Studies involving dietary patterns (DP) and their association with diseases have several benefits over the conventional approach, which has focused largely on the effects of single nutrients or individual foods(Reference Hu1, Reference Willett2). As the measurement of diet is complex, and foods are typically consumed in combinations, the combined effect of nutrients and foods can be observed only when DP are examined(Reference Hu1, Reference Willett2). Moreover, results from DP analyses are more helpful in disseminating diet-related messages to consumers that they may be more likely to adhere to rather than those related to single foods or nutrients(Reference Freeland-Graves and Nitzke3). DP have also been related to selected biomarkers of dietary exposure(Reference Hu1, Reference Willett2) and have been reported to contribute in the development or prevention of CHD and type 2 diabetes mellitus (T2DM)(Reference Brunner, Mosdøl, Witte, Martikainen, Stafford, Shipley and Marmot4).
Recent focus has been on the occurrence of metabolic syndrome (MetS), a constellation of metabolic abnormalities including central obesity, elevated blood levels of CHD-promoting lipids, hypertension, insulin resistance and hyperglycaemia(Reference Grundy, Cleeman and Daniels5). In adults, MetS increases the risk of CHD by two-fold and the risk for T2DM by five-fold(Reference Grundy, Cleeman and Daniels5–Reference Grundy7). The age-adjusted prevalence of MetS in US adults (≥20 years) participating in the 2003–2006(Reference Ervin8) National Health and Nutrition Examination Survey (NHANES) was 34 % v. 29·2 % reported in the 1988–1994 NHANES(Reference Ford, Giles and Mokdad9). Among young adults (20–39 years), the prevalence of MetS has increased from 10·8 % (in 1988–1994)(Reference Ford, Giles and Mokdad9) to 15·6 % (in 2003–2006) in females(Reference Ervin8), and from 15·7 % (in 1988–1994)(Reference Ford, Giles and Mokdad9) to 20·3 % (in 2003–2006)(Reference Ervin8) in males. Young adulthood is an important period of transition from adolescence into adulthood, when individuals begin to live an independent life. Pressures of independence, hurried lifestyles and providing support for new families may lead to shifts in their dietary and lifestyle patterns. Consequently, unhealthy dietary habits such as skipping breakfast(Reference Nicklas, Myers, Reger, Beech and Berenson10), relying on fast food(Reference Pereira, Kartashov, Ebbeling, Van Horn, Slattery, Jacobs and Ludwig11) and eating outside home(Reference Clemens, Slawson and Klesges12) are prevalent among young adults. Moreover, individuals from rural and semi-rural US communities tend to have poorer dietary and health habits because of their lower socio-economic status (SES)(Reference Deshmukh-Taskar, Nicklas, Yang and Berenson13–Reference Smith, Lensing, Horton, Lovejoy, Zaghloul, Forrester, McGee and Bogle15). It is therefore critical to examine the DP of young adults and their relation to risk factors for chronic diseases in order to administer effective dietary and lifestyle prevention and treatment programmes for metabolic disorders such as the MetS, in this age group.
Despite the rising prevalence of MetS, few recent studies have examined the role of DP and their relationship with MetS(Reference Lutsey, Steffen and Stevens16–Reference Sonnenberg, Pencina, Kimokoti, Quatromoni, Nam, D’Agostino, Meigs, Ordovas, Cobain and Millen20); their results, in general, showed that healthy DP were inversely associated with the occurrence of MetS in adults(Reference Lutsey, Steffen and Stevens16–Reference Sonnenberg, Pencina, Kimokoti, Quatromoni, Nam, D’Agostino, Meigs, Ordovas, Cobain and Millen20). However, to date only one Bogalusa Heart Study (BHS)(Reference Yoo, Nicklas, Baranowski, Zakeri, Yang, Srinivasan and Berenson21) has examined the relationship of diet with MetS in young adults, and showed that lower fruit and vegetable consumption and higher sweetened beverage consumption were independently associated with one to two risk factors for MetS(Reference Yoo, Nicklas, Baranowski, Zakeri, Yang, Srinivasan and Berenson21). Yet, the above-mentioned BHS explored the association of only single food groups rather than DP with MetS.
The present study, although an extension of the previous BHS(Reference Yoo, Nicklas, Baranowski, Zakeri, Yang, Srinivasan and Berenson21), had two additional objectives. First, it aimed to identify various DP among young adults and to examine the association of these DP with the risk factors of MetS. Second, because food consumption differs by SES and demographic factors such as gender and ethnicity(Reference Deshmukh-Taskar, Nicklas, Yang and Berenson13), and the occurrence of MetS is related to lifestyle factors such as physical inactivity, smoking and alcohol consumption(Reference Zhu, St-Onge, Heshka and Heymsfield22), the present study also assessed SES, and demographic and lifestyle differences among the DP in these young adults.
