Introduction
After decades of persecution and extermination of large carnivores in Europe, populations have started to recover (Chapron et al., Reference Chapron, Kaczensky, Linnell, von Arx, Huber and Andrén2014). Management policy has improved considerably and large carnivores are protected by law in most European countries (Molinari-Jobin et al., Reference Molinari-Jobin, Marboutin, Wölfl, Wölfl, Molinari and Fasel2010). They are recolonizing their former ranges both naturally and through reintroduction (Linnell et al., Reference Linnell, Swenson and Andersen2001); however, they are confronted with a human-dominated landscape, where their habitats are diminished and fragmented as a result of direct destruction and the development of roads and railways (Fischer & Lindenmayer, Reference Fischer and Lindenmayer2007). With the reduction in habitat, wildlife populations become smaller and more isolated, both of which increase the risk of local extinction. Large carnivores are particularly vulnerable to local extinction in fragmented environments because they require large contiguous spaces, and their populations are low in density (Ripple et al., Reference Ripple, Estes, Beschta, Wilmers, Ritchie and Hebblewhite2014).
For the Eurasian lynx Lynx lynx, stagnation and declines of reintroduced and formerly increasing populations have been reported (e.g. the Vosges–Palatinian population in France; the Dinaric population in Slovenia, Croatia, Bosnia and Herzegovina; the Bohemian–Bavarian population along the Austrian–German–Czech border; Kaczensky et al., Reference Kaczensky, Chapron, von Arx, Huber, Andrén and Linnell2013). The latter population originated from lynx captured in the Carpathian Mountains and reintroduced to the Bohemian Forest in the 1970s and 1980s (Wölfl et al., Reference Wölfl, Bufka, Červený, Koubek, Heurich and Habel2001). The population resides in the Bohemian Forest Ecosystem, in which the Bavarian Forest National Park and the Šumava National Park are embedded. Initially data indicated an increase in numbers and distribution but at the end of the 1990s population increase stagnated, and in the Czech part of the Bohemian Forest the number of individuals decreased (Wölfl et al., Reference Wölfl, Bufka, Červený, Koubek, Heurich and Habel2001). The latest status report on the population also presumes stagnation in population size and range in Germany and Austria. It is estimated the Bohemian–Bavarian population comprises 47–67 individuals (Kaczensky et al., Reference Kaczensky, Chapron, von Arx, Huber, Andrén and Linnell2013).
The reason for the stagnation of this population is of concern for lynx conservation in Central Europe because of the population's central geographical location and thus its potential to act as a link between other small and isolated populations. Isolation of a small population may result in reduced genetic variability, lower reproductive success and increased risk of extinction (Schmidt, Reference Schmidt2008). Human-induced mortality may have an even greater impact than low reproductive success (Linnell et al., Reference Linnell, Swenson and Andersen2001), particularly mortality caused by road traffic or illegal killing (Andrén et al., Reference Andrén, Linnell, Liberg, Andersen, Danell and Karlsson2006; Molinari-Jobin et al., Reference Molinari-Jobin, Marboutin, Wölfl, Wölfl, Molinari and Fasel2010; Müller et al., Reference Müller, Wölfl, Wölfl, Müller, Hothorn and Heurich2014). Habitat availability and connectivity may influence population dynamics and thus may be the factor limiting the distribution of the Bohemian–Bavarian lynx.
Information on the spatial distribution, size and connectivity of suitable habitat patches is a prerequisite when comparing actual and potential lynx distribution to maximize the success of population conservation. Models based on a geographical information system provide an effective means of gathering information about spatial distribution and expanse of potential habitat. Previous analyses of lynx habitat have highlighted the importance of forests, and the negative association of lynx presence with habitat fragmentation, human settlements and areas of intensive land use (Niedziałkowska et al., Reference Niedziałkowska, Jędrzejewski, Mysłajek, Nowak, Jędrzejewska and Schmidt2006; Breitenmoser-Würsten et al., Reference Breitenmoser-Würsten, Zimmermann, Molinari-Jobin, Molinari, Capt and Vandel2007; Basille et al., Reference Basille, Herfindal, Santin-Janin, Linnell, Odden and Andersen2009). In previous models of lynx habitat in Germany habitat suitability was assessed based on the availability of forest cover (Schadt et al., Reference Schadt, Knauer, Kaczensky, Revilla, Wiegand and Trepl2002a), and using VHF-telemetry data for 13 lynx in the Swiss Jura Mountains (Schadt et al., Reference Schadt, Revilla, Wiegand, Knauer, Kaczensky and Breitenmoser2002b). Thus there was a risk of predictive uncertainty because the model was extrapolated beyond the domain of the data (Pearson et al., Reference Pearson, Thuiller, Araújo, Martinez-Meyer, Brotons and McClean2006). At the time of our study, novel techniques for species distribution modelling and global positioning system (GPS) telemetry data for lynx of the Bohemian–Bavarian population were available, which facilitated a qualified assessment of habitat in this area by staying within the domain of the data (Guisan & Zimmermann, Reference Guisan and Zimmermann2000). Müller et al. (Reference Müller, Wölfl, Wölfl, Müller, Hothorn and Heurich2014) analysed monitoring data for lynx in Bavaria and found occurrence of lynx was predicted most clearly by proximity to national park areas. Whereas these authors sought predictors to explain lynx occurrence, we focused on the spatial distribution of suitable habitat and of lynx, and estimated a potential population, considering its entire transnational range.
