Introduction
Health is associated with a variety of factors such that Body Mass Index (BMI) is one of them. The World Health Organization (WHO) defines BMI as a person’s weight in kilograms divided by the square of his/her height in meters (kg/m2) (World Health Organization, 2021a). Therefore, BMI can be employed as a marker to measure the risks of certain diseases related to excessive weight such as obesity, coronary heart disease, stroke, or cancer.
Obesity has started being pronounced more as one of the crucial public health issues governments have to deal with (Tremmel et al., Reference Tremmel, Gerdtham, Nilsson and Saha2017). While investigating factors driving obesity, policy makers need to consider determinants that are likely to drive obesity among individuals. Prior studies suggest that socio-demographic factors along with behavioural preferences have a substantial impact on the prevalence of obesity among individuals (Azagba & Sharaf, Reference Azagba and Sharaf2012a; Bottai et al., Reference Bottai, Frongillo, Sui, O’Neill, McKeown, Burns, Liese, Blair and Pate2014; Mitcell et al., Reference Mitcell, Dowda, Pate, Kordas, Froberg, Sardinha, Kolle and Page2017).
Recent research on the determinants of obesity by examining BMI suggests that there are different channels through which determining the level of BMI among individuals. The very first channel is the socio-demographic factors which are found to have a crucial influence in determining the BMI among individuals (Azagba & Sharaf, Reference Azagba and Sharaf2012a; Demir et al., Reference Demir, Demir and Bilgic2019; Costa-Font et al., Reference Costa-Font, Fabbri and Gil2009; Ljungvall & Zimmerman, Reference Ljungvall and Zimmerman2012; Etile, Reference Etile2009; Karaoglan & Tansel, Reference Karaoglan and Tansel2018). Many empirical models predict a statistically significant association between socio-demographic factors and the levels of BMI. Not surprisingly, the results are consistent across different countries. Behavioural preferences can be reported as the second channel affecting BMI. Individuals might differ from each other concerning their behaviours for sport, diet, smoking, vitamin intake, alcohol, vegetable, and fruit consumptions, etc. WHO defines physical activity as any bodily movement produced by skeletal muscles that require calorie expenditure such that all physical movement during leisure time, for transport to get to and from places, or as part of a person’s work is assumed to be physical activity. WHO suggests that moderate and vigorous intense physical activity is linked to better health outcomes (World Health Organization, 2021b).
Recently, WHO reported that obesity in Turkey is considerably rising and is one of the most important and alarming health conditions in Turkey. According to the estimates employed by the WHO, 38% of the individuals is expected to be obese in Turkey by 2030 (World Health Organization, 2013). Moreover, Turkey has one of the highest obesity prevalence among the European region countries. Parallel to the WHO rates, the previous studies on obesity in Turkey reveal that obesity among adults continues to increase at regional and national levels in Turkey, especially in the last 20 years (Yumuk, Reference Yumuk2005; Erem et al., Reference Erem, Arslan, Hacihasanoglu, Deger, Topbaş, Ukinc, Ersoz and Telatar2004; Erem, Reference Erem2015).
Although obesity is one of the most important health conditions that needed to be taken precaution in Turkey, there are limited studies on it. The earliest studies on obesity in Turkey have been mostly conducted in the early 2000s on the specific regions in Turkey (Hatemi et al., Reference Hatemi, Yumuk, Turan and Arık2003; Erem et al., Reference Erem, Arslan, Hacihasanoglu, Deger, Topbaş, Ukinc, Ersoz and Telatar2004; Yumuk, Reference Yumuk2005). There exists only a few studies investigating obesity by examining the determinants of BMI among individuals living in Turkey at the national level. The national-level studies are rather recent and have been conducted since 2018 (Karaoglan & Tansel, Reference Karaoglan and Tansel2018; Demir et al., Reference Demir, Demir and Bilgic2019; Sengul et al., Reference Sengul, Lopcu and Cam2020). Previous studies in Turkey examining the determinants of BMI specifically focused on the impact of socio-demographic determinants among adults by mostly examining the data before 2014. Therefore, there remains uncertainty regarding the current situation of the impact of socio-demographic and behavioural preferences on the BMI of adults living in Turkey. However, in order to prevent and reduce obesity in Turkey, the possible determinants and their effect on obesity need to be investigated. This may help to provide insights into how to reduce and prevent obesity to relevant institutions and organizations.
This study contributes to a limited literature by adopting two purposes. Different from previous studies, this study firstly investigates the impact of socio-demographic and behavioural factors of adults living in Turkey using the unconditional quantile regression (UQR) method for more current periods from 2014 to 2019 across males and females, separately. As female and male bodies exhibit different genetic and biological characterizations, this study examines the association between socio-demographic and behavioural preferences and BMI among females and males, separately. This study uses a wide range of indicators to be able to capture a full definition of socio-demographic factors and behavioural preferences of individuals, which are explained in the following sections. This makes it possible to predict more robust estimates using the UQR method. In this way, this study may provide a more detailed analysis of the impact of the socio-demographic and behavioural factors across the BMI distribution of males and females. Decomposing and understanding how females and males face obesity risk and the gender differences may be a necessary and important start to develop effective policies (Case & Menendez, Reference Case and Menendez2009). Parallel to the previous explanation, secondly, this study employs the decomposition method, in order to determine the BMI gap by gender and the variation of the BMI gap across the distribution. This leads to examine the BMI gap across the distribution and to determine the socio-demographic and behavioural determinants that might wider or narrow the BMI gap.
