Discrimination, or the behaviors and unfair treatment reflecting negative attitudes and judgments about individuals in a group (Pascoe & Richman, Reference Pascoe and Richman2009), has been consistently found through meta-analysis to be associated with poor mental health across the life span (Schmitt, Branscombe, Postmes, & Garcia, Reference Schmitt, Branscombe, Postmes and Garcia2014; Yip, Wang, Mootoo, & Mirpuri, Reference Yip, Wang, Mootoo and Mirpuri2019). While the greatest amount of attention has been paid to ethnic–racial discrimination (Yip et al., Reference Yip, Wang, Mootoo and Mirpuri2019), where individuals are discriminated against as a function of their racial or ethnic group membership, discrimination has negative implications for psychological well-being regardless of attribution and across racial/ethnic groups, including White populations (Kessler, Mickelson, & Williams, Reference Kessler, Mickelson and Williams1999; Schmitt et al., Reference Schmitt, Branscombe, Postmes and Garcia2014). Indeed, the inherently negative and uncontrollable nature of discrimination may make it particularly harmful to health and well-being (Pascoe & Richman, Reference Pascoe and Richman2009; Williams & Mohammed, Reference Williams and Mohammed2009). Shift-&-persist (S&P) is a coping strategy that has been shown to lead to positive health outcomes for youth (Chen & Miller, Reference Chen and Miller2012) and adults (Chen, Miller, Lachman, Gruenewald, & Seeman, Reference Chen, Miller, Lachman, Gruenewald and Seeman2012) who face uncontrollable stress. More recent work has also found S&P to be protective in the context of general discrimination (Lam et al., Reference Lam, Miller, Chiang, Levine, Le, Shalowitz and Chen2018) and ethnic–racial discrimination (Christophe et al., Reference Christophe, Stein, Martin Romero, Chan, Jensen, Gonzalez and Kiang2019). To better understand how S&P may protect against negative mental health in the face of discrimination, we utilize the Midlife in the United States (MIDUS) dataset, and adopt a life course approach to examine how these factors impact the trajectory of depressive symptoms from age 20 to age 69.
Discrimination and Depression from a Life Course Perspective
Discrimination is a chronic and pernicious stressor that has been associated with greater concurrent and prospective depressive symptomatology (Luo, Xu, Granberg, & Wentworth, Reference Luo, Xu, Granberg and Wentworth2012; Schmitt et al., Reference Schmitt, Branscombe, Postmes and Garcia2014; Williams, Neighbors, & Jackson, Reference Williams, Neighbors and Jackson2003). Despite scholarly work outlining discrimination's ability to concurrently and prospectively predict depressive symptoms, there is much less known from a life course perspective about whether there are particular periods in life course development where discrimination may operate as a particularly harmful stressor in predicting depressive symptoms and depression.
Life course theorists have proposed that the timing of exposure to discrimination may be particularly important in understanding its impact across the life span (Gee, Hing, Mohammed, Tabor, & Williams, Reference Gee, Hing, Mohammed, Tabor and Williams2019). To date, however, only a small number of studies are available that allude to possible changes in discrimination at different stages throughout the life span, possibly due to the scarcity of such wide-ranging data. For instance, using Wave I of MIDUS, Kessler and colleagues (Reference Kessler, Mickelson and Williams1999) observed that, while overall reports of daily discrimination are lower in older cohorts compared to younger cohorts, discrimination had an equal and negative impact on mental health across age cohorts. While this may, initially, imply is that the impact of discrimination on mental health is equivalent across age, this claim cannot be made, as Kessler and colleagues’ study does not measure the intra-individual changes in associations between discrimination and depression across time. This study, and all studies focused solely on inter-individual change, cannot examine sensitive periods in individuals’ lives where risks such as discrimination pose a particularly strong risk for their negative mental health. While not examining links to psychological functioning, Gee and colleagues’ (Reference Gee, Pavalko and Long2007) study of age discrimination among working women followed for 17 years provides a window into potential intra-individual change in exposure to discrimination. Specifically, the authors observed nonlinear trajectories of discrimination frequency, where discrimination starts high in the 20s, drops in the 30s, then increases and peaks when women were in their 50s (Gee, Pavalko, & Long, Reference Gee, Pavalko and Long2007). These meaningful changes in exposure to discrimination across decades may impact individuals’ concurrent and future endorsement of depressive symptoms. In other words, as exposure to discrimination changes across the life course, its impact on concurrent and later psychological functioning may be dependent on the specific developmental stage in the life course.
The life stress literature provides a particularly useful perspective when thinking of sensitive periods where discrimination is more strongly associated with the occurrence or recurrence of depressive symptoms. In an attempt to highlight how stress exposure impacts the likelihood of depression as a function of age, Mazure and Maciejewski (Reference Mazure and Maciejewski2003) created a model, derived from data, illustrating that risk for depression due to life stressors increases in adulthood, peaks between ages 40 and 50, before declining to low levels of risk in the 60s and beyond. This would imply that discrimination may pose a particular risk for depression in midlife relative to early adulthood or old age. While the overall trajectory of depression risk due to life stressors does not fully map onto the trajectory of discrimination exposure outlined by Gee and colleagues (Reference Gee, Pavalko and Long2007), it is important to note that frequency of exposure to discrimination may not be a driver of depressive symptoms as much as the impact of discrimination during different decades/developmental stages of life. For instance, the stress sensitization model of depression (see Monroe & Harkness, Reference Monroe and Harkness2005 for review) asserts that, while initial onset of depression is more likely to be triggered by major life stressors, which typically first occur earlier in development, we become progressively more sensitized to stress and more chronic daily stressors – including discrimination – become more capable in inciting recurrences of depressive symptomatology than they did earlier in life. The authors contend that chronic daily life stressors, relative to major life stressors such as job loss and relationship dissolution, are in fact the modal stressors that precede increases in depressive symptoms later in adulthood.
Taken together, these studies provide evidence that midlife may be a particularly sensitive period where discrimination may, more strongly than is typical across the life course, predict greater levels of depression. Those in midlife are at a crossroads where their life stressors, both major and chronic, intersect leading to greater symptoms (Mazure & Maciejewski, Reference Mazure and Maciejewski2003), and at the same time, they may also be more sensitized to stressors and more likely to become depressed in response to chronic, daily stressors, such as discrimination (Monroe & Harkness, Reference Monroe and Harkness2005). In the current study, we attempt to test the claim that there is a sensitive period during midlife where discrimination is a particularly strong predictor of depression by employing an accelerated longitudinal design (Bollen & Curran, Reference Bollen and Curran2005) to assess intra-individual change across an almost 50-year time period using Waves I–III of the MIDUS study.
Shift-&-Persist
In addition to considering timing effects of discrimination, a life course perspective also posits that psychological resources may be differentially protective at specific sensitive periods in development (Gee et al., Reference Gee, Hing, Mohammed, Tabor and Williams2019). S&P coping may be such a resource that can mitigate the longitudinal negative impact of discrimination on depressive symptoms at specific points in development. Outlined by Chen and Miller (Reference Chen and Miller2012), S&P is a class of coping strategies that involve a combination of accepting and reappraising uncontrollable stressors (shift) coupled with maintaining optimism, purpose in life, and an orientation towards the future in spite of this uncontrollable stress exposure (persist). This coping strategy is theorized to be particularly well suited for uncontrollable stressors relative to direct attempts to eliminate or stop uncontrollable stressors (e.g., poverty, discrimination, etc.) and the combination of shifting and persisting in the context of uncontrollable stress that has been asserted (Chen, Reference Chen2012) and empirically demonstrated (Chen et al., Reference Chen, Miller, Lachman, Gruenewald and Seeman2012) to be more effective than either strategy in isolation. In this sense, S&P coping may be conceptualized as what Lazarus and Folkman (Reference Lazarus and Folkman1984) would refer to as a secondary-control coping strategy, or a strategy where one changes themselves to better fit the demands of the stressful environment. This is contrasted to primary-control coping strategies (Lazarus & Folkman, Reference Lazarus and Folkman1984), where individuals cope by actively altering the conditions of their environment. Attempts to actively and immediately “alter” or changes one's impoverished or discriminatory environment are likely to be unsuccessful, and these unsuccessful attempts to cope with uncontrollable stress may, themselves, elicit subsequent and exaggerated maladaptive stress responses (Chen, Reference Chen2012).
