Introduction
The wheat grain is milled before processing into edible products. Common or bread wheat is used for making several end-use products such as biscuits, breads, cakes and pasta. The processing of wheat flour into a particular product is largely determined by the grain components, especially starch and endosperm storage protein. The major endosperm storage protein in wheat is called Gluten. Gluten is largely responsible for the viscoelastic property of wheat dough (Shewry et al., Reference Shewry, Halford, Belton and Tatham2002; Anjum et al., Reference Anjum, Khan, Din, Saeed, Pasha and Arshad2007).
Gluten consists of a very large number of polymeric (glutenins) and monomeric (gliadins) polypeptide fractions. The glutenin protein subunits comprise two subgroups, the predominant high molecular weight glutenin subunits (HMW-GS) and low molecular weight glutenin subunits (LMW-GS). Though both the fractions influence dough strength and extensibility, HMW-GS chiefly determines dough strength and LMW-GS is crucial for dough extensibility. Generally, bread wheat consists of 3–5 HMW-GS coded by Glu-1 loci on the long arm of chromosome 1-1AL, 1BL and 1DL, (Payne and Lawrence, Reference Payne and Lawrence1983). The coding sequence of all HMW-GS (Ax1, Ax2*, Null Ay, Bx7, By9, Bx14, Bx17, Dx2, Dx5, Dy10 and Dy12) are cloned and characterized (Payne et al., Reference Payne, Law and Mudd1980; Halford et al., Reference Halford, Forde, Anderson, Greene and Shewry1987). LMW-GS exhibits a multigene family with 30–40 genes coded by Glu-3 loci on the short arm of chromosome-1AS, 1BS and 1DS. Both HMW and LMW exhibit a high degree of polymorphism and play a key role in governing bread wheat end-use quality. LMW-GS are more diverse than the HMW-GS however, their associations with grain quality are less well-understood (Shewry and Lafiandra, Reference Shewry and Lafiandra2022).
The allelic combinations of HMW-GS and LMW-GS determine whether the gluten forms a strong or weak network and whether the dough is elastic or extensible. Rheological studies have established that strong and extensible flour dough is desirable for bread, pizza and other leavened products whereas weak and extensible dough is suitable for cake, cookies and other short-textured products. Medium-strong and extensible gluten are desirable for good chapatti-making quality (Rai et al., Reference Rai, Singh, Ganjewala, Kumar, Ahlawat, Singh, Sharma and Jain2019). Exploring and identifying major allelic effects on gluten strength and extensibility have always been the subject of research. Thus, selecting the optimum alleles of these genes is an important consideration for grain quality improvement.
Changing climate and human intervention in crossbreeding programmes have caused an imbalance, resulting in a drastic loss of glutenin gene variability during the shift from wild landraces to modern cultivars. Increasing genetic erosion and loss of diversity is a matter of concern and thus creates the need for introgressing genetic variation in crop species (Moragues et al., Reference Moragues, Zarco-Hernandez, Moralejo and Royo2006). In this direction, CIMMYT worked on expanding the utilization of genetically diverse and widely adapted wheat germplasm available in the gene banks and persistently enriched the gene pool with greater genetic diversity (Kumar et al., Reference Kumar, Choudhary, Kaur and Mehta2022). Several studies have demonstrated wide genetic variability in the HMW-GS (Glu-1 alleles) and LMW-GS (Glu-3 alleles) in bread wheat in different geographical locations (Franaszek and Salmanowicz, Reference Franaszek and Salmanowicz2021). Thus, the characterization of the genetic variation of HMW-GS and LMW-GS among the germplasm is necessary for germplasm conservation and exploitation of genetic resources for crop improvement.
Considering India's geographical locations, climatic conditions, soil types, growing duration of wheat and the number of irrigations given in a particular area, the Indian wheat crop cultivation is divided into five zones namely the Northern eastern plains zone (NEPZ), Northern western plains zone (NWPZ), Northern hill zone (NHZ), Peninsular zone (PZ) and Central zone (CZ). The detailed pictorial representation of different zones with their clear boundaries is depicted in online Supplementary Fig. S1. During and after the period of the green revolution, the wheat breeding schemes were truly focused on developing varieties with high grain yields.
