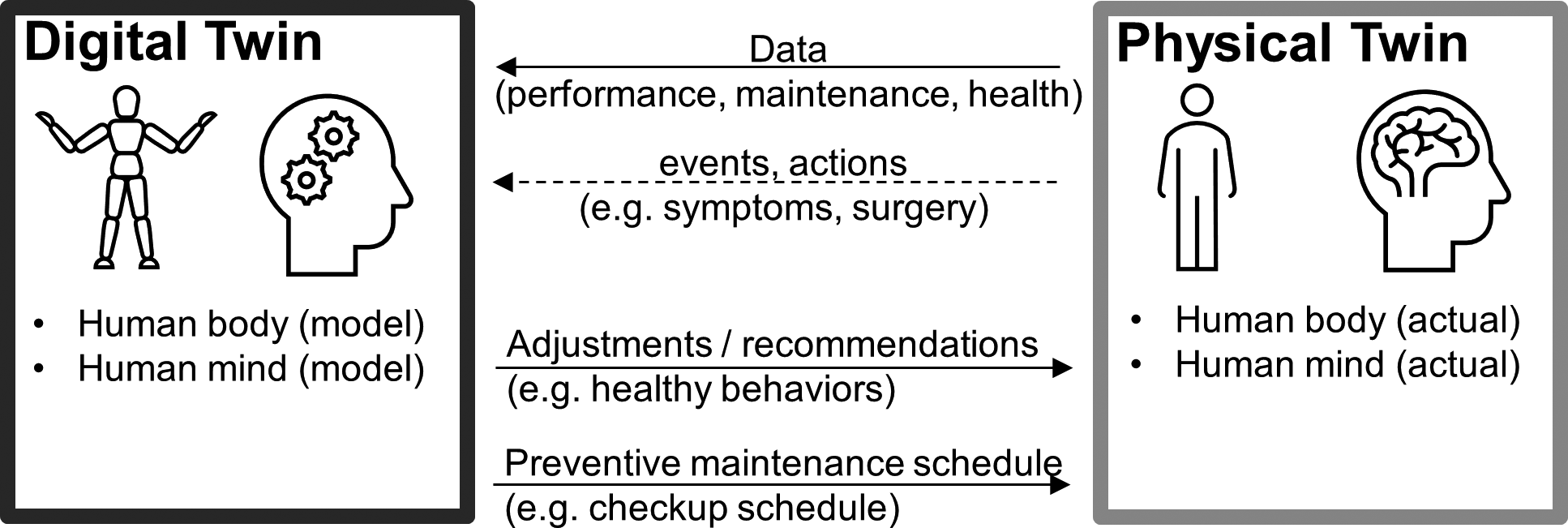
Figure 1 Adopted from Madni,Reference Madni, Madni and Lucero6 the digital twin approach may be extended to humans.
Such models are the domain of biophysics and a large toolbox of modeling techniques is available. Typically, the first step in modeling is geometry generation, where a computer-aided design (CAD) software is used to generate the macro-geometric structures, such as blood vessels or heart valves. Then, discretization of components — where individual cells or blocks of cells may represent discrete items — is undertaken with the finite element method (FEM) being an important method in solid matter modeling and finite volume method (FVM) representing its fluid matter counterpart. In a choice of solver software, such as ANSYS Fluent, a mathematical model is programmed with selections of algorithm, boundaries, and material properties. For example, elasticity of vessel wall structures via stress-strain characteristics may be entered and hemodynamic characteristics including viscosity approximated. Predicted outcomes on each component could be generated by changing one or several variables — such as bursting of an aneurysm in a specific spot by a spike increase of arterial blood pressure. Engineers, physicists and mathematicians dominate the field with their subject matter expertise.
Machine Learning Models
In contrast to mechanistic models, machine learning models work in a way that they learn via experience rather than pre-programmed explicit rules. While some of them may make knowledge explicit and comprehensible by humans, this is an optional and not always feasible step as the discussion on “Explainable AI” shows.Reference Arrieta, Díaz-Rodríguez and Del Ser 10 There is a current hype in medicine with many potential or actual applications in prevention, screening, diagnosis, treatment, and management of disease emerging. While there are many unregulated wellness applications globally, applications regulated as medical devices typically focus on diagnosis of disease. There are only few applications in the therapeutic/management domain so far, such as in opioid abuse disorder.Reference Velez, Colman and Kauffman 11 Common examples of such diagnosis applications include the sphere of image recognition (recognition of cancer or other pathologic conditions from medical images) and general pattern recognition (ECG and heart rate analysis for recognition of atrial fibrillation, tachycardia or bradycardia). Such pattern recognition applications traditionally are strongholds of machine learning.
