Lifestyle approaches to delaying cognitive decline and the incidence of dementia are of considerable interest; delaying disease onset and progression by 1 year would result in approximately 9·2 million fewer cases worldwide by 2050( Reference Brookmeyer, Johnson and Ziegler-Graham 1 ). Dietary intake is a modifiable exposure that potentially influences cognitive status in older age via nutritional mechanisms that contribute to brain health and functioning( Reference Gómez-Pinilla 2 ). Dietary intake is also implicated in the aetiologies of CVD, diabetes and stroke, all of which are risk factors for unfavourable later-life cognitive outcomes( Reference Spiro and Brady 3 ).
Substantial evidence is accruing for both protective and detrimental effects of dietary patterns on older-age cognition( Reference Gu and Scarmeas 4 ). The Mediterranean diet, together with dietary patterns that share a similar profile (so those rich in vegetables, fruits, grains and fish), have been reported to be protective against the risk of dementia and cognitive decline( Reference Scarmeas, Stern and Tang 5 – Reference Wengreen, Neilson and Munger 10 ), and in cross-sectional studies, they have been shown to be associated with better cognitive performance( Reference Wengreen, Neilson and Munger 10 – Reference Samieri, Okereke and Devore 15 ). Conversely, dietary patterns defined by processed foods high in saturated fats and sugar predict poorer cognitive functioning( Reference Akbaraly, Singh-Manoux and Marmot 12 , Reference Torres, Lautenschlager and Wattanapenpaiboon 16 ).
A major challenge when determining dietary strategies for the maintenance of cognitive health is the appropriate timing of any intervention. A large portion of the intellectual variability that exists between older people is present by childhood( Reference Deary, Whiteman and Starr 17 ), and cognitive ageing itself begins during early adulthood( Reference Salthouse 18 ). The brain pathology that underpins dementia accumulates over many years before clinical symptoms become apparent( Reference Sperling, Aisen and Beckett 19 ), and is evident in mid-life for those that are genetically predisposed to Alzheimer's disease( Reference Xiong, Roe and Buckles 20 ). Therefore, the impact of diet on older-age cognition is likely to be a function of intake over the lifetime, with possible sensitive periods when dietary exposures are particularly salient( Reference Benton 21 ).
Only one known study has examined dietary patterns from an earlier life period as predictors of older-age cognitive function. In the SU.VI.MAX 2 (Supplementation with Antioxidant Vitamins and Minerals 2)( Reference Kesse-Guyot, Amieva and Castetbon 22 ) study, a ‘healthy’ pattern consumed at mid-life, defined by fruit, whole grains, vegetables, and negatively correlated with meat and poultry, was associated with better cognitive scores 13 years later( Reference Kesse-Guyot, Andreeva and Jeandel 23 ). Currently, however, there are no cohort studies with comprehensive dietary data over multiple decades to assess the ‘life course approach’ to cognitive ageing( Reference Richards and Deary 24 ) in the context of dietary intake.
Cognitively healthy older people can recall general consumption frequencies for individual foods, and food groups, over multiple life periods with reasonable reliability( Reference Hosking, Danthiir and Nettelbeck 25 ). Additionally, dietary recall of earlier intake has been demonstrated to capture dietary change, and to be associated with relevant demographic and health outcomes( Reference Hosking and Danthiir 26 ). Lifetime dietary recall, therefore, offers a novel and practical solution to examining dietary intake and older-age cognition within a life-course framework.
The present study examined recalled dietary patterns from the life periods of childhood, early adulthood, adulthood and middle age as predictors of comprehensively measured cognitive outcomes in an older sample of cognitively healthy adults.