Recently, the UQR method attracts attention from researchers especially those working in labour and health economics. Since the UQR approach is a relatively new method, there are exists not many studies particularly examining the determinants of the BMI with UQR approach. Therefore, to the best of our knowledge, this is the first study analysing the association between the socio-demographic behavioural factors and BMI of individuals living in Turkey by exploiting the UQR method and decomposing the gender differences via the quantile decomposition method. In this way, this paper aims to contribute to the existing empirical literature.
The study is organized as follows. The next section outlines the data, model, and descriptive statistics. The results section presents empirical results of the UQR and the decomposition methods on the impact of socio-demographic and behavioural factors on the BMI of adults living in Turkey. The results section further contains sensitivity analysis and reveals the limitations of the current study. Finally, the last section concludes remarks and provides further discussion.
Methods
Data
This study exploits the Turkish Health Survey (THS) conducted by the Turkish Statistical Institute (TURKSTAT). THS is cross-sectional data that has been conducted since 2008 for almost every two years in order to produce significant indicators of health that cannot be compiled through administrative records and to create a data source for decision-makers and researchers. This study employs the last three years of the survey 2014, 2016, and 2019.
WHO provides a definition for BMI among adults older than 20 years old (World Health Organization 2021a). By following the WHO definition of BMI, this study restricts the sample to adults in the same age group. The restricted sample contains 17 261, 15 655, and 15 703 observations for the years 2014, 2016, and 2019, respectively. Figure 1 shows the distribution of the BMI across gender by illustrating the boxplots for each year in the study.

Figure 1. The boxplots of BMIs by gender for 2014, 2016, and 2019. 0 represents males and 1 represents females. Authors’ calculations from the THS data.
According to the boxplots in Figure 1, the shapes of the BMI distributions differ by gender with respect to location, spread, and skewness. This provides quantitative evidence for the fact that females and males should be examined separately in the analysis. Furthermore, there are several outliers for both females and males over three years and the distribution of BMIs may be non-Gaussian and long tailed. The outliers observed in the boxplots are due to the natural structure based on the variability of the BMI distribution. Outliers provide important and unusual information about the data and may lead to better model specifications (Zellner, Reference Zellner2007). Therefore, removing the outliers from the data causes a loss of important information regarding the variability of the data. Since the distribution of BMIs contains several outliers, conventional regression models such as Ordinary Least Squares (OLS) based on the mean of the distribution may not suitable and take any arbitrary value.
Individuals who were interviewed do not significantly differ in terms of socio-demographic factors, behavioural preferences, and BMI across 2014, 2016 and 2019. Therefore, this study pools the last three years of the survey in the estimation process. The tests that support this finding are upon on request from the authors. The pooled data set contains 26 788 (55.01%) female and 21 831 (44.9%) male individuals.
Model
OLS is the best linear unbiased estimator to estimate the regression model if the Gauss-Markov assumptions are valid. However, invalidation in the Gauss-Markov assumptions leads OLS to be biased and inconsistent in some cases. The OLS estimator is based on the conditional mean of the distribution, therefore could take any arbitrary value in the presence of outliers and is biased and inconsistent in the case of long-tailed distributions. Since Figure 1 shows that the data examined in this study contains several outliers and provides insights into long-tailed distribution, OLS may be inconvenient for this study. Unlike the OLS, quantile regression models are based on the quantiles of the distributions and allow to examine the different effects of the explanatory variables throughout the entire distribution of the dependent variable, in this case, the BMI.
The first aim of this study focus on the impacts of socio-demographic and behavioural factors on the BMI distribution of males and females and the OLS may be inconvenient for this study because of the outliers and long-tailed distributions. In order to analyse the variation of these determinants on the BMI distributions with a regression method that is robust to both outliers and non-Gaussian distribution, quantile regression is determined as a more suitable method for this study. To assess the impact of socio-demographic factors and behavioural preferences on BMI of the Turkish adults, this study exploits the UQR method proposed by Firpo et al. (Reference Firpo, Fortin and Lemieux2009). UQR is based on Recentred Influence Function (RIF) which is calculated from a transformation of the original dependent variable. RIF provides to observe the effect of each explanatory variable on the transformed dependent variable. The fundamental advantage of the UQR is to provide more interpretable and generalizable coefficients as it marginalizes the effect over the distributions of other explanatory variables in the model (Borah & Basu, Reference Borah and Basu2013). The UQR estimates the impact on the dependent variable at the θth quantile for all observations in the sample unconditional on the explanatory variable. Although the Conditional Quantile Regression (CQR) method proposed by Koenker & Bassett (Reference Koenker and Bassett1978) is frequently used in the literature, the UQR method may be preferable in order to examine the differences across the distribution and provide policy implications since it estimates generalizable results (Rodriguez-Caro et al., Reference Rodriguez-Caro, Vallejo-Torres and Lopez-Valcarcel2016).
To estimate the impact of socio-demographic factors and behavioural preferences of adults on BMI distribution over quantiles, this study constructs the UQR model as below.

Equation 1 is estimated for females and males separately and for each quantile of the explanatory variables denoted by
$\theta$
. Detailed definitions of variables used in the study are available in Table 1. The information for the study period for BMI and socio-demographic factors of individuals such as age, years of schooling, marital status, employment status, number of people living in the household derived from the survey (Table 1, Panel A). The indicators for behavioural preferences e.g., physical activity status, the frequency of tobacco and alcohol use, walking, fruit and vegetable consumptions, the status of food supplements or vitamin intake, and health status are also available in the whole sample and are presented in Panel B of Table 1.