When individuals come from disadvantaged backgrounds, S&P, has been associated with a number of positive physiological health outcomes. For instance, when low-socioeconomic status (SES) individuals utilize high levels of S&P coping, they have been shown to be protected against exhibiting greater body mass indexes (BMIs) (Kallem et al., Reference Kallem, Carroll-Scott, Rosenthal, Chen, Peters, McCaslin and Ickovics2013), experiencing greater asthma-related impairment (Chen et al., Reference Chen, Strunk, Trethewey, Schreier, Maharaj and Miller2011), and demonstrating greater inflammation (Chen, McLean, & Miller, Reference Chen, McLean and Miller2015). Particularly relevant to the current study, which draws from Waves I through III of the MIDUS study, Chen and colleagues (Reference Chen, Miller, Lachman, Gruenewald and Seeman2012) conducted a study using a subset of 1,255 MIDUS II participants who provided biological samples to examine how S&P impacted allostatic load (i.e., physiological “wear and tear”) for those from disadvantaged backgrounds. The authors observed that S&P protected against greater allostatic load for those from low-SES backgrounds. Adults who grew up in low-SES conditions but used high levels of S&P coping displayed the lowest levels of allostatic load (Chen et al., Reference Chen, Miller, Lachman, Gruenewald and Seeman2012). In addition, among youth who faced high levels of general discrimination, another form of uncontrollable stress, individuals who utilized S&P coping in the face of discrimination reported better asthma control and asthma quality of life compared to those who less frequently utilized S&P coping (Lam et al., Reference Lam, Miller, Chiang, Levine, Le, Shalowitz and Chen2018). Similar to what has been found with economic stress, the protective effects of S&P coping were not observed among those who faced little general discrimination.
Recent scholarship has attempted to extend the work on S&P to examine whether S&P coping may be similarly protective depressive symptoms, an important mental health outcome that is, itself, influenced in part by inflammatory processes (Slavich & Irwin, Reference Slavich and Irwin2014). While originally conceptualized as being protective for physiological health (Chen & Miller, Reference Chen and Miller2012), secondary-control coping strategies, which encompass strategies such as S&P, have been meta-analytically associated with fewer internalizing symptoms (Compas et al., Reference Compas, Jaser, Bettis, Watson, Gruhn, Dunbar and Thigpen2017). Meaning and purpose in life, key components of persisting, have also been directly associated with fewer depressive symptoms (Sumner, Burrow, & Hill, Reference Sumner, Burrow and Hill2018). Successful S&P coping with uncontrollable stressors may not only lead to adaptive downregulations in the stress response system (Chen, Reference Chen2012), but may also reduce the impact that these stressors have on mental functioning. This conception of S&P as potentially adaptive for physiological and mental well-being stands, at face value, in contrast to recent work on “skin-deep resilience” (see Brody, Yu, Chen, & Miller, Reference Brody, Yu, Chen and Miller2020 for brief summary of this work), which suggests that upwardly mobile individuals, especially those from minority groups, may demonstrate good mental health and poor physical health. However, the factors suggested to lead to a skin-deep resilience pattern, such as self-control (Brody et al., Reference Brody, Yu, Chen and Miller2020; Miller, Yu, Chen, & Brody, Reference Miller, Yu, Chen and Brody2015) and striving, which includes components such as high educational aspirations, an intense commitment to hard work, and a “single-minded” focus on success (Brody, Yu, Miller, & Chen, Reference Brody, Yu, Miller and Chen2016), are not the same as factors characteristic of S&P coping. Stated differently, while striving and very high levels of self-control have been associated with positive mental health but poor physiological health, it remains to be seen whether S&P coping in the context of uncontrollable stress is or is not protective of mental health in the same way that it has been shown to be protective of poor physical health.
Initial work examining the impact of S&P in the context of depressive symptoms has shown that S&P is protective against greater depressive symptoms depending on the nature of the uncontrollable stressor. For instance, in a sample of Latinx youth, Christophe et al. (Reference Christophe, Stein, Martin Romero, Chan, Jensen, Gonzalez and Kiang2019) found that S&P coping was protective against the harmful effects of economic hardship on youth depressive symptoms. Further, when discrimination was examined as an uncontrollable stressor, discrimination was not associated with depressive symptoms when youth with relatively weak ethnic racial identities (i.e., meaning and importance of race/ethnicity; Umaña-Taylor et al., Reference Umaña-Taylor, Quintana, Lee, Cross, Rivas-Drake, Schwartz and Seaton2014) used high levels of S&P coping (Christophe et al., Reference Christophe, Stein, Martin Romero, Chan, Jensen, Gonzalez and Kiang2019). This protective effect of S&P against greater depressive symptoms has been found in an independent sample of 674 Mexican-origin youth (Reference Stein, Jensen, Cruz, Christophe, Martin Romero and RobbinsStein et al., under review). Specifically, low ethnic-racial identity youth who faced high levels of discrimination endorsed relatively lower levels depressive symptoms in 9th grade and had marginally more steeply declining trajectories of symptoms throughout the high school years (Stein et al., Reference Stein, Jensen, Cruz, Christophe, Martin Romero and Robbinsunder review). These two studies, thus, provide initial evidence that S&P coping may, contrary to striving (Brody et al., Reference Brody, Yu, Chen and Miller2020), be protective against poor mental (Christophe et al., Reference Christophe, Stein, Martin Romero, Chan, Jensen, Gonzalez and Kiang2019) and physical health (Chen et al., Reference Chen, McLean and Miller2015; Lam et al., Reference Lam, Miller, Chiang, Levine, Le, Shalowitz and Chen2018).
While these studies provide the basis for the notion that S&P might protect against discrimination, and specifically protect against depressive symptoms due to discrimination, this concept has not yet been tested in an adult sample, nor has it been tested across a large swath of the life span. Furthermore, while sensitive periods are most commonly explored for risk factors, Gee and colleagues (Reference Gee, Hing, Mohammed, Tabor and Williams2019) assert that sensitive periods may also exist for protective factors. Specifically, there may be times throughout development where protective factors such as S&P display particularly potent effects. Theoretically, these periods may overlap with those for stressors such as discrimination and also occur during midlife. If individuals are particularly sensitized to the effects of daily stressors in midlife (Monroe & Harkness, Reference Monroe and Harkness2005), and most likely to experience depression at that time period due to those stressors (Mazure & Maciejewski, Reference Mazure and Maciejewski2003), coping methods used during those times may be particularly efficacious. Therefore, in the current study we examine whether main effects of S&P coping and interactions between S&P and discrimination predict trajectories of depression across an almost 50-year window, as well as examining whether sensitive periods exist where S&P coping interacts with discrimination in predicting less concurrent and future depressive symptoms.
Across many of these studies, and in line with Chen and Miller's (Reference Chen and Miller2012) original theoretical assertion, S&P coping is often not directly associated with positive physiological outcomes as a main effect (Chen, Lee, Cavey, & Ho, Reference Chen, Lee, Cavey and Ho2013; Chen et al., Reference Chen, McLean and Miller2015; Kallem et al., Reference Kallem, Carroll-Scott, Rosenthal, Chen, Peters, McCaslin and Ickovics2013; Lam et al., Reference Lam, Miller, Chiang, Levine, Le, Shalowitz and Chen2018), but instead moderates the effect of the uncontrollable stressor such that those who face greater levels of uncontrollable stress – be it economic stress or discrimination – are the ones who benefit from S&P coping. Individuals who do not face pervasive uncontrollable stressors likely do not benefit from employing coping strategies such as S&P coping that are especially suited to help people cope with uncontrollable stress (Chen, Reference Chen2012; Chen & Miller, Reference Chen and Miller2012); the effectiveness of S&P, thus, may be observable only for those facing frequent uncontrollable stress (i.e., a Stressor×S&P interaction).
