Diet is largely recognised as a major determinant of health, and nutritional (risk or protective) factors have been consistently associated with mortality(Reference Schwingshackl, Schwedhelm and Hoffmann1), morbidity(Reference Schwingshackl, Hoffmann and Lampousi2–4) and intermediate criteria(Reference Schwingshackl, Schwedhelm and Hoffmann5). Diet is considered a key lever to public health policies as it represents a changeable factor that may be modified through primary prevention interventions. Population dietary guidelines are thus developed and regularly updated according to the progress in scientific knowledge. To quantify the potential impact of such guidelines, the association between adherence to recommendations and mortality and morbidity needs to be tested.
A first step towards this goal is to assess how well populations comply with these guidelines. A common, easy and efficient approach for this is to compute an index reflecting individual adherence to the recommendations. In many countries, such indices of adherence with national dietary guidelines have been developed(Reference Estaquio, Kesse-Guyot and Deschamps6–Reference Guenther, Reedy and Krebs-Smith8), and then correlated with morbidity events(Reference Onvani, Haghighatdoost and Surkan9–Reference Kourlaba and Panagiotakos11) in cohort studies, allowing to estimate the predictive value associated with adherence to nutritional recommendations.
Dietary indices reflecting adherence to guidelines are a priori developed food or nutrient-based diet quality scores(Reference Waijers, Feskens and Ocké12). The major interest of dietary indices over approaches singling out foods or nutrients is their encompassing diet as a whole, therefore taking into account interactions and/or synergies between dietary components(Reference Ocké13). Although national guidelines are usually developed based on nutritional collective expertise, the validity of a given guideline-based index needs to be investigated.
As dietary guidelines evolve over time accounting for advance in scientific knowledge, dietary indices need to evolve concurrently: for example, the Healthy Eating Index (HEI)(Reference Kennedy, Ohls and Carlson14) was updated to the HEI-2005(Reference Guenther, Reedy and Krebs-Smith8), HEI-2010(Reference Guenther, Casavale and Kirkpatrick15) and HEI-2015(Reference Krebs-Smith, Pannucci and Subar16).
In 2001, in the framework of the first French Nutrition and Health Programme (Programme National Nutrition Santé, ‘PNNS’, 2001–2005), 2001–2005 nutritional guidelines were adopted and disseminated in the general population. These guidelines provided eight dietary recommendations and one for physical activity(Reference Hercberg, Chat-Yung and Chauliac17). Dietary recommendations were classified as four adequacy (‘fruits and vegetables’, ‘starchy foods’, ‘milk and dairy products’ and ‘meat, fish and seafood and eggs’), and four moderation recommendations (‘added fat’, ‘sugary products’, ‘drinks’ and ‘salt’). In this context, we developed a specific PNNS – guidelines score (PNNS-GS) allowing measuring adherence with these national dietary guidelines(Reference Estaquio, Kesse-Guyot and Deschamps6).
In March 2017, in the preparation of the fourth PNNS (2017–2021), the High Council of Public Health (Haut Conseil de Santé Publique, ‘HCSP’) issued a report updating the 2001 PNNS nutritional guidelines(18), based on a French Agency for Food, Environmental and Occupational Health & Safety (Agence nationale de sécurité sanitaire de l’alimentation, de l’environnement et du travail; ANSES) scientific report(19). These 2017 recommendations provided six adequacy (‘fruits and vegetables’, ‘nuts’, ‘legumes’, ‘whole-grain food’, ‘milk and dairy products’ and ‘fish and seafood’), and six moderation recommendations (‘red meat’, ‘processed meat’, ‘added fat’, ‘sugary products’, ‘drinks’ and ‘salt’). Physical activity was not addressed by the HCSP.
Therefore, the present study aimed (i) at developing an a priori food-based dietary index reflecting adherence to the 2017 French nutritional guidelines, (ii) at evaluating the validity of this score using a content, construct and criterion model of validation and (iii) at describing its association with sociodemographic and nutritional factors in the NutriNet-Santé cohort.
Subjects and methods
Study population
We used data from the NutriNet-Santé study, a large web-based observational cohort launched in France in 2009. Its design and methodology have been described in detail elsewhere(Reference Hercberg, Castetbon and Czernichow20). The study was designed to investigate the relationship between nutrition and health, as well as determinants of dietary behaviour and nutritional status. Participants aged over 18 years with access to the Internet were recruited among the general population by means of vast multimedia campaigns. All questionnaires were completed online using a dedicated secure website (www.etude-nutrinet-sante.fr). The NutriNet-Santé study is conducted in accordance with the Declaration of Helsinki and was approved by the ethics committee of the French Institute for Health and Medical Research (institutional review board Inserm no. 0000388FWA00005831) and by the National Commission on Informatics and Liberty (CNIL, no. 908450 and no. 909216). Electronic informed consent was obtained from all participants. The NutriNet-Santé study is registered in ClinicalTrials.gov (NCT03335644).