Methods
Study design and participants
The BHS was conducted in the semi-rural community of Washington Parish, Bogalusa, which is 70 miles north of New Orleans, LA(Reference Berenson23). The study began in 1973 as a long-term epidemiological investigation of cardiovascular risk factors and their environmental determinants in a bi-racial (black/white) paediatric population. Eventually, the study was expanded to include observations of young adults. Details of the BHS study design, participation rates and protocols are presented elsewhere(Reference Berenson23). Data for the present study were collected during a follow-up post-high school cross-sectional survey conducted in 1995–1996 on young adults aged 19–39 years (mean age 30 (sd 5·1) years). Data on ninety-four subjects were excluded from an initial sample of 1089 subjects: i.e. females with energy intakes <2092 kJ (500 kcal) or >14 644 kJ (3500 kcal)(Reference Willett2) (n 47), males with energy intakes <3347 kJ (800 kcal) or >16 736 kJ (4000 kcal)(Reference Willett2) (n 23) and pregnant and/or lactating females (n 24). The final sample (n 995) was 61 % females and 39 % males, with 80 % whites and 20 % blacks. The present study was approved by the Tulane Medical Center Institutional Review Board, and written informed consent was obtained from all the participants.
Measurements
Dietary measures
All young adults from the present study completed the youth/adolescent questionnaire (YAQ), a 131 food-item, self-administered, semi-quantitative food-frequency questionnaire(Reference Rockett, Breitenbach, Frazier, Witschi, Wolf, Field and Colditz24, Reference Rockett, Wolf and Colditz25). The YAQ is valid and reliable for use in epidemiological studies(Reference Rockett, Breitenbach, Frazier, Witschi, Wolf, Field and Colditz24, Reference Rockett, Wolf and Colditz25). Briefly, this questionnaire included specific foods/beverages (including alcohol) along with a commonly used unit or portion size. Each food/beverage item provided three to six possible responses, ranging from ‘never or less than once a month’ to ‘five or more times per day.’ Participants indicated how often, on average, they had consumed a given amount of the specified food/beverage during the past year. Usual portion sizes were calculated for each of the food/beverage items. The selected frequency choice indicated by the participants for each food/beverage was converted to daily intake, e.g. one serving/week was converted to 0·14 serving/d. Food/beverage items were then grouped into specific food categories (as reported earlier)(Reference Deshmukh-Taskar, Nicklas, Yang and Berenson13), and were further categorised based on food characteristics, e.g. refined or whole grains, low-fat or high-fat dairy foods and so on. Thirty-six food groups from the YAQ were identified for the analyses, of which twenty-four food groups representing the DP from the current study are presented in Table 1.
Table 1 Components of food groupsFootnote * included in the DP from the YAQ: The Bogalusa Heart Study
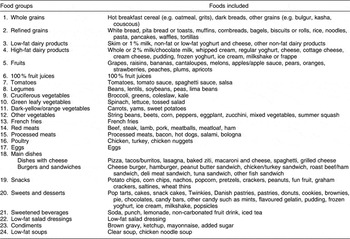
DP, dietary patterns; YAQ, youth and adolescent food frequency questionnaire.
* Only twenty-four food groups identified in the DP (from Table 2) are discussed above from a total of thirty-six food groups from the YAQ.
Anthropometric measures
Duplicate measures of all anthropometric parameters were collected by trained examiners using standardised protocols(Reference Berenson23); least-square means and their standard errors are presented. Height was measured to the nearest 0·1 cm on a stadiometer and weight was measured to the nearest 0·1 kg on a balance-beam metric scale. The National Heart, Lung and Blood Institute reference standards(26) were used to classify participants’ BMI (weight (kg)/height2 (m2)) into normal weight (BMI ≥ 18·5 and ≤24·9 kg/m2) or overweight/obese (BMI ≥ 25 kg/m2). Young adults who were underweight (BMI < 18·5 kg/m2) were included with those in the normal weight category. Waist circumference was measured midway between the rib cage and superior border of the iliac crest. Hip circumference was measured at the greater trochanters. Waist-to-hip ratio was calculated. Triceps skinfold was measured to the nearest millimetre with Lange skinfold calipers (Cambridge Scientific Industries, Inc., Cambridge, MD, USA). A description of the reproducibility of these measures used in the BHS is discussed elsewhere(Reference Berenson23).