We developed a habitat suitability model with a maximum entropy approach for the Bohemian–Bavarian lynx population, based on GPS telemetry data for 10 individuals in the region; considered spatial requirements of lynx, using estimates of annual home range; assessed the size and connectivity of suitable habitat patches; estimated a potential population size for the area along the border of Germany, the Czech Republic and Austria; and compared our results with regional occurrence of lynx according to the latest status report (Kaczensky et al., Reference Kaczensky, Chapron, von Arx, Huber, Andrén and Linnell2013). We used the Bohemian Forest Ecosystem, with its characteristic Central European lynx population and low mountain range habitat, as a model area. Our objective was to provide wildlife managers and administrators involved in the monitoring and management of populations of large carnivores in Europe with a state-of-the-art tool to compile information about local habitat suitability and carrying capacity for the conservation of a population that, as we hypothesize, is not realizing its potential distribution.
Study area
The Bohemian Forest Ecosystem, Europe's largest region of strictly protected forest, comprises a forested mountain range along the German–Czech border and includes the Bavarian Forest National Park (240 km2) on the German side of the border and the Šumava National Park (690 km2) on the Czech side (Fig. 1a). Human population densities are comparably low: < 2 inhabitants per km2 in the National Parks and c. 70 per km2 in nearby regions. Mean annual temperature is 6.7°C in montane and 3.9°C in subalpine elevation zones. Snow cover persists for 100–200 days, depending on altitude. GPS data from radio-collared lynx were collected in an area of 2,400 km2 within the Bohemian Forest. This area, defined by the 100% minimum convex polygon (MCP) of all lynx localizations, served as the model training area (Fig. 1a). Sixty-six percent of this area is covered by forest and 25% is used for agriculture, primarily grassland. Model prediction covered a more extensive area (110,000 km2) along the borders between Germany, the Czech Republic and Austria (Fig. 1a), 33% of which is covered with forest and 60% used for agriculture.

Fig. 1 (a) The study area along the borders between Germany, the Czech Republic and Austria, for which MaxEnt predictions were obtained for distribution of Eurasian lynx Lynx lynx. (b) MaxEnt prediction of habitat suitability for Eurasian lynx in the study area. Patches with habitat suitability index > 0.35 and a coherent area > 120 km2 are considered suitable (Table 3). Least-cost paths between these patches are indicated. White circles denote records of lynx of Status and Conservation of the Alpine Lynx Population categories C1 and C2, in Bavaria, Germany.
Methods
Habitat data
We configured landscape variables based on CORINE (Coordination of Information on the Environment) land cover 2006 with a resolution of 100 m (European Environment Agency, 2012). The multiple land-use features of CORINE were pooled into 10 categories (Supplementary Table S1). We generated one variable that specified the Euclidean distance to human settlements, and another for altitude (Hijmans et al., Reference Hijmans, Cameron, Parra, Jones and Jarvis2005 ; Table 1). All variables were continuous because we calculated land use as the proportion per grid cell to maintain data quality when coarsening spatial resolution (Seebach et al., Reference Seebach, Strobl, San Miguel-Ayanz and Bastrup-Birk2011). We included altitude as a variable because analyses of faecal pellet count indicated a negative correlation between altitude and the density of roe deer Capreolus capreolus (Heurich et al., Reference Heurich, Brand, Kaandorp, Šustr, Müller and Reineking2015), which is the main prey of the regional lynx (Heurich et al., Reference Heurich, Möst, Schauberger, Reulen, Šustr and Hothorn2012).