Trajectories of Depressive Symptoms
While there exist a multitude of studies examining trajectories of depression from adolescence into emerging adulthood, far fewer studies have examined trajectories of depressive symptoms across and through the midlife period. Regardless of the population or trajectory modeling techniques employed, these studies point to meaningful, intra-individual change in depressive symptoms across time. For instance, employing multigroup growth curve modeling using the National Longitudinal Survey of Youth, Walsemann and colleagues (Reference Walsemann, Gee and Geronimus2009) found decreasing linear trajectories of depression from ages 27 to 43 among White, Black, and Latinx participants. By contrast, in a study utilizing Waves I–V of the National Longitudinal Study of Adolescent to Adult Health, Chen and Harris (Reference Chen and Harris2019) observed nonlinear trajectories of depression where unconditional depression scores, stratified by sex, tended to decrease throughout the 20s, and increase throughout the 30s until the end of the study window at age 40. In a study of 8,801 women studied across a 20-year period, Gillis et al. (Reference Gillis, Chang, Wang, Simon, Normand, Rosner and Okereke2019) observed four different trajectories of depressive symptoms from ages 60 to 80 using latent growth curve mixture modeling: a small persistently high depression group (5%), and increasing depression group (14%), a decreasing trajectory group (19%), and a low and stable group that also appeared to be slightly decreasing over time (62%). It is important to note that 81% of this sample across the decreasing and low stable trajectories displayed at least some decline in symptomatology over time. In a study examining trajectories of negative affect in a large (N = 1992 at Wave I, 85% White), multigenerational sample of individuals, Charles, Reynolds, and Gatz (Reference Charles, Reynolds and Gatz2001) observed a linear decrease in negative affect from ages 15 to 55 that then changed trajectory and began to decrease linearly at a slower rate from age 60 through age 85. Finally, and most relevant to our current study that also uses the MIDUS sample, Blöchl and Nestler (Reference Blöchl and Nestler2019) used second-order latent growth curve modeling on Waves I through III of the MIDUS data (see Isiordia & Ferrer, Reference Isiordia and Ferrer2018 for explanation of this model) to examine how vascular risk factors (e.g., hypertension, diabetes, and smoking) impacted the linear trajectory of depression across the three study waves. Largely similar to past studies (Charles et al., Reference Charles, Reynolds and Gatz2001; Gillis et al., Reference Gillis, Chang, Wang, Simon, Normand, Rosner and Okereke2019; Walsemann, Gee, & Geronimus, Reference Walsemann, Gee and Geronimus2009) the authors also found evidence for a slightly decreasing unconditional linear slope of depressive symptoms.Footnote 1
To summarize across these findings from varying samples and using varying modeling techniques, these studies point towards meaningful differences in the intra-individual trajectory of depression during and beyond the midlife period as a function of age. Furthermore, several of these studies (with Chen & Harris, Reference Chen and Harris2019 as a notable exception) broadly point to an on-average decrease in depressive symptoms across the life course. Because intra-individual change across development has been observed for depressive symptoms (Charles et al., Reference Charles, Reynolds and Gatz2001; Chen & Harris, Reference Chen and Harris2019; Gillis et al., Reference Gillis, Chang, Wang, Simon, Normand, Rosner and Okereke2019; Kessler, Foster, Webster, & House, Reference Kessler, Foster, Webster and House1992; Walsemann et al., Reference Walsemann, Gee and Geronimus2009), it is crucial to employ modeling designs that account for developmental stage when examining both predictors of depression across the life course and sensitive periods during development when factors such as discrimination and S&P coping are particularly predictive of symptomatology relative to other developmental stages. This study builds upon these previous examinations of depressive trajectories by transforming the MIDUS study into an accelerated longitudinal design to both examine trajectory of depression across the life course as well as how exposure to discrimination and use of S&P coping influences depressive symptomatology.
Current Study
Based on life course theory, the current study attempted to conduct a developmentally informed examination of the trajectory of depression from ages 20 to 69 and examine how discrimination, S&P, and interactions between the two may concurrently and prospectively predict depressive symptoms during sensitive periods in development – specifically decades – controlling for participants’ expected trajectory of depressive symptoms across the life course. While it is, theoretically, possible to examine the effect of discrimination and S&P on depressive symptoms from year to year, we assert that, when attempting to identify specific sensitive periods during development across the life course, using a decade-based approach to approximate each period in development is more appropriate. Given past studies finding differences in initial levels and the slope of depression from young adulthood through midlife (Walsemann et al., Reference Walsemann, Gee and Geronimus2009), we also examine how initial levels of depression and the trajectory of depression may vary based on race and relevant covariates. Specifically, this study uses multigroup latent growth curve modeling with data from Waves I through III of the MIDUS study to estimate the trajectory of depression across each decade of this nearly 50-year timespan. We hypothesized that (a) depressive symptoms will show a linear decline throughout the life course and (b) controlling for covariates and the downward trajectory of depressive symptoms, discrimination will concurrently predict greater than expected levels of depression during each decade of life. We also predicted, based on the work of Mazure and Maciejewski (Reference Mazure and Maciejewski2003) that (c) a sensitive period will exist during midlife (40s/50s) where discrimination will be an especially strong and predictor of concurrent and later depressive symptoms. Finally, we hypothesized that (d) while S&P will not be associated with depression as a main effect, it will interact with discrimination during midlife such that discrimination will not be associated with depressive symptoms during midlife for those using high levels of S&P coping.
Method
Participants & procedure
Participants were 3,685 adults (M age = 37.93, SD = 6.948 range = 20–49 at Wave I) from the MIDUS dataset, a national study of health and well-being conducted by the MacArthur Foundation Research Network on Successful Midlife Development where participants were first selected through random-digit phone dialing (see Radler, Reference Radler2014 for brief description). The participants in this study represent a subsample of MIDUS participants from Project 1, the original project launched in 1995 assessing psychosocial and health-related outcomes, who were not missing on important demographic information such as race and gender. Participants included those from the national probability sample (N= 1716), the sibling sample (N = 456), the twin sample (N = 1143), and the city oversample (N = 370). Our sample was 89.2% monoracial White, with 5.7% of participants identifying as Black/African American and 2.2% identifying as Hispanic/Latina(o). Given the skewed race/ethnicity distribution, sampling weights were used to increase the influence of responses from racially minoritized participants, thus improving generalizability and partially correcting for the increased attrition observed among racially minoritized participants (see “Sample weights for race” section for greater detail). The sample was fairly evenly split with regards to biological sex (51.6% female) (only binary sex collected in study). A small proportion of our sample (5.3%) was born outside of the United States. At Wave 1, 25.6% of the sample had obtained a high school diploma, an additional 22.2% had obtained at least a bachelor's degree, and an additional 10.7% had obtained a masters of other graduate degree. (e.g., MD, JD, PhD, etc.).