Laboratory measures
Venous blood was collected following a 12 h fast. Plasma glucose concentrations were measured by the glucose oxidase method using a Beckman glucose analyzer (Beckman Instruments, Fullerton, CA, USA); a commercial radioimmunoassay kit measured plasma immunoreactive insulin concentration (Padebas Pharmacia, Piscataway, NJ, USA). Indices of insulin sensitivity were calculated according to the Quantitative Insulin Sensitivity Check Index (QUICKI) formula (=1/(log fasting plasma glucose (mg/dl) + log fasting plasma insulin (μU/ml)); higher QUICKI values indicate greater insulin sensitivity)(Reference Katz, Nambi, Mather, Baron, Follmann, Sullivan and Quon27); and the Homeostasis Model Assessment of insulin resistance (HOMA-IR) formula (=(fasting plasma glucose (mg/dl) × fasting plasma insulin (μU/ml))/405; higher HOMA values indicate greater insulin resistance)(Reference Mathews, Hosker, Rudenski, Naylor, Treacher and Turner28). Serum total cholesterol and TAG concentrations were measured using enzymatic procedures on the Abbott VP instrument (Abbott Laboratories, North Chicago, IL, USA), and serum LDL cholesterol (LDL-C) and HDL cholesterol (HDL-C) were analysed using a combination of heparin–calcium precipitation and agar–agarose gel electrophoretic procedures(Reference Srinivasan and Berenson29). Blind duplicates were used for quality control for all analyses(Reference Berenson23). Right arm systolic blood pressure (SBP) and diastolic blood pressure (DBP) were measured in triplicate by trained nurses(Reference Berenson23), using the first and fifth Korotkoff phase readings with the participant sitting relaxed, and also using the automated instrument (the readings of which were used in the present study). Means of all replicate measures were used for statistical analyses.
Diagnostic criteria for metabolic syndrome
Several definitions exist for MetS(Reference Assmann, Guerra, Fox, Cullen, Schulte, Willett and Grundy30). The International Diabetes Federation (IDF) criteria help to generate greater prevalence estimates for MetS (especially, in the European population) by using central obesity as a mandatory criterion. Conversely, the revised Adult Treatment Panel (ATP III)(Reference Grundy, Cleeman and Daniels5) criteria mandate the selection of a wider range of risk factors for identifying individuals with MetS (as discussed below), with no single mandatory criterion. Although, in the USA, both the IDF and revised ATP III criteria identify mostly the same people, the IDF criteria have a lower predictive power for coronary events(Reference Assmann, Guerra, Fox, Cullen, Schulte, Willett and Grundy30). Therefore, in the present study, the young adults were classified as having MetS using the revised ATP III criteria(Reference Grundy, Cleeman and Daniels5, Reference Assmann, Guerra, Fox, Cullen, Schulte, Willett and Grundy30). Data on medications prescribed to the young adults to increase serum HDL-C and/or lower serum TAG were not available in the present data set. Therefore, we slightly modified the criteria for serum TAG and HDL-C from the original revised ATP III criteria(Reference Grundy, Cleeman and Daniels5, Reference Assmann, Guerra, Fox, Cullen, Schulte, Willett and Grundy30).
The criteria for classifying young adults with MetS for the present study were ≥3 of the following risk factors: (i) abdominal obesity (waist circumference ≥ 102 cm in males and ≥88 cm in females); (ii) high serum TAG (≥150 mg/dl); (iii) low serum HDL-C (<40 mg/dl in males or <50 mg/dl in females); (iv) high blood pressure (≥130 or ≥85 mmHg or those taking medications for hypertension); and (v) high fasting plasma glucose (≥100 mg/dl or those taking medications, i.e. oral hypoglycaemic agents/insulin).
Demographic, socio-economic status and lifestyle information
Participants completed a questionnaire eliciting information on their age, gender, ethnicity, smoking status (i.e. non-smoker, current smoker and ex-smoker) and alcohol intake (based on the frequency, type and length of alcohol use during the past 12 months). White/black males and females were classified into four ethnicity×gender groups. The SES of the young adults was determined using income (i.e. ≤$15 000, $15 001–30 000, $30 001–45 000, >$45 000) and education levels (i.e. ≤12 years or >12 years). To determine marital status, young adults were asked whether they were currently married and/or cohabiting or were single. Physical activity outside work was measured with a self-reported subjective rating on a 5-item Likert scale adapted from the Lipid Research Clinic’s questionnaire(Reference Ainsworth, Jacobs and Leon31). Participants were considered sedentary if they classified themselves as 1 or 2; were considered moderately active if they classified themselves as 3; and were considered very active if they classified themselves as 4 or 5 on the Likert scale. The test–retest reliability of this questionnaire has been reported to be high (r = 0·85). Also, this questionnaire has been noted to be significantly associated with a 4-week physical activity history(Reference Ainsworth, Jacobs and Leon31).