In this study, 107 wheat varieties released across different agricultural zones and different time periods in India were used to investigate HMW-GS and LMW-GS allele polymorphism, gene diversity and genetic variation in the Glu-1 and Glu-3 loci. This research aimed to investigate the diversity of HMW-GS and LMW-GS over the time periods, with the primary objective of accurately predicting the suitability of genotypes for different zones in terms of end-use products. Additionally, the outcomes of this analysis hold substantial significance in facilitating improvements in grain quality.
Materials and methods
Plant material
The experimental material comprised a panel of one hundred seven bread wheat varieties, which were grown in randomized complete block design during the crop season 2019–20 and 2020–21. The wheat genotypes used in this study were selected based on a greater area of cultivation, high yield and popularity among the people. These 107 wheat varieties have been released during recent decades for different agro-climatic zones in India from the period of 1975 to 2017. The set of nine Australian varieties was used as the standard for the identification of gluten subunits. These were imported in 2010 in the collaborative Indo-Australian programme on marker-assisted wheat breeding and were used as reference varieties for Glu-1 and Glu-3 genes. The parentage of the varieties is given in online Supplementary Table S1 (Gupta et al., Reference Gupta, Singh, Kumar, Tyagi, Tiwari, Chatrath and Singh2018).
Protein extraction and identification of HMW-GS
Protein from five seeds of each variety was extracted and Sodium Dodecyl Sulphate-Polyacrylamide Gel Electrophoresis (SDS-PAGE) analysis was carried out as per the protocol described by (Singh et al., Reference Singh, Shepherd and Cornish1991). The standards were Annuello (1, 7 + 8, 2 + 12 at Glu1; b, b at Glu-3), Barham (1, 7 + 8, 2 + 12 at Glu-1; c, b at Glu-3), Baxter (1, 13 + 16, 2 + 12 at Glu-1; b, h at Glu-3), Binnu (null, 17 + 18, 2 + 12 at Glu-1; c, b at Glu-3), Datatine (2*, 7 + 8, 2 + 12 at Glu-1; f, b at Glu-3), Drysdale (1, 17 + 18, 5 + 10 at Glu-1; b, b at Glu-3), EJA_Jitarning (2*, 17 + 18, 2 + 12 at Glu-1; f, b at Glu-3), Gladius (1, 7 + 8, 5 + 10 at Glu-1; c, b at Glu-3), Janz (1, 7 + 8, 2 + 12 at Glu-1; b, b at Glu-3), Yitpi (1, 7 + 8, 2 + 12 Glu1; c, d at Glu-3). Glutenins were separated in polyacrylamide gels (10%) prepared using 1 M Tris buffer, pH of 8.5. Gels were run at 60 V for about 10 h. Gels were stained with Coomassie Blue R and then destained with water. The HMW-GS subunits were named following the nomenclature of Lawrence and Payne (Reference Lawrence and Payne1983). HMW-GS was initially determined by SDS-PAGE, but the alleles at the Glu-1 and Glu-3 locus were difficult to differentiate. Subsequently, the alleles were confirmed using the polymerase chain reaction (PCR) using the allele-specific markers for the Glu-1 and Glu-3 locus.
