Introduction
Rationale
Society today produces more data in two days than it had cumulatively produced prior to 2003 (Sagiroglu and Sinanc, Reference Sagiroglu and Sinanc2013). In human healthcare, data come from a variety of sources at a rapid pace. Data sources include social media, wearable sensors, surveillance systems, electronic medical records, and laboratory databases. Publications indexed in Google scholar that referenced ‘big data’ grew dramatically since 2008 (Andreu-Perez et al., Reference Andreu-Perez, Poon, Merrifield, Wong and Yang2015). The top two health research areas were ‘bioinformatics’ and ‘health informatics’.
In animal health, data also come from multiple sources at a rapid pace. Pet owners post photos and updates of their pets on social media. Wearables and other sensors have been developed for pets (https://www.whistle.com), horses (Peacock, Reference Peacock2012; Thompson et al., Reference Thompson, Luck, Keshwani, Pitla and Karr2018), and production animals (Andersson et al., Reference Andersson, Okada, Miura, Zhang, Yoshioka, Aso and Itoh2016; Haladjian et al., Reference Haladjia, Haug, Nüske, Bruegge, Haladjian, Haug, Nüske and Bruegge2018). Other sources of animal health data include government surveillance on animal diseases, veterinary electronic medical records, farm production records, and species-specific databases. These trends suggest that ‘big data’, ‘informatics’, and ‘bioinformatics’ might be growing in a similar fashion to that of human health. However, no one has evaluated how these terms are used in the veterinary medical and animal health literature.
Big data is frequently described in terms of three ‘V's: volume, velocity, and variety (Schroeck et al., Reference Schroeck, Shockley, Smart, Romero-Morales and Tufano2012). Volume refers to a large amount of data, velocity means that the data are generated quickly, and variety infers that the data come from different data sources and/or consist of different types of data (Schroeck et al., Reference Schroeck, Shockley, Smart, Romero-Morales and Tufano2012). Veracity, or data reliability, is often considered a fourth characteristic of big data. Big data may also require non-traditional storage methods and analytical techniques (Elgendy and Elragal, Reference Elgendy and Elragal2014). Sources of big data in human healthcare include electronic medical records, genomics, imaging data, and data from social networks and sensors (Gaitanou et al., Reference Gaitanou, Garoufallou and Balatsoukas2014).
Definitions of ‘informatics’ and ‘bioinformatics’ are broad and overlap with each other. The American Medical Informatics Association defines ‘informatics’ as ‘the interdisciplinary field that studies and pursues the effective uses of biomedical data, information, and knowledge for scientific inquiry, problem solving, and decision making, motivated by efforts to improve human health’ (Kulikowski et al., Reference Kulikowski, Shortliffe, Currie, Elkin, Hunter, Johnson, Kalet, Lenert, Musen, Ozbolt, Smith, Tarczy-Hornoch and Williamson2012). The National Institutes of Health defines ‘bioinformatics’ as ‘research, development, or application of computational tools and approaches for expanding the use of biological, medical, behavioral or health data, including those to acquire, store, organize, archive, analyze, or visualize such data’ (Huerta et al., Reference Huerta, Downing, Haseltine, Seto and Liu2000).
Examining the use of these terms in the literature will provide insight into the type of research being conducted in each of these fields and may improve our understanding of big data, informatics, and bioinformatics and their relationships to (and how to distinguish them from) each other. Additionally, such examination will illuminate how research in these fields is conducted, who the leaders in the field are, the expertise needed to conduct such research and where the research is published.
For the remainder of this manuscript, we refer specifically to the terms big data, informatics, and bioinformatics with quotes (e.g. ‘big data’, ‘informatics’, and ‘informatics’). When an article or group of articles is described using one of these terms in quotes (e.g. “‘big data' article”, “‘big data' articles”, and “articles about ‘big data'”), we mean that the article or articles contain the quoted term.
Objectives
The purpose of this scoping review was to describe how ‘big data’, ‘informatics’, and ‘bioinformatics’ have been used in the animal health and veterinary medical literature by mapping the literature and describing the publications using these terms.
Materials and methods
Protocol
The authors used a scoping review approach as described by Arksey and O'Malley (Arksey and O'Malley, Reference Arksey and O'Malley2005). Study objectives and eligibility criteria were stated a priori. Most sections of the protocol were developed a priori with sections of the data charting tool and training tool modified after the review process started. The data synthesis plan was modified based on the findings of data charting.
