Introduction
The modulation of deep-brain regions is a promising treatment option for various mental disorders. One such disorder is borderline personality disorder (BPD), which is characterized by pervasive emotion dysregulation related to aberrations in the amygdala and in prefrontal-limbic networks (Schulze, Schmahl, & Niedtfeld, Reference Schulze, Schmahl and Niedtfeld2016; Sicorello & Schmahl, Reference Sicorello and Schmahl2021). To date, little evidence exists whether those with BPD can benefit from neurofeedback (NF) (Howard, Schellhorn, & Lumsden, Reference Howard, Schellhorn and Lumsden2013; Paret et al., Reference Paret, Kluetsch, Zaehringer, Ruf, Demirakca, Bohus and Schmahl2016; Zaehringer et al., Reference Zaehringer, Ende, Santangelo, Kleindienst, Ruf, Bertsch and Paret2019). In principle, NF allows individuals to self-modulate their brain activation: via a brain–computer interface, patients observe and control their brain activation in real time (Paret & Hendler, Reference Paret and Hendler2020). The amygdala lends itself as a target for NF in patients with BPD. However, generating precise feedback from deep-brain regions, such as the amygdala, requires cost-intensive brain scanning with functional magnetic resonance imaging (fMRI) (Paret et al., Reference Paret, Goldway, Zich, Keynan, Hendler, Linden and Cohen Kadosh2019). The limited availability of MR machines, the cost of using them, and the aversion of many patients against lengthy MRI measurements represent considerable barriers for amygdala-NF studies. To overcome these disadvantages of fMRI, an electrocortical surrogate of deep-brain activation was developed: amygdala-related electrical fingerprint (Amyg-EFP) (Meir-Hasson et al., Reference Meir-Hasson, Keynan, Kinreich, Jackont, Cohen, Podlipsky-Klovatch and Intrator2016). The Amyg-EFP can be used as a proxy for deep-brain activation, thereby allowing for amygdala-NF training outside of an MR machine. Previously, Amyg-EFP NF was found to be effective in ameliorating symptoms of post-traumatic stress (Fruchtman-Steinbok et al., Reference Fruchtman-Steinbok, Keynan, Cohen, Jaljuli, Mermelstein, Drori and Hendler2021; Keynan et al., Reference Keynan, Cohen, Jackont, Green, Goldway, Davidov and Hendler2019). The present work had two main goals: (1) to assess whether the Amyg-EFP can be used to probe deep-brain activation in BPD, and (2) to investigate the feasibility of Amyg-EFP NF training with patients who are undergoing a residential dialectical behavior therapy (DBT) program, a standard treatment for BPD (Bohus et al., Reference Bohus, Stoffers-Winterling, Sharp, Krause-Utz, Schmahl and Lieb2021).
Measuring subcortical brain activity via electroencephalography (EEG) is an intricate problem, in particular when it comes to deep-brain structures. To overcome the limited anatomical specificity of EEG, the Amyg-EFP has been developed based on simultaneously acquired fMRI and EEG. Machine learning was used to predict the amygdala blood oxygenation level dependent (BOLD) signal from time and frequency information from bandwidths recorded with three scalp EEG-electrodes: ground, reference, and one more electrode (Fig. 1). The resulting signal is an EEG surrogate of BOLD activation, optimized for the amygdala. The Amyg-EFP was validated in an independent sample to prove its usefulness as a generic feedback signal, that is patients do not require an individual EEG–fMRI session, as the Amyg-EFP algorithm is expected to reliably correlate with the amygdala BOLD signal across participants (Meir-Hasson et al., Reference Meir-Hasson, Keynan, Kinreich, Jackont, Cohen, Podlipsky-Klovatch and Intrator2016). Feedback based on this signal is supposed to facilitate NF training to regulate amygdala activation. In order to transfer this technology to different labs and clinical centers, and to introduce Amyg-EFP to the treatment of a different clinical population, we aimed to investigate whether previous results were replicable and whether they would generalize to BPD. We performed two studies: for study 1, we recorded simultaneous EEG–fMRI data from patients with BPD to test whether (a) the Amyg-EFP would predict amygdala activation, and (b) whether the fMRI-BOLD pattern prediction would be consistent with benchmark findings from Keynan et al. (Reference Keynan, Meir-Hasson, Gilam, Cohen, Jackont, Kinreich and Hendler2016), i.e. whether the results would replicate and generalize to BPD. Study 2 assessed the feasibility of Amyg-EFP NF training within the context of residential DBT.

Figure 1. Amyg-EFP prediction model. (a) EEG data are multiplied by the common model coefficient matrix (b) to produce the predictor of amygdala BOLD activity (c). ‘(a) The EEG data used for the model are a time/frequency matrix recorded from electrode Pz including all frequency bands in a time window of 12 s. (b) The common model coefficients matrix. [CH] × [FQ] × [Delay] × [Time]. fMRI-BOLD activity at time T can be predicted by the EEG using the frequency intensity FQ of electrode CH in delay D from T. In our case, CH includes a selected single electrode (Pz). (c) The predicted right amygdala BOLD activity time course’ (Keynan et al., Reference Keynan, Meir-Hasson, Gilam, Cohen, Jackont, Kinreich and Hendler2016, S. 491). Figure reproduced with permission from Keynan et al. (Reference Keynan, Meir-Hasson, Gilam, Cohen, Jackont, Kinreich and Hendler2016).
We had two hypotheses: one, that the Amyg-EFP signal would predict amygdala-BOLD activation, and two, that the effect sizes from previous research could be replicated with a new dataset. Specifically, we hypothesized that the whole-brain pattern of effect sizes from a ‘reference dataset’ would not be different from the pattern observed in the BPD dataset. Additionally, we explored whether patients improve regulation of the Amyg-EFP with NF training. Lastly, we assessed the feasibility of NF in the context of residential DBT.