Methods
Design and participants
Participants were a subsample of the EPOCH (Older People, Omega-3 and Cognitive Health) trial; an 18-month double-blind, randomised, controlled trial of n-3 fish oil on cognitive functioning in a community-dwelling population of older adults in Adelaide, South Australia. Recruitment commenced in June 2007 and baseline assessment was completed by December 2008. A detailed account of the study's design and measures can be found in the study protocol( Reference Danthiir, Burns and Nettelbeck 27 ). Participants were older adults aged 65–90 years, who had a score >22/27 on a modified telephone-administered Mini Mental State Examination( Reference Newkirk, Kim and Thompson 28 ), and lived in the greater metropolitan area of Adelaide, South Australia. Of the 390 eligible EPOCH trial members, 352 (90 %) contributed lifetime dietary data. The study was conducted in compliance with the Declaration of Helsinki and followed Good Clinical Research Practice Guidelines. All experimental procedures were approved by the Human Research Ethics Committee of CSIRO Animal, Food and Health Sciences. Informed consent was obtained from all participants. The present study reports the cross-sectional relationships between dietary patterns, assessed by recall of dietary intake across the lifetime, and baseline cognitive measures from the EPOCH trial( Reference Danthiir, Burns and Nettelbeck 27 ). All analyses were adjusted for demographic, lifestyle and physiological variables.
Procedure
Participants completed multiple questionnaires to assess demographic, lifestyle, health and well-being factors prior to the cognitive testing sessions held at the study centre (CSIRO Animal, Food and Health Sciences). The sessions were conducted in groups of up to seven people. A fasted venous blood sample was collected, together with measurements of height, weight and blood pressure. After consuming a standardised breakfast, participants undertook the cognitive test battery that was administered by two trained research staff. The battery was divided into computer-based tasks and pencil and paper-based tasks, and took approximately 4 h to complete, with 10 min breaks given every hour.
At 3 months of post-baseline assessment, participants collected their quarterly supply of study capsules from the study centre and provided notification of any medication changes. During this visit, they were given the opportunity to complete the self-administered Lifetime Diet Questionnaire (LDQ)( Reference Hosking, Danthiir and Nettelbeck 25 ) at home and return it to the study centre by post.
Lifetime diet
Lifetime diet was assessed by the LDQ( Reference Hosking, Danthiir and Nettelbeck 25 ), a measure of historical dietary intake covering all life periods from childhood to older age. The questionnaire's rationale and design have been detailed previously, together with its reproducibility in an equivalent population: average reproducibility across the life periods was 0·81 and the average weighted κ was 0·49 for recall agreement between food groups( Reference Hosking, Danthiir and Nettelbeck 25 ). Although the LDQ has not been validated against dietary records, support for the questionnaire's validity was demonstrated by the plausible associations found between early-life dietary intake, as measured by the LDQ, and both demographic and late-life cardiovascular outcomes( Reference Hosking and Danthiir 26 ). A brief description of the measuring tool is as follows. The questionnaire distinguishes between the life periods of childhood (5–18 years), early adulthood (19–30 years), adulthood (31–45 years) and middle age (46–60 years), and uses a non-quantitative approach; response options are on a four-point scale: ‘rarely/never’; ‘two to three times a month’; ‘two to three times a week’; ‘daily’. The food groups and their items remain the same for each life period with exceptions being food items that were unlikely to have been consumed during a given period. The number of items ranged from seventy-four in childhood to seventy-nine in early adulthood, with adulthood and middle age each having seventy-eight items. Physical activity levels across all life periods were also assessed as a proxy measure for energy expenditure. Level of physical activity associated with occupation was categorised as ‘little’, ‘some’, ‘frequent’ or ‘heavy and frequent’. All other physical activities were assessed as the frequency per week of (any or all) vigorous, moderate and light physical activity.