While there are many types of machine learning, supervised classification learning is by far the most common and the underlying technique of the previous examples given. In this type of learning, pre-classified examples in a training dataset are used to train a model with an underlying algorithm to correctly classify a target variable, such as whether the patient has a disease or not or with which probability. First, data is collected, explored and prepared — for example heart disease patient data assembled, cleaned and reformatted into a unified format. Then, a model is trained with an algorithm and appropriate user-defined hyperparameters chosen. Finally, the model would be evaluated by applying it to a dataset yielding results — such as the probability of the presence of atherosclerotic coronary artery disease.
Digital Twin Models are a Combination of Both Technologies and May Break Current Boundaries in Healthcare Delivery
Both types of models are being developed in parallel at increasing speed. And both profit from improved hardware/computing power as well as software/statistical method developments. While mechanistic models profit from a (slowly) increasing understanding of underlying biophysics concepts, machine learning models benefit vastly from exponentially increasing pools of data. “Easy data” (data that is relatively easy to acquire and manipulate) has already been the norm for a few years in non-medical fields such as commercial management. Efforts in the standardization and interoperability of electronic health records and other medical data sources will likely switch the medical domain from big data to (relatively) easy data as well in the near future.

Figure 2 Digital Twins have three user groups: Healthcare Providers, MedTech and Pharma Industry and Individuals. Industry is currently leading the other groups in adoption.
Hybrid models combining mechanistic and machine learning models in the medical field exist and can provide much higher accuracy in predictive models than their individual components alone.Reference Gaw, Hawkins-Daarud and Hu12 The next evolution step lies in combining both types of models with a stream of (near) live data into a digital twin model. Just as a physician receives mechanistic training through medical school and later acquires experience though case work seeing thousands of patients, a digital twin — combining both types of models — can reap the learning benefits of both worlds and derive temporally meaningful (live or near-live) conclusions, and action recommendations. In contrast to a human, there is no reasonable limit to the amount of patient information or the number of patients its model draws on — while a human physician will rarely have meaningfully seen more than a few ten thousand patients, a machine can easily combine the data of millions of patients and recognize even sublime patterns with minimal statistical significance. To our knowledge, the largest medicine-related machine learning study has drawn upon data from more than 246 million patients.Reference Vashisht, Jung and Schuler13
In further contrast, while there are typically continuing education requirements for practicing HCPs, meaningful limits to the amount and detail of mechanistic knowledge exist in humans but not in machines. Physicians train in specialties of typically 6-7 years post-medical school and then focus on one relatively narrow domain of expertise, such as cardiology. Diagnoses may get missed, important information in communication between specialist doctors lost.
Already, many medical pattern recognition-type tasks can be done at the same or higher level of performance by machines vs. their human counterparts.Reference Loh14 We therefore believe that technologically, digital twins are capable of breaking current boundaries of both humans and individual technologies. While a machine will not be perfect either, it is important to set the right benchmark for any machine performance: that of a less than perfect human physician.
Digital Twins — User Groups, Applications, Functions and Specialty Areas
Digital Twin User Groups: Healthcare Providers, Industry and Individuals
The (potential) applications of digital twins can be categorized by user group: Healthcare Providers (HCP), Industry/Device and Drug Developers and Non-HCP Individuals. Non-HCP Individuals may independently use digital twins to monitor and improve their health status in the area of general well-being “wellness” and potentially beyond along the self-care spectrum 15 using models of themselves or even their unborn child in the womb.
Industry users, both MedTech and Pharma, are increasingly using a form of digital twins for in-silico modeling of medical devices and drugs in an attempt to reduce costs in development and time-to-market as well as to increase efficacy and safety of their products. Legislators, such as the U.S. Senate in 2015 16 and the EU Parliament in 2016, 17 are encouraging the use of in silico modeling as a further validation path potentially able to reduce, refine and replace both human and animal experimentation.