Methods and materials
Replication of Amyg-EFP-related brain pattern in BPD
We analyzed a new dataset recorded in a BPD sample (the ‘replication dataset’) and compared it to a ‘reference dataset’ (Keynan et al., Reference Keynan, Meir-Hasson, Gilam, Cohen, Jackont, Kinreich and Hendler2016) to assess two facets of replicability: (1) replication of significant amygdala activation and (2) replication of effect sizes.
Description of the reference dataset
Twenty-four healthy participants (age [mean/s.d.]: 26.75/3.81 years, eight females) underwent a simultaneous EEG–fMRI measurement, which was the last session of a multi-session experiment. The study also included four EEG-only NF sessions and a pre-fMRI assessment, which were not analyzed for this paper. Participants completed five functional runs (baseline, NF runs 1–4) and one anatomical brain scan. The experimental group (N = 17) received continuous feedback from the Amyg-EFP and the control group (N = 7) from the alpha–theta ratio. More details can be found in the original publication (Keynan et al., Reference Keynan, Meir-Hasson, Gilam, Cohen, Jackont, Kinreich and Hendler2016). During NF, participants heard a piano melody that became louder when Amyg-EFP activation increased. In each of the four NF runs, they had to lower the volume of the melody by exercising mental strategies. ‘Instructions were intentionally unspecific, allowing individuals to adopt the mental strategy that they subjectively found most efficient’ (Keynan et al., Reference Keynan, Meir-Hasson, Gilam, Cohen, Jackont, Kinreich and Hendler2016, S. 491). NF blocks (60 s) alternated with rest (60 s) and finger-tapping blocks (30 s).
EEG data acquisition: EEG was acquired with an MR-compatible BrainAmp-MR amplifier (BrainProducts, Munich, Germany) and BrainCap electrode cap with sintered silver/silver chloride (Ag/AgCl) ring electrodes (30 channels, 1 ECG channel, 1 electro-oculogram [EOG] channel; Falk Minow Services, Herrsching-Breitbrunn, Germany). Electrodes were positioned to 10/20 system with the reference electrode between FCz and Cz. The raw EEG was sampled at 250 Hz and recorded using Brain Vision Recorder software (Brain Products).
Online calculation of Amyg-EFP amplitude: The Amyg-EFP signal was calculated online from raw EEG data using a built-in automated average artifact subtraction method implemented in BrainVision RecView (BrainProducts). RecView was custom modified to enable export of the corrected EEG data in real time through a TCP/IP socket. Preprocessing algorithm and EFP calculation models were compiled from MATLAB R2009b to Microsoft.NET in order to execute it within the BrainVision RecView EEG Recorder system. Data were then marshaled to a MATLAB.NET compiled dll that calculated the value of the EFP amplitude every 3 s. The online generated EFP data were used for analyses.
fMRI data acquisition: Structural and functional scans were performed using a GE 3T Signa Excite echo speed scanner with an eight-channel head coil, and a resonant gradient echoplanar imaging system. The scanner was located at the Wohl Institute for Advanced Imaging at the Tel-Aviv Sourasky Medical Center. A T1-weighted 3D axial spoiled gradient echo pulse sequence (TR/TE = 7.92/2.98 ms, flip angle = 15°, pixel size = 1 mm, FOV = 256 × 256 mm2, slice thickness = 1 mm) was applied to provide high-resolution structural images. Functional whole-brain scans were performed in an interleaved top-to-bottom order, using a T2*-weighted gradient echo planar imaging pulse sequence (TR/TE = 3000/35 ms, flip angle = 90°, pixel size = 1.56 mm, FOV = 200 × 200 mm2, slice thickness = 3 mm, 39 slices per volume).
fMRI preprocessing and analysis: fMRI data were imported to the brain imaging data structure (BIDS) (Gorgolewski et al., Reference Gorgolewski, Auer, Calhoun, Craddock, Das, Duff and Poldrack2016), using adapted code-scripts based on the Rapid, automated BIDS conversion (RaBIDS) pipeline (Paret, Reference Paret2023b), and preprocessed with fMRIPrep v20.0.6 (Esteban et al., Reference Esteban, Markiewicz, Blair, Moodie, Isik, Erramuzpe and Gorgolewski2019; see online Supplement). SPM12 v7771 (The Wellcome Centre for Human Neuroimaging, London, UK) was used for first-level analysis. The initial four volumes were discarded to allow longitudinal magnetization to reach equilibrium. The general linear model (GLM) for the first-level analysis contained seven orthogonalized predictors: the Amyg-EFP time-course, i.e. the effect of interest, and the six realignment regressors for nuisance regression. The Amyg-EFP time-course was not convolved with the hemodynamic response function. No high-pass filter was applied to the data. SPM's autoregression AR(1) model was applied. Data quality was assessed based on fMRIPrep's html-output files. To exclude spurious effects, data coinciding with large movements (framewise displacement [FD]>4 mm) was excluded from the analysis (either full runs, or initial/final volumes if movements happened in the beginning/end of the scan). This affected three runs in total.
Replication in sample with BPD
Patients were eligible to participate in this study during the first half of the residential DBT-program (i.e. 6 weeks) if they were female, fulfilled four or more Diagnostic and Statistical Manual of Mental Disorders-IV BPD criteria as determined by a trained clinician, and were aged 18–25 years. They were excluded in case of pharmacotherapy with benzodiazepines, pregnancy, epilepsy, traumatic brain injury, brain tumor, or otherwise severe neurological or medical history, body mass index >16.5, and if they fulfilled the usual MRI exclusion criteria. Participants had to be abstinent from illicit drugs and alcohol. The resulting replication sample consisted of N = 16 participants (21.3/2.19 years, see online Supplementary Table S1). Eleven participants from this sample had participated in study 2, too, and received their EEG–MR-scan following Amyg-EFP NF training. Five participants did only receive the EEG–MR-scan.