The variables representing lifetime dietary intake in the present study were dietary pattern factor scores from each distinct life period of the LDQ. The dietary pattern analysis of the LDQ has been fully described elsewhere( Reference Hosking and Danthiir 26 ), so it is only briefly described here. Exploratory factor analysis for ordinal variables was used to extract dietary factors from each life period of the LDQ using M-plus( Reference Muthén and Muthén 29 ). Each dietary factor was defined by the individual food items with the highest loadings on it( Reference Hu 30 ). In addition, these factors explained the following percentages of the shared variance among the questionnaire items for each life period: 31·6 % in childhood; 31·87 % in early adulthood; 26·93 % in adulthood; 26·12 % in middle age. The extracted dietary factors with their ten highest-loading food items are presented in Table 1. These items do not fully define the factors; the tables of all individual food items and their loadings >0·3 for each factor have been fully reported previously( Reference Hosking and Danthiir 26 ).
Table 1 Dietary patterns extracted from the Lifetime Diet Questionnaire (LDQ) and the ten highest-loading food items on each pattern
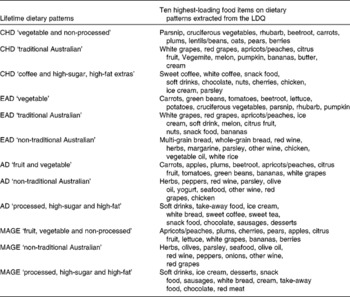
CHD, childhood; EAD, early adulthood; AD, adulthood; MAGE, middle age.
The naming of the dietary factors indicated the foods, or types of foods, that defined the factor or (in the case of ‘traditional Australian’ or ‘non-traditional Australian’) differentiated between the types of diet based on their social determinants( Reference Newby and Tucker 31 ). Dietary pattern scores were calculated by weighting the individuals' frequency of consumption for each item by its loading on each of the dietary patterns and then summing the items( Reference Moeller, Reedy and Millen 32 ). These scores were used as predictor variables in the present study; a higher dietary pattern score equated to greater consumption with the exception of the childhood ‘coffee and high-sugar, high-fat extras’ pattern. For this pattern, all food item loadings were negative, so its dietary pattern score was reverse coded.
Cognitive outcomes
The cognitive test battery used in the EPOCH trial took a comprehensive approach to cognitive functioning with the inclusion of tasks to assess multiple cognitive domains. In particular, a number of cognitive tests were chosen as indicators of cognitive speed constructs( Reference Danthiir, Wilhelm and Roberts 33 ), given that cognitive speed is a sensitive indicator, vulnerable to the effects of ageing, and arguably fundamental to higher-order abilities( Reference Salthouse 34 ). The design, composition and statistical analyses of this test battery have been fully described and reported in the EPOCH trial protocol( Reference Danthiir, Burns and Nettelbeck 27 ). In total, six speed-based and four accuracy-based cognitive constructs were derived from two confirmatory factor analytical models of twenty-six tests. These constructs were found to be empirically distinct and the tests that defined them are listed as follows:
Speed-based constructs
-
(1) Perceptual speed (Finding As, Number Comparison, Digit–Symbol Coding)
-
(2) Simple/choice reaction time (Simple, Two-choice, Four-choice)
-
(3) Speed of memory scanning (Number and Letter Memory Scanning)
-
(4) Reasoning speed (Number and Letter Odd-Man-Out)
-
(5) Inhibition (Simon task, Spatial and Colour Stroop)
-
(6) Psychomotor speed (Simple, Up and Diagonal Movement Tasks)
Accuracy-based constructs
-
(1) Working memory (Operation Span, Counting Span)
-
(2) Retrieval fluency (Word Endings, Categories)
-
(3) Short-term memory (Face Recognition, Word List Recall, Paired Associates)
-
(4) Reasoning (Raven's Standard Progressive Matrices, Letter Sets, Everyday Problem Solving)
Constructs derived by the confirmatory factor analysis exclude error variance and test-specific variance, and so represent only the shared variance between the two or more tests that define them. Construct validity and the reliability of assessment is thereby increased( Reference Danthiir, Burns and Nettelbeck 27 ). Factor scores were estimated for the cognitive constructs in the same manner as for the dietary patterns; scores on each of the cognitive test variables were weighted by their loadings on the relevant factor and then summed.
