Introduction
Spoken language unfolds incrementally and at a fast pace. Children and adults typically have little difficulty accurately recognizing words in running speech (e.g., Allopenna, Magnuson, & Tanenhaus, Reference Allopenna, Magnuson and Tanenhaus1998; Swingley, Pinto, & Fernald, Reference Swingley, Pinto and Fernald1999). One of the mechanisms responsible for this is prediction. Behavioural and neuropsychological studies have shown that both children (e.g., Borovsky, Elman, & Fernald, Reference Borovsky, Elman and Fernald2012; Friedrich & Friederici, Reference Friedrich and Friederici2005; Mani & Huettig, Reference Mani and Huettig2012; Nation, Marshall, & Altmann, Reference Nation, Marshall and Altmann2003) and adults anticipate upcoming input during language comprehension (e.g., Altmann & Kamide, Reference Altmann and Kamide1999; van Berkum, Brown, Zwitserlood, Kooijman, & Hagoort, Reference Van Berkum, Brown, Zwitserlood, Kooijman and Hagoort2005). However, less is known about whether cross-linguistic differences affect predictive processing. The aim of the current study is to address this gap in the literature by looking at two languages that differ in word order (Dutch vs. Turkish) and thus vary in how words come together to create sentence meaning.
A frequently used technique to examine semantic prediction during language processing is eye-tracking (Cooper, Reference Cooper1974; Tanenhaus, Spivey-Knowlton, Eberhard, & Sedivy, Reference Tanenhaus, Spivey-Knowlton, Eberhard and Sedivy1995). Using the Visual World Paradigm (VWP), participants’ eye-movements to pictures on a visual display are recorded while they listen to sentences. Previous work has shown that adults and children look at the target object before the target word is uttered entirely (e.g., Allopenna et al., Reference Allopenna, Magnuson and Tanenhaus1998; Swingley et al., Reference Swingley, Pinto and Fernald1999) or even before the target word is produced (e.g., Altmann & Kamide, Reference Altmann and Kamide1999; Fernald, Zangl, Portillo, & Marchman, Reference Fernald, Zangl, Portillo, Marchman, Sekerina, Fernandez and Clahsen2008; Mani & Huettig, Reference Mani and Huettig2012). Altmann and Kamide were the first to show that adults predict upcoming information during sentence comprehension. They presented semantically constraining sentences such as The boy will eat the big cake and neutral sentences such as The boy will move the big cake to participants, while they were viewing a display with objects (a boy, a cake, and some distractors). Their findings revealed that adults fixated the cake during the verb eat (vs. see) and prior to hearing the target word cake. Fernald et al. (Reference Fernald, Zangl, Portillo, Marchman, Sekerina, Fernandez and Clahsen2008) replicated this work by presenting similar types of sentences and two pictures on the screen to two-year-old children. The two-year-olds also looked at the target before it was heard in semantically constraining sentences. These studies demonstrate that both adults and children can use the semantic meanings of verbs to correctly predict an upcoming noun before it is heard.
Most of the studies mentioned above investigated languages in which the subject typically occurs prior to the verb while the object typically appears after it (Dryer, Reference Dryer, Dryer and Haspelmath2011). In such Subject–Verb–Object (SVO) languages, it is therefore not surprising that both adults and children effectively make use of verb information to predict upcoming referents. Users of SVO languages like English are used to having strict word order constraints. Therefore, the semantics of the verb and the order of the nouns demonstrate the role that each noun plays in a sentence. For example, in example (1) an English speaker would know that the boy is the agent of the verb eat and that what will follow is the patient (i.e., something edible).
(1) The boy eats the …
While about 35% of the languages in the world have SVO word order, around 42% have a canonical word order in which the verb follows its object (Dryer, Reference Dryer, Dryer and Haspelmath2011). These Subject–Object–Verb (SOV) languages are often characterized by flexible word order and the use of case to identify the role of each noun in a sentence. An example of such a language is Turkish. Although this language has a flexible word order, the most typical order is SOV (Erguvanlı, Reference Erguvanlı1984). This pattern was also found in a corpus of Turkish child-directed speech (Slobin & Bever, Reference Slobin and Bever1982). In this corpus, five different words orders were detected but the SOV order was the dominant one accounting for about 48% of the speech data. Example (2) illustrates this predominant word order in Turkish.