Table 1. Definition of variables used in the study
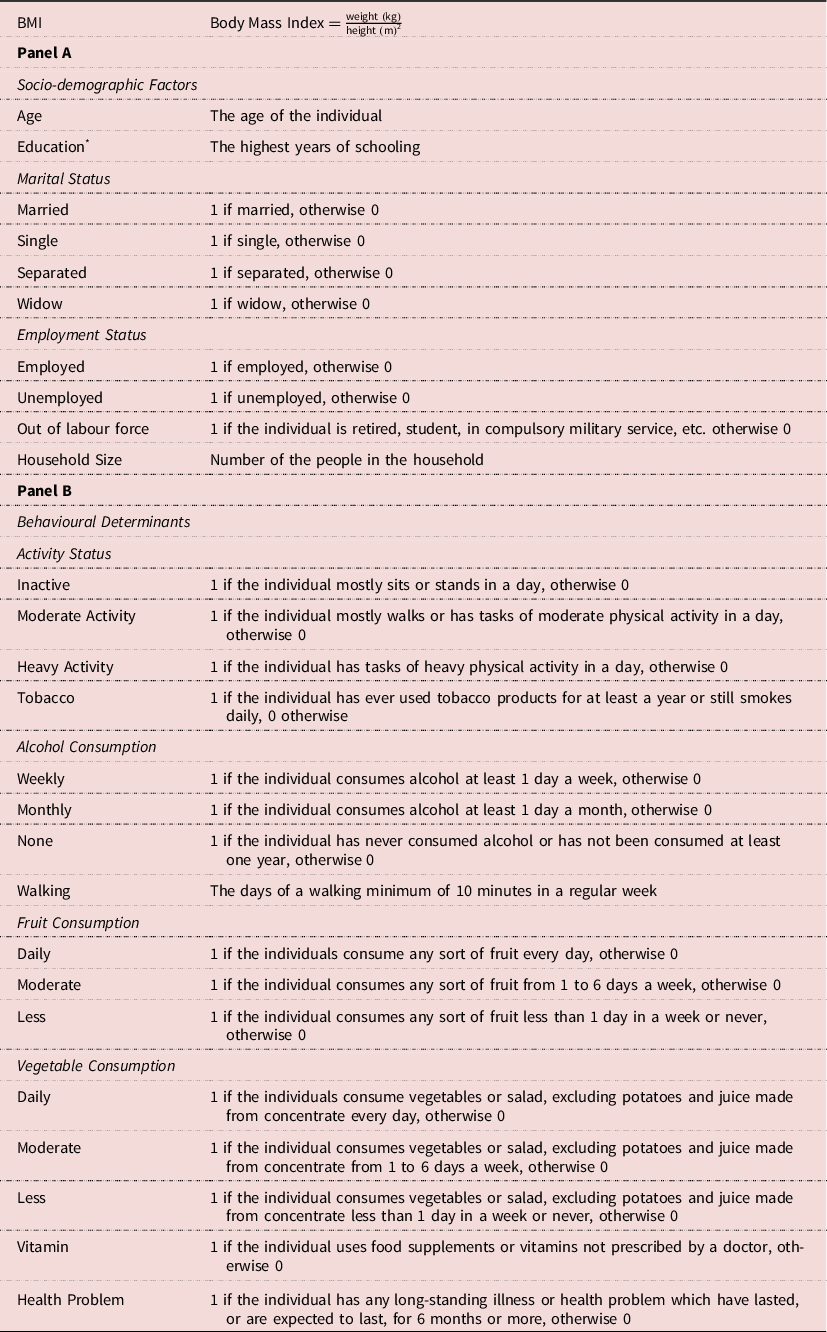
* The official years of schooling derived from the Ministry of National Education as 0 for the no schooling or illiterate, 8 years for compulsory education, 12 years for high school degree, 14.5 years for Associate Degree, 16 for bachelor’s degree, 18 for master’s degree, and 22 years for PhD degree.
The selection of socio-demographic factors and behavioural determinants that are likely to affect BMI is based on the literature investigating the determinants of BMI (Azagba & Sharaf, Reference Azagba and Sharaf2012a; Costa-Font et al., Reference Costa-Font, Fabbri and Gil2009; Kan & Tsai, Reference Kan and Tsai2004; Costa-Font et al., Reference Costa-Font, Fabbri and Gil2010; Azagba & Sharaf, Reference Azagba and Sharaf2012b; Shaikh et al., Reference Shaikh, Siahpush, Singh and Tibbits2015; Bonanno et al., Reference Bonanno, Bimbo, Cleary and Castellari2018; Al-Hanawi et al., Reference Al-Hanawi, Chirwa and Kamninga2020). Since the relationship of age and education with the BMI is parabolic the squared forms of age and education are also included in the model. Furthermore, this study also would like to assess the impact of any food supplements or vitamins intake on the BMI levels of adults. Food supplements or vitamins are not usually prescribed by physicians or GPS. They are known to support immune systems or help individuals develop a healthy diet. This study further introduces an indicator for the status of any illness or health problem which have lasted or are expected to las for 6 months in his/her lifetime.
Descriptive statistics
According to the WHO’s definition of BMI, adults above 20 years old could be categorized as underweight, normal weight, pre-obesity, obesity class I, obesity class II, and obesity class III, respectively. Table 2 presents the average values of continuous variables and the ratios of the binary variables in Equation 1 in the pooled data across the aforementioned levels of BMI. All the values are calculated for the related BMI category for the females and males, separately. Furthermore, Table 2 contains the total mean and ratio values for the females and males in order to examine the change relative to the mean and ratios by the BMI categories. The results in Table 2 are also illustrated in Figure 2 separately by socio-demographic factors (Figure 2.a) and behavioural determinants (Figure 2.b). The horizontal axes in Figure 2 express the aforementioned BMI categories while vertical axes express the values of the related variables.