Data analysis
The Statistical Analysis Software (version 8·2; SAS Inc., Cary, NC, USA)(32) was used to conduct data analyses. To identify the DP, principal components factor analysis was conducted. Factor analysis helps to summarise and refine large data sets containing several variables, simultaneously, into a small number of orthogonal variables named as ‘patterns’. Factor analysis has earlier shown to have good reproducibility and validity with data from a food frequency questionnaire(Reference Hu, Rimm, Smith-Warner, Feskanich, Stampfer, Ascherio, Sampson and Willett33).
In the present study, thirty-six food groups from the YAQ were subjected to principal component factor analysis with varimax rotation to identify the DP. Specific food items were aggregated based on the degree to which the food items were correlated with one another in the data set. Eigenvalues (>1), the scree test (a graph from which the number of factors were chosen where the plot levelled off to a linear decreasing pattern) and interpretability of derived factors were used to derive the DP. Linear regression examined the association between DP and MetS risk factors (dependent variables). Analysis of covariance with Tukey–Kramer’s post-hoc test was used to examine: (i) ethnicity × gender differences in the occurrence of metabolic risk factors (dependent variables); and (ii) differences in mean servings of foods from the DP (dependent variables) by SES, demographic and lifestyle characteristics. The mean number of servings of foods consumed from the DP was used in the latter analyses because factor scores by themselves have no biological meaning. The covariates varied for each analyses and included age, energy intake, gender, ethnicity, ethnicity × gender, SES, marital status, physical activity, smoking, alcohol consumption and BMI. Statistical significance was set at P ≤ 0·05.
Results
Identification of dietary patterns (Table 2)
Factor analysis retained two DP, which contained twenty-four of the original thirty-six food groups from the YAQ. The DP were labelled as: the ‘Western Dietary Pattern’ (WDP; consisting of refined grains, French fries, high-fat dairy products, dishes with cheese, red meats, processed meats, eggs, snacks, sweets and desserts, sweetened beverages and condiments) and the ‘Prudent Dietary Pattern’ (PDP; consisting of whole grains, legumes, vegetables (i.e. cruciferous, other leafy and dark-yellow vegetables), tomatoes, fruits, 100 % fruit juices, low-fat dairy products, poultry, clear soups and low-fat salad dressings). The WDP and the PDP explained 19 % and 12 % of the dietary intake variance, respectively.
Table 2 Identification of DP from factor loadingsFootnote * for foods from the YAQ: The Bogalusa Heart Study

DP, dietary patterns; YAQ, youth and adolescent food frequency questionnaire; WDP, Western dietary pattern; PDP, prudent dietary pattern.
* Data (1–24) are factor loadings (correlation coefficients between the variables and factors) derived from principal component factor analysis. Absolute values of factor loadings <0.30 are indicated by ‘–’ for simplicity.
Covariate-adjusted mean metabolic profiles of young adults (Table 3)
Among the four ethnicity × gender groups, white females had the lowest energy intake, waist circumference, waist-to-hip ratio and SBP; white males had the highest waist circumference and waist-to-hip ratio but the lowest serum HDL-C; and black males had the highest SBP. Compared to white males, white females had lower energy intake, BMI, waist circumference, waist-to-hip ratio, SBP, DBP, plasma glucose, serum total cholesterol, LDL-C and TAG and physical activity, but higher triceps skinfold and serum HDL-C. Compared to black males, black females had higher triceps skinfold and serum HDL-C, but lower waist-to-hip ratio, SBP and DBP. Compared to black females, white females had lower energy intake, BMI, waist circumference, waist-to-hip ratio, SBP, plasma insulin and serum HDL-C, but higher serum TAG. Compared to black males, white males had higher waist circumference and waist-to-hip ratio, but lower SBP and serum HDL-C.
Table 3 Covariate-adjusted metabolic profiles of young adultsFootnote * (19–39 years) by ethnicity and gender: The Bogalusa Heart Study

HDL-C, HDL cholesterol; LDL-C, LDL cholesterol; MetS, metabolic syndrome.
a,b,c,d Mean (percentage) values within a row with unlike superscript letters were significantly different (P < 0·01).