PCR reaction for identification of HMW and LMW-GS
10–12 seeds of each of the genotypes were germinated in the dark in a medium containing sterilized peat and 12–15 day old seedlings were used for the genomic DNA extraction by cetyltrimethylammonium bromide procedure. Molecular typing of HMW-GS at Glu-1 and LMW-GS genes at Glu-3 loci was carried out using allele-specific PCR (AS-PCR) primers (online Supplementary Table S2) (Francis et al., Reference Francis, Leitch and Koebner1995; Ma et al., Reference Ma, Zhang and Gale2003; Zhang et al., Reference Zhang, Gianibelli, Rampling and Gale2004; Liu et al., Reference Liu, Chao and Anderson2008; Wang et al., Reference Wang, Zhao, He, Ma, Appels, Peña and Xia2009, Reference Wang, Li, Peña, Xia and He2010). PCR was performed using 0.3 μl Taq DNA polymerase in 15 μl reaction volumes containing approximately 50 ng of genomic DNA, 1 × PCR buffer with 1.5 MgCl2, 10 pmol of each PCR primer, and 100 μM of each of dNTPs. PCR cycling conditions were 94°C for 5 min following 35 cycles of 94°C for 35 s, 57–60°C for 35 s, 72°C for 90 s, and a final extension at 72°C for 8 min. The PCR products were separated by electrophoresis in 1.5% agarose and visualized by ethidium bromide staining.
Population structure and genetic diversity analysis
Population structure was assessed using a Bayesian Markov Chain Monte Carlo model (MCMC) employed in STRUCTURE v2.3.4 (Pritchard et al., Reference Pritchard, Stephens and Donnelly2000). Three runs per genotype set were performed with the range set from 1 to 10. Burn-in time and MCMC replication number were both set to 100,000 for each run. The most probable K-value was estimated by submitting the STRUCTURE results (Results.zip) in the online available 5 program STRUCTURE harvester (http://taylor0.biology.ucla.edu/struct_harvest/). The optimum K value was calculated by plotting the mean estimate of the log posterior probability of the data L (K) against the given K value. The value of K was constructed on the run with the maximum likelihood. The genetic diversity of the genotypes relied on the amount of allelic variation among the HMW-GS and LMWGS in the set of samples under study. Percentage allele frequency was calculated by using the formula (number of individual alleles divided by the total number of wheat genotypes) multiplied by 100. The relative frequency of a allele occurring at a particular locus was calculated by using the formula (number of times allele occurred in that category or subgroup divided by the total frequency of that group) × 100. POWERMARKER Ver. 3.25 (Liu and Muse, Reference Liu and Muse2005) was used to calculate the genetic diversity, major allele frequency, considering the genetic parameters (Nei's) gene diversity and the polymorphism information content (PIC). A phylogenetic neighbour joining (NJ) tree based on genotypes and zones was made by using POWERMARKER Ver. 3.25 with 1000 bootstrap replicates. The relationship between varieties based on the variation of HMW and LMW glutenin subunits was analysed in the form of phylogenetic trees using the POWERMARKER and MEGA5 software (Tamura et al., Reference Tamura, Peterson, Peterson, Stecher, Nei and Kumar2011). The dendrogram was constructed by computing the frequency-based distance. The r2 (linear regression coefficient) and Pearson's correlation of traits were computed using SPSS software (Version 25.0, IBM SPSS Statistics; SPSS Inc, Chicago, USA) at the P ⩽ 0.05, and P ⩽ 0.01 level of significance. Principal component analysis (PCA) was performed using the XLSTAT statistical software. The analysis was based on a correlation matrix data of all glutenin alleles to reduce the dimensions of data space (Vidal et al., Reference Vidal, Manful, Pham, Stewart, Keough and Thomas2020). The Biplot method permits the plotting of the genotypes, glutenin subunits and various zones with an informative representation of the interrelation among the plotted data.
Results
Allelic variation in wheat varieties
In the current study, we have used both SDS-PAGE and PCR-based allele-specific markers to identify HMW and LMW-GS in a set of Indian genotypes (online Supplementary Table S1 and Figs. S2–S4). The allele patterns of HMW-GS and LMW-GS for the wheat varieties remained consistent throughout the years. The frequencies of different alleles identified were calculated and shown in Table 1 and Fig. 1. The wide variability of HMW-GS was observed in the analysed set of genotypes. Glu-A1b(2*), Glu-B1u and Glu-D1a were present at the highest frequency among the different genotypes at the Glu-1 locus. At the Glu-3 locus, Glu-A3c and Glu-B3j were present predominantly in the major proportion among the different genotypes. The Burt table analysis for the different glutenin subunits is shown in online Supplementary Table S3. The Burt table analysis depicted the various combinations of alleles found among the set of genotypes under this study. The most frequent combination of alleles occurring in most of the genotypes was found to be Glu-A1b in combination with, Glu-B1u (22 times/107 genotypes), Glu-D1a (47 times/107 genotypes), GluA3c (49 times/107 genotypes) and Glu-B3i (19 times/107 genotypes).