Digital Twin Application Areas in Medicine
Digital Twins may assist several medical functions and all phases of the life cycle of disease are actual or potential application areas of digital twins: pre-disease management, disease management as well as post-disease management with distinctions sometimes being not black-and-white. For example, prevention may be in the form of primary prevention, (prevention before disease occurrence), secondary prevention (prevention of progression during early stage of disease) or tertiary prevention (management of established disease states to halt progression and avoid complications). We refer to the categories of pre-disease, disease and post-disease management as medicine hereafter.
-
• Screening, detecting potential disease indicators in large numbers of asymptomatic but at-risk people, and prevention, here intended to mean mostly primary prevention, are functions that may or may not require the involvement of a HCP, often suffer from low adoption and offer potential for automation via the digital twin approach.
-
• Diagnosis, identifying a disease or other type of condition by its symptoms, is the main sphere of current machine learning given its power in pattern recognition. Performance of such systems could be improved by digital twin models.
-
• Prognosis, forecasting the course of development of a disease or other type of condition and its symptoms, is currently a process done by HCPs — depending on the setting — with or without help of tools (such as APACHE IV in the intensive care setting). Performance in this area tends to be relatively low even in forecasting the near future with prognoses typically being overoptimistic. This area is also strongly being developed by machine learning with clinical decision support systems which can profit from the digital twin approach to raise prognostic accuracy.
-
• Treatment, managing of and caring for a patient to combat the disease or condition typically with intent to cure, and alleviation, lessening the burden of a disease or condition, in themselves are currently not areas of application of the digital twin per se but their support is. Mental disease treatments, advanced in general digital health already, could change this soon. Little diabetes sensors in the bloodstream could also feed information to a digital twin and in a closed loop system administer insulin as needed.Reference Deplazes-Zemp, Fussenegger and Biller-Andorno 18
-
• Prediction, predicting the effect of an intervention against a disease or condition, as a support mechanism to treatment is where the strengths come to play as the most promising treatment (and alleviation) options may be selected for specific patients given the highly complex particularities of specific patients. Prediction also includes the function of guiding and optimizing treatments for optimal outcomes.
-
• Monitoring, checking the progress of a disease or condition, then rounds off the core functional picture where it allows for the enhanced observance of the current state as a pre-requisite of all other functions.
Digital Twins are on the Brink of Early Adoption in Medicine
Of the three user group-associated application areas, the most advanced one in terms of adoption is the area of in-silico clinical trials by industry users. Key jurisdictions actively foster the use of such technologies with the aim of speeding up time-to-market, lowering development costs and strengthening safety and efficacy of medical products. Particularly in the area of interventional invasive medical devices, companies such as MedTronic regularly submit in silico trial data as part of their FDA Device Applications. To our knowledge, there are no wellness-directed digital twins marketed at this moment and we foresee that this status will remain the same for the next years. Arguably, the definition of a digital twin given earlier — centering around the combination of mechanistic and machine learning models on a person-specific level with live data feeds — and the associated costs preclude the use of this technology currently.
In order to illustrate the different specialty areas of potential use, two examples are provided of large-impact problems in different specialty areas that are being addressed by digital twin technology. Further areas such as oncology — where, for example, solid tumors still present a sizable problem for choosing the best treatment option — present further use cases.
Example 1: Cardiology
Ventricular Arrhythmia is a type of electrical disorder of the heart that can lead to multiple severe and deadly complications. Often, pharmaceutical interventions are insufficient and the implantation of defibrillatory devices or cardial ablation, the “burning” of endo- and/or epicardial heart tissue via catheterization, may be indicated. The digital twin approach may serve along the entire lifecycle of the disease, but the function of prediction is particularly relevant. Given the combination of specific patient information, incl. various imaging modalities such as MRI and CT, a personalized mechanistic model of the patient’s heart can be built. Drawing from statistics of past interventions in patient cohorts with similar features, learnings — without them necessarily being fully explicit — may be used in recommendations for treatment efficacy. It could be determined early on that the probability of pharmaceutical interventions working is very slim and that preparations for and scheduling of surgical interventions should be made. Such models could then further assist in determining exact spots of the myocardium to be ablated at — leading to better outcomes. One such digital twin approach currently being in development and early use is MUSIC (Multi-modality platform for specific imaging in Cardiology) from LYRIC (Bordeaux, France). 19
Example 2: Neurosurgery
