Introduction
Major depressive disorder (MDD) is a disabling and highly recurrent psychiatric disorder (American Psychiatric Association, 2000; Treadway et al., Reference Treadway, Waskom, Dillon, Holmes, Park, Chakravarty and Pizzagalli2015). The current challenge in the clinical treatment of MDD is maximizing the treatment response and preventing its recurrence. Second-generation antidepressants are the first-line therapeutic strategies for MDD in inpatient and outpatient clinics (Zhang et al., Reference Zhang, Chen, Yue, Liu, Montgomery, Zhi and Wang2016). However, the treatment response to antidepressants varies between individuals (Trivedi et al., Reference Trivedi, Rush, Wisniewski, Nierenberg, Warden, Ritz and Team2006). Studies have demonstrated that approximately one-third of the patients with MDD were unable to achieve full remission even after being administered two or more antidepressants at adequate doses for more than 8 weeks of continued therapy (Angstman, Pietruszewski, Rasmussen, Wilkinson, & Katzelnick, Reference Angstman, Pietruszewski, Rasmussen, Wilkinson and Katzelnick2012; Han et al., Reference Han, Wang, Chan, Kato, Ng, Tan and Liu2020; Sinyor, Schaffer, & Levitt, Reference Sinyor, Schaffer and Levitt2010). In addition, at least one-third of the patients developed a recurrent episode after recovery from the previous depressive episode (Kanai et al., Reference Kanai, Takeuchi, Furukawa, Yoshimura, Imaizumi, Kitamura and Takahashi2003; Moffitt et al., Reference Moffitt, Caspi, Taylor, Kokaua, Milne, Polanczyk and Poulton2010). With each successive episode, there is an increased risk for the next recurrence of major depressive episodes in patients (Moffitt et al., Reference Moffitt, Caspi, Taylor, Kokaua, Milne, Polanczyk and Poulton2010).
Treatment non-response and recurrence were reportedly the main contributors to the disease burden in patients with MDD (Dennehy et al., Reference Dennehy, Robinson, Stephenson, Faries, Grabner, Palli and Marangell2015; Gauthier, Mucha, Shi, & Guerin, Reference Gauthier, Mucha, Shi and Guerin2019; Touya et al., Reference Touya, Lawrence, Kangethe, Chrones, Evangelatos and Polson2022). However, there is a lack of knowledge about the neural mechanism involved in antidepressant treatment related to long-term treatment outcomes in MDD. As a non-invasive neuroimaging technique with a high spatiotemporal resolution, magnetic resonance imaging (MRI) has been widely used in studying neural biomarkers, including predictors, moderators, and mediators of treatment outcomes in MDD (Kang & Cho, Reference Kang and Cho2020). Many studies have indicated that the individuals' neuroimaging characteristics, especially resting-state functional brain networks, may contain meaningful information that could facilitate MDD diagnosis and personalized treatments (Chin Fatt et al., Reference Chin Fatt, Jha, Cooper, Fonzo, South, Grannemann and Trivedi2019; Korgaonkar, Goldstein-Piekarski, Fornito, & Williams, Reference Korgaonkar, Goldstein-Piekarski, Fornito and Williams2020). However, neuroimaging biomarkers related to long-term treatment outcomes in MDD were largely based on ‘post-hoc’ experimental design. In most of the functional and structural MRI studies investigating neurobiological mechanisms involved in the treatment response, neuroimaging indices were investigated after the patients developed the status of treatment non-responders (Geugies et al., Reference Geugies, Opmeer, Marsman, Figueroa, van Tol, Schmaal and Ruhe2019; Guo et al., Reference Guo, Liu, Xue, Gao, Liu, Xiao and Zhao2013a, Reference Guo, Liu, Xue, Gao, Liu, Xiao and Zhao2013b; Phillips, Batten, Aldosary, Tremblay, & Blier, Reference Phillips, Batten, Aldosary, Tremblay and Blier2012; Phillips, Batten, Tremblay, Aldosary, & Blier, Reference Phillips, Batten, Tremblay, Aldosary and Blier2015a). Considering the neuroimaging biomarkers for depression recurrence, studies either cross-sectionally compared the difference in brain imaging features between patients with recurrent MDD and controls (Cano et al., Reference Cano, Martinez-Zalacain, Bernabeu-Sanz, Contreras-Rodriguez, Hernandez-Ribas, Via and Soriano-Mas2017; Figueroa et al., Reference Figueroa, DeJong, Mocking, Fox, Rive, Schene and Ruhe2019; Liu et al., Reference Liu, Ma, Yuan, Song, Jing, Lu and Wang2017, Reference Liu, Tang, Gao, Zhang, Li, Li and Wang2019) or performed a correlation analysis between the number of depressive episodes and brain features (Jacobs et al., Reference Jacobs, Barba, Gowins, Klumpp, Jenkins, Mickey and Langenecker2016; Liu et al., Reference Liu, Ma, Yuan, Song, Jing, Lu and Wang2017, Reference Liu, Tang, Gao, Zhang, Li, Li and Wang2019; Meng et al., Reference Meng, Brandl, Tahmasian, Shao, Manoliu, Scherr and Sorg2014). The identified biomarkers could result from treatment non-response or recurrence rather than a neural mechanism underlying these phenomena in MDD. Thus, untreated patients should be recruited in a study with a long-term follow-up design to elucidate the cause-and-effect relationship (Kim, Reference Kim2018).
In addition, most prospective brain imaging studies focused on identifying the treatment response predictors at baseline rather than the neurobiological change associated with the treatment response during the treatment phase (Phillips et al., Reference Phillips, Chase, Sheline, Etkin, Almeida, Deckersbach and Trivedi2015b). It is unknown whether these brain imaging features could be useful in treating depression or preventing depressive recurrences. Moreover, previous studies investigating the brain mechanism of antidepressant treatment response focused on acute rather than the long-term effects (Li, Chen, Kong, Li, & Hu, Reference Li, Chen, Kong, Li and Hu2022). Although inconsistent preprocessing and network analytical methods have been conducted, functional connectivity decrease in the default mode network (DMN) after antidepressant treatment in MDD has been widely reported. A study using group-independent component analysis reported a decreased resting-state functional network connectivity (rsFNC, the overall network strength) in the posterior DMN of remitted MDD patients after 12 weeks of antidepressant treatment (Li et al., Reference Li, Liu, Friston, Shen, Wang, Zeng and Hu2013). Another study that conducted seed-based analysis found that a 10-week treatment with duloxetine (not placebo) normalized the increased resting-state functional connectivity (rsFC, the single edge strength) within the DMN in patients with dysthymia (Posner et al., Reference Posner, Hellerstein, Gat, Mechling, Klahr, Wang and Peterson2013). Other studies investigating the short-term (hours to 2 weeks) effects of antidepressants on brain functional connectivity in healthy controls (HC) also detected a decreased rsFC in the DMN (Klaassens et al., Reference Klaassens, van Gorsel, Khalili-Mahani, van der Grond, Wyman, Whitcher and van Gerven2015; Schrantee, Lucassen, Booij, & Reneman, Reference Schrantee, Lucassen, Booij and Reneman2018; van de Ven, Wingen, Kuypers, Ramaekers, & Formisano, Reference van de Ven, Wingen, Kuypers, Ramaekers and Formisano2013; van Wingen et al., Reference van Wingen, Tendolkar, Urner, van Marle, Denys, Verkes and Fernandez2014). Lastly, a large multi-site dataset study found a decreased DMN rsFNC in MDD than in HC (Yan et al., Reference Yan, Chen, Li, Castellanos, Bai, Bo and Zang2019). Interestingly, the decreased DMN rsFNC was identified only in medicated patients but not in drug-naïve patients, suggesting that the decreased DMN rsFNC was related to the antidepressant treatment rather than the disease status. However, scarce evidence exists concerning intrinsic brain network modulation during antidepressant treatment related to long-term treatment outcomes in depression. To the best of our knowledge, no data-driven analysis has been performed to investigate the functional connectivity changes in brain networks across the antidepressant treatment time course as a biomarker for treatment non-response and recurrence in MDD.