In addition, during 2011–2014, the NutriNet-Santé participants were invited, on a voluntary basis, to attend a visit for biological sampling and clinical examination in local centres. Electronic and paper written informed consents were obtained from all participants attending the visit. All procedures were approved by the ‘Consultation Committee for the Protection of Participants in Biomedical Research’ (C09-42 on 5 May 2010) and the CNIL (no. 1460707).
Dietary data
Participants provided at baseline and then twice a year three non-consecutive 24 h dietary records assigned over a 2-week period. Days were randomly attributed into two weekdays and one weekend day to account for intra-individual variability intake. Participants recorded all food and drink consumption throughout the entire day (midnight to midnight) via a dedicated online platform allowing users to search for any food by name. Data used in the present paper are based on participants included between 2009 and 2014. Amounts consumed were declared as absolute units when known (in g or ml), common household measures or using generic food portion size from previously validated photographs(Reference Le Moullec, Deheeger and Preziosi21).
Consumption was weighted according to the type of day (weekday v. weekend day) and under-reporters were excluded using the Black method with Goldberg cut-offs, which are based on physical activity level (PAL) and BMR (calculated as per Schofield’s equations(Reference Schofield22)), which itself takes into account sex, age, height and weight. Within-subject day-to-day CV of energy intake was calculated for each participant (median = 0·23, range = 0·001–2·02) based on their dietary record data (number: median = 6, range = 3–15 dietary records), and within-subject CV for BMR and PAL were defined at 8·5 and 15 %, respectively(Reference Black23). The mean calculated lower cut-off was 0·99 (range = 0·90–1·07).
The lower limit was first calculated with a default PAL defined at 0·88 to exclude extreme under-reporters (invalid values), and then re-run with PAL defined at 1·55 to compute the proper coefficient of variation of energy-intake. Daily energy and nutrient intakes were computed using a validated and constantly updated composition tables including more than 3000 food items(24). The NutriNet-Santé web-based, self-administered 24 h dietary records have been tested and validated against an interview by a trained dietitian and against blood and urinary biomarkers(Reference Touvier, Kesse-Guyot and Méjean25–Reference Lassale, Castetbon and Laporte27).
Frequency of organic food consumption was recorded within 2 months after inclusion for fruits, vegetables, bread, rice, pasta and legumes using a previously described questionnaire(Reference Baudry, Méjean and Péneau28). For the present study, ‘rice, pasta and legumes’ organic food consumption frequency was divided into rice and pasta on the one hand, and legumes on the other hand, based on non-null consumption of the food group in 24 h dietary intakes. Frequencies were assessed using eight possibilities of response: (1) most of the time; (2) occasionally; (3) never (too expensive); (4) never (product not available); (5) never (I’m not interested in organic products); (6) never (I avoid such products); (7) never (for no specific reason); and (8) I don’t know. In the present study, all ‘never’ responses (3, 4, 5, 6 and 7) were aggregated.
Other data
Individual sociodemographic and lifestyle characteristics (age, sex, education, occupation, income, marital status, physical activity and smoking habits) were collected, at baseline and then yearly, by a dedicated self-administered web-based questionnaire(Reference Lassale, Castetbon and Laporte27). In the same period of time, height and weight were self-reported to compute BMI. Physical activity was assessed using the International Physical Activity Questionnaire (IPAQ)(Reference Hallal and Victora29). Income per consumption unit (CU) was estimated from monthly household salary and household composition according to a weighting system where 1 CU is attributed for the first adult in the household, 0·5 CU for other persons aged 14 years or older, and 0·3 CU for children under 14 years(30). Although self-reported, these indicators have demonstrated good validity against clinical measures(Reference Lassale, Péneau and Touvier31).
Clinical examinations that included blood pressure measurement and blood samples were performed on 19 620 volunteers between February 2011 and June 2014. Blood pressure, blood glucose (hexokinase on C8000 automat, Abbott), serum TAG (glycerol kinase C8000, Abbott), total serum cholesterol (cholesterol oxidase C8000, Abbott), HDL-cholesterol (direct accelerator C8000, Abbott) were measured, and LDL-cholesterol was calculated using the Friedewald formula(Reference Friedewald, Levy and Fredrickson32). These metabolic variables were not specifically chosen for this analysis, but were part of a standard routine check-up. All blood samples were taken after a minimum of 6 h of fasting. For each biochemical analysis, any patient who had been treated for a related condition was excluded (i.e. we excluded glucose analysis from all patients treated for diabetes).