Other variables
The acknowledged correlates of older-age cognitive status were assessed, in addition to lifestyle or health and well-being outcomes that could plausibly be related to lifetime dietary patterns. These measures were obtained during the course of the EPOCH trial. The full details for these measures have been described in the trial protocol( Reference Danthiir, Burns and Nettelbeck 27 ); however, the variables used in the present analyses are listed below.
Demographic variables
Demographic variables were as follows: age; sex; years of education (school, tertiary and vocational); native language (a dichotomised variable representing English or other); a four-level parental income variable (constructed from parental occupation); current income level (a nineteen-category variable following the Australian Census 2006).
Health/lifestyle variables
Health/lifestyle variables included the following: years of smoking (calculated as pack-years); BMI (kg/m2); systolic blood pressure; depressive symptoms (assessed by the Centre for Epidemiological Studies Depression Scale( Reference Radloff 35 )); medication use for cardiac conditions, hypertension, cholesterol levels and depression (dichotomous variables representing usage or non-usage); past physical activity (assessed by the LDQ( Reference Hosking, Danthiir and Nettelbeck 25 )); current physical activity (assessed by the Yale Physical Activity Scale( Reference Dipietro, Caspersen and Ostfeld 36 )).
Blood-based markers
Blood-based markers were as follows: apoE genotype (dichotomised as the presence or absence of the apoE ɛ4 allele); plasma homocysteine; LDL-cholesterol; HDL-cholesterol.
Present and past dietary covariates
Recall of past dietary intake is known to be confounded and biased by current dietary measures( Reference Friedenreich, Slimani and Riboli 37 ); therefore, three exploratory factor analysis-derived pattern scores from the baseline response frequencies of the Dietary Questionnaire for Epidemiological Studies( Reference Giles and Ireland 38 ) were included as covariates. In addition, all twelve past dietary factors were interdependent, so in order to allow the identification of any unique contribution by a past dietary pattern, principal component analyses were employed to create a set of four unrelated past dietary variables that accounted for the shared variance among all the other past dietary patterns (not including the pattern focused on as the main predictor in each model). Details of both the present and past dietary pattern analyses have been reported previously( Reference Hosking and Danthiir 26 ).
Missing data
For the LDQ, those who had whole life periods (n 9) or >80 % of responses missing across any life periods (n 3) were excluded from missing value analysis. The remaining missing data in the LDQ were of two types: item non-response (mean per person 6·43 (sd 10·79)) and multiple consumption frequencies reported for a food item (mean per person 1·06 (sd 0·55)). The explanation and rationale for the treatment of missing data in the LDQ have been described previously( Reference Hosking and Danthiir 26 ); all missing responses were imputed using the EM (Expectation Maximization)( Reference Dempster, Laird and Rubin 39 ) procedure implemented with Missing Value Analysis in the SPSS for Windows statistical package version 17.0.1 (SPSS, Inc.). There were minimal missing values for the cognitive data, but these were estimated by the Full-Information Maximum-Likelihood method as part of the confirmatory factor analysis of the test battery( Reference Danthiir, Burns and Nettelbeck 27 ). The small proportion of values missing on the continuous covariate data was estimated using the EM procedure; categorical data with missing values were dealt with by pairwise deletion in the analyses.
Regression models
Multiple linear regression models were used to examine the prediction of baseline cognitive performance by individual dietary patterns extracted from the life periods of the LDQ. Separate models were performed with each cognitive factor score as the outcome variable predicted by each of the dietary pattern scores separately. The models were progressively adjusted for three blocks of covariate entries described below.
Block 1
Block 1 was adjusted for age, sex, years of education, smoking history, income level (parents' income for the two earlier life periods and current income for the two later life periods), English as native language, presence of the apoE ɛ4 allele and current dietary intake.