Therefore, we investigated the intrinsic brain network changes related to the long-term treatment outcomes in MDD in a relatively large cohort with 2 years of follow-up data. First, we explored the difference in the longitudinal change in the overall network strength between the MDD groups with different treatment outcomes. Moreover, we recruited HC to investigate the MDD baseline intrinsic brain network abnormalities. Furthermore, we applied network connectome-based predictive modeling (nCPM), a machine-learning pipeline, to test whether the edge strength change in certain networks could specifically discriminate patients with different treatment outcomes. This connectome-based data-driven analysis was conducted in connectivity matrices derived from each network, and the whole-brain and model performances were compared. The edge contributing to the classification and prediction of long-term treatment outcomes in MDD was investigated and visualized.
Methods
Participants and study design
The medical ethics committees of the Second Xiangya Hospital of Central South University and the Zhumadian Psychiatric Hospital approved this study. Written informed consent was obtained from all the participants. A total of 235 patients with MDD were recruited via media advertisement or through referral by a clinician from the inpatient or outpatient departments of the Zhumadian Psychiatry Hospital. The inclusion/exclusion criteria and assessments have been mentioned previously (Liu et al., Reference Liu, Dong, Lu, Sun, Zhang, Wang and Li2020; Lu et al., Reference Lu, Guo, Sun, Dong, Zhao, Liao and Li2018) and are provided in the online Supplemental material. Patients aged 18–55 years, acutely depressed, who had not received any psychotropic drug for at least 2 weeks, and with a 24-item Hamilton Rating Scale for Depression (HAMD24) score of at least 20, were enrolled in our study. In addition, a matched control group of 163 healthy volunteers were recruited from nearby communities.
The initial recruitment period was from March 2013 to June 2017. At baseline, all the participants underwent a structured clinical interview based on DSM-4, and their depressive symptoms were assessed using the HAMD24 scale. Demographic data and clinical characteristics were collected, and the MRI scan was then performed. The following patients were excluded from our analyses: those with incomplete clinical data (N = 10) or MRI scan images (N = 16); those who had excessive head motion (defined as mean frame to frame displacement (FFD) > 0.15 mm, N = 21); those who experienced manic episodes (N = 6); and those who received brain stimulation treatment [electroconvulsive therapy/repetitive transcranial magnetic stimulation (TMS), N = 11] during the 6-month study period (Fig. 1). Besides, HC with incomplete MRI scan images (N = 18) or with excessive head motion (N = 9) were excluded from our analyses. Therefore, 171 patients with MDD and 136 HC who met the inclusion criterion were included at baseline.

Fig. 1. Study design. Of the initial 235 patients with MDD, patients with in-complete clinical (N = 10) or MRI scan (N = 16), excessive head motion (defined as FFD > 0.15 mm, N = 21), those who experienced manic episodes (N = 6), and those who received brain stimulation treatment (ECT/rTMS, N = 11) during the 6-month study period were excluded. Patients were assessed using HAMD24 scale at baseline, 2 weeks after baseline, and then monthly until 6 months post-treatment. One hundred and twenty-two patients returned for the MRI scan and depressive symptom assessments following the 6-month antidepressant treatment. Twelve patients with excessive head motion were excluded. Eventually, 84 remitters and 26 non-remitters with low-motion MRI scans were included in our first phase analyses. After the first phase, patients were followed-up via phone calls or at out-patient clinic settings once every 6 months. Among the 84 patients who achieved remission, 68 patients completed 2 years of follow-up re-assessments, and 28 recurring MDD and 40 stable MDD patients were included in our second phase analyses.
Details of the study design are provided in Fig. 1. The first phase of patient follow-up assessment was from antidepressant treatment initiation to 6 months post-treatment. During the first phase, patients were prescribed antidepressants according to the clinical judgment. Details of the antidepressant administration are provided in the online Supplemental material. The main outcome of the first phase was remission, defined as an HAM-D24 score < 8 (DeRubeis et al., Reference DeRubeis, Zajecka, Shelton, Amsterdam, Fawcett, Xu and Hollon2020). After 6 months of antidepressant treatment, 122 patients with MDD returned for the MRI scans and clinical assessments. Eighty-eight patients achieved remission before the 6-month follow-up time point and were defined as remitters. Thirty-four patients did not meet the remission criteria after 6-month long-term antidepressant treatment (receiving two or more antidepressants, adequate in dose, duration, and compliance) and were defined as sustained non-remitters. Eventually, our first phase analyses included 84 remitters and 26 non-remitters with low-motion MRI scans.
After the first phase, patients were followed-up via phone calls or at outpatient clinic settings once every 6 months. During the second phase, patients continued antidepressant treatment according to the psychiatrists' clinical judgment. The second phase of the follow-up assessment was from October 2013 to December 2019. The main outcome of the second phase was recurrence. Structured clinical interviews based on DSM-4 were applied to assess the recurrence status in patients who had achieved remission. Among the 84 remitters, 16 did not consent and refused to participate in the interview. Eventually, 68 patients completed the 2-year follow-up re-assessments. Six patients reported a remission period of less than 6 months following remission from the initial treatment. The other 22 patients reported a new depressive episode after 6 months of sustained remission. Forty patients remained stable for 2 years. Eventually, our second phase analyses included 28 recurring MDD and 40 stable MDD patients.