Development of the Programme National Nutrition Santé – guidelines score 2
The PNNS-GS2 is an index designed to reflect the 2017 French dietary recommendations(18, 33) which include main and complementary recommendations. PNNS-GS2 components and scoring are presented in Table 1. For comparison, the PNNS-GS(Reference Estaquio, Kesse-Guyot and Deschamps6) components and scoring are presented in online Supplementary Table S1.
Compared with the 2001 recommendations, 2017 recommendations are more numerous (12 v. 9), include more specific food groups and for some were revised according to an update of the scientific literature.
First, some food group components were refined: bread, cereals and potatoes were no longer specific components of the score, and legumes were an independent component, whereas the adequacy component for animal products (meat, poultry, seafood and eggs) was divided into two moderation components for red meat and for processed meat. White ham ratio was added as a conditional component for processed meat because it contains less fat than other processed meats, so its consumption provides additional score points only in processed meat consumers.
Table 1. Programme National Nutrition Santé – guidelines score 2 (PNNS-GS2): components and scoring
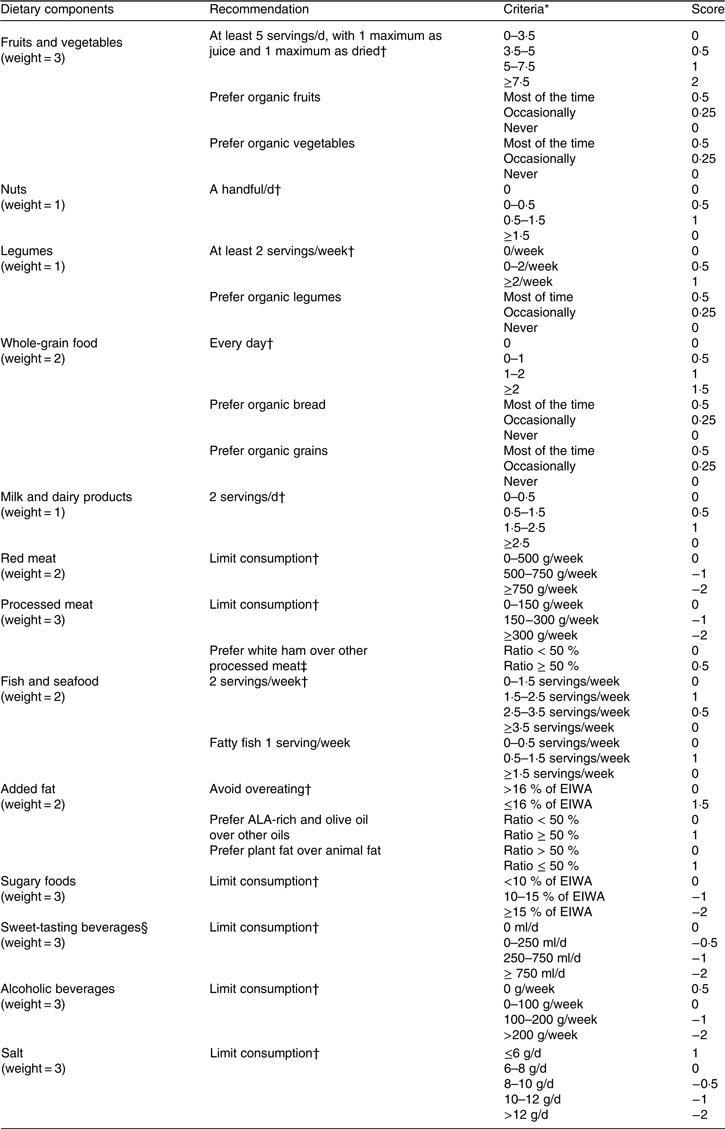
EIWA, energy intake without alcohol; ALA, α-linolenic acid.
* Servings per d unless otherwise stated.
† Principal recommendations.
‡ Conditional: the 0·5 bonus point only occurs if total processed meat consumption is more than 150 g/week.
§ Sweet-tasting beverages are specifically sugary beverages, artificially sweetened beverages and fruit juices.
Second, cut-offs were lowered for the milk and dairy, seafood and alcohol components.
Third, some components were modified. Nuts were added and whole-grain food was modified from a ratio-based to a serving-based component. The beverages component (for which consumption should be limited), compared with the previous guidelines, still contains sugary drinks, but now also includes artificially sweetened (light/diet) beverages and fruit juices, the target being more the sweet taste than the actual sugar content. A sugary drink was defined as a beverage without alcohol and containing added sugar.
Fruits and vegetables (which still do not include potatoes), sugary foods (foods containing added sugar such as most of breakfast cereals, sorbet, biscuits, cakes etc.) and salt components remained unchanged. For added fat, the 2017 recommendation highlighted α-linolenic acid-rich oils and olive oil as preferred options. For clarity purpose we used ‘recommended oils’ throughout the manuscript.