Block 2
In block 2, the four-component scores represented past dietary intake excluding the predictor period of interest.
Block 3
Block 3 was adjusted for systolic blood pressure, HDL-cholesterol, LDL-cholesterol, homocysteine, CES-D score, and medication use for cardiac conditions, hypertension, cholesterol levels and depression. Variables in this block were considered as potential mechanisms for the associations between lifetime dietary patterns and cognitive outcomes.
In total, there were twenty-four predictors in each model. With a total sample size of 352 and α = 0·05 (two-tailed), there was a power of 0·8 to detect an f 2 effect size of 0·023 for the contribution of a single predictor (i.e. the dietary pattern)( Reference Faul, Erdfelder and Lang 40 ).
Examination of the regression diagnostics in preliminary models demonstrated no violation of assumptions; in particular, high multicollinearity was not apparent among the dietary predictors (past and present), so the contribution of individual dietary patterns could be feasibly evaluated.
Alternative analyses were carried out without adjusting for current dietary intake; previous findings have indicated that current dietary patterns accounted for incrementally increasing amounts of variance in past dietary patterns across the lifetime( Reference Hosking and Danthiir 26 ), so in later life periods, it was possible that past dietary effects were being confounded by current dietary intake.
Results
Descriptive statistics for the EPOCH sample are presented in Table 2.
Table 2 Descriptive statistics for the EPOCH (Older People, Omega-3 and Cognitive Health) sample who undertook the Lifetime Diet Questionnaire (Mean values and standard deviations)
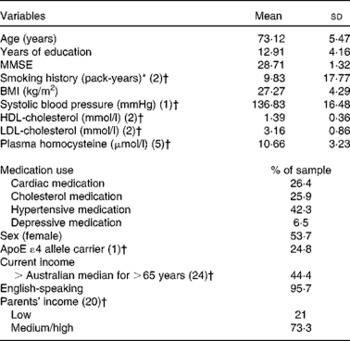
MMSE, Mini Mental State Examination.
* Pack-years = (cigarettes/d × years of smoking)/20.
† Missing values.
A total of 352 individuals (53·7 % female) completed the LDQ, with an age range of 65–91 years (mean 73·12 (sd 5·47)). Of the study participants, 26·4 % of men and 37·6 % of women completed schooling to year 10 and 22·7 % of men and 21·8 % of women completed schooling to year 12. A bachelor's degree was held by 8·2 % of the sample. Mann–Whitney non-parametric tests demonstrated that there were no significant differences between the LDQ participants and non-participants from the EPOCH cohort in any of the ten cognitive constructs or in terms of age (U= 6714·00, P= 0·823), level of education (U= 6378·00, P= 0·454) and the baseline Mini Mental State Examination score (U= 6582·50, P= 0·891).
Individual lifetime dietary patterns as predictors of cognitive constructs
Tables 3 and 4 present the standardised regression weights and their P values for the relationships between each lifetime dietary pattern and the cognitive factors. After adjustment for current dietary intake, demographic and lifestyle variables, in addition to the presence of the apoE ɛ4 allele (model 1), the childhood ‘vegetable and non-processed’ pattern negatively predicted perceptual speed, simple/choice reaction time and working memory. The childhood ‘coffee and high-sugar, high-fat extras’ pattern negatively predicted simple/choice reaction time, and strongly and significantly negatively predicted all accuracy-based constructs. The remainder of significant associations for model 1 were for the accuracy-based rather than the speed-based constructs. Working memory was negatively predicted by the early-adulthood ‘vegetable’ and ‘non-traditional’ patterns and the middle-age ‘fruit, vegetable and non-processed’ pattern. Short-term memory was negatively predicted by the ‘non-traditional Australian’ pattern in both early adulthood and adulthood. When current dietary patterns were excluded as covariates, no additional associations were found between any of the life periods and the cognitive outcomes (results not shown).