Table 2. Descriptive statistics

* indicates a binary variable. All the binary variables in Table 2 are multiplied by 100 in order to present the prevalence in 100 people. The mean values are calculated for the continuous variables.

Figure 2. Descriptive statistics by gender. Females are represented by continuous lines; males are represented by the dashed lines.
The results in Table 2 and Figure 2 suggest that females are more likely to be obese compared to males once they get older. Despite, the change in females’ BMI by age is higher compared to males over the lifetime as pregnancy may provide an explanation for this finding. The average year of schooling is negatively correlated to the prevalence of obesity among both males and females. Since being married reduces the competition for mating, the incentives to keep in good shape may be reduced (Costa-Font et al., Reference Costa-Font, Fabbri and Gil2009). Similarly, the highest ratio of being married is for the Turkish adults who are in the obesity class I. Unlike being married, single females and males are more likely to be underweight or normal weight categories. Similarly, separated individuals are seemed to be associated with a higher probability to be in the normal weight group this holds for both females and males. As for the widow individuals, females are more likely to be in the obesity class III whereas males are more likely to be underweighted. The table further indicates that the prevalence of being in the pre-obesity category is higher for the employed males whereas the prevalence of being in the underweight category is higher for employed females. Therefore, working females have a lower level of BMI compared to males. Unemployed individuals are in the underweight category of BMI, and this holds for both females and males. According to the prevalence, females who are out of labour force are more likely to be in the obesity class III whereas, on the contrary, most of the males who are out of the labour force are underweight.
With regards to the behavioural preferences of individuals and the level of BMI, inactive females and males are more likely to be obese compared to their peers in other activity groups. Moreover, inactive individuals are mostly in the BMI class III. The use of tobacco is associated with slightly lower levels of BMI and the same holds for both females and males. Weekly alcohol consumption ratios are quite different by gender; the females who consume alcohol weekly are mostly underweighted whereas the males are in the obesity class III. Monthly alcohol consumption is associated with slightly lower levels of BMI and the same holds for both females and males. Fruit and vegetable consumptions reveal interesting results. The females and males who consume fruit and vegetables daily are more likely to have a higher level of BMI whereas less consumption of fruit and vegetable leads to having lower levels of BMI. Apart from the slight differences, these results hold for both females and males. The source of this interesting result may be the portion of the consumed fruit and vegetables. If the individuals consume more fruit and vegetable more than they need, this may lead to having a higher level of BMI. Furthermore, Turkish cuisine contains a large variety of foods, and the recipes are mostly oily. Even if the individual consumes a vegetable meal, the meal may contain oil more than they need, and this may lead to a higher level of BMI. The consumption of food supplements or vitamins is higher among females compared to males. The majority of females and males who consume food supplements or vitamins are in the category of underweight. Lastly, more than half of the females and almost half of the males have a long-standing illness health problem.
Results
Table 3 presents the results of the estimation of Equation 1 using the UQR for the Q05, Q25, Q50, Q75, and Q95 quantiles of the BMI. The UQR findings presented in Table 3 are also available in Figure 3 separately for socio-demographic factors and behavioural determinants by gender. Figure 3.a presents the UQR findings for females by socio-demographic factors and behavioural determinants whereas Figure 3.b presents the UQR findings for males by socio-demographic factors and behavioural determinants. The differences in coefficients over the quantiles were also tested by following the proposed procedure by Rios-Avilla (Reference Rios-Avila2020). The test results reveal that there is a significant change in the coefficients of some of the variables over the quantiles and the results are available upon request.
Table 3. UQR findings

***p<0.01, **p<0.05, *p<0.1. The numbers in brackets are the bootstrapped standard errors with 500 repetitions. The OLS findings of this model are available upon request.

Figure 3. UQR findings by gender.
Socio-demographic factors and BMI
Panel A in Table 3 reports the results concerning the impact of socio-demographic factors. Parallel to the previous studies, since the relationship between age and BMI is nonlinear, age is added to the model in a quadratic form, and in general, the results of age are consistent with the results in the literature (Kan & Tsai, Reference Kan and Tsai2004; Baum & Ruhm, Reference Baum II and Ruhm2009; Costa-Font et al., Reference Costa-Font, Fabbri and Gil2010; Bonanno et al., Reference Bonanno, Bimbo, Cleary and Castellari2018; Karaoglan & Tansel, Reference Karaoglan and Tansel2018). The linear term of age is a positive determinant of BMI across each quantile among both females and males whereas the quadratic term is negative. The positive impact of linear age on BMI seems to be higher for females. Education measured as years of schooling enters the model in a quadratic form. The linear term is significant and positive for the females at the 5th, 25th, and 50th quantiles whereas the quadratic term is negative. This only changes for the females at the 95th quantile. As for the males, the linear term of education is positive for the males at the 25th, 50th, and 75th quantiles whereas the quadratic term is negative. Therefore, the effect of education on BMI is positive for lower education and negative for higher levels in all the quantiles. Consistent with the results of this study, previous studies also state that the lower level of education causes a higher level of BMI, especially among the individuals with lower socioeconomic status (Martinez-Ros et al., Reference Martinez-Ros, Tormo, Navarro, Chirlaque and Perez-Flores2001; Hall et al., Reference Hall, Stephen, Reeder, Muhajarine and Lasiuk2003; McLaren, Reference McLaren2007; Shaikh et al., Reference Shaikh, Siahpush, Singh and Tibbits2015; Bonanno et al., Reference Bonanno, Bimbo, Cleary and Castellari2018). The source of this may be that adults with higher years of schooling may have more awareness of their health or the more educated females may have fewer pregnancies than others may. According to marital status binary variables, being single or separated decreases the BMI of females and males, compared to those who are married. Married individuals are seemed to be more likely to be obese, according to those who are not married, and this result is consistent with the results of Janghorbani et al. (Reference Janghorbani, Amini, Rezvanian, Gouya, Delavari, Alikhani and Mahdavi2008) and Sato (Reference Sato2021). Furthermore, the negative contribution of being single or separated is higher for the females and lower weight individuals. These results are compatible with the idea of keeping a good body shape. On the contrary, being a widow increases the BMI of both females and males compared to being married. Aging, the potential depression of losing someone, and giving up the idea of keeping a good body shape could be the result of this. Parallel to the results of the previous studies (i.e., Ball et al., Reference Ball, Mishra and Crawford2002; Costa-Font et al., Reference Costa-Font, Fabbri and Gil2010), employment status coefficients indicate that employed females have less likely to be obese than the females who are out of the labour force. The negative impact of being employed on BMI is higher for more obese females. Furthermore, unemployment has a decreasing effect on BMI than being out of the labour force for both females and males. However, the employment coefficients differ for the males. Employed males have a higher BMI than the males out of the labour force. The household size is more significant for males than females. Increasing the number of people in the household increases the BMI of underweight females whereas it decreases the BMI of males.