(2) Çocuk keki yiyor
boyNOM cakeACC eats
‘The boy eats the cake’
Dutch is also considered an SOV language, but combined with verb-second (V2) word order in main clauses (Zwart, Reference Zwart2011). V2 word order is a specific constraint on the position of the finite verb within a sentence (see (3)). Non-finite verbs and compound verbs, however, follow an SOV pattern in embedded clauses (see (4)). In this case, the finite verb (have) has been moved to second position and the non-finite verb (eat) remains in final position.
(3) De jongen eet de tart
The boy eats the cake
(4) De jongen heeft de taart gegeten
The boy has the cake eaten
‘The boy has eaten the cake’
Children's development of word order has mostly been studied using off-line measurements (e.g., Akhtar & Tomasello, Reference Akhtar and Tomasello1997; Gertner, Fisher, & Eisengart, Reference Gertner, Fisher and Eisengart2006). In such studies, children listen to sentences with different word orders (canonical and non-canonical), and they subsequently need to perform an action (e.g., acting out the sentence or pointing to the picture that matches the sentence). This type of research has shown that children around the age of three years have acquired the canonical word order of their language (e.g., Candan, Küntay, Yeh, Cheung, Wagner, & Naigles, Reference Candan, Küntay, Yeh, Cheung, Wagner and Naigles2012; Slobin & Bever, Reference Slobin and Bever1982; see Küntay & Özge, Reference Küntay, Özge, Arnon, Casillas, Kurumada and Estigarribia2014, for a review).
Only a few studies have looked at whether users of languages with flexible word order are able to use morphosyntactic information to predict upcoming elements in a sentence (on adults: Kamide, Altmann, & Haywood, Reference Kamide, Altmann and Haywood2003a; Kamide, Scheepers, & Altmann, Reference Kamide, Scheepers and Altmann2003b; on children: Özge, Küntay, & Snedeker, Reference Özge, Küntay and Snedekerin press; Özge, Marinis, & Zeyrek, Reference Özge, Marinis, Zeyrek and Özge2013). For example, Kamide et al. (Reference Kamide, Altmann and Haywood2003a) showed that Japanese adult speakers, whose canonical word order is SOV, used case marking to determine the next, upcoming referent.
Similarly, Özge et al. (Reference Özge, Küntay and Snedekerin press) conducted two visual world paradigm experiments with four- and five-year-old Turkish children and adults. In their Experiment 1, they investigated whether Turkish participants were able to use the contrast between the nominative and the accusative case on the first argument to predict the thematic role of the second argument before it became available in verb-medial structures (i.e., SVO vs. OVS). In their Experiment 2, they used verb-final structures (i.e., SOV vs. OSV) to further test whether Turkish children could anticipate the second argument prior to the verb information. Their results showed that children, like adults, could use case marking on a noun to predict an upcoming referent in both verb-medial (Experiment 1) and verb-final (Experiment 2) structures. Moreover, Özge et al. (Reference Özge, Marinis, Zeyrek and Özge2013) investigated how adults processed word order variation online in a self-paced listening paradigm. Their goal was to examine whether object-initial sentences would pose any processing difficulty in verb-medial orders (i.e., OVS and SVO). Their results showed that a sentence-initial object NP did not pose extra processing costs compared to a sentence-initial subject NP. Instead, they showed that sentence-initial objects (OVS) were even processed faster than sentence-initial subjects (SVO). Processing of the verb in both sentence structures did not reveal any significant differences. And finally, the object following the SV string was processed faster than the subject following the OV string. The authors argued that the speed of this integration process depended on the frequency (i.e., subject drop occurs frequently in Turkish) and reliability (i.e., accusative case) of the linguistic structure.
In sum, the studies above demonstrated that speakers of languages like Turkish are adept at incremental interpretation of language based on reliable cues such as case (which cannot be purely formal, but in fact does map fairly transparently onto thematic roles). However, to our knowledge, it is unknown whether Turkish children and adults use the semantics of a verb as a cue to sentence meaning, as they rarely need to (at least in comparison to speakers of languages like Dutch and English). The current study will therefore investigate how meaning is assigned incrementally during the interpretation of Turkish, a language with flexible word order. The current study examines whether Dutch and Turkish adults and children are able to use the semantics of the verb to predict the upcoming noun. We presented our participants with a similar visual world eye-tracking task to that of Mani and Huettig (Reference Mani and Huettig2012). Participants saw a visual display containing two familiar objects (e.g., a cake and a tree) and, depending on their native language, they heard a semantically constraining sentence such as De jongen eet de grote taart or Çocuk yiyor bu büyük keki ‘The boy eats the big cake’, or a neutral sentence such as De jongen ziet de grote taart or Çocuk görüyor bu büyük keki ‘The boy sees the big cake’, while we tracked their eye-movements across the visual display.