Intracranial arterial aneurysms are a common type of vascular condition featuring an abnormal localized dilation of an artery in the brain. The key risk associated is rupture due to mechanical weakness potentially resulting in haemorrhagic stroke. Roughly half of unruptured aneurysms stay asymptomatic and diagnosing them is difficult. Given risk factors of genetic predisposition (and family history), hypertension, smoking, and trauma — a digital twin model of a patient could enable the screening of larger patient populations and identify such asymptomatic but at-risk patients. Once identified, forecasting the probability of rupture of aneurysms is again a difficult task even for experienced specialists.Reference Jin, Song and Leng 20 Digital Twins may provide detailed wall stress simulations both before, during and after intervention with stents. They are combining a mechanistic model of the patient-specific anatomy of intracranial arteries containing the aneurysm partly derived from imaging modalities together with statistical models of large patient populations. One part of such an approach is being investigated.Reference Narata, de Moura and Larrabide 21
Digital Twins on Different Levels: Organ, Body, Mind
The human digital twin concept may be applied on different levels — individual organs (and substructures), the interactions of organs up to a body-level and potentially even on a mind-level, such as a representation of consciousness or select mental functions. Currently, development of the digital twin approach in medicine is on the organ-level with limited models existing for the heart,Reference Corral-Acero, Margara and Marciniak22 lung,Reference Yu, Xiaole and Jianan23 and liver.Reference Subramanian24 Implementations of the body-level digital twins modeling multi organ-organ interactions remain a vision. Mind-level digital twins — depending on degree of realization — are even further away but the subject of a field of interest termed “Whole brain emulation.”25

Figure 3 All stages of disease lifecycle can be supported by digital twins.

Figure 4 Human Digital Twins may be conceived on different levels. While the vision for body-level and mind-level implementation is there, current state of the art remains on the (sub) organ-level
An Unusual Opportunity Presents Itself
As Digital Twins offer strong potential as a technology, we must now ensure they comply with our ethical expectations. While there are plenty of players working on the development of this technology, mass rollout remains a vision for the future. This time window presents us with an opportunity to anticipate ethical issues.
There is very little literature and discourse on the ethical implications of digital twins in medicine — such as questions of what will be the new normal and potential enhancement issues have been discussed.Reference Bruynseels, de Sio and van den Hoven 26 We would like to start the much-needed discussion on potential issues arising with this article.
Framing the Debate — Related Technologies and their Ethical Issues Applicable to Digital Twins
Digital Twins consist of two distinct technologies: Mechanistic models and machine learning models. While mechanistic models may have their own limitations, they are fundamentally different from machine learning models in that they reflect an a priori explicit knowledge-based understanding of the world. On the contrary, machine learning models do not necessitate the conversion of data to information to explicit knowledge to inference — they may skip such steps normal in human-directed scientific discovery and application. The second characteristic of digital twins — the idea of enabling the execution of medical functions based on a person-specific model — leads us to refer to personalized medicine. Personalized — or precision — medicine refers to the execution of medical functions based on individual variability in environmental, genetic and lifestyle circumstances. One could see the digital twin as the perfect implementation of such an approach. It thus makes sense to briefly recapitulate ethical issues native to the underlying technologies before proceeding to more specific and evolving issues of digital twins in medicine. A third characteristic of the technology is its temporal live or near-live dimension which is not currently mirrored in the debate of a specific technology area.
Machine Learning
Machine learning models in medicine have two basic ingredients: data and an algorithm. With further operationalization, such models then lead to deployable digital health devices. The two ingredients, data and algorithm as well as their interactions determine a large part of the ethical challenges of such machine learning models. They are also affected by the choices of the human programmer. The further step from model to deployable device then induces further ethical challenges, such as user interface-dependent usability to different groups like the digitally illiterate. Multiple contributions exist as to which ethical principles machine learning models should adhere to.Reference Fjeld, Achten and Hilligoss 27 For a large-scale European effort including self-assessment tool, see the EU High-Level Expert Group on AI’s assessment list for trustworthy artificial intelligence (ALTAI). 28
The data component primarily drives questions of justice and fairness, non-maleficence and beneficence. The output of any model will be driven by the underlying data it draws on. If such data are not inclusive of racial groups, for example do not include people of color, the resulting performance will also be lower for such groups, potentially leading to inaccurate results, such as false negatives. The same issue will apply to the digital twin. The data component also drives concerns of privacy and solidarity. Data points that were previously considered anonymous can now — using the same underlying trends of big data, higher computing power and advanced algorithms — be de-anonymized.Reference Vokinger, Stekhoven and Krauthammer 29 This frontier will likely continue to be pushed in the future. Large data pools that are necessary as foundations for machine learning models therefore face the issue of a trade-off between an individual right to privacy and solidarity with others that could benefit from the use of data. Given these techniques, the level of privacy expected in the future is far from clear to the donor years away from its potential use.