Behavioural determinants and BMI
Indicators of activity status in Panel B in Table 3 shows that being inactive or having moderate activity increases the level of BMI among both females and males compared to having heavy activity. This impact gets higher over the BMI quantiles. The result regarding less physical activity causing higher BMI is consistent with the literature (King et al., Reference King, Fitzhugh, Bassett, McLaughlin, Strath, Swartz and Thompson2001; Martinez-Ros et al., Reference Martinez-Ros, Tormo, Navarro, Chirlaque and Perez-Flores2001; Costa-Font & Gill, Reference Costa-Font and Gil2008; Azagba & Sharaf, Reference Azagba and Sharaf2012a; Bottai et al., Reference Bottai, Frongillo, Sui, O’Neill, McKeown, Burns, Liese, Blair and Pate2014). The results of both previous studies and this present study indicates that smoking is one of the most important and negative behavioural determinants of females’ and males’ BMI (Costa-Font & Gill, Reference Costa-Font and Gil2008; Costa-Font et al., Reference Costa-Font, Fabbri and Gil2009; Costa-Font et al., Reference Costa-Font, Fabbri and Gil2010; Demir et al., Reference Demir, Demir and Bilgic2019; Sun et al., Reference Sun, Jiang, Sun, Jiagen, Guo, Lv, Yu, Yao and Jin2019). The negative effect of this study is higher among males than females and higher for individuals that are more obese. The literature on the relationship between alcohol consumption and BMI contains mixed results over countries and time. The results among cross-sectional studies generally indicate that alcohol consumption does not have significant impact on males’ BMI in general whereas it has a negative or insignificant impact on females’ BMI (Sayon-Orea, Reference Sayon-Orea, Martinez-Gonzalez and Bes-Rastrollo2011). According to the results of this study regarding alcohol consumption weekly or monthly alcohol consumption are seemed to be associated with lower BMI levels and this finding is consistent with the findings of Shaikh et al. (Reference Shaikh, Siahpush, Singh and Tibbits2015). However, the results of this study differ from the results of Arif & Rohrer (Reference Arif and Rohrer2005) indicating higher alcohol consumption leading to higher BMI levels. They examine previous data from the late 1980s and early 1990s from the United States of America by adopting a binary dependent variable model. As a reason for examining data of a different country, differences in sample selection and in the research method may explain the differences between the results. Parallel to the literature, weekly or monthly alcohol consumption is more significant for females than males and it reduces BMI, compared to those who reported no consumption. An increase in the number of days of walking in a week decreases BMI along with the increase of activity and this finding is consistent with the findings of Demir et al. (Reference Demir, Demir and Bilgic2019). However, walking has a more negative effect on females’ BMI, especially those who are in the obese categories. One might relate walking may have a higher reducing effect on individuals with higher bodies. Although vegetable consumption is mostly insignificant over the quantiles, the level of BMI decreases along with the increase in the moderate or less consumption of fruit and vegetable compared to consuming every day and individuals who consume fruit or vegetable every day have higher BMIs. The results are consistent with the ratios in the descriptive statistics and the reason for this may be because of the portion the individuals consume and the characteristic of Turkish cuisine. If the individuals consume more fruit and vegetables than they need, and they do this every day, this may lead to having a higher BMI. Moreover, Turkish cuisine is mostly based on the consumption of meat, carbohydrate, and oily meals. Therefore, even though the individual consumes vegetable meals every day, if he/she consumes them with oil more than they need, this may cause a higher level of BMI. In addition, similar to the findings of this study, some of the studies in the literature regarding the fruit and vegetable consumption reveal that fruit and vegetable consumption have seemed to be having insignificant impact on the BMI (Costa-Font et al., Reference Costa-Font, Fabbri and Gil2010; Demir et al., Reference Demir, Demir and Bilgic2019). Consistent with the findings of this study in general, the findings of Demir et al. (Reference Demir, Demir and Bilgic2019) for Turkish individuals reveal that fruit consumption has positively effect BMI whereas vegetable consumption either insignificant or negative. Moreover, parallel to this study, fruit consumption lead to a higher likelihood of being in class I and II obesity Italian males and Spanish females whereas unlike the insignificant results of this study, vegetable consumption lead to a lower likelihood of being overweight among Italian males (Costa-Font et al., Reference Costa-Font, Fabbri and Gil2010). Taking additional food supplements or vitamins not prescribed by a doctor decreases BMI. The negative impact is higher for the higher BMI categories, especially for females. Finally, having a long-standing illness or health problem increases the level of BMI for both females and males. However, like other determinants, the increase is faster for females.