Wave I was collected during 1995 and 1996, while Wave II was collected about 10 years later in 2004 and 2005. Wave III was collected during 2013 and 2014. Missingness was 25.3% at Wave II of data collection (N = 2751 present), and 46.4% at Wave III (N = 1974 present). To facilitate our comparison of trajectories of depression across development, participants were separated into three separate birth cohorts based on age at Wave I of data collection. Participants from Cohort 1 were born between 1965 and 1974 and were thus between the ages of 20 and 29 at Wave I in 1995. Participants in Cohort 2 were between 30 and 39 (birth years 1955–1966) at Wave I and Cohort 3 was between 40 and 49 (birth years 1945–1956). This study focused on understanding depression risk and resilience during sensitive developmental periods in adulthood, versus the impact of discrimination and S&P coping on depression in advanced ages (70–90+ years old), where significant physical declines, cognitive declines, and declines of social connectedness may disrupt one's previously observed trajectory of depressive symptoms. Given our focus on the former question, individuals above age 49 at Wave I were excluded from our sample, meaning that the most elderly participants in our sample were between ages 60 and 69 at Wave III of data collection. Additional demographic information for the whole sample and each cohort may be seen in Table 1.
Table 1. Sample demographics for final analytical sample

Note. Questions asking about Latinx/Hispanic origin were first asked at Wave II.
Measures
Depressive symptoms
Depressive symptoms were measured at each time point using the five items from the Kessler-6 (Kessler et al., Reference Kessler, Andrews, Colpe, Hiripi, Mroczek, Normand and Zaslavsky2002). Participants were asked how often they felt, for example, “hopeless”, “worthless”, and “that everything was an effort” in the last 30 days for 1 (all of the time) to 5 (none of the time). One item asking participants how often they felt “nervous” was dropped based on a confirmatory factor analysis with the full MIDUS I – MIDUS III data that indicated that adequate fit of a unidimensional model for depressive symptoms were obtained only when this item was dropped (Blöchl & Nestler, Reference Blöchl and Nestler2019). The remaining five items were reverse-coded such that greater scores indicated more depressive symptoms. The full Kessler-6 has displayed reliability of .89 in the nationally representative telephone-based pilot survey for MIDUS (Kessler et al., Reference Kessler, Andrews, Colpe, Hiripi, Mroczek, Normand and Zaslavsky2002). At Waves I, II, and III of our sample, reliability was .857, .853, and .849, respectively, for the five-item measure. Test–retest reliability between Waves 1 and 2 was .479, and test–retest reliability between Waves 2 and 3 was .567.
Daily discrimination
Daily discrimination was assessed at each time point using the nine-item daily scale (Williams, Yan, Jackson, & Anderson, Reference Williams, Yan, Jackson and Anderson1997). Participants were asked to respond to items such as how often “people ask as if you are dishonest” and “you are treated with less respect than other people” on a 1 (often) to 4 (never) scale. Items were reverse-coded and summed together such that higher scores indicated more frequent daily discrimination. This measure has displayed a reliability of .88 in a diverse sample of 1,139 participants from the Detroit Area Study (Williams et al., Reference Williams, Yan, Jackson and Anderson1997). Reliability in our sample at Wave 1, 2, and 3 was .855, .918, and .916, respectively. Test–retest reliability between Waves I and II was .52, and test–retest reliability between Waves II and III was .569.
Participants who indicated that they have faced some form of daily discrimination were then asked to select the main reason they were subjected to discrimination. Response options available across all three waves of data were: age, gender, race, ethnicity/nationality, religion, height/weight, physical appearance, physical disability. Participants were allowed to select multiple main reasons for facing daily discrimination. Asking about the prevalence of discrimination before asking about the attribution has been theorized to help reduce underestimation of discrimination – a phenomenon that can occur when victims struggle to clearly identify the reason for which they were discriminated against (Williams et al., Reference Williams, Neighbors and Jackson2003).
Shift-&-Persist
Shift was measured at each of the three time points using the four-item positive reappraisal subscale of the Primary and Secondary Control Questionnaire (Wrosch, Heckhausen, & Lachman, Reference Wrosch, Heckhausen and Lachman2000). Participants responded to sample items such as “I can find something positive, even in the worst situations” and “when I am faced with a bad situation, it helps to find a different way of looking at things” on a 4-point scale from 1 (A lot) to 4 (Not at all). Items were reverse-coded and averaged such that greater values indicated greater shifting, or positive reappraisal. Reliability in our sample at Wave I, II, and III was .796, .801, and .788, respectively. Test–retest reliability between Waves I and II was .562, and test–retest reliability between Waves II and III was .646.
Persist was measured at each of the three time points using the four-item “live for today” subscale of the “Planning and Making Sense of the Past Questionnaire” (Prenda & Lachman, Reference Prenda and Lachman2001). Participants responded to sample items such as “I have too many things to think about today to think about tomorrow” and “I live one day at a time” on a 4-point scale from 1 (a lot) to 4 (not at all). Items were reverse-coded and averaged, where greater scores indicated a higher propensity to persist through life. Reliability in our sample at Wave I, II, and III was .641, .637, and .609, respectively. Test–retest reliability between Waves I and II was .522, and test–retest reliability between Waves II and III was .571.
Consistent with past work with the S&P construct (Chen et al., Reference Chen, McLean and Miller2015; Kallem et al., Reference Kallem, Carroll-Scott, Rosenthal, Chen, Peters, McCaslin and Ickovics2013), and the theoretical assertion that S&P exerts a joint influence greater than the sum of its component parts (Chen, Reference Chen2012; Lam et al., Reference Lam, Miller, Chiang, Levine, Le, Shalowitz and Chen2018), we computed a single “S&P” score by creating separate mean scores for shift and for persist, standardizing these mean scores, then averaging these standardized scores together. Higher scores on this composite variable indicate greater use of S&P coping.Footnote 2 Test–retest reliability between Waves I and II was .530, and test–retest reliability between Waves IIand IIIwas .619.
Covariates
Covariates in our study include biological sex, nativity status, race, education as a measure of SES (higher values = greater education/SES), and neuroticism. Sample was also added through three dummy-coded variables for sibling sample, twin sample, and city oversample, with the main probability sample as the reference group. Due to low Ns for non-White participants, race was dichotomized such that those who identify as monoracial white were coded “0”, while those who are multiracial or of other racial minority groups were coded as “1”. Similar to our measures of discrimination and S&P, SES was entered as a time varying covariate, as education/SES may change across time. Neuroticism, which has been proposed as a factor that may influence relations between stressors such as discrimination, and psychological adjustment (Stokes, Reference Stokes2019), was measured at all three time points using a four-item neuroticism subscale generated from previous “Big-Five” inventories for the MIDUS study (Keyes, Shmotkin, & Ryff, Reference Keyes, Shmotkin and Ryff2002; Rossi, Reference Rossi2001). On a 1 (a lot) to 4 (not at all) scale, participants rated how well the words, moody, worrying, nervous, and calm described them. The moody, worrying, and nervous items were reverse coded before creating a mean score, where higher numbers indicate greater trait neuroticism. Reliability was .747 in our sample. Finally, dummy-coded variables indicating the sample a participant was drawn from (i.e., city oversample, sibling sample, or twin sample) were entered as covariates, with the national probability sample serving as the reference group.
Sample weights for race
Because racial minority groups were underrepresented in our sample relative to the population, post-stratification sampling weights were used adjusting the weight of responses by race. Using the same convention as is done with the full MIDUS sample, weights for our subsample were created by comparing the percentages of White, Black, and “Other” (i.e., non-Black minority and multiracial participants) of our sample against those from the 2013 Current Population survey from the US Census Bureau. Dividing the demographic percentages in the Current Population Survey by the percentages in our sample produced weights of .90 for Whites, 2.76 for Blacks, and 2.13 for “Others”. Using sampling weights partially corrects for the underrepresentation of minority groups being in our MIDUS I sample and partially corrects for the observed differential attrition by race in MIDUS II and III.
Results
Descriptive statistics
Before running our multigroup latent growth curve model, we calculated descriptive statistics for the whole sample and each sample separately (see Table 2). To summarize, depressive symptoms were positively associated with discrimination at each time point, and discrimination was also positively associated with S&P across time points. Neuroticism was also moderately associated with depressive symptoms at every time point (rs ≥ .328), whereas minority status and immigrant status were only weakly associated with depressive symptoms (rs .037−.136). Across cohorts and waves of data, gender was the most commonly cited reason for discrimination (36.2%), followed by race (20.4%) and height/weight (19.6%). Discrimination based on physical disability (2.8%) was the most uncommon perceived reason for discrimination.
