Introduction
Glioblastoma (GBM) is the most common and aggressive glioma in adults. The latest World Health Organization guideline classifies GBM as grade IV glioma. Reference Louis, Perry and Reifenberger1 Over the last years, massive efforts have led to a better understanding of the pathology and the genetic of GBM. Reference deSouza, Shaweis and Han2 To date, the most effective and approved standard therapeutic regimen is maximum surgical resection of the tumor followed by concurrent chemoradiation and adjuvant chemotherapy with temozolomide (TMZ). Reference Louis, Perry and Reifenberger1 Despite this very intensive therapeutic regimen, newly diagnosed GBM patients have a dismal outcome with a median overall survival (OS) below 18 months. Reference Marenco-Hillembrand, Wijesekera and Suarez-Meade3 The main known prognostic factors are (i) age, (ii) Karnofsky performance status (KPS), (iii) MGMT promoter methylation status, and (iv) IDH mutational status. Reference Stupp, Mason and van den Bent4
Immunotherapies have dramatically improved melanoma prognosis Reference Leven, Padelli, Carré, Bellissant and Misery5 and other nonneurological solid tumors. Reference Leven, Padelli, Carré, Bellissant and Misery5 In the setting of primary brain cancer, results from clinical trials are still disappointing. Reference Muftuoglu and Liau6 Nonetheless, specific GBM patients responded, supporting the identification of biomarkers to stratify patients in the prescription of immunotherapies. Immune checkpoint proteins such as Cluster of Differentiation 80 (CD80; known as B7-1) and CD86 (known as B7-2) are expressed on the surface of tumor Reference Ville, Poirier, Blancho and Vanhove7 and immune cells Reference Trombetta, Soldano and Contini8 but not glial cells. Reference Uhlén, Fagerberg and Hallström9 CD80 protein expression was observed in infiltrative tumor lymphocytes in melanoma. Reference Hersey, Si, Smith and Thomas10
Cytotoxic T-lymphocyte-associated antigen–4 (CTLA-4) and Cluster of Differentiation 28 (CD28) are located on T-lymphocytes. Both CD28 and CTLA-4 proteins bind to their ligands on the antigen-presenting cells and major histocompatibility complex. Reference Wei, Duffy and Allison11 The interaction between immune checkpoint proteins and their coreceptor at the surface of T-lymphocytes delivers the signal to activate or inhibit T cells function, that is, CTLA-4 has a higher affinity to CD80 and CD86, and when bound to its ligands, T cells remain exhausted. Reference Rowshanravan, Halliday and Sansom12
In preclinical studies, antibodies targeting CTLA-4 were used in to block CTLA-4 from binding to its ligands. Reference Letendre, Monga, Milhem and Zakharia13 Ipilimumab – anti-CTLA4 – has also shown responses in patients with brain metastases, highlighting efficacy within the central nervous system. Reference Savoia, Astrua and Fava14 Expression of the most studied immune checkpoint proteins, programmed death-ligand (PD-L1), was inversely correlated with OS in GBM patients. Reference Nduom, Wei and Yaghi15 However, the expression of CD80 and CD86 in GBM tissues and their prognostic significance in the tumor microenvironment (TME) of newly diagnosed GBM patients has not been reported yet. This study investigated the mRNA and protein expression of CD80 and CD86 in the TME of newly diagnosed GBM patients, aged below 70 years old and with KPS above 70% treated with the standard of care. In addition, this study highlighted a possible correlation between CD80 and CD86 expression and the immune cell populations in the TME of newly diagnosed GBM patients.
Materials and Methods
Patient Samples
OncoNeuroTek (ONT) is a local brain tumor tissue bank collecting samples from patients operated at the University Hospital La Pitié-Salpêtrière. All samples were collected with informed consent from patients. The inclusion criteria of the discovery local cohort (47 patients) were as follows: (i) newly diagnosed and histologically verified GBM, (ii) age at diagnosis is below 70 years, (iii) KPS above 70%, (v) known MGMT promoter methylation status, (vi) known IDH status, (vii) treated with the standard first-line therapeutic regimen including maximal safe surgery, chemoradiation and adjuvant TMZ, and (viii) a documented clinical follow-up. The validation cohort (121 patients, TCGA cohort) clinical information and RNA-sequencing data (read counts) were downloaded from the National Cancer Institute’s Genomic Data commons Data portal and from the NCBI GEO GSE62944, respectively. Similar inclusion criteria were used for both cohorts.
Immunohistochemistry Staining
Paraffin-embedded tissue blocks (5–7 μm) from biopsies of newly diagnosed GBM patients were received from the ONT biobank. The slides were obtained from diagnostic blocks and were selected to get a homogeneous group of patients for prognostic studies. Indeed, we have selected the patients aged below 70 years old, with a KPS > 70% and treated with the standard of care to be in line with inclusion criteria of the clinical trial that has established the standard of care. Reference Stupp, Mason and van den Bent4 Tissue sections (two sections per patients) were deparaffinized using xylene and rehydrated. For antigen retrieval, each slide was embedded in citrate buffer at pH 4.0 and heated for 15 min in the microwave at 800 W. 10% goat serum with 5% fetal bovine serum in 0.2% triton phosphate buffer saline was used as a blocking buffer. 3% hydrogen peroxide was used to block tissue peroxidation. Antihuman CD80 antibody (A16039; Abclonal) and antihuman CD86 antibody (A2353; Abclonal) were used at 1:500 dilution in blocking solution and incubated on the tissue slides overnight at room temperature. Avidin–Biotin Complex kit was used as a signal enhancer before the incubation in 3,3′-Diaminobenzidine (DAB). Slides were embedded in hematoxylin dye and rinsed with tap water for nuclear staining; gradual alcohol and xylene baths were used for dehydration and mounted with a hydrophobic mounting medium (Sigma, 24845633). All stained tissues were scanned via ZEISS Axio Scan 40× for bright field imaging.