* All young adults (n 995) were fasting before the blood draw.
† Least-square means for dependent variables compared by analysis of covariance and Tukey–Kramer’s post hoc test.
‡ 1 kcal = 4194 kJ.
§ Normal weight, BMI ≥ 18·5 and ≤24·9 kg/m2; overweight/obese, BMI ≥ 25·0 kg/m2.
∥ Diagnosis of MetS based on ≥3 of the following risk factors: waist circumference ≥102 cm in males and ≥88 cm in females; serum TAG ≥ 150 mg/dl; serum HDL-C < 40 mg/dl in males and <50 mg/dl in females; blood pressure ≥130 or ≥85 mmHg or taking medications for hypertension; and fasting plasma glucose ≥100 mg/dl or taking medications (oral hypoglycaemic agents/insulin).
¶ Energy intake model adjusted for age, socio-economic status (SES), smoking status, alcohol intake and physical activity.
** Obesity measurement model(s) adjusted for energy intake, age, SES, smoking status, alcohol intake and physical activity.
†† Physical activity model (1 = sedentary, 3 = moderately active and 5 = very active) adjusted for energy intake, age and SES.
‡‡ Blood/plasma/serum parameters model(s) adjusted for energy intake, age, SES, smoking status, alcohol intake and physical activity.
The overall occurrence of MetS in young adults was 12·2 %, with 14·9 % in males v. 10·4 % in females (P = 0·03, data not shown). No ethnic differences in the occurrence of MetS were observed (12·8 % in whites v. 9·6 % in blacks (P = 0·22, data not shown)). However, black males had a higher occurrence of Mets than black females (15·4 % v. 5·8%; P = 0·03).
Covariate-adjusted associations between dietary patterns and components of metabolic syndrome (Table 4)
Using the covariate-adjusted model (excluding BMI), waist circumference, triceps skinfold, plasma insulin and the occurrence of MetS were all inversely associated with the PDP. Insulin sensitivity was positively associated with the PDP. Serum TAG was negatively associated with both PDP and WDP. After adjusting for BMI in addition to other covariates, serum HDL-C was inversely associated with the WDP. The overall occurrence of MetS did not differ by the two DP.
Table 4 Covariate-adjusted associations between risk factors for MetSFootnote * and DP in young adults (19–39 years)

MetS, metabolic syndrome; DP, dietary patterns; PDP, prudent dietary pattern; WDP, Western dietary pattern; Std β, standardised β; HOMA-IR, Homeostasis Model Assessment of insulin resistance; QUICKI, Quantitative Insulin Sensitivity Check Index; LDL-C, LDH cholesterol; HDL-C, HDL cholesterol.
* Diagnosis of MetS based on ≥3 of the following risk factors: waist circumference ≥ 102 cm in males and ≥88 cm in females; serum triacylglycerol ≥ 150 mg/dl; HDL-C < 40 mg/dl in males and <50 mg/dl in females; blood pressure ≥ 130 or ≥85 mmHg or taking medications for hypertension; and fasting plasma glucose ≥ 100 mg/dl or taking medications (oral hypoglycaemic agents/insulin).
† Model 1 adjusted for age, energy intake, ethnicity, gender, ethnicity × gender, socio-economic status (SES), physical activity, alcohol intake and smoking status.
‡ Model 2 adjusted for BMI in addition to age, energy intake, ethnicity, gender, ethnicity × gender, SES, physical activity, alcohol intake and smoking status.
§ BMI calculated as weight (kg)/height2 (m2) with normal weight defined as BMI ≥ 18·5 and ≤24·9 kg/m2; and overweight/obese defined as BMI ≥ 25·0 kg/m2.
Covariate-adjusted demographic, socio-economic status and lifestyle differences in dietary patterns (Table 5)
Overall, young adults consumed more servings from the WDP than the PDP (mean 9·8 (sd 0·2) v. 4·5 (sd 0·2); P < 0·0001, data not shown). Blacks consumed more servings from the WDP than whites, and females consumed more servings from the PDP than males. Whites (males and females) consumed fewer servings from the WDP than black females. White females consumed more servings from the PDP than white males. Older young adults (30–39 years) consumed more servings from the PDP than their younger age group counterparts (19–24 years).
Table 5 Covariate-adjusted demographic, SES and lifestyle differences in servings of foods from DP among young adults (19–39 years): The Bogalusa Heart Study

SES, socio-economic status; DP, dietary patterns; PDP, prudent dietary pattern; WDP, Western dietary pattern.
a,bMean values within a column with unlike superscript letters were significantly different by analysis of covariance and Tukey–Kramer’s post hoc test (P<0·05).