Table 2. Means and correlations among key study variables

Note. Correlation are for the whole sample. Correlations significant at p < .05 are in bold. Dep = depressive symptoms. Disc = discrimination. S&P = shift-&-persist. The number after variable name corresponds to the Wave of data.
Data analytic plan
After running descriptives on observed study variables, inferential data analysis advanced in four steps. In the first step we estimated a multigroup latent intercept model and compared its fit relative to an unconditional linear growth model, where depressive symptoms were entered as indicators of the latent intercept and latent linear slope factors. Time was rescaled such that the intercept for the latent curve model corresponded to participants’ 20s (ages 20–29). Scaling of the slope factor for each cohort therefore corresponded to the deviation in decades from participants’ 20s (see Table 2 for visual representation). This allows the loadings for the slope factor to be identical at overlapping ages and for us to thus model growth in depressive symptoms from age 20 through age 69 (Mehta & West, Reference Mehta and West2000). Second, we tested whether homoscedasticity, or constraining the residual variances in our depressive symptom factors across time to equality, fit as well as a latent intercept and slope model where residual variances were freely estimated. This allowed us to test whether the impact of depressive symptoms on the intercept and slope varied across time.
In Step 3, we added time-invariant covariates (TICs), namely race, biological sex, immigrant status, sample, neuroticism, and parental education predicting the intercept and linear growth factors of depressive symptoms. We also added our main variables of interest, daily discrimination and S&P, as well as their product term, to the model as time-varying covariates (TVCs). In this model, which included both TICs and TVCs, TVCs predicted depressive symptoms at each time point controlling for the impact of the latent intercept and slope factor, while TICs predicted the growth factor of depressive symptoms over and above the effects of the TVCs (Bollen & Curran, Reference Bollen and Curran2005). We tested for contemporaneous and lagged effects of our TVCs (e.g., Wave I discrimination predicting Wave IIdepression) given theoretical work proposing that sensitive periods may exist throughout development where exposure to discrimination at that time is particularly harmful for later health and well-being (Gee et al., Reference Gee, Hing, Mohammed, Tabor and Williams2019). These sensitive periods are also theorized to exist for protective factors. Finally, in Step 4, we compare this conditional model to a model where the covariance between the latent intercept and slope is constrained to equality across our three cohorts of participants. This tests whether initial depression in the 20s is equally related to the slope of depression across all three cohorts. Throughout these steps, model fit was determined based on a combination of indices, where a nonsignificant chi-square, a comparative fit index (CFI) ≥ .95, an root mean square error of approximation (RMSEA) ≤ .05, and standardized root mean square residual (SRMR) ≤ .08 indicate “good” fit (Hu & Bentler, Reference Hu and Bentler1999). When appropriate, competing models are compared using the Satorra–Bentler scaled chi-square likelihood ratio test (Satorra, Reference Satorra, Heijmans, Pollock and Satorra2000). Model parameters were not interpreted in depth until we had decided upon our well-fitting, final model. Because we used sampling weights, model parameters were estimated using a maximum likelihood estimator that was robust to nonnormality (MLR). Because of our accelerated longitudinal design, some data were missing by designFootnote 3 and, thus, intentionally not handled using missing data procedures. Missing data that were not missing by design were estimated using full information maximum likelihood (FIML). All analyses were conducted using Mplus Version 8.4.
Competing latent curve models
We first fit an intercept-only model using summary scores for depression as indicators of the latent intercept (Model 1). This model provided an adequate fit to the data but did not fit better than a subsequent unconditional model (see Table 3 for model fit statistics from competing models) including a random intercept and slope factor (Scaled ΔX 2 (1) = 31.007, p < .0001) where residual variances for depression were freely estimated (i.e., heteroscedastic). This unconditional heteroscedastic model (Model 2) provided a good fit to the data (X 2 (11) = 17.359, p = .0077, RMSEA = .022, CFI = .975, SRMR = .045). There was a significant intercept (M = 1.575, p < .001) and negative slope (M = -.026, p = .001), indicating that depressive symptoms across the sample is expected to go down by .026 points per ~10-year period from ages 20 to 69. The intercept was not associated with the slope for any cohort, meaning that initial levels of depressive symptoms during the 20s was not associated with the downward trajectory of depressive symptoms across time. In this unconditional model, there was significant individual variability around the intercept of depressive symptoms (.200, p = .007) but not the slope (.021, p = .118). The unconditional heteroscedastic model was then tested against a homoscedastic model (Model 3), where residual variances for the depression factors were constrained to equality. The more parsimonious homoscedastic model provided a significantly worse fit to the data (Scaled ΔX 2 (8) = 134.782, p < .0001). Therefore, a heteroscedastic structure was retained and used in subsequent model evaluation.
Table 3. Model fit statistics from principled evaluation of competing curve of factors models

Note. * = final analytical model. Maximum likelihood robust (MLR) scaling factor is needed in the calculation of the Satorra-Bentler Scaled X 2 difference test (Satorra, Reference Satorra, Heijmans, Pollock and Satorra2000). Depressive symptoms are the dependent variable for all models. Unconditional models do not contain any covariates, while conditional models include the following covariates: socioeconomic status (SES) (time-varying), neuroticism (time-invariant), and race (time-invariant).
CFI = comparative fit index; RMSEA = root mean square error of approximation; SRMR = standardized root mean square residual; TLI = Tucker-Lewis Index.
Next, we added TICs (biological sex, immigrant status, parental education, neuroticism, and race) and TVCs (discrimination, S&P, and their interactions at each time point) to our model (Model 4). Contemporaneous and lagged effects (e.g., discrimination in 20s predicting depressive symptoms in one decade later) were assessed for our TVCs. Again, at points where our three cohorts are assessed at overlapping ages (see Supplementary Table 1), parameters were constrained to equality to facilitate the study of quasi-intra-individual change through our accelerated longitudinal design. Sex, immigrant status, and our dummy-coded MIDUS sample variables (i.e., sibling, twin, or city oversample – national probability sample is reference group) were not associated with the slope and intercept of depressive symptoms and were thus dropped for greater model parsimony. This trimmed model provided a good fit to the data (X 2 (121) = 185.896, p = .0001, RMSEA = .021, CFI = .958, SRMR = .031). Given nonsignificant covariances between the intercept and slope between each of the three cohorts, we tested whether a more parsimonious model where these covariances were set to equality would fit the data equally well. This model (Model 5) provided an adequate fit to the data (X 2 (123) = 214.059, p < .0001, RMSEA = .025, CFI = .940, SRMR = .035), but fit the data significantly worse than the Model 4, where these covariances were freely estimated by cohort (Scaled ΔX 2 (2) = 19.020, p < .0001). Given the good model fit and good fit relative to an alternative model, we used Model 4, our conditional model with TICs and TVCs where intercept slope covariance is freely estimated across cohort, as our final analytical model.
Interpreting final analytical model
In our final conditional analytical model (see Table 4), there continued to be a significant intercept (intercept = 1.578, p < .001), and a significant downward linear slope (slope = −.026, p < .001) of depressive symptoms. Again, the intercept is interpreted for all cohorts as the level of depression when participants were in their 20s. There was significant residual variation in the value of depressive symptoms in the 20s (residual variance = .107, p = .030) and the slope of depression across time (residual variance = .023, p = .018) that was not accounted for by our covariates.
Table 4. Standardized parameter estimates for final analytical model (model 4)

Note. Significant values are bolded for increased readability. – = estimate is missing by design. At points where the same parameter is assessed across multiple cohorts, that parameter has been constrained to equality across those cohorts. ↔ indicates a correlation. → indicates a regression path.