Quantification of IHC Staining
Following all slidesʼ imaging, three regions of interest with known dimensions (528 * 528 µm) were randomly selected for each tissue section and quantified using an in-house quantification Fiji code. Shortly, each image was imported to the Fiji program. Reference Schindelin, Arganda-Carreras and Frise16 Using the color deconvolution tool, the area positive for DAB staining was isolated and quantified using a semiautomated in-house generated code. The percentage of DAB positive areas was calculated, and the mean value from the three images was calculated and used in the survival analysis.
Quantitative Reverse Transcriptase Polymerase Chain Reaction
RNA samples were obtained from ONT bank and used to synthesize cDNA. Reverse transcription of RNA samples was performed using the Maxima First Strand cDNA Synthesis Kit (Thermo Scientific, K1442) according to the manufacturer’s recommendation with 100–250 ng of RNA. Quantitative reverse transcriptase polymerase chain reaction was used to quantify the expression levels of CD80 and CD86 in patients. PPIA gene was used as a house reference gene for normalization as previously described. Reference Vessières, Quissac and Lemaire17 Primers were designed using Universal Probe Library (UPL) for Human. Primer’s sequences were as follows: PPIA (left: atgctggacccaacacaaat; right: tctttcactttgccaaacacc; UPL probe 48) CD80 (left: gaagcaaggggctgaaaag; right: ggaagttcccagaagaggtca; UPL probe 10) and CD86 (left: cagaagcagccaaaatggat; right: gaatcttcagaggagcagcac; UPL probe 15). cDNA samples were analyzed using the Light Cycler Probe Master mix 2× (Roche, 04887301001) and the UPL detection system (Roche, 04483433001) in a Light Cycler 96 (Roche). For each qPCR, two independent experiments were completed with duplicate samples in each experiment. The mean of 2^-(CTgene of interest-CTPPIA) from the two different experiments was used in all analyses.
Statistical Analysis
A violin plot was used to visualize our data’s full distribution (GraphPad Prism). Reference Savoia, Astrua and Fava14 Spearman correlation between the expression values (RNA or protein) and age was evaluated to discard age bias. Survival analysis was performed by an open-source validated approach Reference Zhou, Zeng and Zhang18,Reference Li, Chen, Wang, Zhang, Su and Zhang19 by finding a supervised cutoff value for the CD80 or CD86 expression independently using the “survminer::surv_cutpoint” function, which determines the cut point based on the highest/lowest value of the log-rank statistics (low or high expression values), and then using these categories for Kaplan–Meier analysis or Cox proportional hazard regression modeling testing at each variable independently or to adjust for multiple variables including CD80/CD86 expressions and MGMT promoter methylation status p-values lower than 0.05 were considered significant. Reference Greenbaum, Colangelo, Williams and Gerstein20,Reference van Nieuwenhuijze and Liston21 Furthermore, we have used TCGA database to evaluate and profile tumor infiltrating immune populations and whether it differ among the highly expressed C86 tumor cells. TCGA immune data (i.e., CIBERSORT calculated immune populations) was retrieved from https://cavei.github.io/example-datasets/panCancerAnnotation.RData. Comparisons were performed by two-side Wilcoxon-test and p-values were corrected for multiple comparisons using FDR method.
Results
Patients and Tumors Characteristics
Forty-seven patients with a confirmed GBM diagnosis fulfilled the inclusion criteria: 14 men and 33 women (percentage 29.8%–70.2%). The patientsʼ median age at diagnosis was 55.9 years (range: 24.3–69.5 years). KPS was 70% and above in all patients. The median OS is 559 days (range 31–2539), and the median PFS is 266 days (range 26–1355). The IDH status was evaluated as mutant for two patients (4.3%) while wildtype for 45 (95.7%). Furthermore, the MGMT promoter was methylated in 16 patients (34%) and unmethylated in 31 (66%). All patients were treated with the standard of care first-line treatment including maximal safe surgery, radio chemotherapy, and adjuvant chemotherapy with TMZ.
CD80 and CD86 Expression at mRNA and Protein Level
At the mRNA level, CD86 expression was quantitatively higher than CD80 expression in the TME (Figure 1A). In agreement with mRNA expression, immunohistochemistry (IHC) analysis showed that the expression of CD86 is higher than CD80 in our discovery cohort (Figure 1B). Based on the IHC staining, CD80 and CD86 are observed in the cell membrane and/or the cytoplasm (Figure 2). Following protein quantification, we observed a positive correlation between RNA and protein expression for CD86 (Spearman coefficient of correlation Rho = 0.28; p = 0.08; Figure 1C). However, we observed a weaker correlation between mRNA and protein expression for CD80 (p = 0.108; Rho = 0.25; Figure 1D).