*Sample size differs from the original sample (n 995) due to missing data for some subjects for SES, physical activity and marital status.
†Fully adjusted model controlled for age, energy intake, gender, ethnicity, ethnicity × gender, SES, physical activity, smoking status, alcohol intake and marital status.
‡Unadjusted model.
§Partially adjusted model controlled for gender, ethnicity and ethnicity × gender.
A higher percentage of young adults reported to be in the income category of <$15 000 (27·8 %) compared to $30 001–45 000 (20·6 %; P = 0·006, data not shown). Young adults reporting an income level of >$45 000 consumed more servings of the PDP than those reporting lower income, who consumed more servings from the WDP (income model showed significance when adjusted for only gender, ethnicity and ethnicity × gender, but not other covariates). Young adults with >12 years of education consumed more servings from the PDP than those with an education ≤12 years, who consumed more servings from the WDP. Current smokers consumed more servings from the WDP than current non-smokers, who consumed more servings from the PDP. Those who were physically very active (level 5) consumed fewer servings from the WDP than those who were sedentary (level 2).
Discussion
Factor analysis discerned two prominent DP in the present study, the ‘WDP’ mainly characterised by high-fat and high-refined carbohydrate foods, and the ‘PDP’ mainly characterised by low-fat and low-refined carbohydrate foods. A growing body of evidence suggests that increased consumption of healthier foods, including fruits and vegetables(Reference Bazzano, He, Ogden, Loria, Vupputuri, Myers and Whelton34–Reference Rolls, Ello-Martin and Tohill37), whole grains/cereals(Reference Qi, van Dam, Liu, Franz, Mantzoros and Hu38), dairy products(Reference Zemel, Richards, Milstead and Campbell39) and other low-fat foods(Reference Johnston, Tjonn and Swan40), may prevent chronic nutrition-related diseases mainly by their vitamin/mineral(Reference Zemel, Richards, Milstead and Campbell39, Reference Tucker41), phytochemical(Reference Tucker41) and fibre content(Reference Hallfrisch and Behall42, Reference Steffen, Jacobs, Murtaugh, Moran, Steinberger, Hong and Sinaiko43). For example, whole grains have lower glycaemic index and higher fibre content than refined grains, and their consumption may increase insulin sensitivity(Reference Steffen, Jacobs, Murtaugh, Moran, Steinberger, Hong and Sinaiko43) and plasma levels of anti-inflammatory cytokines (e.g. adiponectin)(Reference Yannakoulia, Yiannakouris, Melistas, Kontogianni, Malagaris and Mantzoros44) and reduce serum markers of systemic inflammation (e.g. C-reactive protein and tumour necrosis factor alpha-receptor 2)(Reference Qi, van Dam, Liu, Franz, Mantzoros and Hu38). The calcium content in dairy foods has been hypothesised to lower central obesity and insulin resistance(Reference Zemel, Richards, Milstead and Campbell39, Reference Pereira, Jacobs, Van Horn, Slattery, Kartashov and Ludwig45). Increased consumption of fruits and vegetables has been associated with lower incidence of stroke(Reference Bazzano, He, Ogden, Loria, Vupputuri, Myers and Whelton34), ischaemic heart disease(Reference Bazzano, He, Ogden, Loria, Vupputuri, Myers and Whelton34), hypertension(Reference Alonso, de la Fuente, Martin-Arnau, de Irala, Martinez and Martinez-Gonzalez35), T2DM(Reference Williams, Wareham, Cox, Byrne, Hales and Day36) and increased satiety(Reference Rolls, Ello-Martin and Tohill37), that may help to reduce body weight. Conversely, consumption of energy-dense (i.e. high-fat and/or high-refined carbohydrate foods) may contribute to a surplus intake of ‘discretionary calories’ in the diet (46) and may contribute to the prevalence of overweight/obesity and related chronic diseases over time.