Table 1 Predictor variables used to describe habitats in the Bohemian Forest Ecosystem (Fig. 1a), with their definition, unit, range, and contribution to the MaxEnt model based on GPS data from 10 radio-collared lynx Lynx lynx and environmental variables derived from CORINE land-cover data, given as percentage contribution in regularized log-likelihood distribution compared to a uniform distribution. Estimates were determined at each iteration of the training algorithm (convergence threshold 1 × 10−5), starting with a uniform distribution. The increase in regularized gain was added to or subtracted from the contribution of the corresponding variable. Product, linear, quadratic and hinge features were used with regularization values of 0.05 for the first three features and 0.5 for the hinge feature (Phillips & Dudík, Reference Phillips and Dudík2008; Elith et al., Reference Elith, Phillips, Hastie, Dudík, Chee and Yates2011).

Lynx presence data
The habitat model was based on GPS data from 10 radio-collared lynx (six males and four females). The animals were caught in baited box traps and fitted with GPS–GSM collars (VECTRONIC Aerospace, Berlin, Germany; see Podolski et al. (Reference Podolski, Belotti, Bufka, Reulen and Heurich2013) for details). The lynx were collared during 2005–2012; the operating time of individual collars was 3.5–16 months. To limit autocorrelation we standardized sampling frequency to one location per day and randomly sampled without replacement 350 locations for each individual to avoid uneven contribution. However, for three individuals only 85, 146 and 255 locations were available, resulting in a total of 2,936 locations used. These provided information about daytime resting sites as well as crepuscular and nocturnal habitat use. We assume that the locations used represent a random sample of lynx occurrence because, in a test, reception of GPS collars in various habitats was high (99%) and independent of habitat structure (Heurich et al., unpubl. data).
With repeated sampling of one individual it may be necessary to consider the individuality of the lynx. As MaxEnt does not include random effects, we fitted a generalized linear mixed model beforehand to examine individuality. Using the same data set as used subsequently for MaxEnt we tested peculiar individual effects by sampling background points individually for each lynx in its spatial range. Randomly sampled background points facilitate presence-only modelling, and rather than representing species’ absence they characterize the environment. Tested individual effects were negligible (random effect standard deviation < 1.5% of fixed effect intercept; Supplementary Table S2).
Distribution modelling
To model the potential distribution of the lynx population we used MaxEnt v. 3.3 (Phillips et al., Reference Phillips, Dudík and Schapire2010). MaxEnt attempts to calculate the probability distribution of a species’ presence based on the constraints derived from environmental data at the presence locations. Following the principle of maximum entropy, the method remains as close as possible to uniform distribution. We used 10,000 background points to achieve the best possible results (Phillips & Dudík, Reference Phillips and Dudík2008; Barbet-Massin et al., Reference Barbet-Massin, Jiguet, Albert and Thuiller2012).
As the choice of resolution may affect model predictions (Guisan et al., Reference Guisan, Graham, Elith and Huettmann2007), we aimed to detect the most suitable grid cell size by calculating MaxEnt models under default settings for grids with edge lengths of 200, 500, 700, 1,000, 1,200 and 1,500 m. Following Guisan et al. (Reference Guisan, Graham, Elith and Huettmann2007) we did not reduce the number of records by removing duplicate records in the same grid cell, but left sample size consistent between the models of various grid cell sizes. Each model was fitted and evaluated five times by k-fold cross-validation, and thus each model had random samples of 80% lynx telemetry data for training and 20% for testing (Hirzel et al., Reference Hirzel, Le Lay, Helfer, Randin and Guisan2006). Model performance was assessed by comparing the means of the area under the receiver operating characteristic curves (AUC). With presence-only (PO) modelling, AUCPO depicts the probability that a randomly chosen presence site will be ranked above a randomly chosen background site, whereby a random ranking (reflecting uniform distribution) achieves an AUCPO of 0.5 and a nearly perfect ranking approaches an AUCPO of 1 (but with the use of background locations it never achieves this; Wiley et al., Reference Wiley, McNyset, Peterson, Robins and Stewart2003). AUCPO values vary with the spatial extent used to select background points as well as with the number of background points; because we only modified grid cell sizes but not the extent of the area or the number of background points, value comparison was possible.