The simultaneous EEG–MR-scan was composed of three runs: a resting-state scan (6 min), a short NF run (6 min), and a long NF run (22 min), during which individuals received continuous fMRI-NF. Participants were instructed to downregulate (short NF run) a visual analogue scale illustrating brain activity or to up- and downregulate brain activity in alternating blocks (long NF run, online Supplementary Fig. S1) using mental strategies. Instructions were intentionally unspecified, allowing participants to find their own mental strategy.
EEG data acquisition: The EEG was recorded during image acquisition inside the scanner using an MRI-compatible EEG system with a 5 kHz sampling rate, 32 mV input range and 0.1–250 Hz band-pass filters. The signal was recorded by equidistantly spaced sintered Ag/AgCl scalp electrodes using EEG caps with twisted and fixed electrode cables (64Ch BrainCap-MR with Multitrodes; Easycap, Munich, Germany). The 64-channel EEG montage included most 10–10 system positions. Fz served as recording reference, and AFz as the ground electrode. Four additional electrodes were placed to record the EOG and the ECG. The signal was transmitted from two MRI-compatible amplifiers (BrainAmp MR, BrainProducts, Gilching, Germany) outside the scanner via optic fibers. Electrode impedances were kept below 20 kΩ, except for ECG and EOG electrodes (<30 kΩ) as well as reference and ground (<10 kV). The quality of the EEG was assessed during the MR-scan, using online correction software (RecView BrainProducts, Gilching, Germany).
fMRI data acquisition: Structural and functional scans were performed using a 3 Tesla MRI Scanner (Trio, Siemens Medical Solutions, Erlangen, Germany) with a 20-channel head coil. After the first eight study subjects the MR-scanner received an upgrade (PRISMAfit, Siemens Medical Solutions, Erlangen, Germany) with which all remaining subjects of the study were scanned. Functional images of the BOLD contrast were acquired with a gradient echo T2*-weighted echo-planar-imaging sequence (TE = 30 ms, TR = 2 s, FOV: 192 × 192 mm2, flip angle = 80°, in-plane resolution = 3 × 3 mm2). One volume comprised 36 slices tilted −20° from the AC–PC (anterior and posterior commissures) orientation with a thickness of 3 mm and a slice gap of 1 mm. Participants had their heads lightly restrained in the coil using soft pads. The resting-state scan comprised 180 volumes each, while the experimental runs for the 6 min down-regulation NF were 186 volumes each and the 22 min up–down-regulation NF 666 volumes each. T1-weighted anatomical images were acquired with a magnetization prepared rapid acquisition gradient echo sequence (TE = 3.03 ms, TR = 2.3 s, 192 slices, and FOV = 256 × 256 mm2).
fMRI preprocessing and analysis: Preprocessing and analysis steps were identical with the analysis of the reference dataset. Heavily movement-affected volumes were repaired, using the ArtRepair toolbox (https://cibsr.stanford.edu/tools/human-brain-project/artrepair-software.html). After re-estimating the SPM model using the repaired volumes, the improvement between the repaired and the original model was assessed based on global quality estimates (whole-brain contrast-to-noise ratio). The re-estimated SPM model from four subjects was used for further analysis, as quality improved >5% relative to the original SPM model.
Replication of effect sizes: Following Gerchen et al. (Reference Gerchen, Kirsch and Feld2021), we tested whether the effect sizes found in Keynan et al.'s (Reference Keynan, Meir-Hasson, Gilam, Cohen, Jackont, Kinreich and Hendler2016) ‘reference sample’ would fall into the 90% confidence interval (CI) of our ‘replication sample’. We assessed only positive effects (i.e. Hedge's g > 0), resulting in a one-sided significance threshold for replication of p < 0.05. The statistical analysis was preregistered before results were known (https://doi.org/10.17605/OSF.IO/KYCR6).
Mapping Amyg-EFP signal to neurocircuitries
We analyzed correlations of the Amyg-EFP signal with BOLD signal time courses from five neurocircuitries, which were defined according to masks provided by Goldstein-Piekarski et al. (Reference Goldstein-Piekarski, Ball, Samara, Staveland, Keller, Fleming and Williams2022): negative affect neurocircuitry, salience neurocircuitry, default-mode network (DMN), cognitive control neurocircuitry, and positive affect neurocircuitry. Additionally, we analyzed correlations with the BOLD signal time course from sensory-motor domains. Visual cortex was defined as area hOc1, auditory cortex as area TE 1, and motor cortex as areas 4a and 4p (Eickhoff et al., Reference Eickhoff, Stephan, Mohlberg, Grefkes, Fink, Amunts and Zilles2005). We used SPM's VOI tool to extract the eigenvariate from each region, which was then adjusted for the effect of interest, i.e. the F-contrast received from the Amyg-EFP predictor.
Feasibility of NF training
The study design and results are reported according to best-practice guidelines and we provide the CRED-nf checklist (Ros et al., Reference Ros, Enriquez-Geppert, Zotev, Young, Wood, Whitfield-Gabrieli and Thibault2020) in the online Supplement.