Eligibility criteria
Smith and Williams (Smith and Williams, Reference Smith and Williams2000) conducted a literature review of informatics in veterinary medicine from 1966 through 1995. Therefore, articles published in 1995 and later were selected for inclusion in the current study.
Information sources
The literature search covered the dates 1 January 1995 to 19 June 2017 in the following databases: Agricola (via ProQuest), ProQuest Dissertations and Theses, Medline (via PubMed), Web of Science, and IEEE Xplorer. The literature searches were conducted from 6 June 2017 to 19 June 2017. There were no language restrictions at this stage. Agricola, ProQuest, Medline, and Web of Science were chosen to capture scientific research in the animal health and veterinary medical literature. IEEE Xplorer was chosen to capture relevant engineering research in animal health and veterinary medicine.
Search
The search strategy was developed by a team of animal health and veterinary medical professionals, veterinary epidemiologists, a computer scientist and a library scientist (Table 1). The search strategy included conceptual and contextual terms (Peters et al., Reference Peters, Godfrey, Khalil, McInerney, Parker and Soares2015). The conceptual terms were chosen to represent the topics of interest, which were ‘big data’, ‘informatics’ (lines 1 and 2 of Table 1a), and ‘bioinformatics’ (line 1 of Table 1b). Synonyms for ‘informatics’, ‘information systems’, and ‘information technology’, were also included as conceptual terms in the search strategy. The contextual terms were chosen to represent animal health and veterinary medicine. Contextual terms were limited to major small and large companion animals and food animals. Contextual terms included singular and plural variations (as well as scientific species names, e.g. canine, feline) of the following words: dog, cat, horse, dairy cattle, beef cattle, goat, sheep, layer poultry, broiler poultry, zoonoses, and foodborne (lines 3–17 of Table 1a and lines 2–16 of Table 1b). ‘Zoonoses’ and ‘foodborne’ were included to capture articles from a public health and food safety veterinary medical perspective, respectively.
Table 1. Example of search strategy performed in Medline via PubMed to identify articles that use the terms (a) ‘big data’ or ‘informatics’ and (b) ‘bioinformatics’ in the animal health and veterinary medical literature
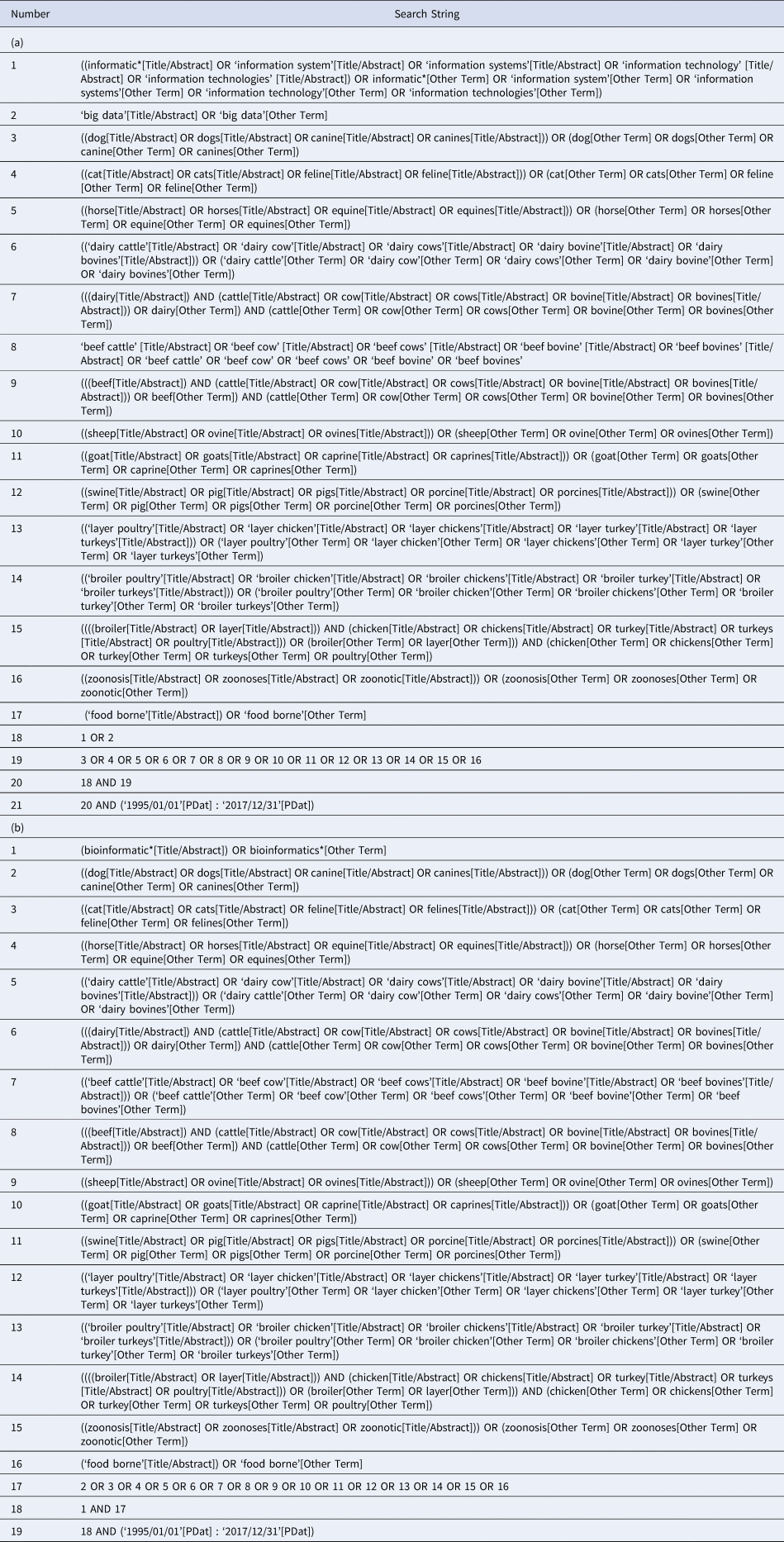
Citations from Medline (via PubMed) were uploaded to Microsoft EndNote and then imported into DistillerSR (Evidence Partners, Ottawa, Canada). RIS files were downloaded from the other databases and uploaded directly to DistillerSR and deduplicated.
Selection of sources of evidence
Relevance screening was performed on title, abstract, and keyword (TAK) followed by full-text screening. The TAK relevance screening tool was piloted on randomly selected articles. Cohen's kappa was used to measure agreement between the primary reviewer (ZBO) and secondary reviewers. Cohen's kappa was used as a guide to help the research team train reviewers and refine questions in the relevance screening tool. Reviewer feedback on the relevance screening tool and/or a Cohen's kappa of 0.7 or more was used to determine sufficient agreement. For both TAK and full-text screening, agreement between two reviewers was required for articles to be included or excluded. Disagreements were resolved by consensus between the dissenting reviewers. If consensus was not achieved between two reviewers, a third reviewer was consulted.