Note that the auditory sentences contained only finite verbs. This restricted the Dutch sentences to (the fixed) SVO word order. We predict that the Dutch participants, both adults and children, will show a clear semantic prediction effect (i.e., more looks to the target cake in the semantically constraining than in the neutral condition) over time, replicating previous work (e.g., Altmann & Kamide, Reference Altmann and Kamide1999; Mani & Huettig, Reference Mani and Huettig2012). Within the Dutch participants, we expect that this prediction effect will become stronger with age due to increased familiarity with the word order structure. That is, we predict that adults will show a larger effect over time (i.e., as the sentence unfolds) than the five-year-old children who, in turn, will show a larger effect over time than the four-year-olds.
For the Turkish participants, the sentences were also always presented in SVO word order. This structure is less frequently used in Turkish, as the typical, most frequent word order is SOV, and the verb thus usually arrives late in this language. We therefore predict different patterns for the Turkish than the Dutch participants. We expect that Turkish adults will show a prediction effect over time (i.e., as the sentence unfolds), as they have encountered the SVO structure throughout their lives and they know that this is an acceptable, though less frequent, structure. We also expect that frequency and reliability will play a role in prediction because they have been found to facilitate the processing of sentence-initial and (specifically) sentence-final objects, as opposed to subjects (Özge et al., Reference Özge, Marinis, Zeyrek and Özge2013). It is therefore possible that Turkish children will make less or no use of verb-based prediction, as they have less experience with the SVO structure, compared to Turkish adults. We thus predict an asymmetry between Turkish adults and children in terms of their semantic prediction skills.
Method
Participants
All participants were native speakers of Dutch or Turkish. Three age groups (four-year-olds, five-year olds, and adults) took part in this study, resulting in six groups. Table 1 illustrates an overview of the characteristics of each group. All participants were without any hearing problems and had normal, or corrected-to-normal, vision. Adults were paid for their participation. For the children, parents gave informed consent, and children were compensated with a toy.
Table 1. Characteristics of the Participant Groups

Material
Dutch and Turkish stimuli were created for the Dutch and Turkish experiment, respectively (see also Brouwer, Özkan, & Küntay, Reference Brouwer, Özkan, Küntay, Blom, Cornips and Schaeffer2017, for the Dutch stimuli). Each experiment consisted of 24 displays with two coloured line-drawings. These visual displays were paired either with a semantically constraining sentence as in (5) (semantic condition) or with a neutral sentence as in (6) (neutral condition). The two objects corresponded to the target (e.g., taart, kek ‘cake’) or a distractor (e.g., boom, ağaç ‘tree’).
(5) De jongen eet de grote taart [semantic condition]
Çocuk yiyor bu büyük keki
‘The boy eats the big cake’
(6) De jongen ziet de grote taart [neutral condition]
Çocuk görüyor bu büyük keki
‘The boy sees the big cake’
Female native adult speakers of Dutch and Turkish recorded the test sentences (16 bits, 44100 Hz). For both languages each sentence consisted of a noun phrase, a verb, and a determiner–adjective–noun combination. The lengths of the different constituents in each sentence were measured in PRAAT (Boersma & Weenink, Reference Boersma and Weenink2015). Both the Dutch and Turkish auditory stimuli were then minimally manipulated in PRAAT to adjust the duration of the region from verb onset until noun onset to 2400 ms. These stimuli were normalized to the same average level of 65 dB. The Dutch and Turkish sentences resembled each other as closely as possible, but in some cases a direct translation was not possible, largely due to a mismatch in the number of syllables between the translation pairs in the two languages. For instance, the adjective rode ‘red’ is a disyllabic word in Dutch, whereas its Turkish translation kırmızı contains three syllables. Therefore, a different adjective beyaz ‘white’ was used for the Turkish correspondent of the stimulus. In other cases, the translation pairs differed in number of words. This was primarily problematic for some verbs. Similarly to the adjectives, a different verb is preferred for cases where the Turkish translation corresponded to a noun–verb compound (e.g., yardım etmek ‘to (do) help’ was replaced with bakmak ‘to look’) (see ‘Appendix’). The words used in the sentences were highly familiar to four- and five-year-olds (as based on a Dutch wordlist for four- to six-year-olds by Damhuis, de Glopper, Broers, & Kienstra, Reference Damhuis, de Glopper, Boers and Kienstra1992). The target–distractor pairs never overlapped phonologically at onset and were equal in frequency.