Decomposition
The second main focus of this study is to examine the BMI differences by gender and its variation across the distribution. The gender-based decomposition analyses are quite common in the research analysis to determine the gender differences and the possible factors that might wider or narrow the gender difference in the related study, and this study adopts a decomposition method to achieve the second aim of this study. Blinder-Oaxaca (OB) decomposition proposed in the same year by Blinder (Reference Blinder1973) and Oaxaca (Reference Oaxaca1973). OB decomposition is one of the most popular methods to analyse the differences between the groups such as gender, race, and occupation. OB decomposition estimates regressions for each group, in this case for females and males, and takes account of possible interactions and the relationships.
OB decomposition has been adapted to the quantile regression methods in recent years to calculate more detailed decompositions. Since the BMI model in Equation 1 was estimated by the UQR method based on RIF, in order to examine the differences in the socio-demographic and behavioural determinants over BMI distribution, the decomposition method is also employed for the quantiles based on RIF in this study. Therefore, in order to compare and decompose the gender disparity in BMI and get insights beyond the mean, this study applies the standard OB RIF-decomposition. By adopting a RIF-based quantile decomposition method, this study derives more detailed information on the BMI gap by gender across body mass index distribution. Table 4 presents the overall decomposition results over unconditional quantiles.
Table 4. Standard OB RIF-decomposition by gender

***p<0.01, **p<0.05, *p<0.1. The numbers in brackets indicate the bootstrap standard errors with 500 repetitions by following the Firpo et al. (Reference Firpo, Fortin and Lemieux2018).
According to the literature, interactions effect by gender would test whether or not the trends are similar in both genders and some studies prefer to analyse the gender differences with interactions effect. However, in this study, after determining the gender differences in the distributions by boxplots and statistics, it is preferred to analyse males and females separately instead of analysing genders in one model. This leads to examining the gender differences using the decomposition method. In the context of this study, the decomposition methods provide detailed information about the gender difference in the BMI, the socio-demographic and behavioural determinants that might wider or narrow the gender gap, and their magnitude effect on the gender differences. Moreover, adopting a RIF-based quantile decomposition method, this study analyses the BMI gap by gender across the BMI distribution. With the explained advantages of the UQR and decomposition methods, this study prefers to analyse the gender differences via the decomposition method instead of interaction terms.
Table 4 shows that the differences of BMI among females and males are significant and the disparity by gender tends to increase over unconditional BMI distribution and this result is consistent with the results of Dunn et al. (Reference Dunn, Tan and Nayga2012) and Al-Hanawi et al. (Reference Al-Hanawi, Chirwa and Kamninga2020) along with the small differences. For the 5th and 25th quantile of the BMI, males have higher BMI whereas for the 50th, 75th, and 95th quantiles females have higher BMI. The disparity in BMI by gender gets higher at the upper and lower quantiles of BMI distribution. Therefore, the BMI gap is higher for the females and males underweight or obese.
Detailed standard OB RIF-decomposition results for both covariate and structure effects are available in Table 5. The covariate effect explains how important the differences in characteristics whereas the structure effect explains the differences in estimates. This study focuses on how the differences in socio-demographic factors and behavioural determinants affect the BMI gap over the BMI distribution. Therefore, the covariate effect of detailed standard OB RIF-decomposition results is illustrated in Figure 4 separately for socio-demographic factors (Figure 4.a) and behavioural determinants (Figure 4.b). Detailed standard OB RIF-decomposition results presented in Table 5 show that all socio-demographic factors are significant for some of the quantiles of the BMI distribution. Therefore, socio-demographic factors are seemed to have a significant effect on the BMI disparity by gender. According to the covariate decomposition results, education, being single (except for the 95th quantile) or widow and being employed have a negative impact on the BMI gap over quantiles. The effect of differences in education is seemed to have a higher negative effect at the upper quantiles such as 75th and 95th. Being single has a smaller negative effect on the BMI gap after the 25th quantile, surprisingly the effect reversed at the 95th quantile and starts to contribute to the BMI gap positively. Unlike being single, even though the effect of both being single and widow negatively affects the BMI gap, the negative effect of being widow gets higher after the 25th quantile. Employment status shows that being employed negatively affects the BMI gap and the effect remains similar except for the highest quantile of the BMI; the effect of being employed has the most negative contribution at the 95th quantile. Unlike the explained socio-demographic factors, the differences in age, being separated, and household size increases the BMI gap.
Table 5. Detailed standard OB RIF-decomposition by gender

***p<0.01, **p<0.05, *p<0.1. The numbers in brackets indicate the bootstrap standard errors with 500 repetitions by following the Firpo et al. (Reference Firpo, Fortin and Lemieux2018).

Figure 4. Covariate effect of standard OB RIF-decomposition by gender.
Activity status, smoking, alcohol consumption, walking, fruit consumption, food supplements or vitamin consumption, and long-standing health problem are some of the most significant behavioural determinants on the BMI gap by gender. Inactivity, tobacco consumption, alcohol consumption, walking, fruit consumption, and having a long-standing illness or health problem contribute to the BMI gap negatively while the consumption of food supplements or vitamins contributes positively, and the positive contribution gets higher at the upper quantiles. The negative effect of inactivity and walking on the BMI gap is seemed to get higher from the 50th quantile to the upper quantiles. The differences in tobacco consumption decrease the BMI gap more over the quantiles and it decreases the BMI gap at the 50th quantile more than two times as in the 5th quantile. The negative effect of the differences in weekly and monthly alcohol consumption on the BMI gap is higher at the 25th quantile whereas the fruit consumption has a smaller effect on the BMI gap, and it remains similar over the quantiles. Having a long-standing illness or health problem has a negative effect on the BMI gap and the negative effect is higher at the upper quantiles.