The algorithm component primarily drives questions of transparency. Transparency on the highest level serves two purposes: 1) understanding how an algorithm learns from data and creates a model enabling verification that it conforms with all other ethical principles, incl. justice/fairness in the process. It also 2) enables explicability to users (HCPs and patients) of the ultimate device that is a pre-requisite of informed consent as part of autonomy.
On a model-level and then deployable device level there are questions of responsibility/accountability and agency. When a model or device is used for medical purposes, accountability (civil and criminal) is assigned on a sliding scale between the device manufacturer and the healthcare professional (and the patient). Liability is assigned based on a standard of diligence that typically relies on knowledge of how the device or drug works. Given the transparency concerns mentioned, this may not be the case and it is unclear at the moment — given lack of both specific legislation and court case history — how this assignment will be done, leaving patients and other actors at risk. Using data and ensuring its authenticity as well as comparability — such as on blood work results with lab- and test-specific reference ranges from many different sources will complicate this problem further. Accordingly, digital twins employing machine learning technologies will suffer from such ethical issues.
Personalized Medicine
Although the vision of precision medicine is that of the digital twin, its technological implementation to date is typically limited to genomic analysis and interpretation, such as identifying a mutation state at a single location within a patient’s genome. This single nucleotide polymorphism (SNP) approach has added value with some tests finding their way into medical practice. We believe that the technological foundations of the digital twin will help propel it towards the vision personalized medicine has failed to achieve so far.Reference Joyner and Paneth 30
The ethical issues with personalized medicine employing genomics technology, particularly in oncology,Reference Krzyszczyk, Acevedo and Davidoff 31 center around autonomy and privacy and have been subject to much debate and review.Reference Brothers and Rothstein 32 Beyond privacy and its tension with solidarity also applicable to ML, issues of consent to future use of data, of a “right to know” and a “right to not know” have a prominent place in the debate. Incidental findings, such as the results on further diseases not searched for initially but either during the original process or later on being identified, pose a day-to-day challenge to clinicians. Such issues would also be common in the digital twin — depending on the scope of implementation — with a potentially much more holistic/integrated picture of the human body driving up the quantity (and potentially impact) of incidental findings.
There are also issues of justice and fairness: personalized medicine, even relatively simple genetic testing, is expensive both in terms of material, equipment investments and personnel. These factors may not be available in all countries to all people, leading to the gap of healthcare delivery between people of different socioeconomic backgrounds widening. On the other hand, making more resources available has opportunity costs elsewhere. It is commonly discussed within personalized medicine that access (process fairness) and then healthcare outcomes (outcome fairness) are problematic. Previous efforts of longitudinal analysis combining genomic, transcriptomic, proteomic, metabolomic and autoantibody information undertaken on a single individual as an integrative personal omics profile (ipop) have demonstrated the costs of such an effort but provided limited value clinically.Reference Chen, Mias and Li-Pook-Than 33 Generally, we find the ethical challenges of the related technologies being applicable to digital twins.
Ethical Challenges Beyond the Underlying Technologies
In the following, key ethical challenges that are distinct from the ethical challenges of related technologies and unique in quality or degree to the digital twin concept are outlined. Transparency plays a key role in these challenges and is thus not listed as a separate section.
Fairness: Access to the Technology
The digital twin in the medical sphere — depending on level of implementation — will likely face very similar challenges in regard to access just as personalized medicine but to a much higher degree. Rather than only executing, analyzing and interpreting genomic tests looking for SNPs, they become one part in the input towards bigger models requiring computing power (equipment) and expert personnel (at specialist MD + Technology PhD level, not at a simpler lab technician and widely available generalist MD level). There is not only already a shortage of medical professionals but also one of IT professionals today. Given the increasing needs for workers with medical domain understanding of IT and vice versa, it is likely that the war for talent — fought with salaries and benefits — will increase the competition for such individuals benefiting resource-rich countries, potentially contributing to a widening of the gap in access. The same holds true within countries where different payors may have different reimbursement policies — if Medicare/Medicaid or European public payors decide to not pay for such services, this will inevitably accentuate different health outcomes based on ability to pay. So who will have access to digital twins and under which circumstances? May access be granted in the short-term only in experimental settings? And then a few decades from now, may this scenario be reversed with cheaper computing power and high-automation so that the default mode of healthcare will be automatic delivery by a digital twin-based setup and access to a flesh-and-blood HCP will require additional payment?