Finally, an organic food consumption recommendation was introduced for fruits, vegetables, breads, legumes and cereals as a proxy of a reduced exposure to contaminants.
The PNNS-GS2 builds on the distinction between malus components (less healthy food groups whose consumption should be limited, carrying a negative score of moderation, e.g. salt) and bonus components (healthier food groups carrying a positive score of adequacy, e.g. legumes).
Servings were defined based on French usual portion sizes, as follows: 80 g for fruits and vegetables, 30 g for dried fruits, 150 ml for fruits and vegetables juices, 30 g for nuts, 200 g for legumes, rice and pasta, 30 g for cereals, 50 g for bread, 150 ml for milk, 125 g for yogurt, 30 g for cheese, and 100 g for fish and seafood.
Cut-offs and associated scorings were defined with the support of nutrition experts who were involved in the development of the guidelines. They were built so that reaching and not reaching a guideline component was rewarded, respectively, with 1 point and 0 point in adequacy components (healthier food groups), and with 0 points and −1 point in moderation components (less healthy food groups), with half-points given linearly to improve discrimination power between and above cut-offs. For milk and dairy products and for fish, a parabolic-shaped relationship was used to allocate points, as the relation to overall health was found non-linear(19). As a sensitivity analysis, we computed a simplified version of the PNNS-GS2, named simplified PNNS-GS2 (sPNNS-GS2), including only principal recommendations (exclusion of organic food, fatty fish, white ham ratio, recommended oils ratio and animal fat components). The sPNNS-GS2 was also built to ensure a higher comparability with PNNS-GS and to take into account the fact that some components are rarely available (organic food consumption in particular). To avoid overrating multi-item components, each component was standardised and weighted. Therefore, scores were computed for each participant as the sum of each component i multiplied by its associated weight and divided by its maximum absolute value:

Weights were defined according to the level of evidence of the association between food groups consumption and health(Reference Schwingshackl, Hoffmann and Lampousi2–Reference Schwingshackl, Schwedhelm and Hoffmann5). They were defined at 3 for fruits and vegetables, processed meat, sugary foods, alcoholic beverages, sweet-tasting beverages and salt, 2 for whole-grain food, red meat, fish and added fat, and 1 for nuts, legumes and milk and dairy products. Physical activity is no longer part of the score as it is handled by specific guidelines.
In order not to overrate overconsumers, and since French dietary guidelines are not expressed as nutrient density, PNNS-GS2 was penalised on energy intake similarly to the method used for the PNNS-GS: energy expenditure was computed from BMR and PAL as per FAO tables(34). Participants reporting energy intake of more than 105 % of their energy expenditure (n 6500) had their score reduced by the same proportion(Reference Estaquio, Kesse-Guyot and Deschamps6).
Theoretical range of both PNNS-GS2 and sPNNS-GS2 went from −17 to 13·5, but actual scores may be lower than the threshold given the penalisation on energy intake. In this article, PNNS-GS2 will be compared with a modified version of the PNNS-GS (mPNNS-GS), computed without the physical activity component.
Sample selection
For the present analysis, we included all participants who filled in at least three 24 h dietary records during the first 2 years after their inclusion in the NutriNet-Santé study (n 115 536). After exclusion of under-reporters, 104 382 participants were selected. Among them, 5928 participants with missing value on energy expenditure were excluded, leaving 98 454 participants for the computation of the sPNNS-GS2. A total of 17 489 participants had missing values on organic food consumption, so the PNNS-GS2 was computed among 80 965 participants. Among these, 16 938 had available clinical and biological data. This sample is referred to as ‘the clinical sample’ hereafter.
Score validation
To evaluate the validity of the construction of the PNNS-GS2, we used the validation process summarised by Bland & Altman(Reference Bland and Altman35):
Face validity is met if the score appears correct for experts (i.e. it addresses relevant items),
Content validity is met if the score appears complete (i.e. it does not lack any important item),
Convergent construct validity is met if the score is associated with the same variables than the object it measures, i.e. diet quality
Criterion validity is met if the score is the same as an objective value.
In the present study, content and face validity were assumed given the expert-based development of the score. Convergent construct validity was evaluated through the association of the PNNS-GS2 with sociodemographic and biological variables known to correlate with diet quality. Criterion validity could not be fully achieved due to the absence of a gold standard for diet quality, but we estimated the correlation between quantiles of PNNS-GS2 and quantiles of mPNNS-GS with Cohen’s κ and Spearman’s correlation coefficient. We hypothesised that concordance estimates should not be too high, as it would have implied that the update did not add much information to the score, nor too low, as it would have implied that the PNNS-GS2 measured a totally different construct than the PNNS-GS. Ultimately, internal consistency was estimated with Cronbach’s α.