Sensitivity analysis
The standard OB RIF-decomposition is based on UQR and one of the requirements of the UQR method to decompose is linearity (Firpo et al., Reference Firpo, Fortin and Lemieux2018). In the case of nonlinearity, the standard RIF-based decomposition results may be affected because of the linearity assumption. However, in the case of beyond the mean analyses, standard OB RIF-decomposition reveal similar results to the flexible method that considers nonlinearity. Therefore, after determining that there is no significant specification error regarding linearity, because of the advantages that standard OB RIF-decomposition proposes such as linear specification not affecting the overall estimates and the linear specification providing a much simpler interpretation, the standard OB RIF-decomposition may be preferred Firpo et al. (Reference Firpo, Fortin and Lemieux2018).
Since the relationship of age and schooling variables with the BMI is nonlinear, the BMI model of this study contains age and schooling variables in quadratic forms. In order to analyse any significant changes in the decomposition results regarding the effect of linearity requirement, both the age and schooling continuous variables are divided into four binary variables (aged between 20-34, 35-49, 50-65, 65+ and compulsory or less education, high school degree, associate or bachelor’s degree, postgraduate degree).The standard OB RIF-decomposition results from this model and the results in Tables 4 and 5 are examined and the findings reveal no significant difference.
Another way to examine the effect of linearity requirement on the standard OB RIF-decomposition results is to combine the standard OB RIF-decomposition with the reweighted procedure, examine the specification error and compare the decomposition results (Töpfer, (Reference Töpfer2017); Firpo et al., (Reference Firpo, Fortin and Lemieux2018)). Therefore, in addition to the standard OB RIF-decomposition, the reweighted OB RIF-decomposition proposed by Firpo et al. (Reference Firpo, Fortin and Lemieux2018) also has been calculated in order to examine the differences. The specification errors from the reweighted OB RIF-decomposition are seemed to be small and insignificant for most of the quantiles; they are significant for only the 25th and 75th quantiles at 5% and 10% significance levels while they are insignificant for the 5th, 50th, and 95th quantiles at 1%, 5%, and 10% significance levels. Likewise, the decomposition results reveal no significant difference between standard and reweighted decompositions. In this way, the standard OB RIF-decomposition provides accurate results in this study, and therefore, this study only presents the standard OB RIF-decomposition results in Tables 4 and 5. However, all the results are available upon request.
Limitations of the study
This present study also has some limitations that should be noted. The limitation regarding the THS data is about the income levels of the individuals. Even though 2014, 2016, and 2019 THS data analysed in this study have a more similar structure than the previous surveys, the survey questions regarding income have been changed from 2014 and 2016 to 2019. The survey question regarding the income level of the individuals in 2014 and 2016 contain five choices and all the individuals have responded to it. However, in 2019, the income level question has twenty choices and unlike in 2014 and 2016, half of the individuals did not prefer to answer the question. Since this study pools 2014, 2016, and 2019 data, this study could not examine the effect of income levels on the BMI distribution. In order to determine the possible bias because of the income levels, this study estimates the BMI model with the income levels for the data of 2014 and 2016, separately, and compared them with the UQR results presented in Table 3. The estimation results reveal no significant change in the coefficients and these results are available upon request.
Another limitation of this study is that there exists no information regarding the geographical regions or habitats of individuals who took part in the survey. Moreover, one might expect that employed people burn more calories than the others in a day as a result of the time and activity spent at work depending on their occupation type. Unfortunately, this study is not able to derive detailed information concerning the type of occupation an individual has in the sample. Therefore, this study could not include any information related to those variables in the model and could not examine their effect on the BMI distribution. Lastly, the tobacco consumption rates of females may be underreported by them because of social norms on females smoking. Likewise, alcohol consumption rates of both females and males may be underreported as well because of religious beliefs.
Discussion
This study investigates the impact of socio-demographic determinants and behavioural preferences on BMI among females and males by employing the UQR method to the Turkish Health Surveys for 2014, 2016, and 2019. The UQR approach allows to model unconditional quantiles of BMI as a function of those aforementioned determinants by gender. UQR findings for the socio-demographic determinants suggest that education has a positive effect on BMI for lower levels of education and a negative effect for higher levels at all the quantiles for both males and females. These effects differ for females at the 95th quantile and education has a negative linear effect on their BMI. In addition to years of schooling, marital status especially being single or separated is negatively associated with the BMI levels and the same results hold for both genders. This result could be arising from the fact that adults who are single or separated could be more concerned regarding their physical appearance. Regarding the behavioural determinants of BMI, indicators of activity status show that being inactive or having moderate activity increases the level of BMI among both females and males compared to having heavy activity. Smoking has a negative impact on BMI levels and this result holds for both females and males whereas weekly or monthly alcohol consumption is more significant for females than males and it reduces the BMI, compared to those who reported no consumption. The findings interestingly suggest that daily consuming fruit and vegetables lead to having a higher level of BMI according to less consumption. Food supplement or vitamin intake helps adults to reduce their weight, especially for females. However, if an adult has any long-standing illness or health problem, he/she is more likely to experience a higher level of BMI.