Responsibility: Complexity, Liability, and Ownership
The allocation of liability is an open question in regard to the underlying technologies. The digital twin pushes this in that the distinction between the sphere and responsibilities of the actors involved in medical decision-making will be blurred. It is no longer just a (lead) physician involving the patient in making a treatment decision based on data he or his team recorded. Given the many data sources, the physician will need to rely on data of the patient he is not qualified to interpret. Currently, digital twin models are limited to the organ-level which may lie within the expertise area of a specialty discipline, such as cardiology or nephrology. Once the digital twin moves beyond this towards an organ-organ interaction and systemic body-level, the degree of complexity gets amplified and there seems to be no human capacity to handle it, let alone for an individual physician to be held responsible. This leaves patients at-risk for not being able to seek legal redress. Already, inter-specialty and interprofessional delivery of care is a complex matter in today’s healthcare systems with issues resulting from gaps and overlaps of responsibility.Reference Schot, Tummers and Noordegraaf 34 In the existing medical device and pharmaceutical drug sphere, on-label use according to the approval of the device or drug transfers many of the liabilities to the manufacturer from the physician. Could a company devising a digital twin be held responsible and would it be classified as a “manufacturer” of a digital twin? Would it even own the patient-specific digital twin model? Without an approval process ensuring safety and efficacy of the “devices,” if the digital twin is classified as such, liability is an open question: Who will be in charge and held liable when digital twins enter medical practice? Will medical education and training need to change to enable its responsible use?
Autonomy and Non-Maleficence: False Sense of Control and Security, Risk of Manipulation
The digital twin concept — particularly on the body-level — would potentially convey a false sense of security to patients and healthcare professionals alike. With the promise of the reflection of our mechanistic knowledge of medicine as well as experience, users of the technology could easily fall prey to relying too much on this technology. The technical scope of digital twins will for the foreseeable future be limited: their use could lead to a false sense of security and over-reliance on digital twins in potentially missed diagnoses, etc. Given both the lack of transparency and such a false sense of security (constituting “controlling influences”), the autonomy of both patient and Healthcare Professional would be endangered. Autonomy in a decision in the medical context is achieved when a person makes a decision intentionally, with understanding and without controlling influences. All three criteria could be challenged by the digital twin. How would both patient and HCP challenge the recommendation of a digital twin? Would the HCP just become a follower uncapable of exercising meaningful autonomy? How would this affect the autonomy of the patient? At the same time, could a digital twin provide individualized decision-aids for the patient taking account his/her individual information processing abilities and preferences and thereby aid shared-decision making, an often unreached ideal?Reference Tan, Mazor and McDonald 35 But who would make the decision on whether to provide informational material vs. material intended to change behavior? Could our own digital twin manipulate us?
Privacy: Maximum Abuse Potential of Digital Twin Data and Model
Integrating so many sources of data and offering analysis and interpretation in the medical functions listed, including prognosis, has massive financial value to both legitimate and illegitimate stakeholders. Imagine a life insurance or health insurance company whose premium/payout ratio calculations would be heavily affected by such information. Or imagine an illegitimate hacker that would blackmail a patient with publishing their profiles containing delicate pieces of information. As recent as 2021, hackers stole psychotherapeutic patient files of provider vastaamo.fi in Finland and — after the healthcare providers refused payment — started blackmailing individual patients. 36 How would we protect the digital twins sufficiently? Are the risks worth the potential benefits for all or should this technology be limited in access to protect some populations? Human cloning is banned globally — shall we also ban the copying of minds through digital twins?
Outlook on the Future Practice of Medicine Using Digital Twins and Evolving Issues
The digital twin paradigm has the potential to change the healthcare approach within the entire human lifecycle: from pre-birth to post-death. In the following, we lay out four of many issue areas evolving with the upcoming use of digital twins in the practice of medicine.
The Digital Twin Will Change the HCP-Patient Relationship and Enable More Self-Care
The digital twin approach in medicine will likely manifest in two steps. First, Healthcare Providers will in the near-term be the primary users of digital twins of their patients. The digital twin will inform the HCP in making decisions along the medical functions described earlier. It is then on the HCP to deal with the patient utilizing relevant learnings. In this classical HCP-centric scenario of technology use, the main issue will be the potential lack of human control as the HCP may not be capable of exercising meaningful oversight.