Eight filler items were created for motivational purposes. They encouraged the children during the task (e.g., “you are doing a great job!”). Four different lists were created in which target position (left vs. right) and sentence (semantic vs. neutral) were counterbalanced. In each list the semantic, neutral, and filler trials were presented pseudo-randomly.
Norming task
Prior to the study, we conducted a norming study to evaluate whether our verbs predictively elicit the nouns to be tested. Twenty-four Dutch and 24 Turkish adults were asked to judge which would be a likely target when given the sentence beginning of their native language (e.g., “The boy eats the nice …”). Participants had to choose between two alternatives, which were the target and distractor presented in the eye-tracking experiments (e.g., ‘cake’ and ‘book’). Performance on the semantic sentences was very high (99% correct for Dutch adults; 94% correct for Turkish adults), showing that our sentences effectively created semantically predictable contexts.
Procedure
For the Dutch children and adults the experimental session took place in a lab at Utrecht University. For some Dutch children the session took place at a Dutch primary school. For the Turkish children and adults the session took place in a lab at Koç University, Istanbul. For some Turkish children the session took place at a preschool. The Dutch children and adults sat in front of the screen of a Tobii T60 and the Turkish children and adults performed the eye-tracking task on a Tobii T120. However, we fixed the sampling rates to be the same across language groups. That is, we sampled the data at 60 Hz for both Dutch and Turkish participants. Stimuli were presented using E-Prime software (Psychology Software Tools, 2016). Before the start of the experiment, a 9-point calibration procedure was performed. Before each trial, children were shown a fixation cross in the middle of the screen, which they were instructed to fixate on before every trial (drift correction). Children were told that they would be seeing two pictures on the screen while hearing sentences. Their task was to listen carefully to these sentences, and to look at the pictures. The two pictures were shown 2000 ms before sentence onset and they remained on screen until sentence offset. The session lasted about 15 minutes.
Results
Figure 1 shows the proportion fixations to targets from verb onset for Dutch and Turkish adults and children (four- and five-year-olds). Numerically, all groups looked more at targets in the semantic than in the neutral condition. This pattern started during the unfolding of the verb. However, it is uncertain whether this numerical pattern holds statistically.

Figure 1. Timecourse of fixating to targets with SE bars in the semantic (The boy eats the big cake) and the neutral condition (The boy sees the big cake) for Dutch and Turkish adults, and four-year-olds and five-year-olds.
To assess whether the two curves that represent proportion fixations to targets in the semantic and the neutral condition differ statistically from each other over time across the different age groups, we used Generalized Additive Mixed Modeling (GAMM; Wood, Reference Wood2006). GAMM is an extension of a generalized linear regression model in which non-linear terms (i.e., smooths) can be included in addition to linear relationships between predictors and the dependent variable. The smooth function is a weighted sum of a set of base functions that each has a different shape, resulting in a non-linear curve. GAMM is particularly suited for analyzing timecourse data (Nixon, van Rij, Mok, Baayen, & Chen, Reference Nixon, van Rij, Mok, Baayen and Chen2016; van Rij, Hollebrandse, & Hendriks, Reference Rij, Hollebrandse, Hendriks, Holler and Suckow2016), as it is able to (1) determine linearity or non-linearity on the basis of the data, (2) analyze continuous variables (e.g., time) and non-linear interactions, (3) include random effects, and (4) deal with autocorrelation.Footnote 1 The analyses were conducted in R (version 3.2.2; R Core Team, 2018) using the package mgcv 1.8.20 (Wood, Reference Wood2009). The results were plotted using the itsadug 2.3 R package (van Rij, Wieling, Baayen, & van Rijn, Reference Rij, Wieling, Baayen and Rijn2015). For reproducibility, the data and commands used for the analysis are available in the Supplementary materials: S1 File (Dutch data), S2 File (Turkish data), and S3 File (analysis script) (available at <https://doi.org/10.1017/S0305000918000375).
A logistic GAMM was fitted (using the binomial family), as our dependent variable was binary (target fixation: 1, distractor fixation: 0). As Wieling (Reference Wieling2012, p. 129) points out: “Logistic regression does not model the dependent variable directly, but it attempts to model the probability (in terms of logits) associated with the values of the dependent variable. A logit is the natural logarithm of the odds of observing a certain value (in our case, a target fixation).” In the model summary, the parameter estimates will therefore correspond to the likelihood of target fixation (expressed as the log of odd ratios).