As for OB RIF-decomposition, the findings report a BMI gap among males and females because of some potential socio-demographic and behavioural factors (i.e., education, marital status, activity status, smoking, alcohol consumption, walking, fruit consumption, food supplements or vitamin consumption, and the existence of a long-standing illness or health problem). The BMI gap becomes wider among under-weight or obese adults. Surprisingly, the BMI gap is in favour of females among underweighted or normal weighted individuals whereas it is in favour of males among the individuals who are in the pre-obesity and obesity class I, II, and III categories. In the other words, the females are seemed to have lower BMI levels than males among underweighted or normal weighted individuals. However, this is reversed for the higher levels of BMIs, and females are seemed to be more obese than males. Having obtained evidence for the fact that males and females differ in terms of the effect of socio-demographic and behavioural factors on the distribution of BMI, policies designed to prevent obesity should consider such differences by gender. The results of this study are seemed to be similar to the results of the studies conducted for some of the European and Middle East region countries, along with some differences. Therefore, the results of this study regarding obesity and overweight by considering gender differences may provide an insight in designing and implying more effective and strengthened future obesity-related policies not only for Turkey but also for other countries having similarities in obesity.
The findings of this study within the context of Sustainable Development Goals (SDG) and Agenda 2063 provide insights into how to reduce, prevent and control obesity and narrow the gender gap in obesity. SDG have been launched by the United Nations (UN) in 2015 with 17 goals and some of them are related to health. The third goal in SDG entitled ‘good health and well-being’ aims to achieve to ensure healthy lives and promote well-being for all of all ages. Since obesity and overweight are major risk factors for human bodies and prevent healthy lives, obesity is one of the most important health situations that should be prevented and controlled in achieving the SDG 3 target. The current situation in Turkey shows that obesity and overweight are some of the most important health conditions among Turkish adults. Therefore, Turkey has acknowledged obesity under SDG 3 in Turkey’s Voluntary National Review (VNR) presented in 2019. Obesity can be considered not only under SDG 3 but also SDG 12 target entitled ‘ensure sustainable consumption and production patterns’. Achieving the SDG 12 target by reducing food waste and increasing the connection between nature and humans with healthier foods and more physical activity may help to reduce obesity indirectly. By determining the similar targets with SDG, Agenda 2063 was launched by the African Union (AU) in the same year with SDG. Therefore, similarly to the SDG, obesity can be considered under the third and seventh targets as ‘healthy and well-nourished citizens’ and ‘environmentally sustainable and climate-resilient economies and communities’ with the sub-target ‘sustainable consumption and production patterns’.
The findings of this study induce some policy implications to reduce obesity in the context of these SDG and Agenda 2063 targets. In recent years, local governments in Turkey have built a large number of outdoor fitness parks including walking paths and fitness equipment. Citizens are very interested in such parks as it is freely accessible and even exists in rural areas where people have limited access to professional gyms or sports centres. However, the proficiency levels of each individual in sports might differ. As a result, sports injuries, bruises or twisting of ankles might occur. Professional help (e.g., physical education teacher) provided by local governments at each outdoor fitness park might prevent sport injuries and motivate people in terms of weight control and help them do proper exercises for weight loss. Moreover, celebrities might have a potential impact on society to promote exercise and a healthy diet. Therefore, public service announcements or public adverts on television by celebrities on the importance of weight loss and a healthy diet can induce society to be more interested in adopting a healthy lifestyle. In Turkey, smoking cessation services including helplines or health centres are available by the Turkish Ministry of Health. The main duty of quitting services is to support individuals to quit smoking in a more efficient way. Accessing such lines or health centres are free of charge and accessible from anywhere to anyone. Mental support is also included in the scope of such services. Therefore, the existence of similar services for obesity might actually motive people and help them keep sustainable progress in weight loss. In some countries, there are organizations and charities supporting people to fight with obesity. They help society understand what obesity is and how one can deal with obesity. Governments should support these organizations in order to lessen society’s pressure and the stigma on obese people. Public bodies can also support promotions on healthy foods (e.g., buy one get one free or discounts on special days) by coordinating food trades within the country.
As the findings of this study indicate, another important target that obesity needed to be considered under is Gender Equity SDG 5 entitled ‘achieve gender equality and empower all women and girls’ and Agenda 2063 target 17 entitled ‘full gender equality in all spheres of life’. According to the findings of this study, reducing the differences in schooling and employment would reduce the BMI gap by gender. Since there is a significant gap in educational level and employment rates between females and males, in favour of males, reducing the gender differences in these socio-demographic factors would reduce the obesity and overweight among females and the gap between females and males would be narrowed. Moreover, the decreasing effect would be higher for individuals with a higher level of BMI. Along with the socio-demographic factors, the differences in behavioural determinants should be reduced. Encouraging females to perform more physical activity and walk more on a regular day may help to narrow the gender gap in BMI.
This study may provide a clear understanding for policymakers on how to design efficacious obesity policies considering the differences in the effect of socio-demographic factors and behavioural preferences on the distribution of BMI across females and males. The results suggest that the Ministry of Health should specifically target different groups for males and females and should reduce the differences in socio-demographic and behavioural determinants between females and males to prevent and reduce obesity prevalence in Turkey.
Acknowledgements
The authors would like to thank the Editor and two anonymous reviewers for their insightful comments and suggestions which help to improve the manuscript.
Author contributions
Ebru Caglayan-Akay, Merve Ertok-Onurlu, and Fulden Komuryakan contributed equally to the study conception, design, material preparation, data collection, model estimation, writing, revision, and editing. All authors read and approved the final manuscript.
Funding
This research received no specific grant from any funding agency, commercial entity, or not-for-profit organization.
Conflict of interest statement
The authors have no conflict of interest to declare.
Ethical approval
The analysis in this manuscript uses secondary data containing no identifying information for any of the individuals in the survey.
Data accessibility statement
The data that support the findings of this study are available from the Turkish Statistical Institute, but restrictions apply to the availability of these data, which were used under license for the current study, and so are not publicly available. Data are however available from the authors upon reasonable request and with permission of the Turkish Statistical Institute.