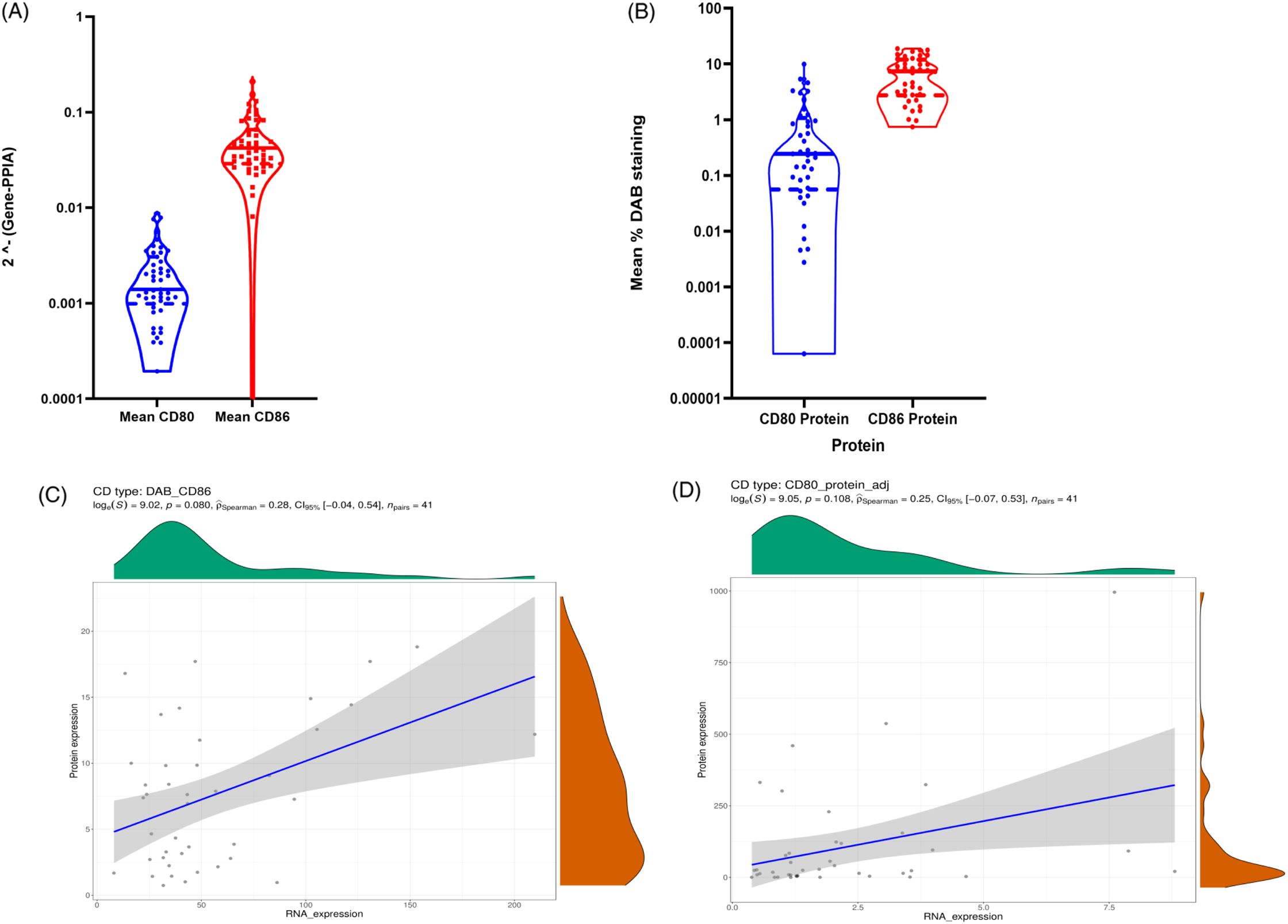
Figure 1: (A) Violin plot to visualize the data distribution of CD80 and CD86 mRNA expression in ONT database; (B) shows CD80 and CD86 protein expression in ONT database. (C–D) Spearman correlations between CD86 protein values and CD86 RNA values. (C) represents CD86 protein quantification based on the mean percentage of positive DAB signals correlation with mRNA values. (D) shows CD80 protein values quantified based on the mean percentage of positive DAB signals correlation with mRNA values.