Statistical analysis
For descriptive purpose, micronutrients intakes were adjusted for energy intake by the residual method(Reference Willett and Stampfer36), and macronutrients were computed as a percentage of energy intake without alcohol (since French recommended levels of intake are expressed without alcohol). All the nutritional indicators were presented across quintiles (Q) of the PNNS-GS2 calculated for men and women separately.
Difference between Q5 and Q1 was computed as relative to total ((Q5 − Q1)/total) and as absolute (Q5 − Q1). Relative difference was thought to be more appropriate to describe components reached by a low percentage of subjects, and absolute difference to describe other components. Reaching a score component was defined as having a score of 1 or more for adequacy components and of 0 for moderation components.
Associations were tested with a Pearson χ 2 association test for unordered factors with more than two levels, with a Cochran–Armitage test for trend for unordered factors with two levels exactly, with a Spearman correlation test for ordered factors and with a linear contrast test for numeric variables. All analysis on biological and clinical variables for the clinical sample were conducted on the logarithm values and adjusted for energy intake without alcohol, alcohol consumption and age at baseline.
All statistical analyses were conducted using R (version 3.4.2) with a significance level of 5 % for two-sided tests.
Results
The sample included 78·1 % women and 21·9 % men, with an average of 6·6 (sd 2·8) dietary records (minimum = 3; maximum = 15). PNNS-GS2 and sPNNS-GS2 were approximately normally distributed (kurtosis = −0·1 and −0·03, skewness = −0·23 and −0·27, respectively). Excluded participants (with missing value for energy expenditure or for organic food consumption) compared with included ones were significantly younger (mean difference = 3·73 years), with a higher BMI (mean difference = 0·21 kg/m²). They were more likely to be female, students or employees, smokers, to live alone, but were less likely to have achieved higher levels of education, to be working as managerial staff or to be retired and were less physically active and had lower levels of income (online Supplementary Table S2). For participants for which the mPNNS-GS was calculated (n 77 211), it was lower among excluded participants.
Participant in the clinical sample had a healthier dietary pattern than non-participants: they were on average older, with a lower BMI, more educated, with higher levels of income, less likely smoker, more likely men, had a higher level of physical activity, were less likely to live alone and had higher dietary scores (PNNS-GS, mPNNS-GS and PNNS-GS2) (all P value <0·05, data not shown).
Sociodemographic and lifestyle characteristics across quintiles of PNNS-GS2 for both sexes are presented in Table 2. PNNS-GS2 was higher for women (mean 2·1, sd 3·1) than for men (mean −0·3, sd 3·6). Higher PNNS-GS2 was significantly associated with higher age, lower BMI, higher education, higher physical activity, lower rates of smoking and higher rates of living alone. Participants of lower quintiles were more likely to be employees, students or manual workers, and people of higher quintiles were more likely to be managerial staff or retired, which is consistent with the age difference between quintiles.
Table 2. Characteristics of the participants by quintile (Q) of the Programme National Nutrition Santé – guidelines score 2 (PNNS-GS2), NutriNet-Santé study, n 80 965
(Mean values and standard deviations; percentages)
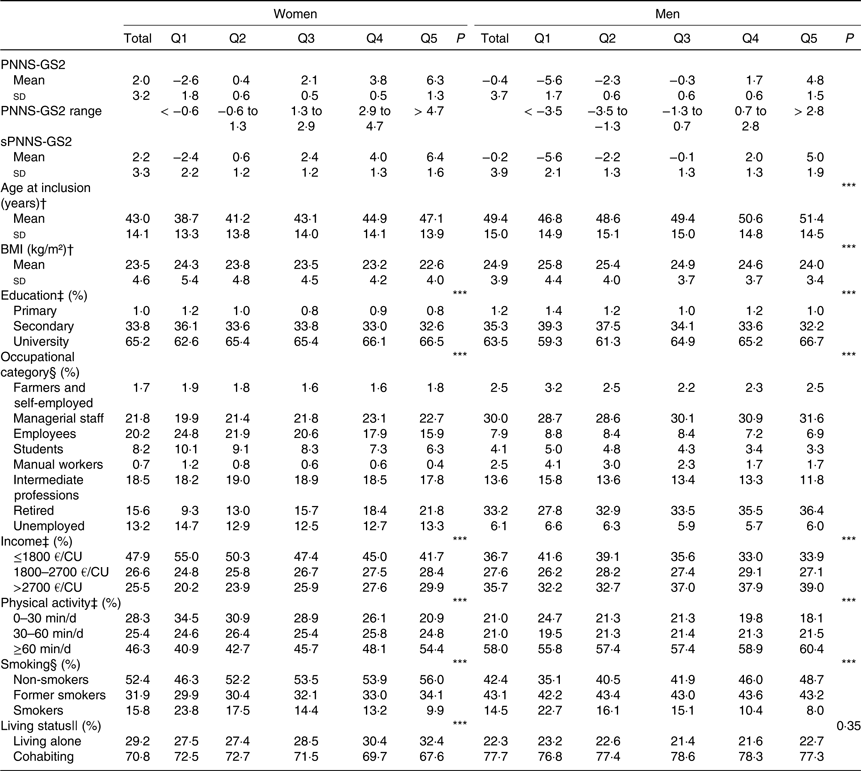
sPNNS-GS2, simplified PNNS-GS2; CU, consumption unit.