Articles with TAKs containing at least one contextual term and at least one conceptual term proceeded to full-text relevance screening. Reviewers could select ‘unsure’ during TAK relevance screening. These articles also proceeded to full-text screening.
Searches for full-text articles were conducted on the University of Guelph library website. If not available, an interlibrary loan request was placed. Full-text articles that were not acquired via interlibrary loan were then searched for in the Google search engine and in Google Scholar using titles and first author. Any full-text articles that were not found on Google or Google Scholar were excluded.
In full-text relevance screening, reviewers determined whether at least one contextual term present in the article referred to an animal (e.g. ‘cat’ versus ‘CAT scan’) and whether the contextual terms implied that the study was relevant to animals (e.g. a study that utilized an equine virus in the development of a human vaccine for use in humans with no mention of animal health implications would be excluded; a study that utilized an equine virus in the development of a human vaccine that has implications for both human and animal health would be included). If the contextual terms satisfied these conditions, the article proceeded to the final stage of relevance screening. In the final stage, reviewers determined whether the conceptual terms were used to describe the study or if the conceptual terms described a study referenced by the article (e.g. an article that stated ‘The current study utilizes big data’ would be included; a study that stated ‘Previous studies utilizing big data suggested an association’, but ‘big data’ did not apply to the study itself would be excluded). If the conceptual terms were used to describe the study in the article, the article proceeded to full-text screening. Non-English articles were excluded at this stage of the study.
Data charting process
We developed a data collection form which went through two iterations of review by the entire research team and was piloted among ZBO, RE, RM, AT, and KW before being finalized.
Data collection was performed by eight members of the review team (ZBO, AT, EM, RE, VS, KW, JS, and IS). Reviewers were given a set of articles and initially met with ZBO for consensus after 10–50 articles were complete. Questions about the review protocol were addressed and disagreements in data collection were resolved.
Data items
Articles were identified as either describing: (1) primary studies (studies where the research team collected original data, conducted an original analysis or performed simulation-modeling); or (2) reviews (systematic, scoping, narrative), commentaries/editorials, letters-to-the-editor or conference proceedings. Although conference proceedings may have described primary studies, due to variations in the format of conference proceedings (i.e. some were abstracts only while others resembled complete scientific papers), conference proceedings were not grouped with primary studies.