Overall, the time-invariant and time-varying predictors in the model explained a significant amount of variance in the intercept (R 2s = .447−.633, ps < .001) but not in the linear slope (R 2's = .079−.136, ps = .062−.186). The intercept of depression was not associated with the slope for any of the three cohorts. Greater neuroticism predicted a higher latent intercept of depression in the 20s (B = .446, p < .001) and a more steeply decreasing trajectory of depressive symptoms across the life course (B = −.060, p = .001), again controlling for our TVCs. Moving to our TVCs, whose levels and effects may vary by decade of life, we first examined the effects of SES (measured by education level) on depressive symptoms. During each decade of life, greater SES was associated with fewer same-decade depressive symptoms. Controlling for the negative slope of depression and all TICs, there were no contemporaneous nor lagged effects of S&P on depressive symptoms during any decade of life. During all decades of life, discrimination was concurrently associated with greater depressive symptoms controlling for the TICs and the negative linear slope of depressive symptoms across time (see Figure 1 for visual representation). There was one significant lagged effect where discrimination experienced in participants’ 40s led to greater depression 10 years later when participants were in their 50s (B = .010, p = .019), implying a sensitive period where discrimination during the 40s was particularly harmful in predicting later depression. This lagged effect occurred over and above the significant contemporaneous effect of discrimination in the 50s.

Figure 1. Graph of depressive trajectory across the life course and the same-decade impact of discrimination on depressive symptoms. Note. The symptom trajectory value should be interpreted as the level of depressive symptoms predicted based on one's symptom trajectory across time. The effect of same decade discrimination value should be interpreted as the predicted level of depressive symptoms based on one's exposure to discrimination during that decade of life. The difference between these values is equal to the significant (p < .05) beta weight for discrimination. The gray bar indicates the predicted level of depressive symptoms if participants experienced discrimination and used mean levels of S&P coping.
Examining interactions between S&P and discrimination, when participants were in their 30s, S&P and discrimination significantly interacted with each other and predicted less concurrent depression than would be expected given the negative trajectory of depression (B = −.011, p = .015). Specifically, at mean levels of S&P, discrimination predicted greater concurrent depression when participants were in their 30s (B = .019, p < .001); this effect was intensified for participants who endorsed low S&P coping in their 30s (B = .027, p < .001), but was attenuated for those who were high in S&P coping at this time (B = .011, p = .018). This means that, when participants were in their 30s, high levels of S&P coping partially buffered against the concurrent association between discrimination and depressive symptoms (see Figure 2).
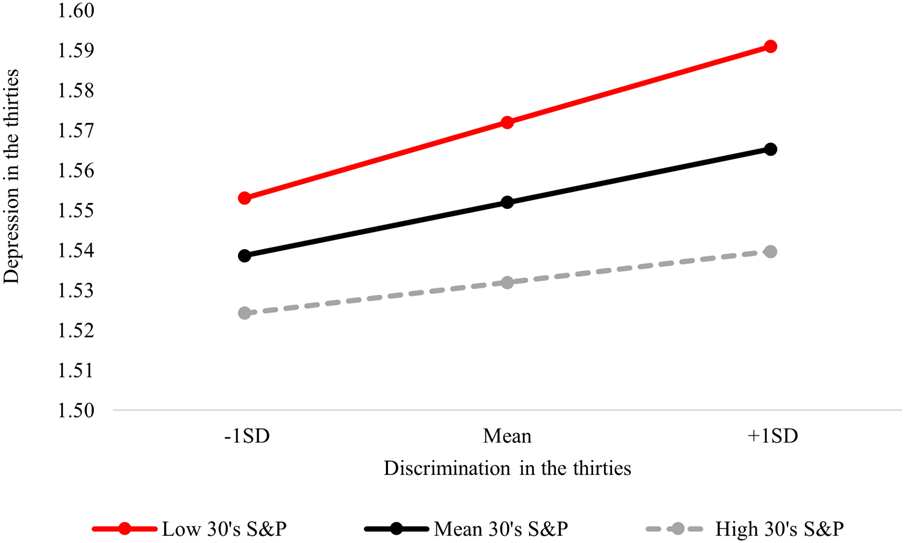
Figure 2. Simple slopes plot of the Discrimination×Shift-&-Persist (S&P) interaction predicting same-decade depressive symptoms in the 30s.
In addition, we found evidence of a lagged interactive effect where the interaction between S&P and discrimination during the 40s was predictive of depression during the 50s than what would be expected given the negative slope of depression (B = −.012, p = .022). Probing this interaction (see Figure 3) revealed that, while discrimination when participants were in their 40s predicted greater depression 10 years later if participants endorsed low (B = .019, p < .001) and mean (B = .010, p = .019) S&P coping at that earlier time, this association did not hold for those who had displayed high S&P coping while in their 40s (B = .001, p = .902). This means that while discrimination was associated with concurrent greater depression in the 40s regardless of S&P, high levels of S&P prevented discrimination from having an impact on depression 10 years later; this fully protective effect may only be observed during this specific sensitive period of development.

Figure 3. Simple slopes plot of the 10-year lagged interaction effect predicting depressive symptoms in the fifties.
Discussion
This study took a life course perspective to examine how exposure to discrimination and S&P coping impacted depressive trajectories from ages 20 to 69. The accelerated longitudinal design improves our ability both to track the trajectory of depressive symptoms throughout a large swath of the life span by modeling intra-individual change over time while also testing whether sensitive periods in development exist where discrimination, S&P, and their interactions are especially strong predictors of concurrent and prospective deviations from individuals expected trajectory of depressive symptoms. Broadly, this study aligns with past work demonstrating change across the life span in the expression of depressive symptoms (Charles et al., Reference Charles, Reynolds and Gatz2001; Gillis et al., Reference Gillis, Chang, Wang, Simon, Normand, Rosner and Okereke2019; Walsemann et al., Reference Walsemann, Gee and Geronimus2009) and affirms that discrimination is a significant predictor of depressive symptoms throughout much of the life span (Schmitt et al., Reference Schmitt, Branscombe, Postmes and Garcia2014). Furthermore, our findings expand what is known regarding the efficacy of S&P coping beyond the realm of physiological health outcomes by contributing to a small but growing body of literature demonstrating the protective effects of S&P coping for depressive symptoms. Specifically, our findings provide additional evidence that S&P coping may protect individuals against depressive symptomatology during certain sensitive periods in life course development.
We first found evidence for a significant downward trajectory of depression from ages 20 to 69. This finding builds off of previous work by Blöchl and Nestler (Reference Blöchl and Nestler2019) examining the trajectory of depression in the MIDUS sample. By transforming the MIDUS data into an accelerated longitudinal design, we, contrary to Blöchl and Nestler (Reference Blöchl and Nestler2019), were not only able to model trajectories of depressive symptoms as a function of time (i.e., the 20 years that pass between Wave I and Wave III), but also as a function of development (i.e., estimating intra-individual change from ages 20 to 69). This emphasis on intra-individual change is especially needed given previously documented within-person changes in symptomatology across the life course (Charles et al., Reference Charles, Reynolds and Gatz2001; Chen & Harris, Reference Chen and Harris2019; Gillis et al., Reference Gillis, Chang, Wang, Simon, Normand, Rosner and Okereke2019; Kessler et al., Reference Kessler, Foster, Webster and House1992; Walsemann et al., Reference Walsemann, Gee and Geronimus2009). Our findings coincide with much of this previous work and suggest that, on average, individuals endorse fewer depressive symptoms as they age up until age 69.
Examining predictors of slope of depression, neuroticism emerged as a significant predictor. Across the life course, neuroticism has been strongly associated with greater internalizing symptoms (Tackett & Lahey, Reference Tackett, Lahey and Widiger2016) and trajectories of negative affect (Charles et al., Reference Charles, Reynolds and Gatz2001). This corresponds with our finding that neuroticism in the 20s is related to initial depressive symptoms in the 20s and the slope of depressive symptoms through the 60s. Given that 20's neuroticism predicted a steeper decline in depressive symptoms over time, our finding may represent a “regression to the mean” where individuals who are highly neurotic in their 20s endorse fewer depressive symptoms across development – effectively placing these individuals back on the modal downward trajectory of depressive symptoms.