MRI data acquisition, preprocessing and functional network construction
Details of the MRI acquisition, preprocessing, and intrinsic functional network construction have been published previously (Ju et al., Reference Ju, Horien, Chen, Guo, Lu, Sun and Li2020) and can be found in the online Supplemental material. The MRI data were collected on the GE MRI system (Signa HDxT 3.0-tesla scanner) using a 16-channel head coil. The acquired DICOM files were then converted into a single NIFTI file for each participant's structural and functional image. The structural image was skull-stripped manually to improve the co-registration step. Then, the stripped structural image was co-registered to the functional image. Next, the structural image was nonlinearly registered to the standard Montreal Neurological Institute (MNI) template to obtain the transformation matrix. The functional image was slice time- and motion-corrected, and the covariates of no interest in the functional magnetic resonance imaging (fMRI) signals were regressed from the data. Finally, the functional image was temporally smoothed by a zero-mean unit-variance low-pass Gaussian filter (cut-off frequency of 0.12 Hz). After preprocessing, intrinsic functional connectivity was obtained using the 268 region-of-interest (ROI) functional atlas (Finn et al., Reference Finn, Shen, Scheinost, Rosenberg, Huang, Chun and Constable2015). First, using the inverse transformation matrix in the preprocessing nonlinear registration step, the 268 ROIs functional atlas were warped from the MNI space to the single subject space. Then the individual 268 ROIs atlas was applied to the fMRI data and the time course of each ROI was subsequently calculated from the fMRI data. Twelve ROIs were excluded owing to the lack of signals in at least one participant (online Supplemental Table S1). Finally, the Pearson correlation coefficient of all the ROI time series pairs (256 × 256) was calculated and converted into Z scores.
rsFNC calculation and General Linear Model Repeated Measure (GLM-RM) analysis
Resting-state networks were defined using eleven network masks established by Power et al. (Power et al. Reference Power, Cohen, Nelson, Wig, Barnes, Church and Petersen2011). The macro-scale rsFNC was calculated by averaging the rsFC of all the edges within a certain network. For example, the correlation between each pair of the fifty ROIs (Fig. 2a) in DMN yielded a 50 × 50 DMN correlation matrix. The correlation matrix contained 1225 unique rsFC edges. The DMN rsFNC was then calculated by averaging the rsFC of all the DMN edges in each individual. The rsFNC of the other ten networks was calculated in the same manner.

Fig. 2. Time by treatment outcome effect on DMN rsFNC revealed by GLM-RM analysis. a. Fifty ROIs in DMN. b. Effect of time by remission on DMN rsFNC. C. Effect of time by recurrence on DMN rsFNC. The line plots showed DMN rsFNC at baseline and follow-up. The scatter plots showed individual DMN rsFNC change pre- and post-treatment. Error bars indicate standard errors of the variables. * p < 0.05; ** p < 0.01. Abbreviations: DMN, default mode network; n.s, non-significant; rsFC, resting-state functional connectivity; ΔrsFC, change in pre- and post-treatment resting-state functional connectivity strength.
Next, the GLM-RM analysis was conducted to evaluate the effects of time and time by treatment outcome (non-remitters v. remitters or recurring v. stable MDD) on rsFNC in MDD. The rsFNC acquired by baseline and post-treatment scans were included as the repeated measures within-subject variable. The treatment outcome was included as the between-subject variable in the GLM-RM. In all the GLM-RM analyses, demographics (age, sex, education), motion parameters (FFD at both scans), and the onset status (first onset/recurrent) were included as covariates. Variables will be removed if they demonstrated no significant interactive effect with time. Least-significant-difference post-hoc tests were used to detect the differences in rsFNC between baseline and post-treatment within the subgroups.
Data-driven analysis
Leave-one-out cross-validation (LOOCV) was performed in a nCPM pipeline combined with a Support Vector Machine (SVM) algorithm to investigate whether the micro-scale rsFC changed in certain networks or the whole brain could classify remitters and non-remitters, or recurring and stable MDD. As the group size was not balanced between subgroups, the cost parameter was applied to penalize misclassification of the class with fewer samples (non-remitters and recurring MDD). The SVM cost parameters were optimized by grid search using a nested LOOCV procedure within the main LOOCV loop (Varoquaux et al., Reference Varoquaux, Raamana, Engemann, Hoyos-Idrobo, Schwartz and Thirion2017). The optimization ranges of cost parameters were set at (1, 105). The final cost parameter was generated by averaging the optimized cost parameters in all the nested LOOCV to reduce overfitting (Varoquaux et al., Reference Varoquaux, Raamana, Engemann, Hoyos-Idrobo, Schwartz and Thirion2017). Other parameters in SVM were set as default values. In each nCPM, the input was the change of each rsFC pre- and post-treatment within each network or the whole brain. For example, in DMN nCPM, the input was the change of each rsFC within DMN between the two scans, stored in a 50 × 50 matrix containing 1225 unique features for every participant. The change of rsFC was calculated using the following formula:

Twelve ΔrsFC matrices were used as inputs (eleven network ΔrsFC matrices and one whole-brain ΔrsFC matrices) for each treatment outcome variable (non-remitters/remitters, recurring MDD/stable MDD).
In each nCPM LOOCV, ΔrsFC matrices and treatment outcome data (non-remitters = 1, remitters = −1; recurring MDD = 1, stable MDD = −1) were divided into independent training and testing sets. The training set included N-1 subjects (N = total number of subjects), and the testing set included the left-out participants. First, feature selection was performed within the training set. Spearman's rank correlation was used to select the ΔrsFC highly related to the treatment outcome. During feature selection, different edge-selection thresholds (cut-off p value of Spearman's rank correlation analysis, p < 0.01, p < 0.05, p < 0.1) were applied in this study, considering the inevitably arbitrary nature of this threshold (Greene, Gao, Scheinost, & Constable, Reference Greene, Gao, Scheinost and Constable2018; Ju et al., Reference Ju, Horien, Chen, Guo, Lu, Sun and Li2020). Under each p threshold, treatment outcome-related functional connections lower than the current p value threshold were selected. The selected edges were separated into a positive edge set (ΔrsFC positively related to the treatment outcome) and a negative edge set (ΔrsFC negatively related to the treatment outcome). The model fitting step was performed thereafter. Using the training set data (N-1 subject data), the SVM algorithm is used to fit the multivariate classification model for the relationship between the selected edges and the treatment outcome. After fitting the SVM classification model, the same feature selection step was carried out in ΔrsFC matrix of the left-out participants to obtain the predicted treatment outcome label. The whole step was repeated N times, and the predicted treatment outcome label was obtained in all the N patients.
The model performance was evaluated by four indices, including accuracy, sensitivity (the percentage of non-remitters/recurring MDD correctly predicted), specificity (the percentage of remitters/stable MDD correctly predicted) derived by cross-validation, and p value estimated by permutation tests repeated 1000 times (p = K/1000, K is the number of iteration where the permutation accuracy > actual accuracy). Since we performed 12 × 3 (12 ΔrsFC matrices as input under 3 edge-selection p thresholds) nCPM analyses in our dataset to predict treatment outcome, Bonferroni corrections were applied to adjust for multiple testing. The prediction model was considered significant if the permutation p value was < 0.05/(12 × 3) = 0.0014. The contribution of the functional connectivity to the classifier was denoted by the frequency of its appearance across all the SVM classification LOOCV iterations (Zeng et al., Reference Zeng, Shen, Liu, Wang, Li, Fang and Hu2012).