To identify whether our predictors Condition (semantic vs. neutral) and Age (adults vs. four-year-olds vs. five-year-olds) were significant, we included smooths modeling the difference between two original smooths (cf. Wieling, Reference Wieling2018). For example, we measured the difference between the original smooth for the semantic condition and the original smooth for the neutral condition. If such a difference smooth is significant, this indicates that the additional complexity of distinguishing the levels of our predictors is required. To fit such a model, we created binary (i.e., dummy) variables which are equal to 0 for one level of the categorical variable and 1 for the other level. For example, Condition was converted into the binary variable IsSemantic (semantic condition: 1, neutral condition: 0), to explicitly test for the non-linear difference between the two conditions (i.e., a semantic prediction effect). As we were interested in the timecourse of processing, a smooth for the predictor Time was also included (30 ms time bins). The time-window from verb onset until noun onset was selected for analysis. Each binary variable was included as a non-linear interaction with Time, to test whether the levels of the predictors showed variations in gaze pattern over time. In addition, non-linear random effects over Time for participants and items were included.
Note that the analyses can only be conducted within each language separately, as there are timing differences in the length of the constituents in our sentences across languages. For example, the offset of the verb occurs 260 ms earlier in our Dutch sentences than in our Turkish sentences. We will therefore present the Dutch and Turkish data separately.
Dutch eye-gaze data
Table 2 summarizes the results of the best model fit. The estimates of the parametric coefficients show that the four- and five-year-olds numerically give rise to lower target fixations compared to adults (intercept). However, the parametric part is less informative, as it does not distinguish between our conditions or time values. The deviance explained by the model is 10.1%.
Table 2. Summary of the Dutch Results of the GAM Model

Notes. Since Condition was converted into a binary variable, ‘Semantic’ models the difference curve. A positive estimate indicates that this predictor increases target fixations, while a negative estimate indicates the opposite effect. The explained deviance of this model is 10.1%.
The smooth terms show the significance of the non-linear patterns associated with Age over Time. They directly indicate significance of the difference between target fixations in the semantic versus the neutral condition. The binary smooth [s(Time):IsSemantic] reveals that the non-linear pattern for the difference between the two conditions over time is significant, indicating that our Dutch participants demonstrate a semantic prediction effect over time. Moreover, the significant binary smooth [s(Time):IsAdultSemantic] shows that the adults’ non-linear pattern for the difference between the semantic and the neutral condition contrasts with the four- and five-year-olds. This pattern is illustrated in Figure 2. The Dutch adults are represented by the solid curve and the Dutch children by the dotted curve. These data indicate that the adults show a stronger semantic prediction effect than the two child groups. Note, however, that the binary smooth [s(Time):IsSemantic] was significant, indicating that both child groups also benefitted from the semantic as opposed to the neutral condition in their predictive sentence processing.

Figure 2. Non-linear smooths (fitted values, excluding. random effects) for the Dutch adults (solid line) and the Dutch four- and five-year-olds (dotted line). The pointwise 95%-confidence intervals are shown by shaded bands.
Note that in an earlier model we also included a binary smooth to compare the four-year-olds to the five-year-olds (and the adults). However, that smooth did not reach significance and was therefore taken out of the model. Finally, non-linear random effects over time were significant for both participants and items.
Turkish eye-gaze data
Table 3 summarizes the results of the best model fit for the Turkish data. The estimates of the parametric coefficients show that adults (intercept) numerically give rise to slightly higher target fixations compared to the four- and five-year-olds. The deviance explained by the model is 11.3%.
Table 3. Summary of the Turkish Results of the GAM model

Notes. Since Condition was converted into a binary variable, ‘Semantic’ models the difference curve. A positive estimate indicates that this predictor increases target fixations, while a negative estimate indicates the opposite effect. The explained deviance of this model is 11.3%.
The smooth terms show the significance of the difference between target fixations in the semantic versus the neutral condition. The binary smooth [s(Time):IsSemantic] reveals that the non-linear pattern for the difference between the two conditions over time is not significant, indicating that the semantic prediction effect is not present across all our participants. More specifically, the significant binary smooth [s(Time):IsAdultSemantic] shows that the adults’ non-linear pattern for the difference between the semantic and the neutral condition contrasts with the four- and five-year-olds. This pattern is presented in Figure 3. The adults (solid curve) show a prediction effect, whereas the four- and five-year-olds do not show such an effect (dotted curve). Note that an earlier model showed no differences between the four-year-olds and the five-year-olds (and the adults). As this smooth was not significant, it was taken out of the model. Finally, non-linear random effects over time were significant for both participants and items.

Figure 3. Non-linear smooths (fitted values, excluding random effects) for the Turkish adults (solid line) and the Turkish four- and five-year-olds (dotted line). The pointwise 95%-confidence intervals are shown by shaded bands.