*** P≤0·0001.
† Linear contrast trend test.
‡ Spearman correlation test for ordinal variables.
§ Pearson χ 2 association test.
‖ Cochran–Armitage trend test.
As expected, the percentage of subjects reaching each recommendation component of the score was significantly associated with a higher quintile of PNNS-GS2 for both men and women but disparities in magnitudes were observed (Table 3). The highest absolute differences between Q5 and Q1 were observed for processed meat and fruits and vegetables and the highest relative differences for organic foods, salt and nuts. The lowest absolute differences were observed for added fat and legumes, and the lowest relative differences were observed for added fat and recommended oil ratio.
As for nutrients, higher PNNS-GS2 was significantly associated, in both men and women, with a higher intake of total carbohydrates and sugars, plant-based proteins, all PUFA whether in total or individually, starch, fibres, total Fe, K, Ca, Mg, Se, β-carotene, thiamine (vitamin B1), nicotinamide (vitamin B3), pantothenic acid (vitamin B5), pyridoxine (vitamin B6), vitamins C, D, and E and folic acid, and with a lower intake of animal protein, total lipids, SFA, Na, haeme Fe, cholesterol, retinol, vitamin B12 and Zn (Table 4). The relationship with MUFA was not linear. Proteins were positively associated with the score in women and negatively in men. Opposite associations were observed for plant proteins (increase across the quintiles) v. animal proteins (decrease across the quintiles). Riboflavin (vitamin B2) was positively associated with the score in women, but this association was not significant in men. Nevertheless, compared with the French recommended intakes of nutrients(37), nutrients negatively associated with the score were (on average) still consumed enough to stay in line with recommended limits in the highest adherents to the PNNS-GS2 (online Supplementary Table S3). In addition, food groups’ consumption across quintiles is presented in online Supplementary Table S4.
In the clinical sample, a higher quintile of PNNS-GS2 was significantly associated, for both men and women, with higher HDL-cholesterol concentration and lower blood glucose, TAG, total cholesterol and LDL-cholesterol concentrations and blood pressure (Table 5). Though significant, the observed variations were of rather low amplitude, with a difference of around 5 % between Q5 and Q1 (relative to total), except for TAG which had a 14 % difference.
Weighted Cohen’s κ coefficients were 0·58 between quintiles of PNNS-GS2 and quintiles of mPNNS-GS (i.e. without physical activity) and 0·54 between quintiles of PNNS-GS2 and quintiles of PNNS-GS (i.e. including physical activity). Those results can be extended to the sPNNS-GS2, as it is highly correlated with PNNS-GS2, with a correlation coefficient of 0·95 (online Supplementary Table S5). Cronbach’s α coefficients were 0·42 for PNNS-GS2 and 0·34 for sPNNS-GS2 (data not tabulated).
Table 3. Percentage of subjects reaching recommendations component† by quintile (Q) of the Programme National Nutrition Santé – guidelines score 2 (PNNS-GS2), NutriNet-Santé study, 2009, n 80 965
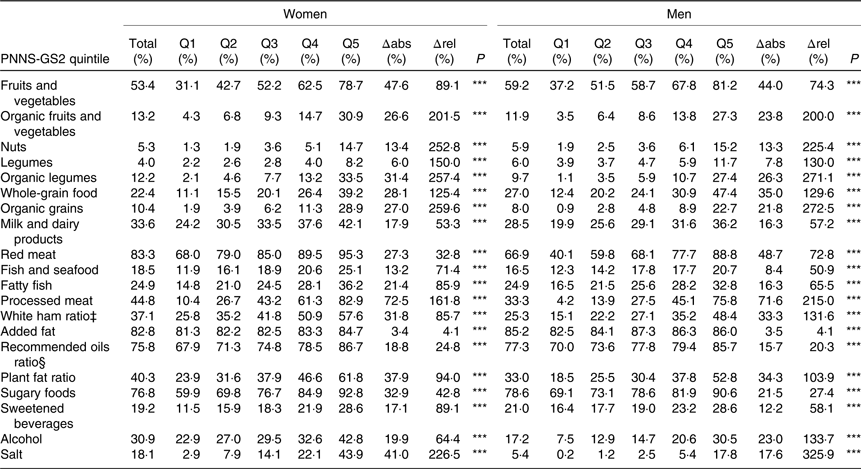
Δabs, Absolute difference between Q1 and Q5; Δrel, relative difference between Q1 and Q5, regarding total.