In a second step, more long-term, the patient may be informed by and consult with the digital twin directly and thereby replace the HCP as the primary user for at least some more accessible use cases. In such a model of healthcare delivery, the flesh-and-blood HCP may serve only as an escalation step, if needed and/or wanted, depending on the preferences of the patient and the kind of disease or condition present. This would represent a direct shift on the self-care continuum enabling the patient to provide care for himself in a greater range of disease or conditions.
While such changes — in an ideal scenario — would lead to a patient-empowered, automatized healthcare delivery model, both path and outcome are plastered with risks. How could we enable patients to take charge of their healthcare and avoid a widening digital divide between users able to interact with their digital twins and tech interfaces and those that are not or only partially? Will those groups that are already vulnerable and left with lower access to care today be abandoned completely? And even in the intermediate step of digital twin use by HCPs, how will medical school curricula need to change in order to enable HCPs to critically use this new technology?
Beyond the ethical and societal challenges, there are interesting legal-regulatory questions evolving. What kind of prescription drugs could be prescribed by a digital twin-based model and who would be legally held accountable for it? Would there need to be a filter/screening function executed by pharmacists and therefore their role in healthcare delivery strengthened? Could a digital twin model be tricked by the patient into prescribing drugs of abuse or enhancement?
The Digital Twin May Become the Primary Treatment Object to an HCP — A Bit like a Tamagotchi
In the late-1990s, a wave of handheld digital pets swept through classrooms and homes around the World. Tamagotchis, a portmanteau between Japanese words tamago (egg) and uotchi (watch), were about tending to an alien animal undergoing life cycle stages from egg to adult and ultimate death where the user could have influence on the well-being of the animal through better care. It captured an entire generation with the IG Nobel Prize for Economics awarded to its inventors in 1997 for “diverting millions of person-hours of work into husbandry of virtual pets.” 37
Digital Twins may arouse similar captivity as tamagotchis once did. Healthcare Providers — being able to work directly with a near-perfect model of a patient — may be drawn to it in a way that they make it their primary treatment object. In such a scenario, HCPs may be satisfied by the gratification provided by a digital twin model — feedback of which could be much more immediate than that of the actual patient and the digital twin being much easier to work with than an actual patient. In a post-Covid-19 world where telemedicine, the remote provision of medical functions by clinicians via telecommunications technologies, takes a more normal role and in-office visits become fewer and technologies of augmented reality more prevalent, this scenario does not seem far-fetched. If an HCP never sees a patient in-person and chatbots, artificial voices and even deep fakes become more and more realistic, can he even differentiate between who is the real patient? Does this pace the way to dehumanization and its repercussions for dignity and beyond? The comparison to a tamagotchi may be extreme but already occurring shifts in the mode of healthcare delivery make this concern realistic.
The Digital Twin Will Influence its Physical Twin — But Maybe Not Always to the Better
In technology, various devices often interact in a hierarchical manner where one device (master) controls one or several other devices (slaves). Similarly, such a master database may also hold the single “one truth” and disseminate it to its slaves. This is a common concept also applied within databases or even atomic clocks. The digital twin (model) and its physical twin (patient) can also be allocated master and slave roles — the physical twin serves as the master from which the digital twin takes over the overriding truth of its physical and mental state.
However, the relationship between the two entities, physical twin and digital twin, is not unidirectional. Information flows in both directions: The digital twin feeds back information and recommendations influencing the behavior and state of the physical twin. This means the distinction between master and slave gets blurred and with it the power relationship of who controls who (or what controls who). May it be that the digital twin may influence the behavior of the physical twin in ways that are not always to the better or in the interest of the physical twin? May a digital twin become the target of a cybersecurity attack and hacking the digital twin may — to some level — control the behavior of the physical twin?
