16 results
3 - Specific Kinds of Scientific Explanations
-
- Book:
- Understanding How Science Explains the World
- Published online:
- 17 June 2022
- Print publication:
- 07 July 2022, pp 29-42
-
- Chapter
- Export citation
8 - From Explanation to Knowledge
-
- Book:
- Understanding How Science Explains the World
- Published online:
- 17 June 2022
- Print publication:
- 07 July 2022, pp 86-101
-
- Chapter
- Export citation
6 - Explanatory Quality and Felt Understanding
-
- Book:
- Understanding How Science Explains the World
- Published online:
- 17 June 2022
- Print publication:
- 07 July 2022, pp 69-79
-
- Chapter
- Export citation
4 - Explanation and Prediction
-
- Book:
- Understanding How Science Explains the World
- Published online:
- 17 June 2022
- Print publication:
- 07 July 2022, pp 43-54
-
- Chapter
- Export citation
2 - The General Nature of Explanation
-
- Book:
- Understanding How Science Explains the World
- Published online:
- 17 June 2022
- Print publication:
- 07 July 2022, pp 13-28
-
- Chapter
- Export citation
1 - Why Explanation Matters in Science
-
- Book:
- Understanding How Science Explains the World
- Published online:
- 17 June 2022
- Print publication:
- 07 July 2022, pp 1-12
-
- Chapter
-
- You have access
- HTML
- Export citation
7 - False Theories, But Accurate Explanations?
-
- Book:
- Understanding How Science Explains the World
- Published online:
- 17 June 2022
- Print publication:
- 07 July 2022, pp 80-85
-
- Chapter
- Export citation
5 - Evaluating Explanations
-
- Book:
- Understanding How Science Explains the World
- Published online:
- 17 June 2022
- Print publication:
- 07 July 2022, pp 55-68
-
- Chapter
- Export citation
Chapter 5 - A Look to the Future
-
- Book:
- Helping People Learn
- Published online:
- 16 June 2022
- Print publication:
- 30 June 2022, pp 114-156
-
- Chapter
- Export citation
Chapter 2 - The Invention and Use of CmapTools Software in Schools, Corporations, and Other Organizations
-
- Book:
- Helping People Learn
- Published online:
- 16 June 2022
- Print publication:
- 30 June 2022, pp 42-72
-
- Chapter
- Export citation
Chapter 4 - The Design of Better Instructional Programs
-
- Book:
- Helping People Learn
- Published online:
- 16 June 2022
- Print publication:
- 30 June 2022, pp 98-113
-
- Chapter
- Export citation
Chapter 1 - Developing the Foundations to Help People Learn
-
- Book:
- Helping People Learn
- Published online:
- 16 June 2022
- Print publication:
- 30 June 2022, pp 1-41
-
- Chapter
- Export citation
Chapter 3 - Building a Theory of Education
-
- Book:
- Helping People Learn
- Published online:
- 16 June 2022
- Print publication:
- 30 June 2022, pp 73-97
-
- Chapter
- Export citation
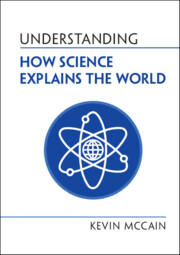
Understanding How Science Explains the World
-
- Published online:
- 17 June 2022
- Print publication:
- 07 July 2022
2 - Science and Truth
-
- Book:
- Measuring Behaviour
- Published online:
- 07 May 2021
- Print publication:
- 06 May 2021, pp 11-28
-
- Chapter
- Export citation
80 - Contemporary Japanese fiction
- from Part V - The modern period (1868 to present)
-
-
- Book:
- The Cambridge History of Japanese Literature
- Published online:
- 05 January 2016
- Print publication:
- 17 December 2015, pp 760-767
-
- Chapter
- Export citation