*** P≤0·0001 (Cochran–Armitage trend test over Q1, Q2, Q3, Q4 and Q5).
† A participant is reaching a component if he or she earns 1 point or more on an adequacy component, and 0 on a moderation component.
‡ White ham ratio percentage is only computed for people who eat more than 150 g/d of processed meat (n 59 330).
§ Recommended oils are α-linolenic acid-rich oils and olive oil.
Table 4. Nutrient intakes by quintile (Q) of the Programme National Nutrition Santé – guidelines score 2 (PNNS-GS2), NutriNet-Santé study, 2009, n 80 965†
(Mean values and standard deviations)

n-3 ALA, α-linolenic acid; n-6 ALA, α-linoleic acid; DPA, docosapentaenoic acid.
* P≤0·05, *** P≤0·0001 (linear contrasts trend test).
† Percentage values are calculated as the percentage of total daily energy intake without alcohol. All other values are calculated by the residual method.
‡ To convert kcal to kJ, multiply by 4·184.
Table 5. Nutritional biomarkers concentration by quintile (Q) of the Programme National Nutrition Santé – guidelines score 2 (PNNS-GS2), NutriNet-Santé study†
(Geometric means and 95 % confidence intervals)

Δrel, Relative difference between Q1 and Q5, regarding total; BP, blood pressure.
*P≤0·05, *** P≤0·0001 (linear contrasts trend test on logarithms of values).
† Values are adjusted on alcohol intake, energy intake without alcohol and age at inclusion.
‡ Effectives are given as n women/men.
Discussion
In the present study, the PNNS-GS2, which was built to reflect the overall adherence to the 2017 French dietary guidelines, was significantly associated with sociodemographic variables in the expected direction. Results from the percentage of participants reaching each of the recommendations showed that each component was discriminant and added to the overall score, but with disparities in terms of contribution. The associations with nutrient intakes showed that an increase in the PNNS-GS2 was associated with an overall healthier dietary profile. Clinical and biological indicators were also all associated to the score in the expected direction.
Associations between sociodemographic variables and dietary scores are not always consistent in the scientific literature. This can be explained by the broad heterogeneity of studies, in terms of methodology, sample size and population selection. However, positive association of PNNS-GS2 with higher age, lower energy intake without alcohol and lower smoking habit was consistent with previous studies developing dietary scores reflecting nutritional guidelines(Reference Estaquio, Kesse-Guyot and Deschamps6, Reference Toft, Kristoffersen and Lau38–Reference Verger, Mariotti and Holmes41). In these studies, association of diet quality with BMI, physical activity and education is overall similar to the present study.
Likewise, associations with nutrient intakes were overall similar to those reported in these studies(Reference Estaquio, Kesse-Guyot and Deschamps6, Reference Toft, Kristoffersen and Lau38–Reference Verger, Mariotti and Holmes41), with higher adherence to nutritional guidelines associated with more favourable intakes in micronutrients. However, the variations across quintiles of score were somewhat higher in the PNNS-GS2 for several nutrients (for example n-3 PUFA, haeme Fe, β-carotene, fibres and vitamin C) which are some of the main compounds implied in the nutritional prevention of health(Reference Deschasaux, Zelek and Pouchieu42–Reference Gamage, Dissabandara and Lam45).
MUFA intakes decreased overall across quantiles of the PNNS-GS2 but increased in Q5. This could be explained at least partly by a high consumption of MUFA-rich oils (i.e. olive oil) by Q5 participants (data not shown), along with a specifically low energy intake which influenced the residual value. Likewise, PNNS-GS2 was negatively associated with intakes of Zn, haeme Fe, vitamin B12, cholesterol and SFA, which is consistent with the decrease in meat consumption as the score increases.
Some components had a very low percentage of subjects reaching them, whatever the overall level of adherence to guidelines (nut, legumes and salt in men). These food groups should therefore be a main target for public health initiatives, in order to increase population compliance with the recommendations. Some other components had a large difference between Q1 and Q5, whether in absolute value (processed meat, fruits and vegetables) or in relative value (salt, nuts, organic food). This would suggest that public health initiative may be more effective in specific segments of the population.
The decrease in concentrations of serum TAG, LDL-cholesterol and systolic and diastolic blood pressure and the increase in HDL-cholesterol concentrations with higher diet quality have already been reported in previous studies(Reference Toft, Kristoffersen and Lau38–Reference Zamora, Gordon-Larsen and He40). The fact that blood pressure was related to overall nutritional quality of diet, beyond salt intake, was consistent with other dietary strategies aiming at reducing hypertension(Reference Appel46). Despite the fact that these associations were significant, the rather low magnitude of variation argues for limited interpretation in terms of clinical effect. In addition, the cross-sectional design limits the interpretation in terms of causality which could be further studied in a prospective design.