General discussion
The present study examined whether Dutch and Turkish children and adults, whose languages differ in word order, are able to extract information from the verb and use this information to predict an upcoming noun. In Dutch, the finite verb is always in second position, therefore sentences with finite verbs look like SVO. Turkish, however, has a flexible word order (based on pragmatic constraints), of which the most typical order is SOV. Turkish language users are therefore less familiar with the SVO structure used in this study than Dutch language users. To assess whether this difference in word order between the languages matters for their predictive behaviour, we conducted a visual-world eye-tracking experiment. We presented Dutch and Turkish four-year-olds, five-year-olds, and adults with two familiar objects on a visual display while they heard semantically constraining and neutral sentences in their native language (e.g., De jongen eet/ziet de grote taart or Çocuk yiyor/görüyor bu büyük keki ‘The boy eats/sees the big cake’). For each language group, we analyzed whether they showed a semantic prediction effect (i.e., difference between looks to targets in the semantic vs. the neutral condition) across different ages over time.
Our Dutch data demonstrated a semantic prediction effect over time for all our age groups (see also Brouwer et al., Reference Brouwer, Özkan, Küntay, Blom, Cornips and Schaeffer2017). This replicates previous work with children and adults with different native languages such as English (e.g., Altmann & Kamide, Reference Altmann and Kamide1999) and German (Mani & Huettig, Reference Mani and Huettig2012). Adults showed a stronger prediction effect than the four- and five-year-olds. This finding is in line with a usage-based approach (Bybee, Reference Bybee1985, Reference Bybee1995; Langacker, Reference Langacker and Rudzka-Ostyn1988; Tomasello, Reference Tomasello2000) in which experience plays a key role. Due to accumulated experience with the V2-rule, the adults may be able to create stronger links between verbs and their arguments than children, creating higher probabilities of pre-activating appropriate nouns on the basis of the verb. Alternatively, it may (also) be possible that this difference between adults and children has to do with automatization of language processing routines (e.g., faster lexical access and more efficient online sequencing and integration of linguistic material).
Prediction ability in the Dutch children's two age groups did not differ significantly from each other. This finding contradicted our expectation. We expected that the five-year-olds would show a larger effect than the four-year-olds. As Dutch four-year-olds already have fairly robust knowledge of SVO sentences with finite verbs (Jordens, Reference Jordens1990), it is perhaps not surprising that the five-year-olds did not do significantly better. It is possible that an older age group would show an increase compared to four-year-olds. This might reveal a developmental pattern. Alternatively, it would be interesting to manipulate the amount of experience under more controlled settings. For example, Roth (Reference Roth1984) provided children with additional experience and feedback on processing relative clauses (experimental group). This significantly improved children's comprehension rates compared to a group of controls who only received training in processing other structures. Roth argued that the experience with relative clauses led to improved processing efficiency. A similar experience-based process may be at work for linking verbs to arguments.
The results of the Turkish participants showed a semantic prediction effect for the adults. This demonstrates that Turkish adults, who had sufficient experience with the SVO word order throughout their lives, are able to use the verb to predict the upcoming noun. However, the semantic prediction effect was absent for the four- and five-year-olds. This indicates that they were not able to make use of the semantics of the verb to predict the noun in the sentence.
Note that, ideally, we would have preferred to analyze the data from our two language groups in one statistical model. However, it appeared to be impossible to create identical stimuli sets for the two languages (e.g., timing differences). A way around this would be to manipulate our stimuli to align onsets and durations of the critical constituents to reduce between-item differences. The drawback of such manipulations is that the resulting stimuli often sound less fluent and natural, thereby negatively influencing ecological validity. We would like to speculate, though, on an explanation for the numerical differences between the Dutch and the Turkish children's eye-gaze patterns (see Figure 1, especially the five-year-olds).