Species that the articles were describing were identified. Species were limited to those described in Table 8. The search and subsequent data collection was limited to the major domestic species encountered in veterinary medicine and animal health. Inclusion of other species (e.g. exotics, wildlife) was beyond the scope of this review.
Data were collected on the geographic region of the study. If it was not provided, the first author location was used. Geographic regions were based on the Standard Country or Area Codes for Statistical Use published by the United Nations (https://unstats.un.org/unsd/methodology/m49/).
The first author affiliation was collected to provide an understanding of the fields of study involved in producing research in big data, informatics or bioinformatics in veterinary medicine and animal health. Journal of publication was collected to provide an understanding of who is interested in this research. The classification scheme for the first author affiliation and journal of publication is presented in Table 2.
Table 2. Classification scheme of first authors and journal types

The data items shown in Table 10 were collected for articles that described primary studies. Primary studies were classified into types (Table 10) and study levels (Table 3). Studies classified as having study levels at the ‘genes, proteins, molecules and metabolites of animals’ or ‘genes, proteins, molecules and metabolites of organisms found on/in animals’ investigated genetic material will be referred to as ‘genetic studies’, and may include, but not limited to, gene sequencing, genomic, metagenomic, and microbiome studies. Data sources used in primary studies were also categorized (Table 4).
Table 3. Study level classification (organized by subject area domain) for data charting of primary studies using the terms ‘big data’, ‘informatics’, and ‘bioinformatics’

Table 4. Descriptions and examples of data sources used in primary studies using the terms ‘big data’, ‘informatics’, and ‘bioinformatics’

Initially, no distinction was made between genetic databases and non-genetic databases in the government-sourced category. After data classification was completed, it was decided post-hoc to estimate the number of government genetic databases. The number of articles classified as using government data sources that had the terms ‘NCBI’ (National Center for Biotechnology Information), ‘GenBank’ or ‘DAVID’ (Database for Annotation, Visualization and Integrated Discovery) were counted. GenBank and DAVID are nucleotide and protein sequence databases. GenBank is hosted by NCBI, which is an organization that hosts search engines of several databases, including GenBank. Genetic data from non-government databases were classified under ‘genetic databases’.
Reviewers were given the option of selecting multiple answers for each data item. For the study level and study type, each selection must have been stated in the study objectives. Thus, an article with a study objective that states that only prevalence of a bacterium was measured may have reported the results of a hypothesis test; however, the reviewer could not select ‘hypothesis test’ under study type because it was not reflected in the study objectives.
Synthesis of results
The number of articles per year that used the conceptual terms ‘big data’, ‘informatics’, and ‘bioinformatics’ was compiled into a timeline (Fig. 2). The frequency of articles that used the conceptual terms was compared to publication type (Table 7). Data regarding species, geographic region, first author affiliation, and journal of publication for each conceptual term were extracted for all articles and compiled in Table 8. A layered barplot (Fig. 4) (post-hoc) was created to illustrate the number of articles about each species by the geographic region. Most studies about pigs used the term ‘bioinformatics’ (Table 8), so it was decided post-hoc to determine if this was true for each geographic region (Table 9). The study level, study type, and data sources for each conceptual term were collected and were presented in Table 10.
Results
Selection of sources of evidence
The literature search yielded 8602 articles. There were 1093 articles included in data characterization after de-duplication, TAK relevance screening, and full-text screening. Of these, 918 were full-text articles that described a primary research study and 175 articles were conference proceedings or were not primary research studies (e.g. narrative reviews, scoping reviews, letter-to-the-editor, conference proceedings, and commentaries). Of the 578 articles that were excluded on full-text screening, 147 articles were not found, 93 articles were not in English, and 338 articles did not pass full-text relevance screening (Fig. 1).

Fig. 1. Flow of articles and citation from literature search through data characterization.
Results of individual sources of evidence and synthesis of results
Figure 2 shows that the use of the term ‘bioinformatics’ increased rapidly since 1995. The use of ‘informatics’ increased until 2012, then began to decline. The term ‘big data’ was first used in 2012 in one publication and was used in one publication in 2013 and 2014. The use of the term increased to four articles in 2015 and five articles in 2016. Data for 2017 are for a partial year, as the search period ended June 19, 2017

Fig. 2. Frequency of the use of ‘big data’, ‘informatics’, and ‘bioinformatics’ per year.