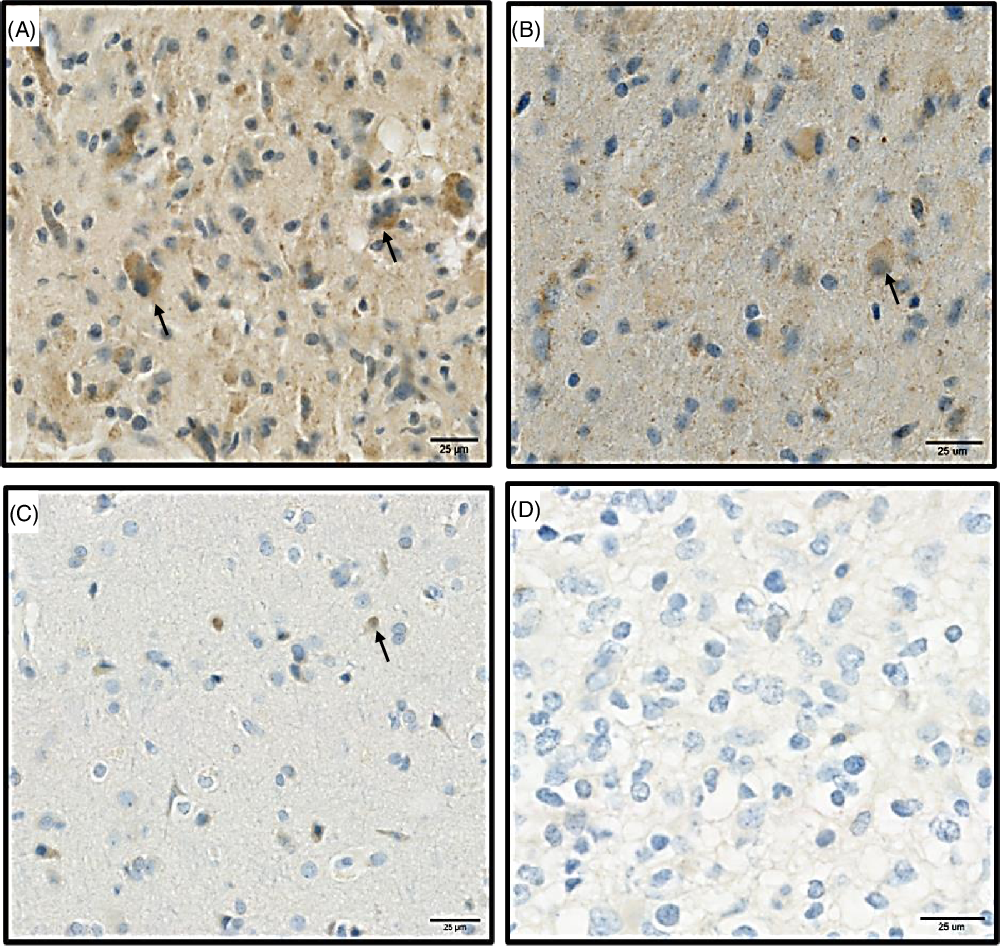
Figure 2: Represents the protein expression of CD86 and CD80 proteins in paraffin sectioned GBM samples. (A) High expression of CD86 protein. (B) Low expression of CD86. (C) High expression of CD80. (D) Low expression of CD80. Black arrows (brown signals) highlight a positive staining for CD80 and CD86 proteins and represent the signals that were used for quantifications, blue staining correspond to hematoxylin dye which was used as counterstaining.
Prognostic Value of CD80 and CD86 Expression
Our patient’s cohort was used as a discovery cohort, while the TCGA dataset was used as a validation cohort. In a univariate analysis, mRNA expression of CD80 and CD86 was not significantly correlated with OS in both the ONT cohort and TCGA dataset (Table 1). On the other hand, CD80 and CD86 mRNA high expression was significantly associated with shorter PFS (p = 0.04 and p = 0.005, respectively; Figure 3A,B). Moreover, these findings were validated using the TCGA cohort; higher CD80 and CD86 expressions were correlated with shorter PFS (p-value; 0.0428, 0.00283; Figure 