Sample
Twenty-nine female patients diagnosed with BPD were allocated to the NF group. Fifteen of them completed the study, receiving the full dose of 10 Amyg-EFP NF sessions over 5 weeks in addition to their residential DBT treatment. Twenty-two female patients diagnosed with BPD were assigned to a control group, receiving no NF training in addition to DBT treatment (no-NF group). Fifteen of them completed the study. Eligibility criteria were the same as reported above. The NF group (N = 15, age: 21.4/1.84 years) did not differ in age from the no-NF group (N = 15, 20.7/1.98; T(27.86) = −1.046, p = 0.305). The two groups did not differ in clinical characteristics such as psychopathology (BSL-23 [Wolf et al., Reference Wolf, Limberger, Kleindienst, Stieglitz, Domsalla, Philipsen and Bohus2009]), comorbidities or psychotropic medication (Table 1). No differences were observed between the groups in baseline depression (Beck's Depression Inventory [BDI-II] [Steer, Clark, Beck, & Ranieri, Reference Steer, Clark, Beck and Ranieri1999]), anxiety (State-Trait Anxiety Inventory [STAI] [Laux, Glanzmann, Schaffner, & Spielberger, Reference Laux, Glanzmann, Schaffner and Spielberger1981]), affective lability scale (ALS [Harvey, Greenberg, & Serper, Reference Harvey, Greenberg and Serper1989]; https://www.zotero.org/google-docs/?qqWhxh), and alexithymia scores (Toronto alexithymia scale [TAS-26] [Taylor, Ryan, & Bagby, Reference Taylor, Ryan and Bagby1985], Tables 1 and 2). Completers v. non-completers were compared regarding their age, psychopathology, comorbidities, and psychotropic medication (online Supplementary Tables S2 and S3). Non-completers were younger than completers (completers: 21.0/1.9 years, non-completers: 20.0/1.6 years, T(47) = −2.03, p = 0.048) and were less likely to be diagnosed with an eating disorder (completers: 14, non-completers: 4, U = 228, p = 0.046). Statistical trends (p < 0.10) were observed for higher comorbidity of anxiety disorders in the non-completer group and higher proportion of ‘other comorbidities’ in the completer group. Comparisons of completers and non-completers were not planned a priori and p values were not corrected for multiple comparisons. Online Supplementary Fig. S2 presents a comprehensive patient flow chart.
Table 1. Study 2 sample characteristics: demographics and psychiatric characteristics
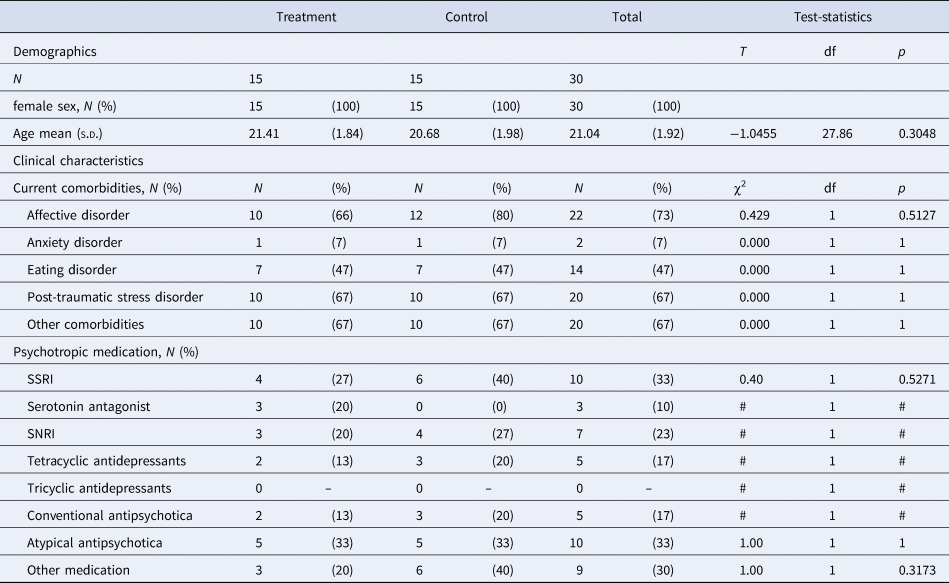
s.d., standard deviation; SSRI, selective serotonin reuptake inhibitor; SNRI, serotonin–norepinephrine reuptake inhibitor; #, frequencies too small for test.
†p < 0.10; *p < 0.05; **p < 0.01.
Table 2. Study 2 sample characteristics: clinical psychological characteristics
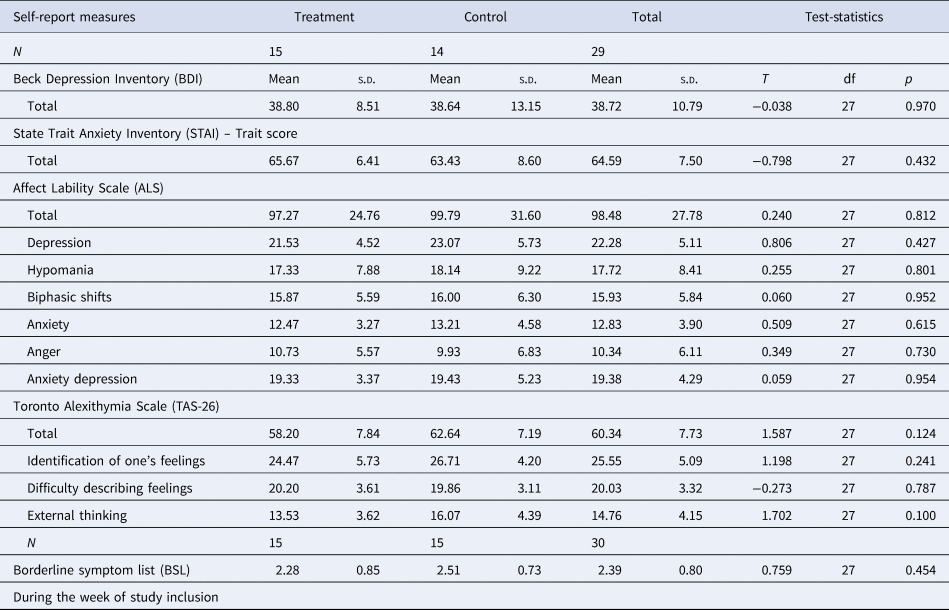
s.d., standard deviation.