The majority of articles used ‘bioinformatics’ (Fig. 3). Articles about ‘informatics’ were the second most common, of which 57% (250/438) described using geographic information systems (GIS). Only 14 articles in the veterinary medical and animal health literature used the term ‘big data’, and half of them were narrative reviews, commentaries, editorials or letters-to-the-editor (Table 7). ‘Informatics’ and ‘bioinformatics’ articles were most frequently primary studies. The characterization for the ‘big data’ articles is shown below (Tables 5 and 6).

Fig. 3. Number of articles that used the words ‘big data’, ‘informatics’ or ‘bioinformatics’.
Table 5. List of five primary studies that contain the term ‘big data’

Table 6. List of nine reviews, commentaries, editorials, letters-to-the-editor, and conference proceedings that contain the term ‘big data’

Table 7. Frequency of ‘big data’, ‘informatics’, and ‘bioinformatics’ in 1093 publications in the animal health and veterinary medical literature

a Exceeds 1093 because articles may contain multiple conceptual terms.
General characteristics of the articles are included in Table 8. Articles about small animals (dogs and cats) used ‘informatics’ more than ‘bioinformatics’. ‘Informatics’ and ‘bioinformatics’ were relatively balanced between articles about cattle where the production system (dairy, beef) was specified. Articles where the production systems were unspecified were more often about ‘informatics’. Articles about pigs, on the other hand, tended to be about ‘bioinformatics’ (Table 8). For articles that used the term ‘informatics’, there were ~2.1 species mentioned per article. For articles that used the term ‘bioinformatics’, there were ~1.4 species mentioned per article.
Table 8. General characteristics of 1093 included articles containing terms related to ‘big data’, ‘informatics’, and ‘bioinformatics’ in the animal health and veterinary medicine literature

a May exceed n because articles may contain multiple conceptual terms.
b May exceed 1093 because articles may have been classified into multiple categories.
Five of six geographic regions produced articles about ‘big data’. Articles about ‘informatics’ and ‘bioinformatics’ have been published in all geographic regions. North America and Europe had similar numbers of publications for ‘informatics’ and ‘bioinformatics’; however, most publications from Asia were about ‘bioinformatics’.
Articles about cattle were most common across all geographic regions except Asia. Articles about pigs were the most common in Asia (Fig. 4). To determine whether studies about pigs conducted in Asia contributed significantly to the counts for articles that used the term ‘bioinformatics’, we present data specific to pigs in Table 9. Articles describing studies performed in Asia or with the first authors based in Asia overwhelmingly used the term ‘bioinformatics’ more than ‘big data’ and ‘informatics’. Articles describing studies performed in North America or with first authors based in North America also used the term ‘bioinformatics’ more often, however, the difference was not as pronounced.

Fig. 4. Number of articles about each species, by geographic region.
Table 9. Number of ‘big data’ or ‘informatics’ articles versus ‘bioinformatics’ articles, by geographic region, for studies related to swine populations

Most of the articles had first authors with affiliations in ‘veterinary medicine and animal health’ (Table 8). ‘Informatics’ articles more frequently had first authors from ‘physical sciences’ (29 versus 6), ‘computer science and information technology’ (15 versus 0), and ‘social sciences’ (24 versus 1) than ‘bioinformatics’ articles.
The two most common types of journals of publication were ‘biological’ (484) and ‘veterinary medicine and animal health’ (355) (Table 8). ‘Veterinary medicine and animal health’ was the most common journal of publication for ‘big data’ and ‘informatics’ articles. ‘Biological’ was the most common journal of publication for ‘bioinformatics’ articles. ‘Informatics’ articles were more frequently published in ‘physical sciences’ (25 versus 3) and ‘computer science and information technology’ (49 versus 2) journals than ‘bioinformatics’. ‘Bioinformatics’ articles more frequently published to ‘bioinformatics’ journals (81 versus 6) than ‘informatics’ journals.
Primary studies described in ‘bioinformatics’ articles tended to be conducted at the ‘animal genes, proteins, metabolites’ level (354/589; 60%) (Table 10). ‘Informatics’ articles describing primary studies tended to be conducted at the ‘animal bacteria, virus, parasite, fungus’ level (121/326; 37%) and ‘animal’ level (67/326; 21%) or were ‘software, analytical technique, lab technique development/validation studies’ (87/326; 27%). Primary studies described by ‘informatics’ articles focused more on the ‘effects of animals on environment’ (35/326; 11%) than those described by ‘bioinformatics’ articles (2/589; 0.3%).