3C,D). Interestingly, higher CD86 protein expression was associated with shorter PFS in the ONT cohort (p < 0.005; Table 2). CD80 and CD86 protein expression were not available in the TCGA dataset for validation purposes. However, we have used TCGA database to profile tumor-infiltrating immune cells in the selected cohort.
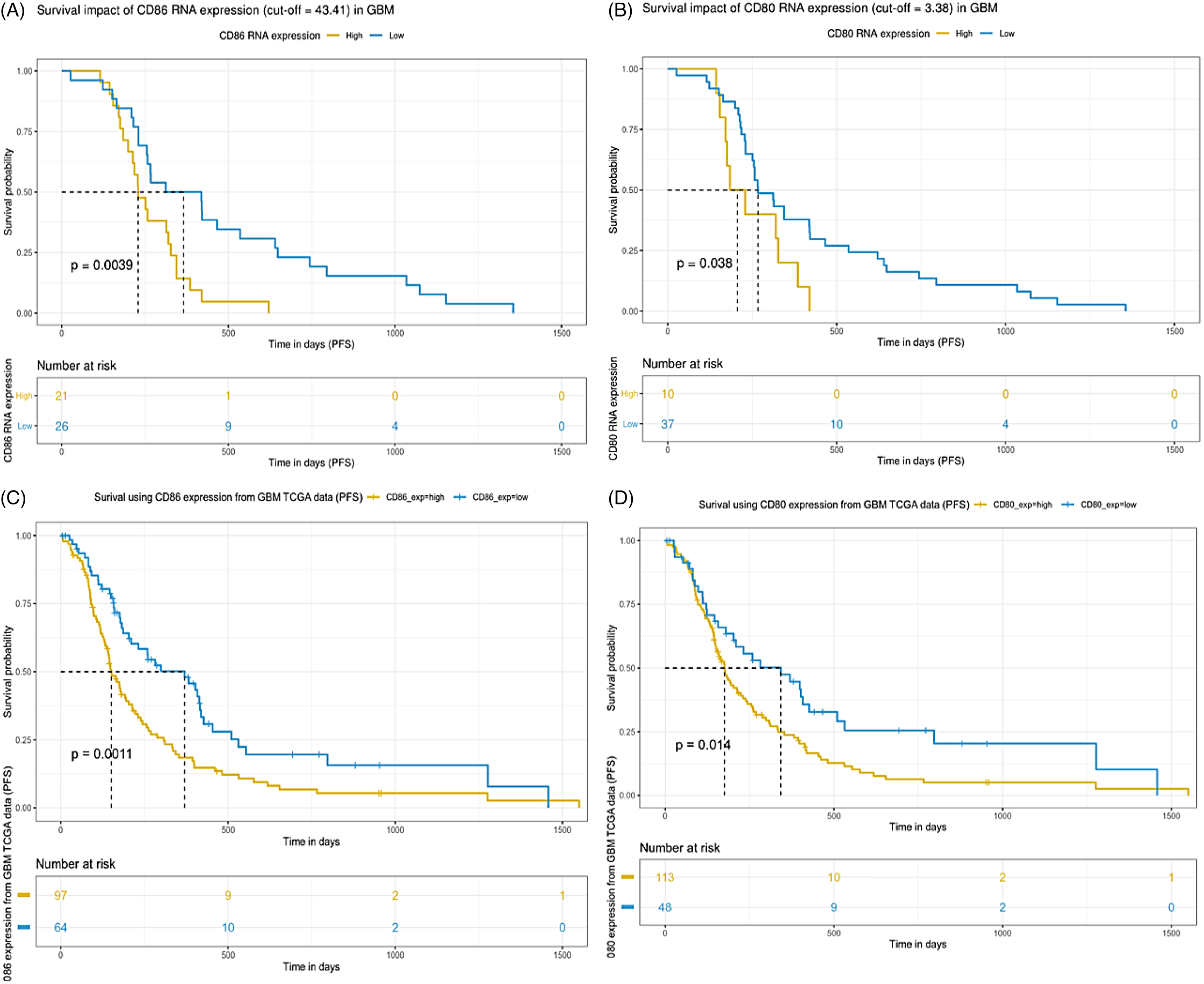
Figure 3: CD80 and CD86 mRNA expression and outcome in GBM in both ONT and TCCA database. (A) Kaplan–Meier PFS estimates in GBM patients in relation to CD86 (ONT database) (B) Kaplan–Meier PFS estimates in GBM patients in relation to CD80 (ONT database). (C) Kaplan–Meier PFS estimates in GBM patients in relation to CD86 (TCGA database). (D) Kaplan–Meier PFS estimates in GBM patients in relation to CD80 (TCGA database).
Table 1: Univariate analysis (Cox-P regression) for OS in both ONT and TCGA database
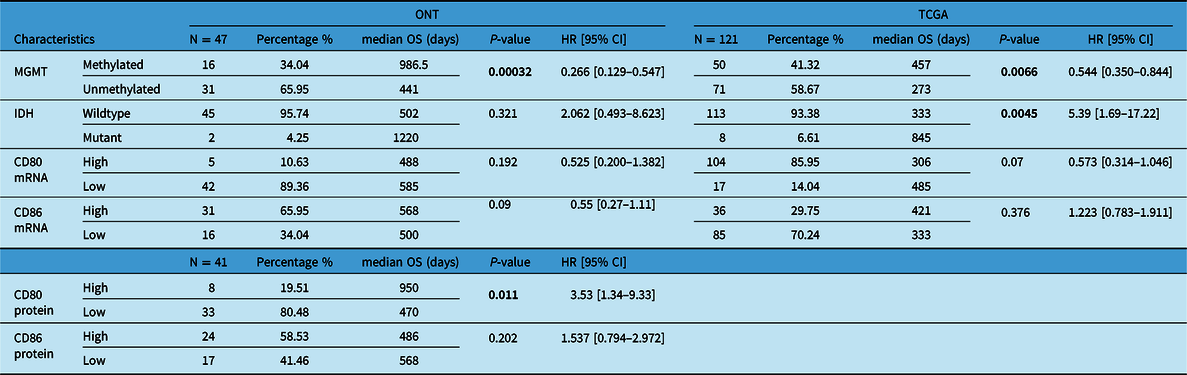
Boldface values are considered significant.
Table 2: Univariate analysis (Cox-P regression) for PFS in both ONT and TCGA database
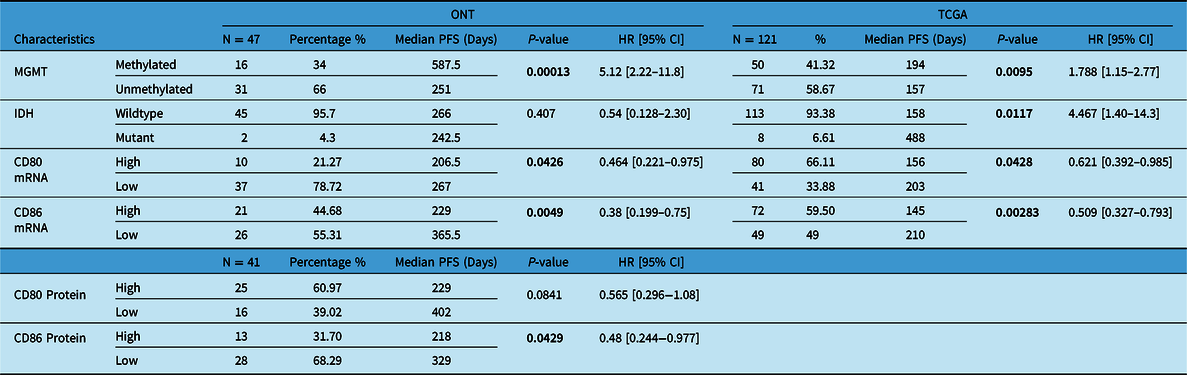
Boldface values are considered significant.