Table 3 Lifetime dietary factors as predictors of the speed-based constructs* (Standardised regression weights (β) with their P values)
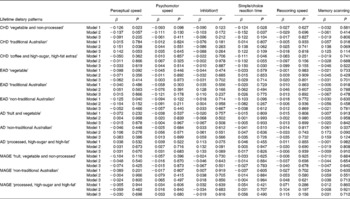
CHD, childhood; EAD, early adulthood; AD, adulthood; MAGE, middle age.
* Model 1 adjusted for current diet, age, sex, years of education, smoking history, income level (parents' income for the two earlier life periods and current income for the two later life periods), English as native language and apoE ɛ4 allele; model 2 adjusted for model 1 variables plus other past dietary patterns; model 3 adjusted for model 1 and model 2 variables plus mechanistic health-related variables.
† Reversed β sign for inhibition, so a higher score equals better performance.
Table 4 Lifetime dietary factors as predictors of the accuracy-based constructs* (Standardised regression weights (β) with their P values)
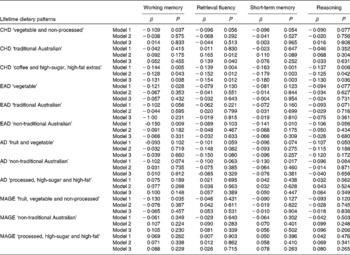
CHD, childhood; EAD, early adulthood; AD, adulthood; MAGE, middle age.
* Model 1 adjusted for current diet, age, sex, years of education, smoking history, income level (parents' income for the two earlier life periods and current income for the two later life periods), English as native language and apoE ɛ4 allele; model 2 adjusted for model 1 variables plus other past dietary patterns; model 3 adjusted for model 1 and model 2 variables plus mechanistic health-related variables.
Model 2 additionally controlled for the variance shared between the dietary pattern of interest and the other lifetime patterns. Significant negative associations remained between the childhood ‘vegetable and non-processed’ pattern and simple/choice reaction time, and between the childhood ‘coffee and high-sugar, high-fat extras’ pattern and all accuracy-based constructs. In this model, two additional relationships reached significance; the childhood ‘traditional Australian’ pattern positively predicted perceptual speed and retrieval fluency. As a final step, the mechanistic health-related variables were included in all models. The childhood ‘traditional Australian’ pattern remained positively associated with retrieval fluency and the childhood ‘coffee and high-sugar, high-fat extras’ pattern remained negatively associated with all the accuracy-based constructs, and reached significance for simple/choice reaction time. The strength of significant associations between model 2 and model 3 changed very little. No other associations remained significant or reached significance.
Discussion
In models adjusted for relevant demographic covariates, the apoE ɛ4 allele and current dietary intake, lifetime dietary patterns predicted cognitive performance in normally functioning older adults. In models additionally adjusted for all other dietary patterns, the associations that remained were for the period of childhood only, with all the three patterns from this period demonstrating associations with cognitive performance in older age.
Adequate nutrition is essential for cognitive development in childhood( Reference Benton 41 – Reference Heys, Jiang and Schooling 43 ), and cognitive ability in childhood is strongly related to cognitive ability across the lifetime( Reference Deary, Whiteman and Starr 17 ). The period of childhood for these participants spanned varying periods of time during the years of the Great Depression, World War Two and the early post-war years. During this period, there were food shortages and food rationing; the availability of animal products was limited and reliance on garden-grown vegetables was promoted by the government to combat food shortages( Reference Gaynor 44 ). The childhood ‘vegetable and non-processed’ pattern could be a marker for early-life nutritional deprivation that was not captured by adjusting for parental income levels, given that both rich and poor alike were subject to limited food availability during these years( Reference Collingham 45 ). This pattern was defined by garden-grown vegetables, legumes and an absence of animal products with the exception of oily fish. (Given the era of recall, the oily fish consumed is likely to have been tinned sardines or salmon; fish consumption at that time was relatively low in Australia compared with other nations and the fish industry was in its infancy)( 46 ). The ‘vegetable and non-processed’ pattern was negatively associated with all cognitive constructs, and after adjustment, it remained a negative predictor of simple/choice reaction time.