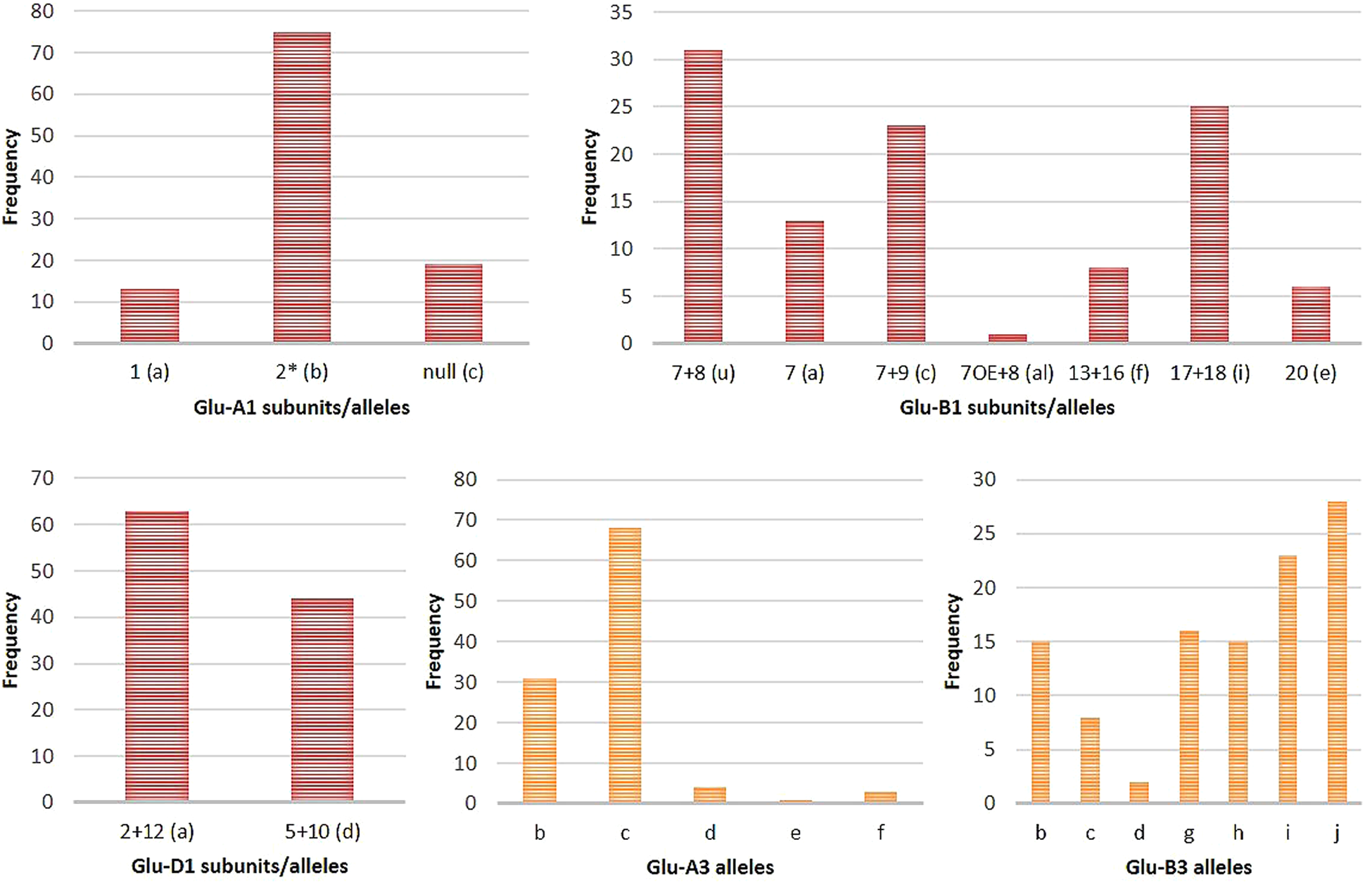
Figure 1. Bar graph representation of frequency distribution of HMW and LMW alleles/subunits present in 107 wheat genotypes.