As expected, MGMT promoter methylation was associated with longer PFS and longer OS in the ONT cohort (p < 0.05 and p < 0.05, respectively) and TCGA dataset (p < 0.05 and p < 0.05, respectively) (Tables 1 and 2). Furthermore, IDH mutations were also associated with better OS and PFS in the TCGA database (p < 0.05 and p < 0.05, respectively); however, in the ONT cohort, the limited number of IDH-mutant GBM did not allow a robust analysis (n = 2). In multivariate analysis, CD80 mRNA expression did not provide additional prognostic information to MGMT promoter methylation in the ONT cohort. On the other hand, multivariate analysis of CD86 mRNA expression was an independent prognostic factor for PFS in the TCGA dataset only (p < 0.05; Figure 4). We have observed a similar trend (p = 0.27; Figure 4) in the ONT cohort, yet the trend was not significant, which could be related to the lower number of patients (n = 47) in the ONT cohort compared to (n = 121) in the TCGA database.
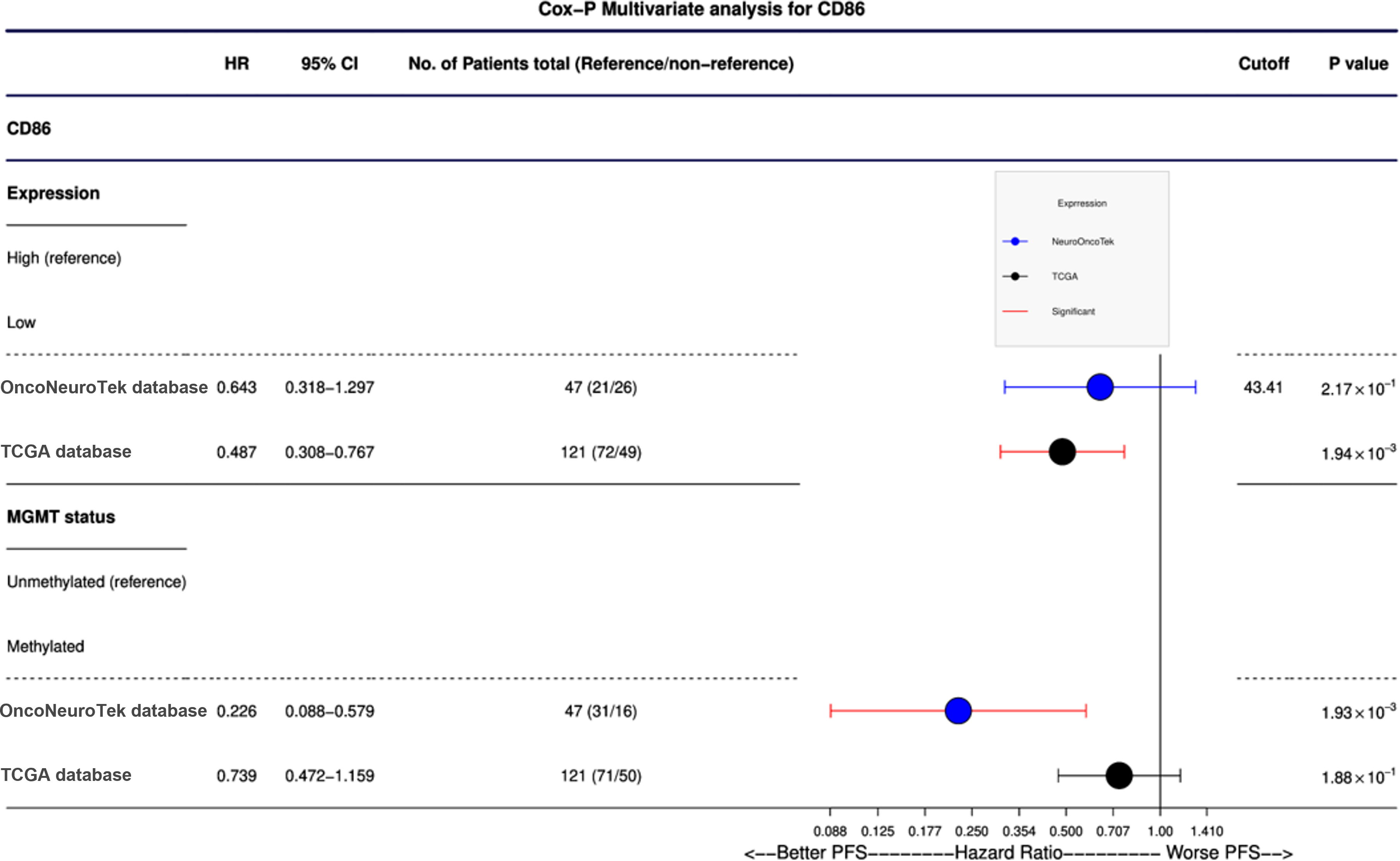
Figure 4: Cox-P (proportional hazards) multivariate analysis of CD86 protein expression and mRNA expression. CD86 was found to be an independent prognostic factor in TCGA database (p = 0.0019); mRNA expression of CD86 is a more predictive prognostic factor than MGMT methylation. A nonsignificant trend was observed in our ONT cohort.