†p < 0.10; *p < 0.05; **p < 0.01.
General procedure
Participants for this study were recruited in the inpatient units of the Department of Psychosomatic Medicine and Psychotherapy, Central Institute of Mental Health, between May 2018 and February 2021. We approached patients during their first 3 weeks of the 12-weeks DBT program. Participants of the control arm were recruited after completion of the NF group. Questionnaires and an MR scan were completed at the beginning of the study (pre-measurement), followed by 10 EEG-NF-trainings over the course of 5 weeks for the NF group. After 5 weeks, the questionnaires and MR scan were completed again by both groups (post-measurement).
The authors assert that all procedures contributing to this work comply with the ethical standards of the relevant national and institutional committees on human experimentation and with the Helsinki Declaration of 1975, as revised in 2008. The experiments were conducted at the CIMH in Mannheim, Germany. All participants provided informed written consent before participation and received no reimbursement for participation. Two of five subjects who were recruited for the simultaneous EEG–fMRI scan only received 50€ for participation.
EEG acquisition for NF training
EEG was recorded with three electrodes: the ground (AFz), reference (FCz), and active electrode (Pz) were mounted according to the 10–10 system using a standardized cap (Easycap, Herrsching, Germany). The EEG signal was recorded with BrainAmp ExG-amplifier (BrainProducts, Gilching, Germany) with a sampling rate of 250 Hz and the following filters: low-cutoff = 3 Hz, high-cutoff = 70 Hz and no notch filter. Electrode impedances were kept below 5 kΩ. The software used was BrainVision Recorder (BrainProducts, Gilching, Germany).
Feedback protocol
Participants were sitting with eyes open in a relaxed position in front of a black computer screen. A piano melody of 3 s was repeatedly played to participants (Kinreich et al., Reference Kinreich, Podlipsky, Jamshy, Intrator and Hendler2014). Participants were instructed to downregulate the volume. The study was designed to assess the effects of Amyg-EFP downregulation and was preregistered accordingly (online preregistration: https://doi.org/10.17605/OSF.IO/6ZDS5, clinicaltrials.org: NCT03964545). Due to a programming error that was revealed after the completion of data acquisition, the audio volume was inversely coupled with Amyg-EFP activation. That is, the piano volume decreased when patients upregulated Amyg-EFP.
Each of the 10 training sessions lasted 20 min and consisted of five cycles with a duration of 4 min each. Every cycle was composed of a baseline block of 1 min followed by a feedback block of 3 min. The audio volume was adjusted to the measured Amyg-EFP signal in feedback blocks and was fixed at 70% of the maximum volume in baseline blocks. Participants reached the minimum/maximum volume when the Amyg-EFP signal was <−2 s.d./>2 s.d. from the preceding baseline mean (baseline values of the initial 6 s were dropped).
Clinical self-report outcomes
Self-report and training success data were analyzed using R software version 4.2.2. Clinical questionnaires were assessed 2–7 days before the first measurement to ensure matching of groups at baseline (‘pre’). Clinical outcomes (BDI, ALS, TAS-26) were assessed again 2–7 days after the last NF session (‘post’), or in case of the no-NF group, 5 weeks after the pre-measurement. Extreme values were defined as values x < Q (quartile) 1–3 × IQR (interquartile range) or x > Q3 + 3 × IQR according to the rstatix package (Kassambara, Reference Kassambara2023b) and were excluded from analysis. The afex package was used to analyze mixed analyses of variance (Singmann et al., Reference Singmann, Bolker, Westfall, Aust, Ben-Shachar, Højsgaard and Christensen2023).
NF training success
Training success was quantified as the personal effect size (PES) (Paret et al., Reference Paret, Goldway, Zich, Keynan, Hendler, Linden and Cohen Kadosh2019). PES measures the change of the Amyg-EFP signal from a NF block (3 min; 60 samples) relative to the preceding baseline block (1 min; 20 samples) divided by the pooled standard deviation. PES values of each block were averaged and analyzed with multilevel regression analysis using the lme4 package (Bates, Mächler, Bolker, & Walker, Reference Bates, Mächler, Bolker and Walker2015). The model reflected the nested data structure of blocks within sessions and sessions within participants, and included a Subject random effect. To analyze the linear effect of session progression, capturing the incremental learning effect across training sessions, ‘Session’ was included as a random effect. The random effect for the ‘Subject × Session’ interaction was included. The fixed effect ‘Session’ was assessed for significance. Session was centered on the first run (i.e. x centered = x − 1). Data were assessed for heteroskedasticity via visual inspection of quantile–quantile plots.
Correlation of self-report with NF success
The final two sessions and the initial two sessions were averaged and the difference was calculated. Correlations were calculated with the change in clinical measures (i.e. post minus pre). The R package ggpubr was used to assess explained variance R and significance p of Pearson correlation (Kassambara, Reference Kassambara2023a).
Availability of materials
Questionnaire data and individual fMRI and EEG data are available on reasonable request and can be shared online with applicable data protection regulations. Analysis code of self-report and NF training data is openly available (Paret, Reference Paret2023a). The T-map from the second-level fMRI analysis (i.e. aggregated data across subjects) is available on neurovault (https://neurovault.org/collections/JBICXOQC/).