The following online Supplementary analyses were employed to detect possible confounders and ensure the significance of the nCPM results. First, nCPM was repeated by applying ten-fold cross-validation, as previous studies have shown that over-fitting could occur in LOOCV (Scheinost et al., Reference Scheinost, Noble, Horien, Greene, Lake, Salehi and Constable2019). Owing to sample partition randomness during the ten-fold cross-validation, the above process was repeated 100 times and the average accuracy, sensitivity, and specificity were obtained to demonstrate model performance. Second, to exclude the effect of brain parcellation on the nCPM performance, 368 ROIs' functional atlas (Shen, Tokoglu, Papademetris, & Constable, Reference Shen, Tokoglu, Papademetris and Constable2013) were used to obtain the functional connectivity, and the nCPM LOOCV was performed again. Third, to eliminate the influence of head movement or medication on the model performance, the nCPM LOOCV was performed again with FFD or medication index as a covariate during the feature selection step. Lastly, to investigate whether the regression of global signal during MRI preprocessing would impact nCPM prediction, the functional connection matrices were generated without global signal regression, and the nCPM LOOCV was performed again.
rsFC visualization
Edges that appeared in all the LOOCV were extracted and visualized. Two visualization strategies in Bioimge Suite (https://www.nitrc.org/projects/bioimagesuite/) were applied to facilitate the characterization of the identified edges. Firstly, the ‘circle plot’ was adopted (Horien, Shen, Scheinost, & Constable, Reference Horien, Shen, Scheinost and Constable2019; Rosenberg, Hsu, Scheinost, Todd Constable, & Chun, Reference Rosenberg, Hsu, Scheinost, Todd Constable and Chun2017). Each edge was represented by a line connected between each pair of the 256 nodes. The 256 nodes were divided into 10 macro brain regions, including the prefrontal lobe, motor region, parietal lobe, temporal lobe, occipital lobe, limbic brain regions, insular lobe, cerebellum, subcortical brain regions and brainstem. Each macro brain region was represented by different colors in the circle plot. The ‘circle plot’ helped in the identification of the contribution of each macro brain region to the edge set. Secondly, a ‘glass brain plot’ was used to illustrate the connections, where each node is represented as a sphere, and the size of the sphere indicated the number of edges emanating from that node. The ‘glass brain plot’ helped identify the individual brain regions' contribution to the identified edge sets.
Other statistical analysis
All the statistical analyses were performed using SPSS Version 25.0 and MATLAB (Mathworks). Differences in the demographic, clinical or FFD data between the groups were examined using a t test. Sex differences were determined by the Pearson's chi-squared test. Differences in rsFNC between groups were assessed by analysis of covariance (ANCOVA), using age, sex, education, baseline FFD, and onset status (first onset/recurrent) as covariates. Pre-and post-treatment changes in rsFNC were measured by GLM-RM, with age, sex, education, FFD at both scans, and the onset status as covariates. The effect size in ANCOVA and GLM-RM analysis was provided as η 2 (η 2 of 0.01–0.059 indicates small effect, 0.059–0.138 indicates medium effect, and > 0.138 indicates large effect). And least-significant-difference test was used for post-hoc pairwise comparisons. Correlation between the DMN rsFNC change and the percentage improvement in the HAMD scores was measured by Spearman's rank correlation analysis, using age, sex, education, FFD at both scans, and onset status as covariates. Statistical significance was set at a 0.05 alpha level (two-tailed), and results were reported without multiple comparisons correction.
Data and code availability
The Matlab code to perform nCPM was based on a modified version of the publicly available CPM code (https://github.com/YaleMRRC/CPM). Custom scripts used in this study can be found on GitHub (https://github.com/jym115/CPM_MDD_long_term). The data supporting this study's findings are available from the corresponding author upon reasonable request.
Results
Sample characteristics
Details of the demographic and clinical characteristics in the different study groups are provided in Table 1. No significant difference was observed in the demographics between the patients with MDD and HC at baseline. No significant difference was observed in non-remitters and remitters except for the number of first-episode/recurrent patients. The recurring and stable MDD groups showed no significant difference in the basic demographic and clinical characteristics. In addition, no difference in the motion parameters were observed between the patients with MDD and HC or between the MDD subgroups.
Table 1. Demographic and clinical variables of participants

HAMD, Hamilton Depression Rating Scale; n, number of patients with MDD; M/F, male/female; MDD: major depressive disorder; M, median; P25, 25th percentile; P75, 75th percentile; s.d., standard deviation; SNRI, serotonin and norepinephrine reuptake inhibitor; SSRI, selective serotonin reuptake inhibitors.
Bold values indicate statistical significance.
P1, Baseline MDD v. Baseline HC; P2, Remitters v. Non-remitters; P3, Stable MDD v. Recurring MDD.
a p values obtained by independent-sample t test.
b p values obtained by Pearson's χ2 test.
c p values obtained by Kruskal-Wallis H test.
Effect of time by treatment outcome on rsFNC
The GLM-RM analysis revealed a significant interactive effect of time by remission on DMN rsFNC [F = 6.763, p = 0.011, η 2 (95% confidence interval (CI)) = 0.059 (0.003, 0.175); Figure 2b]. Post-hoc analysis revealed a significant decrease in the DMN rsFNC post-treatment in remitters (t = 3.345, p = 0.001) but not in non-remitters (t = 1.021, p = 0.309). However, the Spearman's rank correlation analysis did not detect a significant correlation between the DMN rsFNC decrease and the percentage improvement in the HAMD scores (R = 0.148, p = 0.127).
Similarly, the GLM-RM analysis identified a significant time effect by recurrence in the DMN rsFNC [F = 10.51, p = 0.002, η 2 (95% CI) = 0.146 (0.038–0.329); Figure 2c]. Post-hoc analysis revealed a significant DMN rsFNC decrease in the stable group (t = 3.960, p = 0.0002) but not in the recurring group (t = 1.102, p = 0.262). There was no significant effect of time by remission (online Supplementary Table S2) or recurrence (online Supplementary Table S3) on all the other rsFNC. Therefore, we excluded the treatment outcome variable in the GLM-RM analysis. The following analysis revealed a significant time effect on the cingulo-opercular [CON; F = 3.937, p = 0.049, η 2 (95% CI) = 0.014 (0.001–0.053)] and salience networks [SN; F = 4.952, p = 0.028, η 2 (95% CI) = 0.012 (0.001–0.046); online Supplementary Table S4].
rsFNC abnormalities related to the state of depression
To further explore whether rsFNC abnormalities were present in MDD, the rsFNC of the 11 networks were compared between 110 MDD and 136 HC at baseline. The results are detailed in online Supplementary Table S4. ANCOVA demonstrated a significantly decreased rsFNC in the ventral attention network (VAN) in MDD than in HC at baseline [F = 13.67, p = 0.0003, η 2 (95% CI) = 0.053 (0.014–0.116)]. The VAN rsFNC in MDD did not change significantly after the 6-month antidepressant treatment, remaining lower than that of HC [F = 8.584, p = 0.004, η 2 (95% CI) = 0.030 (0.003–0.084)]. Subgroup analysis demonstrated that the VAN rsFNC in both groups was significantly lower than that in HC. At 6 months, the VAN rsFNC did not change significantly in both remitters and non-remitters. Moreover, we found that the rsFNC in MDD was significantly lower than that in HC in CON [F = 5.151, p = 0.024, η 2 (95% CI) = 0.020 (0.001–0.071)] and DMN [F = 6.535, p = 0.011, η 2 (95% CI) = 0.026 (0.002–0.074)] after 6 months.