The Relationship Between CD86 Expression and Immune Cell Populations
Immune cell populations were evaluated using CIBERSORT, and we compared the immune cell populations between patients expressing both CD80 and CD86 as high and low expression. CD80 and CD86 are expressed on the surface of tumor-associated macrophages’ surface suggesting a role in immunosuppressive TME. Immune cell population analysis showed low fraction of classically activated macrophages (M1) and higher fraction of immunosuppressive macrophages (M2). High CD86 expression group contained more patients with high M2 macrophages fraction (p = 0.00013; Figure 5). On the other hand, high CD86 expression group contained more patients with low tumor-infiltration lymphocytes fraction (p = 0.005; Figure 5). This effect was not observed in CD80 expression patients. Additionally, high CD86 expression group contained more patients with low CD8+ cell fraction (p = 0.039; Figure 5) whereas, the low CD86 expression group contained more patients with high CD8+ fraction. Although further studies are warranted, these data suggest association between high CD86 expression, immunosuppressive TME, and low activity of CD8+ cytotoxic T lymphocytes.
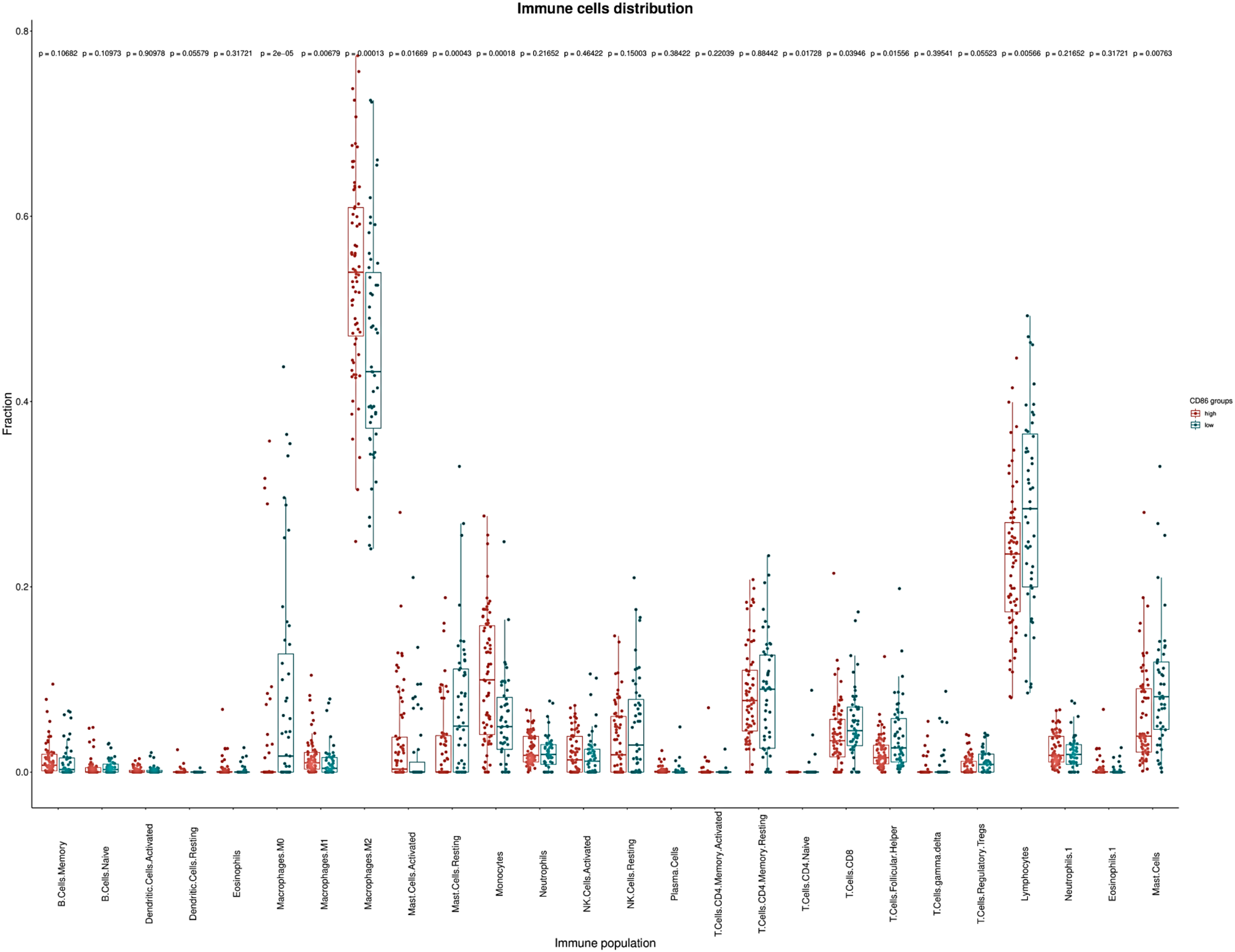
Figure 5: CIBERSORT calculated tumor infiltrating immune populations in TCGA database. Immune cell populations represented fraction of the X-axis immune cells to the whole gene expression mixture. Box plots depicting the estimated relative fractions of immune cell types by GBM category according to CD86 expression. The Y-axis here show the relative proportion which can range from 0 to 1. Relative fraction estimates the percentage of a given cell population in the total tumor infiltrate. In our analyses immunosuppressive M2 macrophages and lymphocytes were the most frequently observed immune phenotypes.