Table 1. Genetic diversity analysis for Glu-1 and Glu-3 locus and their respective alleles

Period-wise distribution of Glu-1 and Glu-3 alleles
The given set of 107 wheat genotypes was divided into two groups-varieties released between 1975–1999 and varieties released between 2000–2017. Table 2 represents the allele frequency distribution of wheat varieties released between two different time periods in India. At the Glu-A1 locus, Glu-A1 subunits were present almost uniformly across the periods. However, at the Glu-B1 locus, the occurrence of subunit 17 + 18 (i) showed an increase in frequency in the newly released varieties. Interestingly, it was observed that the allele frequency of Glu-D1d (5 + 10) increased in the varieties released after the year 1999. At the Glu-A3 locus, the Glu-A3c allele showed an increase in frequency in the newly released varieties. At the Glu-B3 locus, for the first time, allele Glu-B3d was observed in the Indian varieties released after 1999. While the allele frequency of Glu-B3h was observed to be increased in the newly released varieties over the periods.
Table 2. Allele frequency distribution of 107 Indian wheat genotypes based on different periods geographical zones

Geographical distribution of Glu-1 and Glu-3 alleles
The geographical regions-wise relative frequencies of the Glu-1 and Glu-3 alleles in the set of 107 genotypes are presented in online Supplementary Fig. S5a and b, Tables S2 and S4. The values of relative frequencies are depicted in Table 2 which describes the number of times a particular allele is occurring at its respective locus in a zone. As for the Glu-B1 locus, the Glu-B1al allele (1/107 varieties) was among the rare allele only found in the NWPZ (variety of the state Delhi). Among the Glu-A3 locus, Glu-A3f was observed to occur only in NWPZ and NEPZ zones. Glu-A3e, also a rare allele was only found to be concentrated in the NEPZ zone. Glu-B3 showed a wide diversity and distinction in the presence and absence of certain Glu-B3 alleles. The Glu-B3d, a relatively rare allele was found to be strongly associated with the Punjab state of NWPZ and Himachal Pradesh state of NHZ zone.
Genetic diversity for Glu-1 and Glu-3 locus
PIC value is a good indicator of genetic variation. A high value of PIC > 0.5 indicates high genetic diversity, PIC between 0.25 and 0.5 depicts intermediate diversity, while PIC < 0.25 indicates low diversity. Genetic diversity and PIC values of individual alleles and Glu locus are depicted in Table 1. Among the different HMW-GS, the highest genetic variation was observed at the Glu-D1 locus with both Glu-D1a and GluD1d possessing genetic variation of 0.490, 0.484 and PIC values of 0.370, 0.367 respectively. The LMW-GS also depicted a wide genetic diversity. Glu-A3c was present on par with an allele frequency of 63.551. The highest genetic variation was observed at the Glu-A3c and Glu-A3b possessing a genetic variation of 0.463, 0.411 and PIC value of 0.356, 0.326 respectively.
The zone-based genetic variation also showed a diverse distribution of alleles among the different genotypes (online Supplementary Table S4). The genetic variation of most of the alleles among the zones remained uniform. However, a few alleles such as Glu-A1c and Glu-A3b depicted significantly highest genetic variation in the NHZ. Likewise, Glu-B1i and Glu-D1d genetic variation was higher in NWPZ and Glu-B3g genetic variation was highest in the PZ.