Food shortages during the war years did not necessarily have an equal impact across the population, with those living in some farming communities having greater access to a wider range, and possibly a greater quantity, of food( Reference Gregory 47 ), as would have younger participants whose childhood dietary recall period extended into the 1950s when food production and supply had recovered from war-time austerity measures( Reference Truswell and Wahlqvist 48 ). The childhood ‘traditional Australian’ pattern was defined by a greater variety of foods, and a higher score on this pattern may represent more adequate nutritional intake during childhood in comparison with the more frugal ‘vegetable and non-processed’ pattern. In adjusted models, this pattern positively predicted perceptual speed and retrieval fluency, although for perceptual speed, the association did not remain significant once the mechanistic health-related variables were controlled for.
Consumption of a processed pattern in childhood contributes to childhood obesity that has been shown to predict higher blood pressure in adulthood( Reference Lawlor and Smith 49 ). Hypertension is a well-established risk factor for cognitive decline and dementia, and has been demonstrated to predict vascular brain injury in adults as early as the fourth decade( Reference Maillard, Seshadri and Beiser 50 ). Hypertension during this particular life period, therefore, provides a potential pathway by which a childhood diet high in fat and sugary foods may have an impact on older-age cognitive performance. However, it is interesting that when systolic blood pressure and hypertensive medications were adjusted for in the final model, the associations were not attenuated. It is possible that later lifestyle modification may have reduced adulthood hypertension, so it did not continue into older age. Alternatively, higher consumption of a ‘processed’ (high fat and sugar content) pattern in early childhood has been associated with lower overall intelligence quotient, and in particular verbal intelligence quotient, assessed at 8·5 years( Reference Northstone, Joinson and Emmett 51 ). Early-life childhood intelligence measures strongly predict older-age cognitive functioning( Reference Deary, Whiteman and Starr 17 ) and for participants in the present study, greater consumption of the ‘coffee and high-sugar, high-fat extra’ pattern in childhood may have had a negative impact on cognitive performance via its influence on the development of childhood cognitive ability.
A comparison with the findings of others is difficult because very few studies have been able to test the associations between dietary patterns from the relatively distant past (greater than a decade previously) and late-life cognitive outcomes. Mid-life consumption of a healthy pattern in the SU.VI.MAX 2 cohort has been shown to be positively associated with global cognitive functioning in addition to verbal memory 13 years later( Reference Kesse-Guyot, Andreeva and Jeandel 23 ). Unexpectedly, in the present study, there were no comparable associations between the dietary pattern scores for middle age and any of the cognitive constructs in the adjusted models. It was considered possible that in these models, adjusting for current intake suppressed associations, given the previously demonstrated strong relationship between current and mid-life dietary patterns in this sample( Reference Hosking and Danthiir 26 ). Supplementary analyses, however, demonstrated that this was not the case; when current diet was excluded, no further associations emerged. It could be argued that dietary patterns extracted from recalled intake over such a long period are of questionable validity; however, the LDQ was designed around the cognitive strategies known to underpin long-term dietary recalls, such as the use of generic food memories rather than detailed quantitative information( Reference Smith 52 ) and life-period-cue questions to contextualise dietary memories( Reference Friedenreich 53 ). In addition, plausible associations between the lifetime dietary patterns and both demographic and cardiovascular variables have been demonstrated previously in this sample( Reference Hosking and Danthiir 26 ), which supports the validity of both long-term dietary recall and the dietary patterns extracted.