Predicting treatment outcome using nCPM
Classifying remitters and non-remitters selective to DMN ΔrsFC
We ran nCPM using ΔrsFC of each network or whole-brain as input data. The receiver operating characteristics (ROC) for classifying remitters and non-remitters are presented in Fig. 3a. The model performance of each nCPM under different edge selection thresholds is detailed in online Supplementary Table S5. Using 1225 ΔrsFC in the DMN, nCPM successfully distinguished remitters and non-remitters under edge selection threshold p = 0.01. Specifically, under edge selection threshold p = 0.01, the DMN nCPM achieved a 80.0% overall accuracy (88 of 110 in the whole group, permutation p < 0.001), 46.2% sensitivity (12 of 26 non-remitters) and 90.5% specificity (76 of 84 remitters). The permutation distribution of the accuracy is shown in online Supplemental Fig. S1, indicating that the prediction accuracy was not by chance. When other control analyses were performed, results of DMN nCPM prediction performance were similar, with slightly decreased performance observed. However, when rsFC was calculated without GSR, the DMN nCPM model performance was not significant (online Supplementary Table S6). Notably, nCPM prediction did not reach significance in other networks or whole-brain connectome (permutation p > 0.05).

Fig. 3. The ROC for predicting sustained non-remission (a) and recurrence (b) in MDD. Each point on the graph represents the nCPM model performance using ΔrsFC within a network or whole-brain connectome as input. The true positive rate (sensitivity) is plotted on the y-axis, denoting the proportion of non-remitters/recurring MDD who are correctly identified. The false positive rate (1 – specificity) is plotted on the x-axis, depicting the proportion of remitters/stable MDD who are wrongly categorized as non-remitters/recurring MDD. The diagonal dotted line indicates the performance of a purely random prediction. Points above the diagonal line indicate better than random classification results. The prediction results get better nearing the upper left corner. Prediction of nCPM achieved the best performance in DMN. Significant predictions were marked in bold. Abbreviations: ΔrsFC, change in pre- and post-treatment resting-state functional connectivity strength; nCPM, network connectome-based predictive modeling; SMN, somatosensory network; CON, cingular opercular network; AUN, auditory network; DMN, default mode network; VN, visual network; FPN, frontoparietal network; SN, salience network; SCN, subcortical network; VAN, ventral attention network; DAN, dorsal attention network; CBN, cerebellum network.
Sustained non-remission network
Next, we set out to visualize the DMN edges significantly characterizing sustained non-remission after the 6-month antidepressant treatment. Edges that were selected as features in each iteration of the LOOCV procedure were considered consensus edges consistently discriminating remission and non-remission at 6 months. Consensus edges are shown in Fig. 4a, and the detailed information on each edge is listed in online Supplementary Table S7. All the nine consensus edges belong to the negative edge set (of which lower ΔrsFC was characterized non-remission at 6 months). No consensus edge belonged to the positive edge set (of which higher ΔrsFC was characterized non-remission at 6 months). We named this consensus edge set as ‘sustained non-remission network’. In addition, edges that appeared in any LOOCV were examined. Details are listed in online Supplementary Table S7.
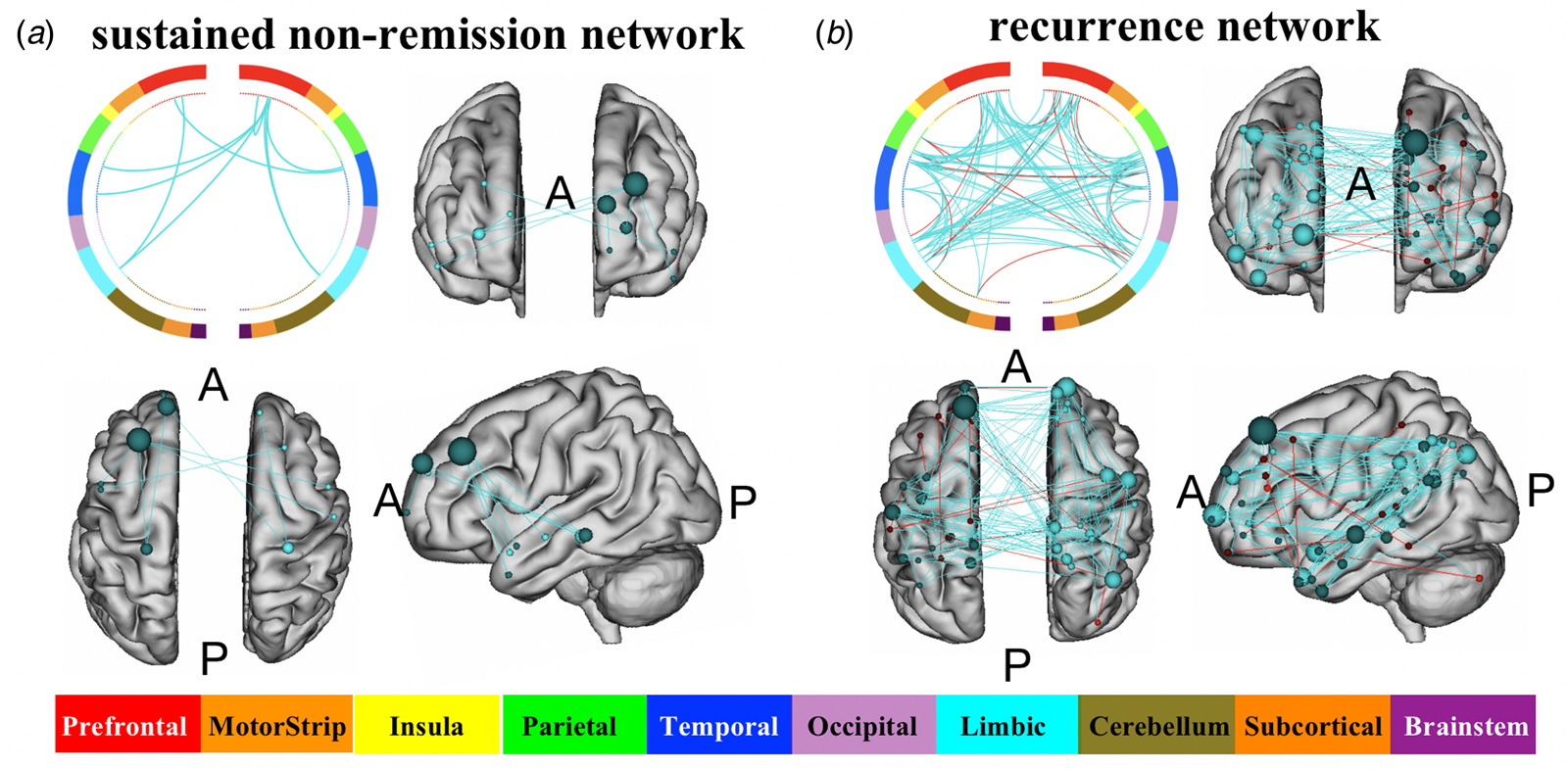
Fig. 4. Network anatomy of consensus edges included in the sustained non-remission network (a) and recurrence network (b). The upper left showed the ‘circle plot’ (as in method 2.6) of consensus edges. The top of the circle represents the anterior part of the brain; the bottom of the circle represents the posterior part of the brain. The left side of the circle corresponds to the left hemisphere of the brain. Legends indicating the anatomic lobe is shown below. The other three images showed the glass brain plot (as in method 2.6), where each node is represented as a sphere. Abbreviations: A, anterior; P, posterior.