Results
EEG-informed fMRI analysis
Amyg-EFP-related brain-BOLD activation
Cluster-based control of type-I error resulted in five significant voxel clusters, with one cluster including the right amygdala (amygdala-voxel with highest activation at [30, 4, −19], MNI coordinates, T(15) = 4.02). In line with a priori expectations, we found that the correlation between Amyg-EFP and the effect size of fMRI-BOLD activation was replicated in large parts of the brain, including the amygdala (Fig. 2).
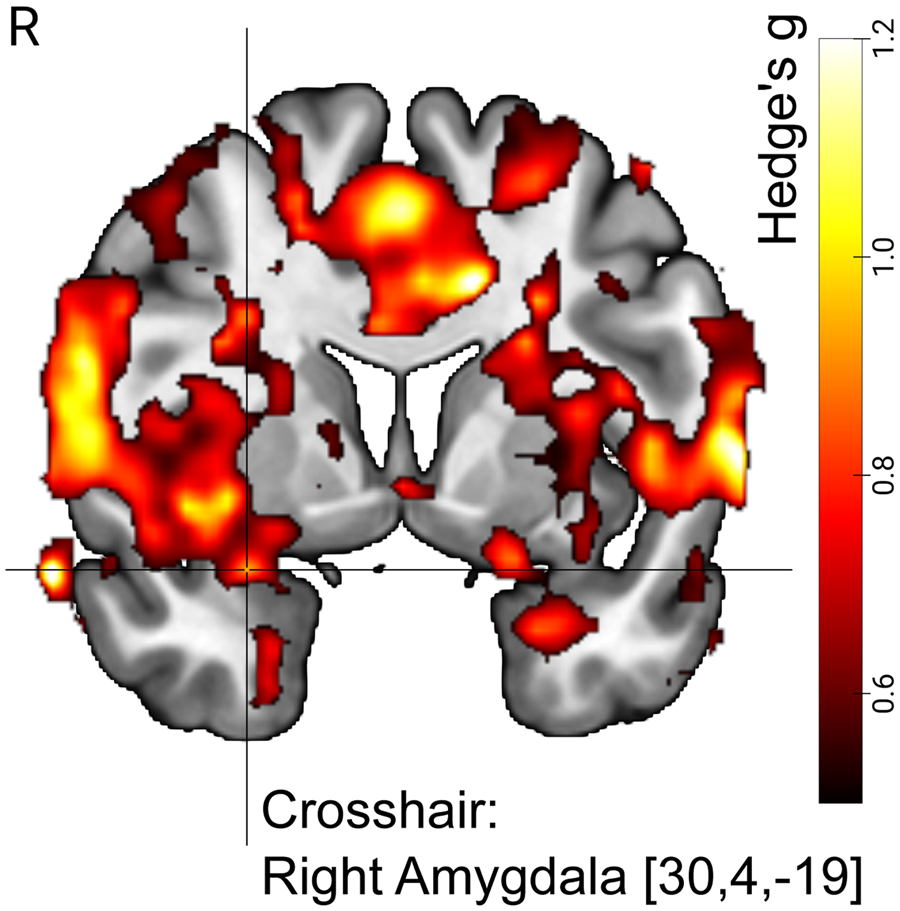
Figure 2. Amyg-EFP signal predicted right amygdala BOLD activation in N = 16 individuals with BPD undergoing simultaneous fMRI–EEG measurements. Visualization shows map of effect sizes (Hedge's g). The BPD dataset served as the ‘replication sample’ in the analysis to replicate previous findings from Keynan et al. (Reference Keynan, Meir-Hasson, Gilam, Cohen, Jackont, Kinreich and Hendler2016), i.e. the ‘reference sample’. Voxels shown are limited to those voxels with effect sizes that were within the 90% CI of the reference sample. With other words, the image illustrates replicated effects. The visualization is further limited to voxels with medium effect size or higher (Hedge's g > 0.5). Crosshair position (MNI coordinates) indicates the amygdala region that was part of a significant cluster with size k = 129 570 voxels. For significance testing we used cluster correction for multiple comparisons (p < 0.05, FWE (family wise error), k > 149) with a cluster-defining threshold of p < 0.001 (T(15) > 3.728). R, right.
Exploring neurocircuitry engagement
In order to investigate the involvement of different neurocircuitries in the generation of the Amyg-EFP signal, we calculated correlation coefficients of the Amyg-EFP with different brain regions. The results show that the Amyg-EFP is positively correlated with regions of the negative affect neurocircuitry, the salience neurocircuitry, and the cognitive control neurocircuitry. This finding was driven by significant correlation of Amyg-EFP with the BOLD-activation of bilateral amygdala, dorsal anterior cingulate cortex (dACC) and left dorsolateral prefrontal cortex (dlPFC, Fig. 3). Note that some regions (including amygdala) are part of more than one circuitry. No significant correlations were observed with the DMN and the positive affect neurocircuitry. Correlations with visual, auditory, and motor areas were significant and, descriptively, larger in comparison to the other networks. Due to the explorative nature of this analysis, we did not adjust significance thresholds to control for type-I error.

Figure 3. Correlations of Amyg-EFP with brain regions from different neurocircuitries (N = 16). Mean Fisher-z transformed Pearson correlation is shown with 95% CI. Correlations can be said to be significant when the 95% CI does not overlap with 0. Note that size of CIs was not corrected for multiple comparisons, limiting the utility of significance thresholds. L, left; R, right; Amy, Amygdala; AI, anterior insula; ACC, anterior cingulate cortex; dACC, dorsal ACC; pgACC, perigenual ACC; sgACC, subgenual ACC; AG, angular gyrus; PCC, posterior cingulate cortex; PFC, prefrontal cortex; amPFC, anterior medial PFC; dlPFC, dorsolateral PFC; vS, ventral striatum; vmPFC, ventromedial PFC.