One of the main confounders of past diet memory is the impact of current diet on the recall process( Reference Wu, Whittemore and Jung 54 ). The adjustment for current diet in all models was one of the strengths of the present study; this ensured that associations between the past dietary factors and cognitive performance were not driven by current intake.
An inevitable limitation to the study design is the problem of controlling for unmeasured life period-specific covariates that potentially would have an impact on the findings. Despite the extensive range of theoretically relevant demographic, lifestyle and health-related variables included in the analyses, it is acknowledged that confounding by unmeasured life period-specific covariates cannot be discounted. The determinants of dietary choice and behaviour are embedded within complex personal and social systems( Reference Shepherd 55 ). Given the time period across which dietary intake was being measured, covariate control could not be comprehensive. This is particularly relevant given the associations that we have reported between dietary patterns consumed in childhood and cognition in later life. It would be of interest to explore these associations further by including additional appropriate early-life autobiographical information from participants in analyses.
Cross-sectional designs investigating the impact of current dietary exposures on the measures of cognition are limited by the possibility of reverse causation. Preference for sweet foods may be influenced by changes in brain glucose metabolism that occur in those that develop dementia( Reference Talbot, Wang and Kazi 56 ) and such changes may determine dietary choices, rather than dietary intake making an impact on cognitive outcomes. Although technically cross-sectional, the present study assessed dietary intake from earlier decades preceding the periods when such changes are likely. This adds weight to the causal direction of dietary pattern predictions of cognitive measures. Reverse causation is also possible because early-life intellectual ability has an impact on later food choice( Reference Gale, Deary and Schoon 57 ). However, this was unlikely in the present study because the associations between dietary patterns and later-life cognitive performance were all from the childhood period, when individuals' dietary choices would have been largely determined by their families, although the influence of parental intellectual ability on family diet cannot be discounted.
The extensive test battery available to assess multiple cognitive domains in this sample was one of the strengths of the present study. Constructs were measured by two or more tests that increased reliability, and the battery overall was designed specifically to be sensitive to the effects of ageing on cognitive abilities( Reference Danthiir, Burns and Nettelbeck 27 ).
This is the first known study to examine the associations between recalled dietary patterns from multiple life periods and older-age cognitive performance; replication of this approach is necessary and results from a sample of convenience cannot be generalised to other populations. Moreover, it is acknowledged that the LDQ from which the lifetime dietary patterns were derived is a new measure and further testing of the questionnaire's validity is desirable. Investigation of the associations between recalled dietary patterns and longitudinal cognitive change is also warranted. Additionally, assessing long-term dietary intake and late-life cognition performance in culturally varied cohorts would be of interest.
The finding that dietary patterns from across the lifetime significantly predicted cognitive performance over and above its associations with current diet supports the relevance of early-life dietary exposures to later-life cognitive outcomes. After adjustment for the variance shared between the lifetime dietary patterns, childhood was the only life period for which dietary patterns remained to be significant predictors of later-life cognitive performance. These preliminary findings are relevant in terms of identifying childhood as a ‘critical period’ for intervention to minimise the possibility of later-life cognitive deficits.
Acknowledgements
The authors gratefully acknowledge the contribution of the EPOCH research team and thank the EPOCH participants for their commitment and participation.
The EPOCH trial was supported by the University of Adelaide and the CSIRO Animal, Food and Health Sciences Brailsford Robertson Award (to V. D., T. N. and C. W.) and a National Health and Medical Research Project Grant (no. 578800; to V. D., T. N. and C. W.). D. E. H. was supported by a PhD scholarship jointly funded by the Faculty of Health Sciences at the University of Adelaide, South Australia, and the CSIRO Animal Food and Health Sciences.
The funders had no role in the design, analysis or writing of this article.
The authors' contributions were as follows: V. D., T. N., C. W. and D. E. H. designed the research; D. E. H. and V. D. conducted the research and performed the statistical analysis; D. E. H. wrote the paper. All authors read and approved the final manuscript.
There are no conflicts of interest.