In Turkish, word order variations take place as a function of discourse pragmatics (Erguvanlı-Taylan, Reference Erguvanlı-Taylan1987). Sentence-initial position is reserved for the topic (i.e., the entity that the discourse is about), while the focus (i.e., the entity that introduces new or contrastive information) is placed in the immediately preverbal position. Postverbal position, on the other hand, is the site where backgrounded arguments are located (Erguvanlı-Taylan, Reference Erguvanlı-Taylan1979; Erkü Reference Erkü1983; Göksel & Özsoy, Reference Göksel and Özsoy2000, Reference Göksel and Özsoy2003; İşsever Reference İşsever2003). In Turkish, SVO therefore indicates a topicalized/focused subject and a backgrounded object. Corpus-based and experimental research on Turkish-speaking children's acquisition of word order indicates that they have early sensitivities to such pragmatically motivated word order variations (Batman-Ratyosyan & Stromswold, Reference Batman-Ratyosyan and Stromswold2002; Demir, So, Özyürek, & Goldin-Meadow, Reference Demir, So, Özyürek and Goldin-Meadow2012; Ekmekçi, Reference Ekmekçi1979, Reference Ekmekçi, Slobin and Zimmer1986; Küntay, Reference Küntay2002). These early sensitivities emerge in children's spontaneous productions as early as 19 months (Ekmekçi, Reference Ekmekçi, Slobin and Zimmer1986) and become stronger at ages four and five (Sağın-Şimşek, Reference Sağın-Şimşek, Haznedar and Ketrez2016). For instance, unlike three-year-olds, Turkish-speaking four- and five-year-olds did not perform above chance in a comprehension task of reversible actions in uninflected OVS frames, which was attributed to their knowledge that these structures are pragmatically infelicitous when presented as isolated sentences (Stromswold & Batman-Ratyosyan, Reference Stromswold and Batman-Ratyosyan1999). These studies indicate that Turkish-speaking children perceive word order as a discourse-pragmatic device and exhibit difficulties in comprehending non-canonical structures in the absence of appropriate discourse context.
Turkish-speaking children also perceive word order as a structural device for sentence interpretation. For instance, two-year-old Turkish-speaking children, like their English-speaking peers, could use the canonical word order of their language to identify participant roles in simple transitive sentences by looking longer to the correct video clips that were associated with the sentence they heard (Candan et al., Reference Candan, Küntay, Yeh, Cheung, Wagner and Naigles2012). However, Turkish-speaking children displayed more frequent switches of looks between the two scenes compared to their English-speaking peers, suggesting more uncertainty with their event choice, which was solely based on canonical word order. In fact, numerous studies indicate that Turkish-learning children consistently rely on case markers more than word order as a structural cue to sentence meaning and as a strategic device for online comprehension and prediction (e.g., Göksun, Küntay, & Naigles, Reference Göksun, Küntay and Naigles2008; Özge et al., Reference Özge, Marinis, Zeyrek and Özge2013; Özge et al., Reference Özge, Küntay and Snedekerin press; Slobin & Bever, Reference Slobin and Bever1982).
In sum, word order might not be as available as a cue for Turkish-speaking children compared to their Dutch-speaking peers to process the argument structure and to pre-activate appropriate nouns on the basis of the verb. For Dutch children, SVO word order (i.e., the V2-rule) signals that a direct object will follow a transitive verb; hence verb semantics can be used to anticipate this direct object. For Turkish children, SVO word order does not always guarantee that a direct object will appear following a transitive verb because Turkish also allows frequent noun ellipsis. For this reason, Turkish children may have failed to anticipate, or even avoided anticipating, the pragmatically backgrounded postverbal direct object, which could potentially be elided. It is thus conceivable that flexible and pragmatically motivated word order variation in the input of the Turkish as opposed to the Dutch children led to differences between the two child samples with respect to the semantic prediction effect. The different mechanisms for deriving SVO structures (i.e., Turkish: pragmatic rules; Dutch: structural rules) may have played a role here. The prediction effect observed for the Turkish adults, on the other hand, could be attributed to their much longer experience with such non-canonical constructions.
Recently, researchers have started looking at what makes people good versus bad predictors. For children, it has been found that receptive (Borovsky et al., Reference Borovsky, Elman and Fernald2012) and productive vocabulary size (Mani & Huettig, Reference Mani and Huettig2012) play an important role in semantic prediction. The current work adds to this discussion because our data have demonstrated that it is also important to take into account the linguistic factor of word order when investigating verb-based prediction. In particular, it is important to consider the frequency and the reliability with which a certain word order occurs in a language. This is consistent with previous work (Özge et al., Reference Özge, Marinis, Zeyrek and Özge2013; Özge et al., Reference Özge, Küntay and Snedekerin press). Further research needs to be done to see which other linguistic and/or cognitive factors might play a role in languages with different word order structures.
Taken together, our findings reveal that experience with word order structures and/or automatization of language processing routines plays an important role for semantic prediction. Differences in word order in typologically distinct languages create different distributional information for language learners. This idea has also been proposed for previous work on relative clause comprehension (Fitz, Chang, & Christiansen, Reference Fitz, Chang, Christiansen and Kidd2011; Wells, Christiansen, Race, Acheson, & MacDonald, Reference Wells, Christiansen, Race, Acheson and MacDonald2009). In line with those studies, it seems that the ability to semantically predict emerges gradually and as a function of exposure to the distributional and specific properties of a language. Future work should validate our explanations further by looking at other ages and other languages with different word order systems.