Genetic distance among the genotypes and zones
Zone-based genetic similarities and distance for Glu-1 and Glu-3 locus among the different wheat varieties is shown in online Supplementary Table S5. Close genetic similarity was observed between the genotypes falling in the region of NEPZ and CZ (0.010) for the LMW-GS at the Glu-3 locus respectively. Moreover, a close genetic similarity was also observed between NEPZ and PZ (0.016) for HMW-GS at the Glu-1 locus.
The dendrogram analysis for the Glu-1 and Glu-3 locus is shown in online Supplementary Fig. S6a and b. The phylogenetic analysis revealed that PZ and NEPZ are clustered in one group and NHZ and CZ are clustered in another group, while NWPZ forms a separate group for the HMW-GS at the Glu-1 locus. While PZ and NHZ form a cluster and CZ and NEPZ form another cluster for the LMW-GS at the Glu-3 locus.
Population structure and cluster analysis
The population structure of the 107 wheat varieties was determined by employing the Bayesian-based approach. The K value for all 107 varieties ranged between 1 and 10. The log-likelihood analysis showed the optimum K value as 2 (K = 2). Based on the (delta) ΔK method, the result suggests that the 107 varieties can be grouped into two subgroups. Cluster analysis for genotypes and their zone areas were performed based on different allelic forms found at the HMW-GS and LMW-GS loci (Fig. 2) which depicted similar results as the STRUCTURE dendrogram analysis (Fig. 3). The NJ method of cluster analysis grouped 107 varieties into two major groups. Group, I include the varieties from sub-group III, V, VIII and IX which consisted of varieties that are majorly grown in NEPZ and NWPZ zone. Additionally, a specific pattern of the combination (2* (b) at Glu-A1, 2 + 12 (a) at Glu-D1, and allele ‘c’ at Glu-A3 locus) was observed among these subgroups. Group II consisted of the remaining varieties from subgroup I, II, IV, VI and VII and was the most genetically diverse group comprising varieties from all geographical zones of India. Subgroup I, IV and VII consisted of genotypes from all the five zones and mostly have Glu-A1b, GluD1d, GluD1a and GluA3b, GluA3c, and GluB3i common in all the genotypes. The HD2270 genotype which has the rare allele Glu-B1al falls in the subgroup VI category; however, it forms a separate sub-subgroup.

Figure 2. (a) Delta K (ΔK) for different numbers of genotypes in the set (K); (b) the average log-likelihood of K-value against the number of K; (c) estimated population structure of 107 wheat varieties on K = 2 according to geographical locations. Accessions in red were clustered into group1 and the ones in green were clustered into group 2.

Figure 3. Dendrogram obtained by neighbour-joining method based on shared allele genetic distance estimates of 107 Indian wheat genotypes.
PCA analysis
PCA of HMW-GS and LMW-GS was performed to get an overview of the relationship among different alleles and genotypes. The first two components contributed to 34.75% of the variability between the samples. The first component (PC1) was formed by Glu-D1a, Glu-D1d, Glu-A1a, Glu-A1b, Glu-B1c, Glu-B3j, Glu-B3i which explained 34.75% of the initial variability between the samples, while Glu-B1a, Glu-B1i, Glu-A3d, Glu-B3c variables largely made up the second component (C2) with 13.96% of the variability. The other variables made smaller contributions to the formation of these components. The distribution of the genotypes in the space created by PC1 and PC2 is depicted in Fig. 4, online Supplementary Fig. S7. Seven alleles namely- Glu-B3c, A3f, Glu-A3e, Glu-B3i Glu-A1c, Glu-B1a and Glu-B1al depicted close association of distribution of these alleles in their respective zones (Fig. 4). A highly distinguishable dispersion in the first quadrant is formed by the Glu-B1al allele positioned near the NWPZ region. Further, the score plot differentiated all the genotypes in separate regions according to their zones and HMW-GS and LMW-GS alleles (online Supplementary Fig. S7). The result analysed by the PCA corresponds to the cluster formed by the dendrogram. The distribution of the genotypes in different clusters in the quadrants also corresponds with the groups formed by the NJ-tree analysis.