The Digital Twin May Challenge the Notion of the End-of-Life
The human lifecycle traditionally spans from conception via birth to death with embryonal and fetal development, childhood and adolescence, adulthood, golden years and geriatrics being phases in between those milestones. The concept of evidence-based medicine leaves little room for post-death after-life as the person (also legally) is tied the body. Bioethics deals with issues that arise during the traditional human lifecycle. Issues of afterlife, with the notable exception of the evolving debate on the digital afterlife in the context of social media and the internet use,Reference Öhman and Floridi 38 are barely the realm of bioethics. The digital twin technology challenges this and will require us to think past death of the body as the digital twin model of its physical twin may remain. Literature such as “Klara and the sun” — a recent dystopian science fiction novel by Nobel laureate Kazuo Ishiguro — has started to explore this theme.Reference Ishiguro 39 It will also challenge us by dividing the concept of congruence between the body and the person again. When we can have mind-level implementations of the digital twin, may some part of the consciousness of a person remain post-death of the body? How would we govern what happens with the digital twin past death of the physical twin? If a digital twin is so sophisticated and truly reflects a patient as a person, would the digital twin not also deserve some sort of rights? And if the rights of the digital twin are in conflict with the rights of the physical twin, will we need to weigh them against each other?
Conclusion: A Window of Opportunity
Digital Twin technologies offer strong performance potential in the wider area of healthcare and medicine specifically. Their potential power comes from the combination of underlying technologies machine learning and mechanistic models from biophysics. This directly mimics the human physician’s approach: applying both his mechanistic understanding of the human body and collected experience from seeing many patients throughout his training and practice. With more and more applications of the human digital twin being developed in the medical area and superiority of performance to existing approaches in some niches being established, this technology is now at the brink of early clinical adoption presenting a window of opportunity for the identification of ethical, legal and societal implications. This systematic technology-driven evaluation of ethical issues has identified key ethical concerns that digital twins share with their underlying technologies: fairness and justice, autonomy and privacy as common concerns of both underlying technologies as well as solidarity, non-maleficence/beneficence, responsibility and accountability and transparency stemming from machine learning technology. While it is not entirely surprising that a derivative technology shares the ethical concerns of its underlying technologies, we found that five ethical concerns get accentuated significantly: fairness and justice, autonomy, privacy, responsibility & accountability as well as transparency as a generally fundamental issue as it enables an understanding of all other ethical concerns. The field is rapidly evolving, and machines may soon assist medical doctors in moral decisions, such as whether to resuscitate when patient preferences are unknown.Reference Biller-Andorno and Biller 40 The digital twin will likely also incorporate or support similar functionalities.
On the other hand, there are non-classical issues as the practice of medicine may change using digital twins. Increased provision of self-care by patients while not having healthcare providers in the loop or their roles changed may lead to additional risks. Even when HCPs are in the loop, the digital twin may become their primary treatment object. Undue influence and conflicts of interest may arise between the digital twin and the physical twin. And the scope of questions for bioethics gets extended to afterlife and the pandora’s box of what constitutes a person gets opened.
Currently, any application of digital twin technologies to patients must be deemed non-standard with regulations on experimental procedures applying. This has important ramifications for HCPs utilizing digital twins: they are generally hit with an increased legal liability standard. In turn, adoption of digital twin technologies will be delayed. To our understanding, no digital twin-based medical device has been approved in any major jurisdiction yet. This can be good and bad: While desired benefits to patients and other stakeholders may not materialize as quickly, the window of opportunity for understanding and acting upon the identified ethical challenges will likely remain open for some more time. We should make use of this opportunity and come up with adequate regulation before it is too late.
On the other hand, there are non-classical issues as the practice of medicine may change using digital twins. Increased provision of self-care by patients while not having healthcare providers in the loop or their roles changed may lead to additional risks. Even when HCPs are in the loop, the digital twin may become their primary treatment object. Undue influence and conflicts of interest may arise between the digital twin and the physical twin. And the scope of questions for bioethics gets extended to afterlife and the pandora’s box of what constitutes a person gets opened.
Consider the case of Nayeon, a South Korean girl who died of leukemia in a fast succession from diagnosis to death within Summer to Fall 2017. From her voice recording, video and photo material as well as other children’s movement recordings, a limited virtual reality simulation was put together allowing her mother to communicate with her deceased child. The interaction was filmed and broadcast by Korean TV. 41 The mother described the process as helping her deal with the pain of loss particularly by allowing her to say what she felt she had not during the fast procession of events earlier. The technology from this real-life example is not yet at the digital twin level, as the daughter barely answered any of the questions by the mother. But it opens a window into the many complexities that we as bioethicists need to deal with. It’s time we start thinking about the challenges that may otherwise overwhelm us as the technology develops rapidly.
Note
The authors have no conflicts to disclose.