Classifying recurring and stable MDD selective to DMN ΔrsFC
The ROC for classifying recurrence and stable MDD are presented in Fig. 3b, and the nCPM model performance is detailed in online Supplementary Table S8. When ΔrsFC of the DMN was taken as input, nCPM successfully predicted whether the patient remained stable or had a recurrence during the 2-year follow-up. The model performance achieved significance under the edge selection threshold p = 0.05 and 0.1. Specifically, under edge selection threshold p = 0.05, the DMN nCPM predictive model reached a 76.5% overall accuracy (52 of 68 in the whole group, permutation p < 0.001), 60.7% sensitivity (17 of 28 recurring MDD), and 87.5% specificity (35 of 40 stable MDD); under edge selection threshold p = 0.1, model performance achieved a 75.0% accuracy (51 of 68 in the whole group, permutation p < 0.001), 67.9% sensitivity (19 of 28 recurring MDD), and 80.0% specificity (32 of 40 stable MDD). The permutation distribution of the accuracy is shown in online Supplemental Fig. S2. Results of DMN nCPM prediction showed similar performance when we conducted other control analyses, with slightly decreased or increased model performance observed compared to our main results. However, the DMN nCPM model performance was not significant without GSR (online Supplementary Table S9). When applying the nCPM pipeline on ΔrsFC of other networks or whole-brain connectome, nCPM failed to predict MDD recurrence. None of the permutation tests reached statistical significance (permutation p > 0.05).
Recurrence network
The DMN edges that significantly predicted MDD recurring states were investigated and visualized. Consensus edges are shown in Fig. 4b, and detailed information on each edge is listed in online Supplementary Table S10. One hundred and sixteen consensus edges belonged to the negative edge set (of which ΔrsFC negatively predicts MDD recurrence), and 16 consensus edges belonged to the positive edge set (of which ΔrsFC positively predicts MDD recurrence). We named the consensus edge set as ‘recurrence network.’ Edges that appeared in any LOOCV under the edge selection threshold p = 0.1 were also extracted. Detailed edge information is provided in online Supplementary Table S10.
Discussion
In the present study, we examined the intrinsic brain network change during the 6-month antidepressant treatment for refractory depression and recurrence. By exploring macro-scale network connectivity and micro-scale functional connectivity in the resting state, we found that DMN network modulation is related to sustained non-remission and recurrence in MDD. Moreover, by developing nCPM, a data-driven analysis, we observed that the pre-and post-treatment rsFC change in DMN (but not other networks) specifically discriminated patients with different long-term treatment outcomes. These findings indicate that the DMN may be a unique and important target in the long-term treatment effect of antidepressants.
The current study demonstrated that the treatment outcome by time showed a significant interaction effect in the DMN rsFNC but not in the other brain networks. Specifically, the rsFNC of DMN decreased significantly in remitters but not in non-remitters after the 6-month treatment. These results suggest that antidepressants may exert a therapeutic effect by decreasing the functional connection in DMN. Previous studies have found that the intrinsic functional connectivity in DMN decreased following antidepressant treatment. However, inconsistent findings on the relationship between the DMN connectivity alteration and antidepressant treatment response were observed. A study incorporating a placebo-controlled design found that antidepressants decreased the DMN rsFC of patients with dysthymia (Posner et al., Reference Posner, Hellerstein, Gat, Mechling, Klahr, Wang and Peterson2013). However, the authors did not find an association between DMN rsFC normalization and reduction in depressive symptoms (Posner et al., Reference Posner, Hellerstein, Gat, Mechling, Klahr, Wang and Peterson2013). Our study also did not detect a correlation between change in DMN rsFC and depressive symptom improvement. However, a recent study found that depressive symptom improvement in 2 months correlated with the connectivity change between the brain regions in the VN to regions in the DMN, somatosensory network and frontal-parietal network (Li et al., Reference Li, Su, Wu, Castellanos, Li, Li and Yan2021). Besides, another randomized placebo-controlled study found that reduction of rsFNC in DMN during one-week antidepressant treatment could predict the treatment response in 8 weeks (Nemati et al., Reference Nemati, Akiki, Roscoe, Ju, Averill, Fouda and Abdallah2020). Inconsistent findings on the relationship between the DMN connectivity change and treatment response could be owing to the use of varying treatment outcomes (binomial or continuous), variable assessment time points (weeks to months), and differences in the fMRI analytic approaches (ROI or graph theory). Our study demonstrated for the first time that the reduction in DMN rsFNC was specifically related to the remission after the 6-month antidepressant treatment. The rsFNC decrease in DMN might be associated with the monoamine transporter modulation by antidepressants (Hahn et al., Reference Hahn, Wadsak, Windischberger, Baldinger, Hoflich, Losak and Lanzenberger2012; Kunisato et al., Reference Kunisato, Okamoto, Okada, Aoyama, Demoto, Munakata and Yamawaki2011). These serotonin and norepinephrine transporters are widely distributed in the raphe nuclei, which densely innervate the brain regions in the DMN (Smith, Beveridge, & Porrino, Reference Smith, Beveridge and Porrino2006; van de Ven et al., Reference van de Ven, Wingen, Kuypers, Ramaekers and Formisano2013). Therefore, antidepressants may indirectly modulate DMN connectivity strength by regulating neurotransmitters in these brain regions (Hahn et al., Reference Hahn, Wadsak, Windischberger, Baldinger, Hoflich, Losak and Lanzenberger2012; Schrantee et al., Reference Schrantee, Lucassen, Booij and Reneman2018; Turner et al., Reference Turner, Fischer, Sivakolundu, Hubbard, Zhao, Rypma and Backman2020; Varnäs, Halldin, & Hall, Reference Varnäs, Halldin and Hall2004). In addition, it has been widely acknowledged that DMN is involved in rumination and self-reference (Whitfield-Gabrieli & Ford, Reference Whitfield-Gabrieli and Ford2012), which are prominent cognitive vulnerabilities in patients with MDD (Hamilton, Farmer, Fogelman, & Gotlib, Reference Hamilton, Farmer, Fogelman and Gotlib2015). Therefore, the DMN rsFNC decrease could be an antidepressant neurobiological mechanism for modulating internal psychological processes in MDD. However, the intrinsic brain network changes in the current study could be merely underlying the symptom improvement in patients (i.e. from the acute state to the remission state), rather than a change caused by antidepressants. Future placebo-controlled studies could further elucidate the intrinsic network mechanism underlying antidepressant treatment.