In terms of our time-varying effects, discrimination, largely consistent with Hypothesis 1, predicted greater concurrent levels of depression than would be expected based on the downward trajectory of depressive symptoms. The findings that discrimination predicts greater depressive symptoms at each decade of development is unsurprising and aligns with a large body of work (see Paradies et al., Reference Paradies, Ben, Denson, Elias, Priest, Pieterse and Gee2015; Pascoe & Richman, Reference Pascoe and Richman2009; Schmitt et al., Reference Schmitt, Branscombe, Postmes and Garcia2014; Williams & Mohammed, Reference Williams and Mohammed2009 for reviews and meta-analyses) research attesting to cross sectional positive associations between discrimination and depression. In addition, we did not observe any significant main effects of S&P on depressive symptoms. While this may cast doubt on the efficacy of S&P as a coping strategy, these findings also align with previous work finding interactive, but not main effects of S&P (Chen et al., Reference Chen, McLean and Miller2015; Christophe et al., Reference Christophe, Stein, Martin Romero, Chan, Jensen, Gonzalez and Kiang2019; Kallem et al., Reference Kallem, Carroll-Scott, Rosenthal, Chen, Peters, McCaslin and Ickovics2013). Indeed, S&P is conceptualized as a set of coping strategies that is particularly effective in the context of uncontrollable stressors (Chen & Miller, Reference Chen and Miller2012). In the absence of uncontrollable stressors such as low-SES or discrimination, S&P is not theorized and has not been shown to be associated either with improved physical (Chen et al., Reference Chen, Miller, Lachman, Gruenewald and Seeman2012; Lam et al., Reference Lam, Miller, Chiang, Levine, Le, Shalowitz and Chen2018) or mental health (Stein et al., Reference Stein, Jensen, Cruz, Christophe, Martin Romero and Robbinsunder review; Christophe et al., Reference Christophe, Stein, Martin Romero, Chan, Jensen, Gonzalez and Kiang2019). “Goodness of fit” is an important factor to consider when examining coping; other active coping strategies that are aimed at altering the environment itself may be more suited to coping with controllable stressors, while changing oneself to the demands of the environment (e.g., shifting and persisting) may be more adaptive when stressors are uncontrollable (Lazarus & Folkman, Reference Lazarus and Folkman1984; Stanisławski, Reference Stanisławski2019). Therefore, theory would assert that S&P should be principally adaptive in the context of high levels of uncontrollable stress, which is what we found when examining time-specific interactions between S&P and discrimination.
In terms of context-specific effects, which imply especially sensitive decades in development where the effects risk and protective factors may be particularly pronounced, we found evidence for Hypothesis 2 in the form of two time-specific interactions between S&P and discrimination. The first was a concurrent interactive effect observed during the 30s. Specifically, the positive relation between discrimination and depressive symptoms in the 30s were weakened at high levels of S&P coping. We also observed a lagged interactive effect where discrimination when participants were in their 40s predicted greater depression than would be expected in the 50s. This lagged effect, however, was attenuated by S&P coping during the 40s and nonsignificant when S&P was at high levels. This lends credence to the hypothesis proposed by Gee and colleagues (Reference Gee, Hing, Mohammed, Tabor and Williams2019) that sensitive periods exist both for risk factors (i.e., discrimination), but also for protective factors (i.e., S&P).
Relative to other points along the life course, there may be something unique about facing discrimination and using S&P coping in the 30s and especially in the 40s where these two factors interact to predict depressive symptoms a full 10 years later. Historically, psychologists such G. Stanley Hall conceptualized midlife as spanning from 25 or 30 to 40 or 45 years of age and described this as when we are, generally, at the peak of our abilities (Reference Hall1922). While other theorists commonly place “midlife” slightly later in the life cycle, Lachman and colleagues (Reference Lachman, Teshale and Agrigoroaei2015) highlight that Jung and Erikson also saw midlife as a critical time where individuals are “negotiating and regulating growth and decline” (p. 21). Given that midlife likely constitutes the intersection of various areas of growth and decline (see Lachman, Teshale, & Agrigoroaei, Reference Lachman, Teshale and Agrigoroaei2015 for visual representation), uncontrollable stressors such as discrimination when individuals are supposed to be approaching and at this peak of life may be particularly detrimental to their psychological well-being. Specifically, midlife may be theorized as a time of relative social and financial stability (Alwin, McCammon, Mortimer, & Shanahan, Reference Alwin, McCammon, Mortimer and Shanahan2003); to the extent that discrimination in different social and occupational contexts has the ability to disrupt this life stability, one may expect meaningful concurrent and long-lasting impacts on well-being and on depressive symptoms.
These life course perspectives explaining the justification for our finding that sensitive periods for risk and protective factors occur when entering and in midlife align with those from the life stress field. For instance, stress sensitization theory would predict that, during midlife, daily and chronic stressors such as discrimination may more easily trigger bouts of depressive symptoms than at points earlier in development (Monroe & Harkness, Reference Monroe and Harkness2005). Interestingly, the partially protective, buffering interactive effect of S&P occurs during the 30s a time where risk for depression due to different life stressors is gradually increasing (Mazure & Maciejewski, Reference Mazure and Maciejewski2003). However, it is only at the peak of risk for depression due to life stressors, the 40s, where S&P demonstrated a fully protective effect in minimizing the typically harmful effect of discrimination on depressive symptoms. Our results, therefore, may illustrate the gradual start of this sensitive period in the 30s that then reaches its zenith during the 40s; S&P coping may be, thus, increasingly important in the 30s and maximally important and effective in the 40s relative to other times during adult development.
While theoretical scholarship has posited the existence and highlighted the potential importance of identifying sensitive periods during adulthood (Gee, Walsemann, & Brondolo, Reference Gee, Walsemann and Brondolo2012; Gee et al., Reference Gee, Hing, Mohammed, Tabor and Williams2019), the broader field of psychology has studied sensitive periods almost exclusively in the context of early childhood development. Indeed, much work has focused on increased capacity for how factors such as early infant–mother separation and child maltreatment influences epigenetic changes implicated in the development of mental disorders (Roth & Sweatt, Reference Roth and Sweatt2011), while other work has broadly pointed to the benefits of positive parenting “the earlier the better” to take advantage of sensitive periods in neurodevelopment occurring during the first months of life (Zeanah, Gunnar, McCall, Kreppner, & Fox, Reference Zeanah, Gunnar, McCall, Kreppner and Fox2011). Our findings, in concert with previously cited empirical work proposing sensitive periods in adulthood for discrimination (e.g., Gee et al., Reference Gee, Walsemann and Brondolo2012) and life–stress work pointing to variable risk for depressive episodes at different points in the life course (Mazure & Maciejewski, Reference Mazure and Maciejewski2003), suggest that sensitive periods are not, however, exclusively found during early development. In studying sensitive periods during other periods of development, scholars’ conceptions of the potential “window of opportunity” during which sensitive period may occur may need to be adjusted based on the developmental stage and phenomenon being studied. In our study, for instance, we operationalize a sensitive period for depression during the life course as a 10-year window, which stands in stark contrast to the month-long to week-long sensitive periods observed in human and animal models of early development (see Knudsen, Reference Knudsen2004 for examples). Ultimately, much more empirical work is needed to uncover and understand the environmental, social, neural, genetic, and epigenetic mechanisms behind potential sensitive periods in midlife. These nascent efforts may be strengthened by adapting and synthesizing the schemas, models, and methods used when studying early childhood sensitive periods with those employed by those studying life course development.