Table 10. Data classification of 918 primary studies into study level, study type and data sources
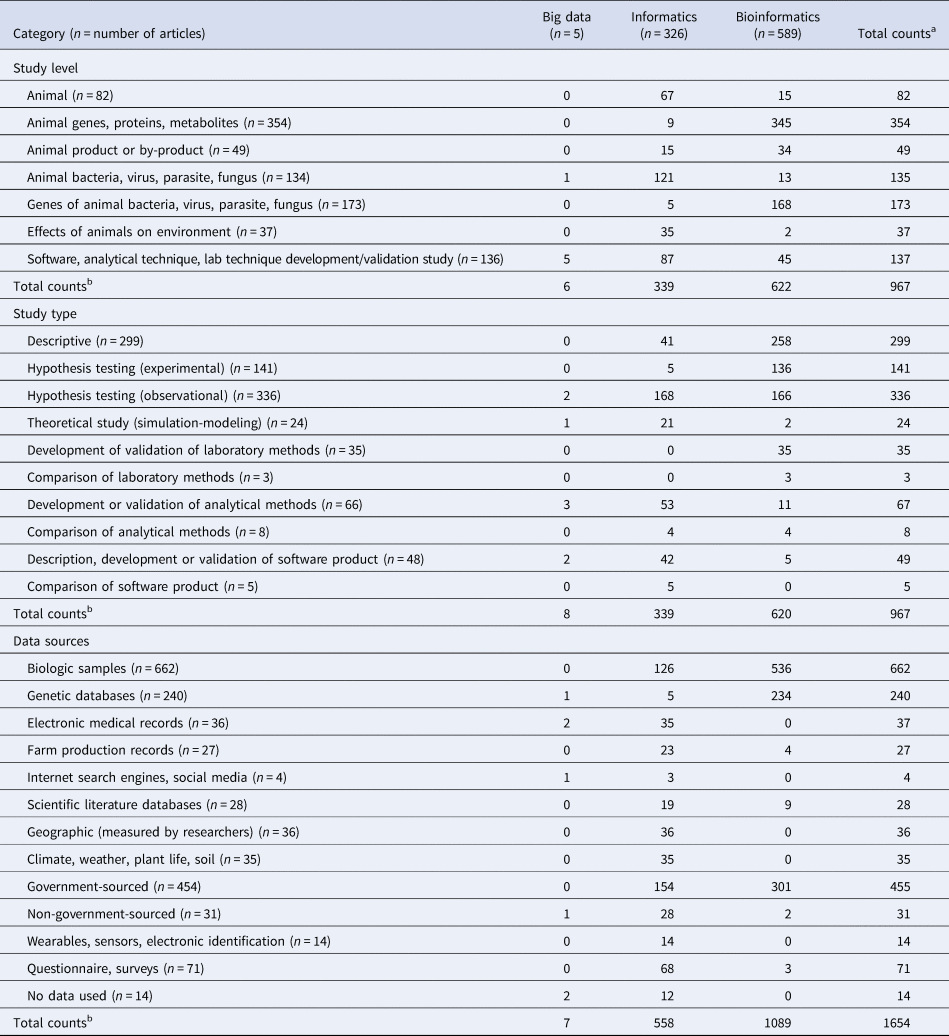
a May exceed n because articles may contain multiple conceptual terms.
b Total may exceed 918 because articles may have been classified into multiple categories.
‘Bioinformatics’ articles also described ‘software, analytical technique, lab technique development/validation studies’ (45/589; 8%) (Table 10). Of these articles, ‘bioinformatics’ articles were largely about laboratory techniques while ‘informatics’ articles were about analytical techniques and software.
Primary studies classified as ‘hypothesis testing (observational)’ were more frequently in ‘informatics’ articles (168/326; 52%) than in ‘bioinformatics’ articles (166/589; 28%) (Table 10). Primary studies classified as ‘hypothesis testing (experimental)’ were more frequently in ‘bioinformatics’ articles (136/589; 23%) than ‘informatics’ articles (5/326; 2%). ‘Bioinformatics’ studies (258/589; 44%) were also more often classified as ‘descriptive’ than ‘informatics’ studies (41/326; 13%).