We found that reduction in DMN rsFNC during the treatment phase could predict 2-year sustained remission in MDD. This is consistent with previous studies that prospectively investigated neural biomarkers of depression recurrence. One task fMRI study found that neural reactivity in the medial prefrontal cortex (anterior hub of DMN) following mood challenge was associated with relapse after 18 months in remitted MDD (Farb, Anderson, Bloch, & Segal, Reference Farb, Anderson, Bloch and Segal2011). Another cohort study used structural MRI and found that the small anterior cingulate cortex (ACC, core brain region of DMN) volume was associated with a chronic course of MDD (Serra-Blasco et al., Reference Serra-Blasco, de Diego-Adelino, Vives-Gilabert, Trujols, Puigdemont, Carceller-Sindreu and Portella2016). The Munster Neuroimage Cohort included 75 remitted MDD patients and found several structural and functional brain features that could predict depression recurrence. Two fMRI studies found that the rsFC between sgACC and other DMN brain regions during resting-state and self-blame tasks could predict MDD recurrence within 14 months (Lythe et al., Reference Lythe, Moll, Gethin, Workman, Green, Lambon Ralph and Zahn2015; Workman et al., Reference Workman, Lythe, McKie, Moll, Gethin, Deakin and Zahn2017). One structural MRI study revealed that MDD relapse was associated with the brain structures that underlie emotion regulation (Zaremba et al., Reference Zaremba, Dohm, Redlich, Grotegerd, Strojny, Meinert and Dannlowski2018). All the identified brain structural and functional characteristics were located in the DMN. These previous studies provided us with some prospective predictors of MDD recurrence and relapse. However, whether and how these neural biomarkers could be used as targets for preventing MDD recurrence is uncertain. Our research filled this void by investigating the recurrence-related change in the intrinsic brain networks pre- and post-treatment. The DMN rsFNC reduction observed in our study could contribute to the long-term disease remission. The mechanism by which DMN rsFNC reduction results in a reduced risk of future recurrence in MDD could be explained by reduced rumination, since studies have confirmed that rumination can predict relapse and a poorer clinical course of MDD (Figueroa et al., Reference Figueroa, Ruhe, Koeter, Spinhoven, Van der Does, Bockting and Schene2015; Michalak, Holz, & Teismann, Reference Michalak, Holz and Teismann2011; Nolen-Hoeksema, Wisco, & Lyubomirsky, Reference Nolen-Hoeksema, Wisco and Lyubomirsky2008). However, our current study did not collect data on rumination behavior in patients. Future studies should examine the rumination level and the DMN network pre-and post-treatment in MDD to further testify this hypothesis.
It should be noted that there was no significant difference in the DMN rsFNC between patients with MDD and HC at baseline. However, the DMN rsFNC was decreased in remitters post-treatment, and was lower than in the HC group. The baseline result is consistent with a recent finding that the DMN rsFNC showed no difference in medication-naive patients with MDD and HC (Yan et al., Reference Yan, Chen, Li, Castellanos, Bai, Bo and Zang2019). Although we did not identify a significant alteration in the overall DMN network strength in MDD, there could be an abnormal increase and decrease in individual DMN functional connectivity in depressive patients (Li et al., Reference Li, Su, Wu, Castellanos, Li, Li and Yan2021). After 6 months, the antidepressant could have reduced the connectivity strength of most of the edges within the DMN, including several key functional connectivities underpinning depression. Therefore, the remitters showed an overall reduction in the DMN strength. Besides, we found rsFNC decreased significantly in CON and SA after antidepressant treatment. However, the rsFNC change was not related to the change in depressive symptoms; thus, it could be a non-specific modulation attributed to antidepressant treatment. This result is consistent with a recent finding that extensive edges showed functional connectivity reduction post-antidepressant treatment; however, only a few connectivity changes were significantly related to depressive symptom improvement (Li et al., Reference Li, Su, Wu, Castellanos, Li, Li and Yan2021). Finally, the VAN rsFNC was lower than that of HC at baseline and follow-up, indicating that this may be a trait biomarker in patients with MDD.
Using nCPM, we also identified the network in which functional connectivity changes were associated with the long-term treatment outcome in MDD. The results demonstrated that only the pre-and post-treatment rsFC changes in DMN were specifically related to remission and recurrence. This finding further illustrates the importance of DMN in the network mechanism by which antidepressants may reduce depression. nCPM also helped delineate the functional connectivity change in which the edge characterized remission and recurrence in MDD. Particularly, brain regions, including the anterior prefrontal cortex (APC), dorsal lateral prefrontal cortex, dorsal ACC and hippocampus (HP), and temporal pole (TP), formed consensus edges set that classified remitters/sustained non-remitters. Whereas frontal eye field, APC, angular gyrus, and TP have the highest node degree in the consensus edges that classified stable MDD/recurring MDD. These brain regions have been consistently implicated in previous longitudinal studies examining the effects of antidepressant treatment on functional brain connectome, with most studies reporting a decrease in the functional connectivity (Klaassens et al., Reference Klaassens, van Gorsel, Khalili-Mahani, van der Grond, Wyman, Whitcher and van Gerven2015; Li et al., Reference Li, Liu, Friston, Shen, Wang, Zeng and Hu2013; McCabe & Mishor, Reference McCabe and Mishor2011; Posner et al., Reference Posner, Hellerstein, Gat, Mechling, Klahr, Wang and Peterson2013; Wu et al., Reference Wu, Andreescu, Butters, Tamburo, Reynolds and Aizenstein2011), degree centrality (Gong et al., Reference Gong, Hou, Wang, He, Yin, Yuan and Zhang2018), or nodal efficiency (Gong et al., Reference Gong, Hou, Wang, He, Yin, Yuan and Zhang2018; Wang et al., Reference Wang, Xia, Li, Zeng, Su, Dai and Si2015) among these brain regions post-treatment. Nevertheless, it is worth mentioning that the DMN nCPM model was not significant when rsFC was calculated without GSR, which is consistent with previous studies using intrinsic brain networks to predict behaviors (Greene et al., Reference Greene, Gao, Scheinost and Constable2018; Ju et al., Reference Ju, Horien, Chen, Guo, Lu, Sun and Li2020; Lake et al., Reference Lake, Finn, Noble, Vanderwal, Shen, Rosenberg and Constable2019). Global signal regression was suggested to be able to reduce imaging artifacts and therefore might help to strengthen the association between rsFC and behavior (Li et al., Reference Li, Kong, Liegeois, Orban, Tan, Sun and Yeo2019; Murphy & Fox, Reference Murphy and Fox2017). Lastly, it is worth noting that the DMN FC change during antidepressant treatment could be a common factor in other treatment forms, since previous studies have demonstrated that the TMS and transcutaneous vagus nerve stimulation significantly modulated the DMN FC during treatment (Fang et al., Reference Fang, Rong, Hong, Fan, Liu, Wang and Kong2016; Philip et al., Reference Philip, Barredo, van ’t Wout-Frank, Tyrka, Price and Carpenter2018). Therefore, DMN modulation could be considered important in MDD prognosis regardless of the type of treatment.