The present study found that several risk factors for CHD, T2DM and MetS were associated with the DP (especially, the PDP). The occurrence of MetS (i.e. more than or equal to three MetS risk factors) was inversely associated with the PDP; however, no association was noted in the occurrence of MetS with the WDP in this study. In a recent longitudinal study(Reference Lutsey, Steffen and Stevens16), participants who were in the highest quintile of the WDP scores (comprising of refined grains, processed meat, fried foods and red meat) had an 18 % greater risk of MetS than those in the lowest quintile for the WDP scores; however, in the same study, consumption of the PDP was not associated with MetS(Reference Lutsey, Steffen and Stevens16). In another cross-sectional study(Reference Williams, Prevost, Whichelow, Cox, Day and Wareham19), a dietary pattern characterised by a healthy balanced diet (with a frequent intake of raw and salad vegetables, fruits, fish, pasta and rice, and low intake of fried foods, sausages, fried fish and potatoes) was inversely correlated with central obesity, plasma glucose and TAG, and positively correlated with plasma HDL-C. The above dietary pattern(Reference Williams, Prevost, Whichelow, Cox, Day and Wareham19) was also negatively associated with the risk of having undiagnosed diabetes, and this association was independent of age, gender, smoking and obesity.
In our present study, the finding of serum HDL-C being inversely associated with the WDP (after controlling for BMI and other covariates) is not in agreement with earlier theories(Reference Samaha47, Reference Pelkman, Fishell, Maddox, Pearson, Mauger and Kris-Etherton48). In general, diets high in saturated fatty acids tend to increase the cardio-protective serum HDL-C levels along with increasing other CHD-causing lipids (e.g. serum total cholesterol)(Reference Samaha47). Conversely, low-fat, high-carbohydrate diets tend to decrease serum HDL-C, but also decrease serum total cholesterol and LDL-C(Reference Pelkman, Fishell, Maddox, Pearson, Mauger and Kris-Etherton48).Whether the consumption of a mixture of high-fat and high-refined carbohydrate foods from the WDP led to the inverse association of serum HDL-C with the WDP, or whether the adjustment of BMI as a covariate in the model led to this finding, is not fully understood.
We also found an inverse association of serum TAG with both the DP. Diets high in refined carbohydrates and low in fat, tend to increase serum TAG owing to increased VLDL cholesterol TAG secretion, which is a result of increased hepatic fatty acid availability due to lower fatty acid oxidation(Reference Pelkman, Fishell, Maddox, Pearson, Mauger and Kris-Etherton48). The WDP included a mixture of high-fat and high-refined carbohydrate foods that may have resulted in the inverse association of serum TAG with the WDP. Conversely, the inverse association of serum TAG with the PDP could be because of the consumption of low-refined carbohydrate foods (e.g. whole grains, legumes, vegetables and so on) as well as consumption of some low-fat foods (e.g. low-fat dairy products, poultry and low-fat salad dressings). Nevertheless, further investigation in this area is warranted.
Blacks and white males had more risk factors for MetS than white females in the present study. Further, blacks consumed more servings of the WDP, and females consumed more servings from the PDP. Earlier research has reported that blacks were less likely to modify meats to make them lower in fat and ate more fried foods than whites(Reference Gans, Burkholder, Risica and Lasater49). Ethnic disparities in dietary intakes could be attributed to the fact that a larger number of blacks are in the lower SES group than whites(Reference Goodman, Adler, Daniels, Morrison, Slap and Dolan50) and hence may consume poorer diets(Reference Deshmukh-Taskar, Nicklas, Yang and Berenson13, Reference Champagne, Bogle, McGee, Yadrick, Allen, Kramer, Simpson, Gossett and Weber14) owing to either their inability to afford healthier foods(Reference Drewnowski51), or may have a decreased accessibility to healthier foods(Reference Baker, Schootman, Barnidge and Kelly52). White females had the lowest BMI, waist circumference and waist-to-hip ratio than other ethnicity × gender groups, and consumed fewer servings from the WDP than black females. Black females (especially, from low SES groups) tend to be less health conscious than white females, and hence may be less likely to choose healthy dietary and lifestyle patterns(Reference Colhoun and Prescott-Clarke53, Reference Duncan, Anton, Newton and Perri54).
In the present study, young adults in the higher SES group (especially, those with higher education) consumed more servings from the PDP. Similar results with respect to SES and food group consumption were suggested earlier(Reference Deshmukh-Taskar, Nicklas, Yang and Berenson13, Reference Williams, Prevost, Whichelow, Cox, Day and Wareham19). The relationship between higher SES and the consumption of a PDP could be because of increased knowledge and health awareness, or increased pressures of social acceptability that occur with the increasing SES, which may influence their food consumption habits(Reference Colhoun and Prescott-Clarke53). Among the lower SES groups, the increased cost of healthier foods or decreased access to healthier foods may be important factors influencing their food choices(Reference Drewnowski51, Reference Baker, Schootman, Barnidge and Kelly52).