Convergent construct validity was satisfying as associations with age, smoking habit, sex, energy intake and clinical and biological variables were globally similar to the literature(Reference Estaquio, Kesse-Guyot and Deschamps6, Reference Toft, Kristoffersen and Lau38–Reference Verger, Mariotti and Holmes41). Weighted Cohen’s κ and Pearson’s correlation coefficients between PNNS-GS2 and mPNNS-GS gave expected criterion validity with medium values, although there is no consensual cut-off that would allow reaching a definite conclusion. Cronbach’s α coefficients revealed a low internal consistency, which was highly expected as components measure very different aspects and since Cronbach’s α does not consider weightings.
Nevertheless, some limitations of the present study should be reported. The major limitation is the self-recruiting bias in the population. Indeed, participants of the NutriNet-Santé study were volunteers in a nutrition-related cohort and were thus more interested in nutritional issues and healthy lifestyles than the general population. Thus, any generalisation of our findings should be undertaken with caution. Another selection bias pertains to the availability of score components, as excluded and included participants had different sociodemographic characteristics. Caution in interpreting findings is also advised, as our very large sample tends to yield highly significant test values although magnitude may be too low to attest for a real clinical difference.
The present study’s results should also be interpreted in the light of the inherent limitations to dietary index development. As reviewed by Waijers(Reference Waijers, Feskens and Ocké12), somewhat arbitrary decisions are involved in scores’ construction. This cannot be avoided in a guideline-based dietary score development, and components, cut-offs, weights and food groups’ assignment were indeed sometimes subjective. Values were, however, collegially decided by a panel of nutrition and public health experts who participated in the 2017 dietary guidelines revision, and based on the scientific literature, which mitigates this issue.
On the other hand, our study exhibits important strengths. First, it is based on nutritional data whose quality has been validated(Reference Lassale, Castetbon and Laporte26, Reference Kesse-Guyot, Assmann and Andreeva47). Moreover, the web-based format of the questionnaire might lead to higher data consistency and lower social desirability bias(Reference Kesse-Guyot, Assmann and Andreeva47). The large sample of the NutriNet-Santé allows access to a wide range of dietary behaviours. To the best of our knowledge, the present study is the first that integrates exposure to contaminants (with the proxy of organic food consumption) in dietary score computation allowing to assess all components of the 2017 recommendations.
Further studies should deepen construct validity by confronting the PNNS-GS2 to health conditions, such as obesity, CVD and cancer in the French population. In such studies, comparing the predictive value of PNNS-GS2 over PNNS-GS could very be useful to demonstrate the added value of the new recommendations.
Acknowledgements
We warmly thank all the scientists, dietitians, technicians and assistants for their technical contribution to the NutriNet-Santé study. We especially thank Cédric Agaesse, Vristi Desan and Cynthia Perlin (dietitians); Thi Hong Van Duong, Younes Esseddik (IT manager), Paul Flanzy, Régis Gatibelza, Jagatjit Mohinder and Aladi Timera (computer scientists); Julien Allegre, Nathalie Arnault, Laurent Bourhis and Fabien Szabo de Edelenyi, PhD (supervisor) (data-manager/statisticians) for their technical contribution to the NutriNet-Santé study and Nathalie Druesne-Pecollo, PhD (operational manager).
The NutriNet-Santé Study is supported by the French Ministry of Health (DGS), the French Public Health Agency, the French National Institute for Health and Medical Research (INSERM), the Medical Research Foundation (FRM), the French National Institute for Agricultural Research (INRA), the National Conservatory for Arts and Crafts (CNAM), the National Institute for Prevention and Health Education (INPES) and the Paris 13 University. MA was supported by a doctoral fellowship from the Ecole Doctorale Galilée, Paris 13 University, Sorbonne Paris Cité. Researchers were independent from funders. Funders had no role in the study design; the collection, analysis, and interpretation of data; the writing of the report; and the decision to submit the article for publication.
E. K.-G., S. H., C. J., V. D. and M. T. were responsible for the development of the design and protocol of the study; D. C. performed the statistical analysis and wrote the paper; E. K.-G. provided methodological guidance; D. C., M. A., V. D., M. T., S. H., C. J. and E. K.-G. were involved in developing the score, interpreting the results and editing the manuscript for important intellectual content. All authors read and approved the final manuscript.
None of the authors declares any conflicts of interest.
Supplementary material
To view supplementary material for this article, please visit https://doi.org/10.1017/S0007114519001181