Previous studies have tried identifying predictors and neural correlates of antidepressant treatment response. Yet most of these studies have employed comparison and correlation analyses. Recently, machine learning models have been increasingly used in identifying brain-based biomarkers in MDD (Chekroud et al., Reference Chekroud, Bondar, Delgadillo, Doherty, Wasil, Fokkema and Choi2021). However, relatively few studies include appropriate validation procedures (Chekroud et al., Reference Chekroud, Bondar, Delgadillo, Doherty, Wasil, Fokkema and Choi2021). Many data-driven models were built based on the feature selected from the same dataset, which may result in an inaccurate evaluation of the predictive model performance (Kriegeskorte, Simmons, Bellgowan, & Baker, Reference Kriegeskorte, Simmons, Bellgowan and Baker2009; Scheinost et al., Reference Scheinost, Noble, Horien, Greene, Lake, Salehi and Constable2019). Our current data-driven machine learning analysis provides a validation framework for identifying neuroimaging biomarkers for long-term treatment outcomes. We could produce relatively reliable brain features that could be potential therapeutic target in the long-term action of antidepressants by using the machine-learning pipeline. Furthermore, we could easily visualize and interpret the features with predictive value. However, generalizing our findings to unseen data is critical for improving the reliability and clinical feasibility of the neuroimaging biomarkers in the future.
There were certain limitations in our current study. First, although we tried to maintain and followed up the patients at specified intervals, 49/171 (40.2%) patients were lost to follow-up during the 6-month antidepressant treatment. Considering the high remission rate (88/122 = 72.1%) in our samples, those who did not achieve remission may have dropped out during our follow-up investigations, which probably resulted in the attrition bias in our study. Second, we could not rule out the placebo effect in our current study. Therefore, future studies should account for the neurobiological placebo effect when studying the mechanism of antidepressant treatment. Moreover, important treatment-related characteristics, such as dosage, duration, and adverse effects of antidepressant therapy, must be systematically investigated in future research to further eliminate confounding factors. Third, it should be acknowledged that our prediction models had relatively low sensitivity in identifying non-remitters, which might be related to imbalanced class distribution. However, our main objective was to test the hypothesis that functional connectivity change in certain networks could better characterize long-term treatment outcomes and identify the potential therapeutic targets for remission and recurrence prevention in MDD. Future research on predictive modeling could improve model fitting algorithms or use sampling methods to better deal with the imbalanced groups and increase the prediction robustness. Besides, future research could also collect data on other potential predictors, such as sociodemographic (Chekroud et al., Reference Chekroud, Zotti, Shehzad, Gueorguieva, Johnson, Trivedi and Corlett2016), psychosocial, and clinical characteristics (Chekroud et al., Reference Chekroud, Zotti, Shehzad, Gueorguieva, Johnson, Trivedi and Corlett2016; Etkin et al., Reference Etkin, Patenaude, Song, Usherwood, Rekshan, Schatzberg and Williams2015), HPA-related index (Kennis et al., Reference Kennis, Gerritsen, van Dalen, Williams, Cuijpers and Bockting2020), neuroticism personality (Trivedi et al., Reference Trivedi, South, Jha, Rush, Cao, Kurian and Fava2018), and childhood trauma (Trivedi et al., Reference Trivedi, South, Jha, Rush, Cao, Kurian and Fava2018) to maximize predictive performance. The multi-dimensional data may offer more meaningful and generalizable predictions (Chekroud et al., Reference Chekroud, Bondar, Delgadillo, Doherty, Wasil, Fokkema and Choi2021). Lastly, we were unable to acquire an external replication sample to test the generalizability of our findings. Other independent datasets would be needed to further confirm the identified neuroimaging biomarkers.
In conclusion, the present study examined the intrinsic brain network changes during the antidepressant treatment phase associated with long-term treatment outcomes in MDD. We found that the functional connectivity change within DMN is specifically related to sustained non-remission at 6 months and disease recurrence within 2 years. The present findings indicate the brain mechanism by which antidepressants effectively treat and prevent long-term MDD. However, the results need to be replicated and validated using other independent datasets (especially in placebo-controlled design) to generate more accurate and generalizable neuroimaging biomarkers for clinical practice.
Supplementary material
The supplementary material for this article can be found at https://doi.org/10.1017/S0033291722002628
Acknowledgements
We would like to thank all the patients and healthy volunteers who participated in our study.
Financial support
This work was supported by the National Natural Science Foundation of China (82101612 to Yumeng Ju), the science and technology innovation Program of Hunan Province (2021RC2040 to Yumeng Ju), the Natural Science Foundation of Hunan Province, China (2022JJ40692 to Yumeng Ju), the National Key Research and Development Program of China (grant number 2019YFA0706200 to Yan Zhang and Lingjiang Li, 2021ZD0202000 to Yan Zhang). Yumeng Ju's work is supported by the Central South Postdoctoral Programme. The authors would gratefully acknowledge financial support from the above affiliations. The sponsors had no role in the study design, data collection and analysis, interpretation of the results and writing of the report.
Conflict of interest
The authors report no conflicts of interest with respect to the content of this manuscript.
Ethical standards
The authors assert that all procedures contributing to this work comply with the ethical standards of the relevant national and institutional committees on human experimentation and with the Helsinki Declaration of 1975, as revised in 2008.
Data availability statement
For more information on the data supporting the analyses and results, please contact the corresponding author Lingjiang Li.