Supplementary Materials
For supplementary materials: S1 File (Dutch data), S2 File (Turkish data), and S3 File (analysis script), please visit <https://doi.org/10.1017/S0305000918000375).
Acknowledgements
We are very grateful to Louis ten Bosch and Martijn Wieling for their helpful statistical advice. We would also like to thank Sanne Alblas, Marloes Berkers, Angelina Corbet, Anouk van den Eijnden, Laurette Gerts, Maike van Grinsven, Liesbeth van Hoogdalem, Rajshrie Kalloe, Serpil Kilic, Rachel van Moorst, Cindy de Sousa Fortes, Fréderique Spigt, Vera Stoffers, Christel Vinke, and Angela van de Weg for their assistance with participant running. Finally, we thank the Prince Claus Chair for Development and Equity awarded to Aylin Küntay in 2012–2014 for her to be a visiting professor at Utrecht University, and the NWO/WOTRO funds (W 02.24.104.00) which made this collaboration possible.
Appendix
Sentences used in the Dutch and Turkish eye-tracking task. The two versions of each sentence are presented on the same line, with the semantically constraining sentence first. The distractor object of each sentence is within parentheses.
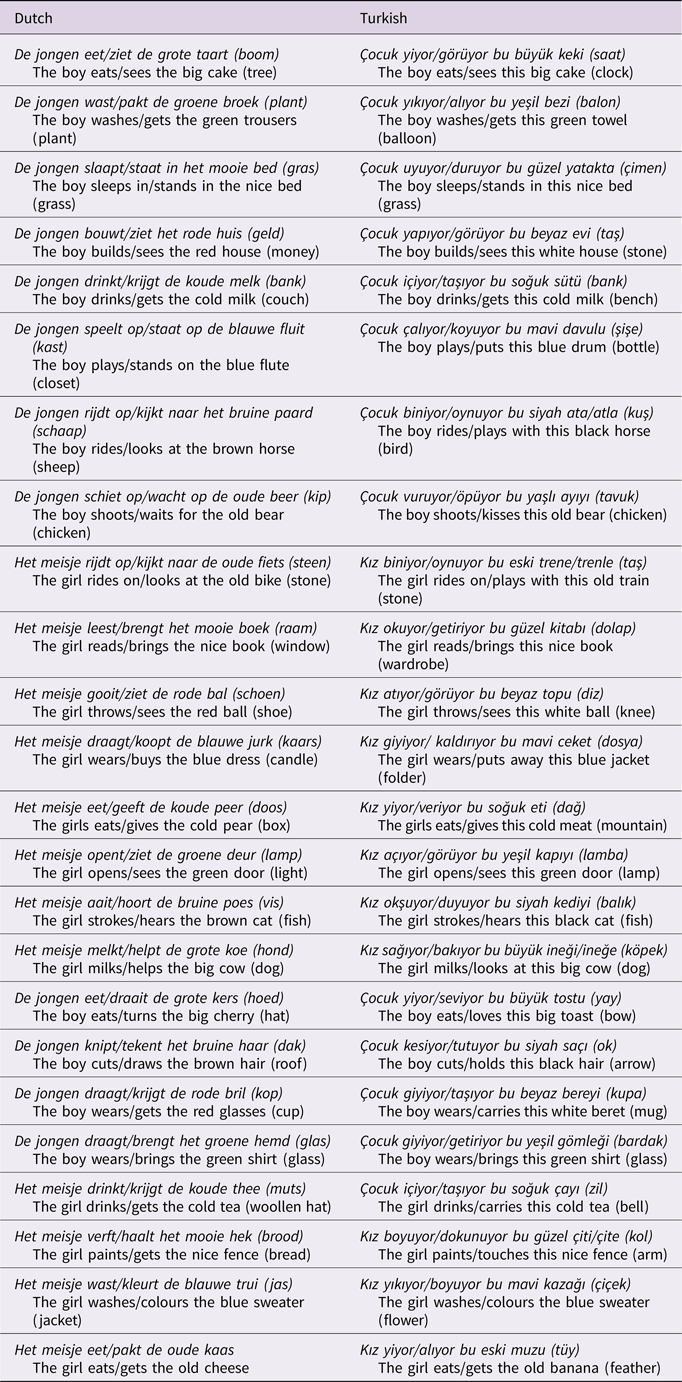