Feasibility of Amyg-EFP training
High patient drop-out due to early discharge from residential DBT program
Out of 51 patients (29 [number in brackets: NF-group participants]) entering the trial, 31 (15) completed the study until the post-measurement. This corresponds to a drop-out rate of 39% (48%). Twelve (8) patients dropped out, because they left the residential DBT program early. When drop-outs due to early DBT termination are discounted, the drop-out rate is reduced to 16% (21%) (see online Supplementary Fig. S1).
Patients improved regulation of the Amyg-EFP signal
Fifteen participants completed the training regimen and entered the analysis. The analysis of Amyg-EFP NF training success resulted in a significant Session fixed effect (T(14.172) = 2.375, p < 0.05) and evidenced a linear increase of the Amyg-EFP as indicated by the PES measure across training sessions. Note that participants practiced up-regulating rather than down-regulating the Amyg-EFP signal due to a programming error in NF software. The auditory feedback was inversely coupled to their Amyg-EFP signal, decreasing volume as their Amyg-EFP signal increased. Therefore, it can be concluded that they improved to regulate brain activation in the direction that they were trained to (Fig. 4a). The Subject intercept was significant (T(14.537) = 3.624, p < 0.005). Model fit was assessed with conditional R 2GLMM = 0.3545 (i.e. variance explained by the entire model) and marginal R 2GLMM = 0.042 (i.e. variance explained by fixed effects). The analysis was repeated with the feedback values, i.e. the volume of the auditory interface, which is given as a number between 0 = minimal and 1 = maximal amplitude. Results of this analysis were consistent with the results from the previous analysis (Session fixed effect: T(14.150) = 2.323, p < 0.05, Subject intercept: T(14.955) = 45.872, p < 0.001; conditional R 2GLMM = 0.249, marginal R 2GLMM = 0.033) and showed that subjects downregulated the auditory feedback in line with instructions (Fig. 4b).
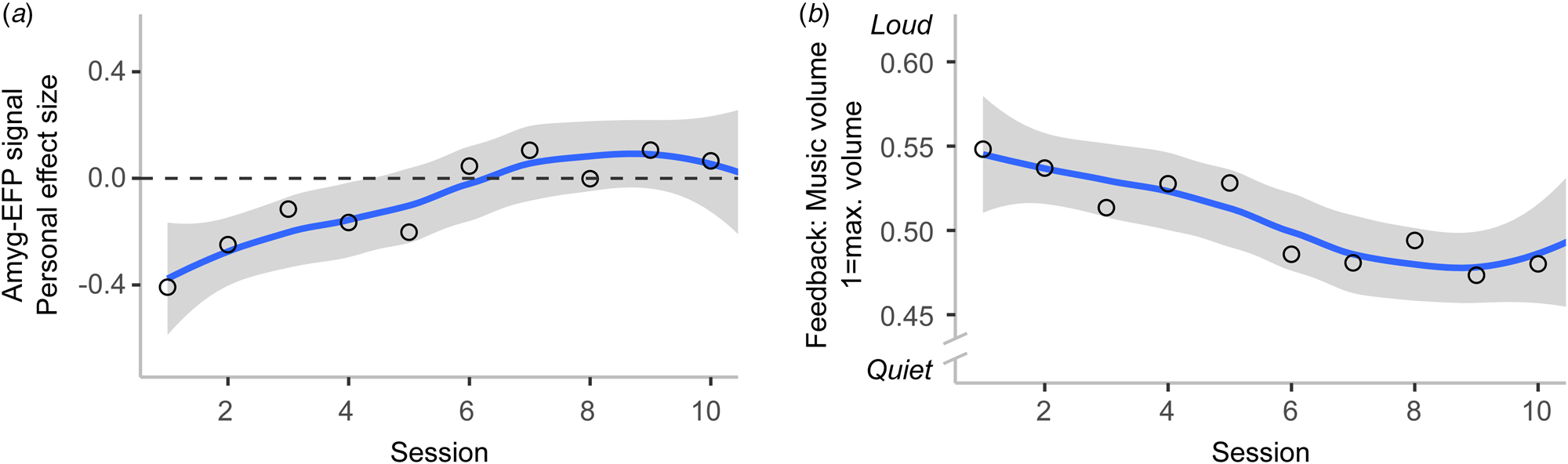
Figure 4. Participants learned to regulate the Amyg-EFP with NF training (N = 15). Regression line with standard error is shown. Circles indicate session mean. (a) Participants increased the Amyg-EFP signal across training sessions, which was reflected in increasingly higher values of the success measure. (b) In line with the instructions given to participants, they learned to downregulate the music volume of the auditory brain–computer interface across training sessions. As the Amyg-EFP signal was inversely coupled with auditory feedback due to a programming error in the NF software (i.e. the higher the brain signal, the quieter the music), lower music volume means greater regulation success.