Figure 4. PCA of 107 wheat genotype lines using first two principal components from Glu-1 and Glu-3 alleles.
Discussion
The current study reports the significant genetic diversity between the Glu-1 and Glu-3 alleles in different geographical zones. Numerous combinations of HMW-GS and LMW-GS are observed in the set of 107 wheat varieties. HMW-GS depicted a wide polymorphism among the genotypes. There was no significant variation in the distribution of HMW-GS in different zones. However, this study revealed that the majority of the varieties across the geographical regions possessed Glu-A1b allele except for the NHZ which depicted the highest frequency of GluA1-null allele (50%). This study is in accordance with the earlier report which also reported the prevalence of Glu-A1b in most of the geographical zones in India (Ram et al., Reference Ram, Sharma and Sharma2015). Thus, it may be suggested that null allele was more common in varieties developed in cooler regions as compared to dry areas. Although, the adaptability of null allele to NHZ can only be confirmed if varieties with ‘b’ allele occurring in rest other can be replaced with null allele to analyse if it has any significant effect or no effect in other zones.
Among the Glu-B1 locus, subunit 20 (e) was present as the rare allele which corresponds to the earlier findings by the researchers who also reported a low frequency of subunit 20 (e) at the Glu-B1 locus (Yan et al., Reference Yan, Dai, Liu, Wei and Zheng2007). With respect to periods, there was a decrease in the frequency of 7 + 9 (c) and an increase in the frequency of 7 + 8 (u) and 17 + 18 (i) after 1999. Both (i) and (u) subunits have high glu score of 3 and 11 are associated with short mixograph mixing time, high alveograph P/L ratio, and strong gluten strength suited for bread making (Rasheed et al., Reference Rasheed, Xia, Yan, Appels, Mahmood and He2014). At the GluD1 locus, a distinguishable shift of alleles has been observed over the years. In the newly released varieties, a higher frequency of GluD1d is observed as compared to GluD1a which has drastically reduced from 72.5 to 46.4% over the two periods. The possible reason for this is the targeted selection or breeding due to inflow of CIMMYT varieties majorly possessing 5 + 10 alleles. Most of the new varieties released in India have strong base of CIMMYT lines inflow into the country. Majority of these lines comprises of 5 + 10 subunit (as per personal commn. between Ravi Singh (CIMMYT) and Anju M Singh). These lines are mostly selected and used as such based on their multilocation trials performance. The current study differs from the interpretation of earlier work by Mohan and Gupta (Reference Mohan and Gupta2017) who claimed the adaptability of GluD1a in cooler environments compared to GluD1d which is only adaptive for suppressive environments for wheat growth.
LMW-GS also depicted a high polymorphism, twelve different alleles were identified among the studied genotypes. These findings were in accordance with the study conducted by (Rai et al., Reference Rai, Singh, Ganjewala, Kumar, Ahlawat, Singh, Sharma and Jain2019). At the Glu-A3 locus, most of the alleles depicted a random distribution across the zones. However, the predominance of Glu-A3c was observed over the years and a reduced frequency was observed for Glu-A3b allele. Similar findings were observed across the zones except for NHZ, depicting the highest frequency of Glu-A3c allele. Glu-A3c is most suited for providing extensibility to the flour. Thus, it may be inferred that among the zones as well as over the years, the predominance of Glu-A3c allele in most of the varieties favours the wheat suitability for end products such as bread and pasta (Rai and Han, Reference Rai and Han2023). Further, the periodic analysis shows that the frequency Glu-B3d and GluB3h has considerably increased and Glu-B3g and Glu-B3j have decreased over the years in the Indian varieties. Across the various zones, no specific trend was observed for Glu-B3 alleles. Earlier reports by Branlard et al. (Reference Branlard, Dardevet, Amiour and Igrejas2003) and Ram et al. (Reference Ram, Sharma and Sharma2015) have indicated the presence of Glu-B3d and Glu-B3h alleles in a large number of cultivars. Glu-B3d, g and h are associated with strong gluten strength (Guzmán et al., Reference Guzmán, Crossa, Mondal, Govindan, Huerta, Crespo-Herrera, Vargas, Singh and Ibba2022). Thus, indicating that the new varieties and across the zones possess Glu-B3 alleles favourable for superior bread baking.