Current smokers in the present study consumed more servings of the WDP than current non-smokers who consumed more servings from the PDP. Those young adults who reported to be in the highest level of physical activity consumed lesser number of servings from the WDP than those who reported to be less physically active. Often, healthy dietary and lifestyle habits tend to cluster together among individuals. In a recent study(Reference Delahanty, DiClemente, Havas and Langenberg55), relative to non-smokers, current smokers reported higher overall energy intake, higher percentages of energy from fat, sweets and alcohol, and a lower percentage of energy from protein among low-income women. Yet, our study failed to find differences in DP by alcohol consumption and marital status.
The use of factor analysis to identify DP is a major strength of the present study. Although factor analysis takes into account the issue of high inter-correlations of foods within the diet, decisions based on factor loadings may be subjective or arbitrary and can affect the study results and interpretation(Reference Martinez, Marshall and Sechrest56). Nevertheless, a recent study depicted that the young adult age-group frequently consumed less healthier foods(Reference Pereira, Kartashov, Ebbeling, Van Horn, Slattery, Jacobs and Ludwig11) resembling the WDP from the current study, suggesting that the factor loadings from our study are robust and the DP are meaningful. Also, the similarity in the results on DP and MetS from our study to those reported in longitudinal(Reference Lutsey, Steffen and Stevens16) and cross-sectional studies(Reference Panagiotakos, Pitsavos, Skoumas and Stefanadis17–Reference Sonnenberg, Pencina, Kimokoti, Quatromoni, Nam, D’Agostino, Meigs, Ordovas, Cobain and Millen20) strengthens the present findings.
The present study has some limitations. Owing to its cross-sectional design, causal inferences cannot be made(Reference Gordis57). Despite the large sample size, blacks were under-represented. The findings from this study may be specific to young adults of Bogalusa, and are not representative of national findings. The YAQ, which was originally developed for the dietary assessment of adolescents, was used for the dietary assessment of young adults in the current study. In comparison with a 24 h dietary recall, the YAQ has been more helpful in characterising snack food consumption among the young adults(Reference Nicklas58). Further, the energy intake when measured by YAQ or the 24 h dietary recall in young adults has been similar(Reference Nicklas58). Lastly, the dietary data used in our study were collected over 10 years ago; yet, it is not uncommon to publish results from long-term epidemiological studies with data that were collected earlier (e.g. the Framingham offspring cohort study)(Reference Sonnenberg, Pencina, Kimokoti, Quatromoni, Nam, D’Agostino, Meigs, Ordovas, Cobain and Millen20, Reference Imamura, Lichtenstein, Dallal, Meigs and Jacques59). The present BHS findings are thus still noteworthy, as they provide valuable information on the role of DP, MetS and its association with sociodemographic and lifestyle factors. These findings may also help to generate new hypotheses for future research.
Conclusions
Overall, DP are important in identifying relationships with occurrence of diseases such as the MetS. Specifically, a prudent balanced dietary pattern may be helpful in preventing MetS in this sample of BHS young adults. More studies are warranted to confirm these findings in other populations. Nonetheless, nutrition intervention programmes for young adults to promote healthy dietary and lifestyle habits tailored-based on their SES, demographic and lifestyle characteristics, may be beneficial.
Acknowledgments
The present study was supported by funds from the United States Department of Agriculture (USDA), Economic Research Service Cooperative Agreement No. 43-3AEL-2-80122; the National Heart, Lung and Blood Institute, grant HL-38844; National Institute of Aging grant AG-16592; and USDA Hatch 940-36-3104 Project 93673 and LAB936760199070. This work is a publication of the United States Department of Agriculture (USDA/ARS) Children’s Nutrition Research Center, Department of Pediatrics, Baylor College of Medicine, Houston, TX. The contents of this publication do not necessarily reflect the views or policies of the USDA, nor does mention of trade names, commercial products, or organisations imply endorsement from the US government. The contributions of the authors were as follows: P.R.D-T. interpreted the data, designed and wrote the manuscript; C.O.N. reviewed and edited the manuscript; T.A.N. designed the study and edited the manuscript; S.J.Y. and Y.L. conducted the statistical analyses; J.G. contributed to the initial conceptualising of the study; and G.S.B. directed and designed the study. None of the authors had a personal or financial conflict of interest. The authors thank the many people who contributed to the Bogalusa Heart Study collaborative effort. The authors also thank the children and young adults of Bogalusa, without whom this study would not have been possible. The authors would like to thank Ms Bee Wong for library assistance and Ms Pam Harris for submitting the manuscript.