Discussion
This study applied the Amyg-EFP, an EEG model of fMRI-defined amygdala activity, to BPD patients in order to test its predictive reliability and the feasibility of NF training in this cohort. Significance testing as well as formal replication analysis of whole-brain effect sizes confirmed correlation of the Amyg-EFP with deep-brain fMRI-BOLD activation as hypothesized. These findings demonstrate that the Amyg-EFP is a generic EEG-based model of fMRI-BOLD activation that generalizes to clinical populations. Comparing effect sizes between two datasets, as performed in this analysis, enables replication analysis without the decision to exclude voxels based on conventional and somewhat arbitrary significance thresholds. Namely, the comparison of effect sizes can be regarded as a quantitative evaluation of replication success, as opposed to the visual, hence qualitative, evaluation of two significance maps from two independent analyses. Next, we assessed the feasibility of Amyg-EFP NF as an additional training during a 12 weeks residential DBT program. Patients were able to improve regulation of the Amyg-EFP across 10 sessions. This finding aligns with previous research, showing that subjects can learn to modulate the Amyg-EFP with NF (Fruchtman-Steinbok et al., Reference Fruchtman-Steinbok, Keynan, Cohen, Jaljuli, Mermelstein, Drori and Hendler2021; Goldway et al., Reference Goldway, Ablin, Lubin, Zamir, Keynan, Or-Borichev and Hendler2019; Keynan et al., Reference Keynan, Cohen, Jackont, Green, Goldway, Davidov and Hendler2019, Reference Keynan, Meir-Hasson, Gilam, Cohen, Jackont, Kinreich and Hendler2016). However, it is not possible to conclude from the present study that NF-training causally improved Amyg-EFP regulation, as we did not compare brain self-regulation after v. before NF-training with an active control group.
A qualitative review of patient drop-out evidenced high drop-out rates in the overall sample as compared to rates usually observed in clinical trials (Dixon & Linardon, Reference Dixon and Linardon2020). Increased drop-out was considerably driven by patients leaving the residential DBT treatment earlier than planned, due to reasons unrelated to this study. Discharge before the post-measurement led to exclusion from the study and inflated the drop-out rate of this trial. Completers and non-completers of this study differed in the proportion of reported comorbidities, with patients who were diagnosed with an eating disorder in addition to BPD being more likely to complete the study. Further analyses showed that patients with younger age were more likely to drop out. This finding is at odds with a recent meta-analysis that did not find a significant effect of age on attrition rate in psychological treatment for BPD (Arntz et al., Reference Arntz, Mensink, Cox, Verhoef, van Emmerik, Rameckers and Grasman2023). The reported drop-out analyses were explorative, the results may not be robust and differences between completers and non-completers should be interpreted with caution.
Exploratory correlation analysis of the Amyg-EFP with regions of interest besides the amygdala revealed correlations with the dACC and the dlPFC. Furthermore, the Amyg-EFP signal mapped to the negative affect neurocircuitry, salience neurocircuitry, and cognitive control neurocircuitry, which relate to neurobehavioral functional domains as defined by the research domain criteria (Goldstein-Piekarski et al., Reference Goldstein-Piekarski, Ball, Samara, Staveland, Keller, Fleming and Williams2022; Insel et al., Reference Insel, Cuthbert, Garvey, Heinssen, Pine, Quinn and Wang2010). Additionally, activation from sensory and motor cortex correlated with the Amyg-EFP. Future studies comparing the Amyg-EFP to other e.g. more local brain measures are needed to investigate advantages and disadvantages of more locally focused brain signals for NF-training. A limitation concerns the explorative nature of this analysis. Hence, these findings require replication in an independent sample.
Originally, this study was designed to assess whether patients would generalize Amyg-EFP NF to amygdala-BOLD downregulation and whether the treatment would return a clinical benefit. A programming error in the NF-training script resulted in patients receiving feedback inverse to the original design defined in the trial protocol, i.e. Amyg-EFP upregulation instead of Amyg-EFP downregulation was rewarded with positive feedback for the participant. This programming error was only detected after data collection was completed. Therefore, it is not possible to assess generalization and clinical utility with this dataset. We did not observe any differences between the NF group and the DBT-only group in clinical measures, and no correlations of NF success and clinical change were significant (see online Supplement), suggesting that patients incurred no harm from upregulating Amyg-EFP.
Conclusions
The Amyg-EFP correlates with deep-brain activation and can be modulated with NF training. This research extends previous findings to a different laboratory and to a different sample, namely female patients with BPD. Salience/negative affect neurocircuitry and cognitive control neurocircuitry activation was found to correlate with the Amyg-EFP, a finding that awaits replication in future studies. NF as add-on therapeutic training is feasible, although considerable patient dropout in the residential treatment context was observed. Future research investigating the clinical utility of Amyg-EFP NF in BPD is needed.
Supplementary material
The supplementary material for this article can be found at https://doi.org/10.1017/S0033291723003549
Acknowledgements
The authors want to thank Madita Stirner for conducting patient measurements, Nike Unverhau and Ida Mueller for contributing to R-code, Florian Kirn for R-code review, Maurizio Sicorello for support with MLM analysis, and Martin Fungisai Gerchen for helpful advice on fMRI-replicability analysis.
Author contributions
C. S.: conceptualization, supervision, writing – review and editing. C. P.: conceptualization, methodology, data curation, formal analysis, verification, supervision, visualization, writing – original draft. G. G. and J. N. K.: software, data curation, formal analysis, writing – review and editing. M. J.: investigation, writing – review and editing. M. Z.: investigation, project administration, data curation, formal analysis, visualization, writing – original draft. S. B., P. A., and D. B.: methodology (EEG), writing – review and editing. S. C.: conceptualization, investigation. T. H.: conceptualization, supervision, software, writing – review and editing.
Funding statement
This study did not receive third-party funding.
Competing interests
This project was funded by intramural funds of the Department of Psychosomatic Medicine and Psychotherapy, Central Institute of Mental Health. The authors declare the following financial interests/personal relationships which may be considered as potential competing interests: T. H. is Chief Medical Scientist of GrayMatters Health co Haifa, Israel. C. P. received funding from GrayMatters Health for a different project not related to this work. D. B. served as an unpaid scientific consultant for an EU-funded neurofeedback trial unrelated to the present work. The other authors declare no conflicts of interest with respect to the authorship or the publication of this article.