‘Bioinformatics’ primary studies tended to use genetic databases (234/589; 40%) and government-sourced databases (301/589; 51%) (Table 10). Of the 301 ‘bioinformatics’ primary studies that used government-sourced data, 89% (269/301) of those databases were NCBI (National Center for Biotechnology Information), GenBank or DAVID (Database for Annotation, Visualization and Integrated Discovery). ‘Informatics’ primary studies tended to use non-genetic sources of data. Although ‘informatics’ primary studies used biologic samples, they also used other data sources, e.g. electronic medical records, farm production records, internet search engines, climate data, questionnaires, and wearables/sensors. Forty-seven percent (154/326) of ‘informatics’ primary studies used government-sourced data; however, only seven of these data sources were NCBI, GenBank or DAVID.
Discussion
Summary of evidence
Although research in ‘big data’, ‘informatics’, and ‘bioinformatics’ has been growing in human medicine, with the exception of ‘bioinformatics’, we currently do not see a similar growth in the animal health and veterinary medical research literature. There appears to be a lag in the production of ‘big data’ articles in veterinary medicine and animal health compared to human health (Andreu-Perez et al., Reference Andreu-Perez, Poon, Merrifield, Wong and Yang2015).
The use of the term ‘big data’ is relatively recent and uncommon, perhaps due to the rapidly evolving definition of what big data is (Natarajan et al., Reference Natarajan, Frenzel and Smaltz2017). The greater number of reviews compared to primary studies would suggest that the potential of big data in veterinary medicine and animal health is still being explored (see Table 6). Researchers interested in learning about ‘big data’ in veterinary medicine and animal health may need to search other bodies of literature.
An effective definition needs to address what characteristics are necessary for a study to be considered a big data study. The development of such a definition could be addressed by a systematic review. Big data is often characterized by the Vs, e.g. volume, velocity and variety (Laney, Reference Laney2001; Schroeck et al., Reference Schroeck, Shockley, Smart, Romero-Morales and Tufano2012). Although data volume remains a necessary component for the approach to be considered a big data approach, the latter two components are becoming equally or more important (Natarajan et al., Reference Natarajan, Frenzel and Smaltz2017), a trend which has been attributed to more widespread availability of large volumes of data. It has also been argued that the relationships between the three Vs of big data should be examined in order to declare data as ‘big’ (Natarajan et al., Reference Natarajan, Frenzel and Smaltz2017). This complexity, when coupled with the relatively stringent initial definition of big data, and the definition's now evolving nature (Ylijoki and Porras, Reference Ylijoki and Porras2016) could have influenced, in different ways, the low number of studies declared as using a big data approach in the veterinary medical and animal health literature. First, it is possible that research conducted in this area did not fit the contemporary definition, even if loosely defined, of big data. Second, it is possible that published literature addresses only one component of big data (e.g predictive analytics) in isolation from other components and therefore cannot be, and was not considered, an approach to research consistent with big data. Only when combined with other components, do these isolated parts form an approach to big data. This integration may be beyond the scope of individual research contributions. Finally, it is also possible that the big data research has been conducted, but has not been communicated under the name ‘big data’, or the approach has been utilized not for the purposes of publication but for product or process development within specific organizations, e.g. livestock commodity groups that are used by industry and researchers. Research about one component of big data and big data research used within specific organizations, if published at all, may only be found within specialized literature.
Another possible explanation for why ‘big data’ was uncommon is that existing big datasets in veterinary medicine and animal health, like human health, may have been extracted from data sources that were not designed to answer questions currently held by researchers (Lazer et al., Reference Lazer, Kennedy, King and Vespignani2014; Chen and Asch, Reference Chen and Asch2017), making it difficult to conduct studies that use big data. This supports the notion that pipelines must be created to ‘turn big data into “smart data”’ (VanderWaal et al., Reference VanderWaal, Morrison, Neuhauser, Vilalta and Perez2017). Further, large datasets that do exist may contain meaningful information that does not answer predefined research questions. Unsupervised machine learning and pattern recognition algorithms may shed light on what is hidden in these datasets, by revealing patterns that were not expected. Such methodologies may be relatively new to animal health and veterinary medicine. Finally, big datasets may simply be difficult for researchers to acquire.
‘Informatics’ studies tend to use a variety of data sources, such as ‘geospatial information systems’, government databases, scientific literature databases, and electronic medical/production records, that have been described as being or becoming big data (VanderWaal et al., Reference VanderWaal, Morrison, Neuhauser, Vilalta and Perez2017). Remote sensing technologies have existed in dairies since the 1980s, which would explain the large number of cattle studies classified as ‘informatics’ studies (Rutten et al., Reference Rutten, Velthuis, Steeneveld and Hogeveen2013).