Discussion
CD80 and CD86 molecules play an essential role in influencing the immune recognition of GBM cells. They bind to the CD28 molecule with a costimulatory signal for T-lymphocytes activation. On the other hand, they bind to CTLA-4, resulting in an immunosuppressive effect. CTLA-4 has a higher affinity to CD80 and CD86, making these moleculesʼ role in immunosuppressive effect higher than their costimulatory effect. Reference van Nieuwenhuijze and Liston21 The current study has linked CD80 and CD86 expression on GBM TME to PFS. We observed a low correlation between mRNA and protein expression of CD80. However, a better correlation was observed between CD86 protein and mRNA expression. Low correlation between the mRNA and protein expression might be due to posttranscriptional mechanisms involved in turning mRNA into protein. Not to mention, there is a possible error and noise in protein quantification and mRNA extraction that could influence mRNA stability and protein expression. Reference Greenbaum, Colangelo, Williams and Gerstein20 In addition to DAB staining intensity used in our study, quantification of protein using the number of positive cells should also be evaluated in future IHC analyses to better understand expression of proteins and mRNA of interest.
Number of patients (n = 47) in the ONT cohort is lower than the number of patients in the TCGA dataset (n = 121). The higher number of TCGA GBM samples could be one reason that affected the statistical analysis and provided a better prognostic value than the ONT cohort. Indeed, GBM samplesʼ availability with comprehensive clinical and biological annotations and fulfilling the inclusion criteria is a limitation for a larger cohort. Larger patient cohort is needed to evaluate the prognostic value of CD86 expression in the TME of GBM patients. Using TCGA data to profile immune cell populations interestingly revealed that CD86 expression is associated with an immunosuppressive TME with low activity of cytotoxic T cells however protein analysis of immune cell populations is needed to validate our findings from TCGA immune cell population profiling. Indeed, high CD86 expression is associated with a cold immune microenvironment with a limited antitumor immune response promoting tumor growth and poor prognosis.
The expression of 50 immune checkpoint molecules was investigated in breast cancer. The study showed that high expression of costimulatory immune checkpoint molecules was associated with better PFS. However, no significant effect on prognosis was associated with CD80 and CD86 expression in the selected cohort. Reference Fang, Chen, Liu, Gu, Chen and Wang22 Feng et al. Reference Feng, Lu and Wang23 reported that low expression of CD80 is a predictive biomarker for poor prognosis in gastric adenocarcinoma. Furthermore, CD80 and CD86 were found to be potential biomarkers for better prognosis survival in nasopharyngeal carcinoma. Reference Chang, Chang, Hsu, Lin and Chung24 Additionally, the molecular characterization of PD-L1 expression was correlated with other checkpoint proteins, that is, CD80, highlighting that higher levels of immunosuppression are associated with GBM than lower-grade gliomas (LGG). Reference Wang, Zhang and Liu25 In myeloma cell lines, silencing the CD28–CD86 pathway resulted in myeloma cellsʼ significant cell death. Reference Gavile, Barwick and Newman26 A recent study constructed a more robust model, using GBM and LGG data from the TCGA and Chinese Glioma Genomic Atlas, and identified that low expression of CD86 molecules is a good prognostic indicator for OS. PFS analysis was not applied in this study. Reference Qiu, Li, Cheng, Li, He and Li27
In 2017, Berghoff et al. described a specific signature to predict the success of TMZ in MGMT-methylated patients. They showed that the TME signature could be used to indicate an individual’s TMZ sensitivity. The TME was identified to be different between IDH-mutant and IDH-wildtype. A richer tumor infiltrative lymphocyte and a higher expression of PD-L1 were observed in IDH-wildtype tumors. Reference Berghoff, Kiesel and Widhalm28 However, to date, no studies have linked MGMT promoter methylation with the TME. A recent research article has studied the expression of immune checkpoint inhibitor Tim3 and MGMT methylated status. They identified that a high expression of Tim3 in MGMT-unmethylated patients is linked to poor prognosis. Reference Zhang, Sai and Wang29 Pratt et al. Reference Pratt, Dominah and Lobel30 have reported that the expression of PD-L1 is a negative prognostic biomarker in recurrent IDH-wildtype GBM. In line with these findings, our study supports that the expression of immune checkpoint inhibitors may inhibit T-lymphocyte and antitumor reaction. A recent integrated analysis of the prognostic value of CD86 reveals that CD86 is heterogeneously expressed in gliomas and is an independent unfavorable prognostic value in LGG. Reference Qiu, Tian and He31
CD86 molecular status could be explored as a predictor of response to immunotherapies in the setting of future clinical trials dedicated to GBM patients. Our study suffers from the limitation of retrospective studies with a limited number of patients. Nonetheless, our results were validated in an independent dataset and support investigations of immune checkpoint molecules as potential prognostic biomarkers and potential predictive biomarkers of response to immunotherapies in GBM.
Acknowledgements
This work was supported by the European Union’s Horizon 2020 research and innovation program under the Marie Skłodowska-Curie grant agreement #766069 (GLIO-TRAIN).
Conflicts of Interest
No potential conflicts of interest were disclosed.
Author Contributions
MA and AI designed the experiments, wrote the manuscript, and approved the manuscript’s final version. MA performed the experiments. IHV performed the statistical analysis and revised the manuscript. FB, JL, and MV provided a technical support for IHC optimization and protein quantification. All authors reviewed the manuscript.
Ethics Approval
All samples were collected with informed consent from patients.
Data Statement
All annotated data will be available upon request from the authors.