The genetic distance analysis showed close similarities between PZ and NEPZ at the Glu-1 locus and between CZ and NEPZ at the Glu-3 locus. The phylogenetic analysis also supported this analysis inferring that the distribution of HMW-GS and LMW-GS in the NEPZ and CZ and PZ regions are very similar compared to the subunits in the rest of the zones. The PCA analysis of these genotypes also depicted the predominance of few specific alleles in particular zones supporting the frequency distribution of alleles observed in this study. The dendrogram and cluster analysis distinguished the 107 wheat varieties into separate groups. NJ and cluster classified the genotypes based on the Glu-1 and Glu-3 allele combinations.
Interestingly, there were no distinguishing groups of zones formed based on the HMW and LMW glutenin subunits distribution among the zones. The possible reason for this may be the random distribution of alleles at the Glu-3 locus and the presence of few specific alleles (such as Null, 7, 5 + 10, 7 + 9) at the Glu-1 locus which depicted a pattern among the periods and across the zones. Thus, it may be inferred that geographical diversity had no major impact on the concurrence of most of the subunits. However, a more constructive conclusion can be made by studying the effects of individual alleles at multi locations by creating near-isogenic lines. Moreover, the periodic change or replacement of the HMW-GS and LMW-GS indicates that the newly released Indian wheat varieties are more suitable for making European bread and pasta compared to the old varieties which were more suitable for making chapatis and several kinds of flatbread.
Conclusion
The current study describes the allelic compositions of Indian wheat genotypes which displayed high allelic variability. The climate, environment and human cultural practices affected the wheat grain quality-associated alleles, leading to the variation in the frequency of HMW and LMW alleles in different zones. Moreover, from the periodic and zone-wise analysis of the varieties, it may be inferred that there are few alleles and one specific zone i.e. NHZ which has depicted distinguishing features. However, for most of the varieties across the zones, the characteristics of HMW and LMW alleles are not specific and thus it may be inferred that HMW and LMW alleles do not have any adaptive advantage and any major agroclimatic influence in their distribution among the various zones. PCA, population structure and cluster analysis in the study elucidate a better understanding of the genotypes to zone correlations. This study will help in understanding different allele combinations found in a particular zone and may be further used to predict the zone-wise genotype suitability for end-use products.
Authors’ contributions
A. M.-S. was involved in the planning and supervision of experiments, mentoring, financial facilitation and editing of the manuscript. A. R. contributed to the execution of experiments, analysis of data and drafting of the manuscript. S. S. H. helped in the availability of funds and reviewing and editing the final draft. P. S. contributed to Glu-3 molecular markers analysis of genotypes. A. K. A. contributed to grain hardness analysis. S. K. S. contributed to the analysis of HMW-GS by SDS-PAGE. P. S., S. K. S. were involved in field work in the main and off-seasons. All the authors have read and approved the final manuscript.
Financial support
AMS acknowledges the funding support provided by the ICAR under the IARI in house project on Wheat yield and quality improvement. S. S. H. acknowledges the support by the National Research Foundation of Korea (NRF) (Grant Nos. 2020R1A6A1A03044512, 2020R1A2C1012586) and the Korea Institute of Planning and Evaluation for Technology in Food, Agriculture and Forestry (IPET) through High Value-added Food Technology Development Program, funded by Ministry of Agriculture, Food and Rural Affairs (MAFRA) (321027-5).
Supplementary material
The supplementary material for this article can be found at https://doi.org/10.1017/S1479262123000382.