Despite an overlap in the definitions of ‘informatics’ and ‘bioinformatics’, there is a strong distinction in the literature. ‘Bioinformatics’ studies were about genes, amino acids, and proteins while ‘informatics’ studies were about an organism or pathogen (e.g. animal, bacteria, and virus). ‘Bioinformatics’ studies also tended to about laboratory techniques while ‘informatics’ studies tended to be about analytical techniques and software. Bioinformatic laboratory techniques may contain an analytical component; however, if this was not stated explicitly, the study was not classified as being about analytical techniques. Genetic datasets (including genomic and metagenomic datasets) are often considered large, and multiple sources of data may be used (e.g. biological samples, government databases). However, once collected for a research study, the genetic dataset does not change. This lack of velocity may explain why most ‘bioinformatics’ articles do not use the term ‘big data’.
Limitations
The literature search was limited to the conceptual terms ‘big data’, ‘informatics’, and ‘bioinformatics’. A more complete picture of the concepts of big data and informatics may require a search of a larger list of terms. For instance, articles describing studies that used big data may be better identified by the names of analytical techniques designed specifically for big data. Similarly, many articles about informatics or big data may have been excluded for not using those specific words. Research conducted using data sources such as animal industry datasets (e.g. performance, health, and breeding records) as well as data from animal (and human) health surveillance systems may be relevant to ‘informatics’ research. Further, searches using words such as ‘robotic milkers’, ‘wearable sensors’, and ‘electronic medical records’ may also have provided articles relevant to ‘informatics’. Although the search yielded a large number of publications, it is possible that the search would have been more complete by including these terms in the search. The authors began with a literature search with a larger list of conceptual terms; however, the number of articles returned was extremely large (data not shown).
The literature search was limited to English abstracts. Articles with English abstracts but non-English full-text were excluded from the study. Articles that used the terms ‘big data’, ‘informatics’, and ‘bioinformatics’ in non-English languages would not have been captured potentially biasing the study.
Conclusions
‘Big data’ was an uncommon term. ‘Bioinformatics’ was the most common term. There were more ‘informatics’ articles about small animals and livestock with unspecified production systems (e.g. cattle, poultry) than ‘bioinformatics’ articles. A large number of ‘pig’ articles contributed to ‘bioinformatics’ studies.
All geographic regions produced literature using the terms ‘informatics’ or ‘bioinformatics’. Two geographic regions (South America, Africa) did not produce literature using the term ‘big data’. Asia produced the most literature using the term ‘bioinformatics’. Articles about pigs contributed heavily to the ‘bioinformatics’ articles from Asia.
While most articles had first author affiliations in ‘veterinary medicine and animal health’, a higher proportion of ‘informatics’ articles had affiliations that were not veterinary/animal, medical/health or biologically related. ‘Big data’ and ‘informatics articles’ were more often published in ‘veterinary medicine and animal health’ journals. ‘Bioinformatics’ articles were more often published in ‘biological’ journals.
‘Bioinformatics’ studies tended to be conducted at the gene level. ‘Informatics’ studies tended to be conducted at the ‘animal’ or ‘animal bacteria, virus, parasite, fungus’ level. ‘Informatics’ studies also tended to examine analytical techniques and software. ‘Bioinformatics’ studies tended to examine laboratory techniques. ‘Informatics’ studies were often observational. Experiments were more common in ‘bioinformatics’ studies.
‘Bioinformatics’ studies used biologic samples, genetic databases, and government databases. ‘Informatics’ studies used a wider variety of data sources (e.g. ‘electronic medical records’, ‘farm production records’, ‘scientific literature databases’, ‘geographic’, ‘wearables, sensors, electronic identification’).
The definition of big data has evolved rapidly and should be taken into account when describing research. As big data research is more common in human medicine, it may serve as a model for researchers in animal health and veterinary medicine. Techniques such as unsupervised machine learning and pattern recognition algorithms may uncover unrecognized associations within big datasets.
Finally, as with any study, it is important to focus resources on collecting and analyzing data in a way that meets the research objectives.
Acknowledgements
This research was undertaken thanks in part to:
• IDEXX Laboratories,
• Funding from the Canada First Research Excellence Fund through the Food from Thought program at the University of Guelph,
• International Graduate Tuition Scholarships at the University of Guelph,
• International Doctoral Tuition Scholarships at the University of Guelph,
• The Natural Sciences and Engineering Research Council's Undergraduate Summer Research Awards, and
• Undergraduate Research Assistantships at the University of Guelph.
We thank Erin McGill, Vivienne Steele and Inthuja Selvaratnam for their assistance with data extraction.