Controlling for the effect of discrimination and S&P at each wave of data, we failed to find significant differences in the intercept and slope of depression between White and non-White participants. It is important to note that tests for racial differences in initial levels of depression and its trajectory were done controlling for discrimination, a robust predictor of greater depressive symptoms among ethnic/racial minorities at different stages of development (Benner et al., Reference Benner, Wang, Shen, Boyle, Polk and Cheng2018; Paradies et al., Reference Paradies, Ben, Denson, Elias, Priest, Pieterse and Gee2015). Therefore, by controlling for discrimination, there may be no remaining difference in depressive trajectories between Whites and racially minoritized individuals, as the construct of race may have no longer served as a proxy variable for social disadvantage. Indeed, only 10.8% of our sample were from ethnically/racially minoritized groups, and despite our use of sampling weights to partially correct for this low level of representation, our sample sizes are too imbalanced to draw definitive conclusions as to the impact of race on the trajectory of depressive symptoms across the life course. In addition, given our small percentage of non-White participants, we made the decision to dichotomize race and preserve statistical power despite the ready admission that the lived experiences of various marginalized groups may differ widely and that this approach may not be sensitive to important between (i.e., between minoritized groups) and within-group variability (Hall, Yip, & Zárate, Reference Hall, Yip and Zárate2016). Future culturally sensitive longitudinal work on discrimination may be better suited to tease apart potential differences in the course of depression throughout the life span if they include balanced samples of Whites and individuals from multiple ethnically/racially minoritized groups.
Limitations and Conclusions
Despite its contribution, this study has several limitations that set the stage for future work informed by a life course perspective. First, while our cohort sequential, or accelerated longitudinal design, allowed us to examine the trajectory of depression across a 49-year period, each cohort included individuals as far as 9 years apart in age. Our inferences are, thus, limited to the level of the decade, whereas the effects of discrimination and S&P coping likely also have more short-term effects on depressive trajectories. While difficult to extend over such a time period, future work should test for both short-term (e.g., 1-, 3-, or 5-year-) and long-term (10+-year) effects of these factors on the course of depression throughout the life span. These types of short-term longitudinal tests of S&P may be particularly useful in the study of child and adolescent development, where there are already well-established, albeit shorts sensitive periods that spur rapid developmental change.
Secondly, we chose to measure depressive symptoms and S&P as TVCs which assumes that these factors themselves do not change over time in a systematic way. While cognitively based coping strategies, such as S&P, may come online during adolescence (Compas et al., Reference Compas, Jaser, Bettis, Watson, Gruhn, Dunbar and Thigpen2017), we are aware of no scholarly work that maps changes in the frequency of S&P coping across time. A limited amount of work has begun to examine how discrimination unfolds across long periods of time. For instance, using 3,032 participants from Wave I of MIDUS, Kessler and colleagues (Reference Kessler, Mickelson and Williams1999) found that younger cohorts (age 25–44) reported greater everyday discrimination than did those over 65. However, because these differences are between individuals, inferences for how discrimination unfolds across the life span (i.e., age effects) cannot be inferred. Furthermore, it is unclear whether those observed differences in discrimination reflect what Gee et al. (Reference Gee, Walsemann and Brondolo2012) refer to as “age-patterned responses”, or differences in prevalence due to stage in life, or period effects, or changes brought on by historical events and/or social transition. Indeed, participants who were over the age of 65 during MIDUS I data collection in 1995/1996 were young adults during the civil rights movement; living through this time may have both influenced what these individuals perceive (and report) as discrimination relative to those younger individuals in Kessler et al.'s (Reference Kessler, Mickelson and Williams1999) MIDUS I sample who were not alive during this period of social change.
By contrast, in a sample of 7,225 working women followed for 15 years, Gee and colleagues (Reference Gee, Pavalko and Long2007) found that age-based discrimination peaked in the 20s and 50s, was low during the 30s, and declined from the 50s peak around age 55 age discrimination, which implies that an individual's exposure to discrimination changes over as a function of their age. While Gee et al.'s (Reference Gee, Pavalko and Long2007) study design is particularly useful in its ability to illustrate within-person change over time, such a design is not well suited to account for significant legal or social changes during this 15-year time frame (i.e., any period effects) that may have impacted women's exposure to workplace discrimination. While we have no reason to believe specific period effects significantly impacted our findings related to individual's trajectory depressive symptoms over time or age-based changes in the effects of discrimination and S&P on symptomatology, it is impossible to rule out the potential influence of small and unmeasured influences due to time and context. To better disaggregate potential period effects, which may be observed between cohorts, from time patterned responses, which may only be observed via intra-individual change over time, scholars should endeavor to examine and compare intra-individual changes in factors such as discrimination between samples exposed and not exposed to potentially meaningful period effects (e.g., pre-post civil rights legislature, pre-post federal legalization of same-sex marriage, etc.).
Finally, a notable limitation examining the effects of discrimination in the MIDUS sample is the relative racial/ethnic homogeneity of the MIDUS sample. Although we utilized sampling weights to increase the influence of non-White participants in the estimation of our parameters, the fact remains that White participants made up almost 90% of our sample. This sample homogeneity limited both the amount of reported racial/ethnic discrimination we observed across the life course and limited our power to detect reliable differences in our estimates based on race. Future work would benefit from examining large, targeted samples of racially/ethnically minoritized individuals to better and more reliably observe the impact of racial discrimination on depressive symptoms throughout the life course. Indeed, if systematic change over time exists in discrimination, the pattern of change over time may vary based on the type of discrimination. While used as control variables, Gee and colleagues (Reference Gee, Pavalko and Long2007) observed that patterns of racial and gender-based discrimination did not mirror patterns of age-based discrimination. Future work may, therefore, benefit from examining multiple types of discrimination concurrently and from using more restricted samples if the substantive interest is on understanding specific types of discrimination (e.g., using a Muslim-American sample to study religious and ethnic–racial discrimination).
Despite its limitations, the current study adds to our understanding of how depressive symptoms change over the life course, as well as how discrimination and S&P coping impacts depressive symptomatology both contemporaneously and up to 10 years later during sensitive periods in adult development. Our findings demonstrated that, among a representative sample of adults in the US, depression follows a slightly downward linear trajectory from ages 20 through 69. Our use of an accelerated longitudinal design allows us to approximate intra-individual change in depressive symptoms over time and move beyond population-based epidemiological studies that compare rates of depression between individuals at different ages. In addition, our findings add to the bodies of work that have independently examined the impact of discrimination at different points along the life course by showing that discrimination is predictive of greater depressive symptoms throughout human development over and above the natural negative trajectory of depression observed with aging. Finally, our study provides further evidence that, similar to what has been found with physiological health, S&P may also be protective for mental health. Chen et al. (Reference Chen, Miller, Lachman, Gruenewald and Seeman2012), using MIDUS Waves I and II, found that adults who grew up in poverty but displayed high levels of S&P coping endorsed the lowest levels of physiological wear and tear, whereas our findings using MIDUS Waves I, II, and III suggest that S&P buffers against the harmful impact of discrimination on depressive symptoms in the 30s and is crucial in the 40s at protecting against the long-term impact of discrimination 10 years later. These findings point to potential sensitive periods of human development (Gee et al., Reference Gee, Hing, Mohammed, Tabor and Williams2019) where the impact of S&P may be particularly important in promoting psychological well-being. In conclusion, this study adds to the field by adopting developmental and life course perspective to the study of how discrimination and S&P coping impact depressive symptoms from ages 20 to 69.
Supplementary material
The supplementary material for this article can be found at https://doi.org/10.1017/S0954579421000146
Financial Support
This work was supported in part by a predoctoral fellowship provided by the National Institute of Child Health and Human Development (T32-HD07376) through the Carolina Consortium on Human Development, University of North Carolina at Chapel Hill, to N. Keita Christophe
Conflicts of Interest
None.