In the model prediction we aimed for an output grid comprising a map of suitability scores. We used the logistic output to obtain a relative habitat suitability index of 0–1, with 0 indicating unsuitable habitat and 1 indicating highly suitable habitat.
To select only raster cells for which we were able to make reliable predictions (i.e. cells characterized by a parameter composition similar to that of cells inside the model training area) we conducted a principal component analysis for all raster cells within the model prediction area, including the training area. Positions of raster cells were mapped in a scatterplot and a range of predictability was set on the basis of the distribution of raster cells characterizing the model training area. We predicted the model only on raster cells located within this range.
Model evaluation
To assess model accuracy by AUC we used monitoring data recorded for lynx in eastern Bavaria during 2005–2010, a data set independent of our GPS telemetry locations used for training the model. We recorded observations of lynx, using the categories of the Status and Conservation of the Alpine Lynx Population project (SCALP; Molinari-Jobin et al., Reference Molinari-Jobin, Kéry, Marboutin, Molinari, Koren and Fuxjäger2012): category C1 denotes proof of lynx presence (dead or captured lynx, photographs, genetic samples); C2 accounts for confirmed observations (e.g. lynx kill or paw prints confirmed by an expert); C3 accounts for unconfirmed observations. We used C1 and C2 observations for model evaluation, resulting in a data set of 625 observations.
Habitat quantification and connectivity
To estimate the potential population size we needed information on the spatial requirements of lynx and on the amount of suitable habitat. We assessed spatial requirements by calculating 95% MCPs (MCP95) for home range size in accordance with previous studies (Herfindal et al., Reference Herfindal, Linnell, Odden, Birkeland Nilsen and Andersen2005; Breitenmoser-Würsten et al., Reference Breitenmoser-Würsten, Zimmermann, Molinari-Jobin, Molinari, Capt and Vandel2007), and chose 100% MCPs (MCP100) as well as 90% MCPs (MCP90) to obtain a range of population estimates. We selected time periods of 365 days and standardized the sampling frequency to one location per day.
To quantify available habitat it is necessary to convert continuous habitat suitability index values into binary results. There are various approaches that can be used to select a threshold (Liu et al., Reference Liu, Berry, Dawson and Pearson2005). We used a new approach based on our model evaluation, which we considered to be the most consistent approach. We assumed all values representing suitable habitat were above the point at which the density of presence locations exceeded that of the background locations that characterized the environment.
For the potential population estimate we considered only suitable habitat patches larger than the mean home range of a female lynx, and calculated the percentages of suitable habitat cells within their spatial ranges (for MCP95, MCP90, MCP100).
To assess the connectivity between suitable habitat patches we calculated least-cost paths based on a resistance grid at a resolution of 250 × 250 m. The resistance grid resulted from an overlay of the reciprocal resampled habitat suitability map (low habitat suitability index implies high resistance and vice versa; LaRue & Nielsen, Reference LaRue and Nielsen2008) and a rasterized road layer (ESRI, Redlands, USA) with resistance values assigned according to road status (Hebblewhite et al., Reference Hebblewhite, Zimmermann, Li, Miquelle, Zhang and Sun2012). We assigned values within the range of the inverse habitat suitability, with the highest cost assigned to highways (Autobahn, resistance value = 1), medium cost assigned to main roads (Bundesstraße, resistance value = 0.85), and low cost assigned to municipal roads (Landstraße, resistance value = 0.7). Least-cost paths start at the centre of the patch of departure and end when the contour of the target patch is reached. We extracted the path lengths (> 5 km) between the patch contours to define the distances that lynx must travel when moving from one patch to another. For the assessment of patch connectivity we also considered 84 VHF telemetry positions of a subadult dispersing lynx, recorded in 1997. The comparison of suitable patches with lynx occurrence in the region according to the latest status report (Kaczensky et al., Reference Kaczensky, Chapron, von Arx, Huber, Andrén and Linnell2013) resulted from a spatial overlay.
MaxEnt modelling, generalized linear mixed modelling, and home range computations were carried out in R v. 2.15.1 (R Development Core Team, 2012) using the packages dismo (Hijmans et al., Reference Hijmans, Phillips, Leathwick and Elith2012), lme4 (Bates et al., Reference Bates, Maechler and Bolker2012), and adehabitatHR (Calenge, Reference Calenge2006). We used ArcMap v. 10.2 (ESRI, Redlands, USA) to calculate least-cost paths and create maps.
Results
Distribution modelling
We tested the MaxEnt model at various grid cell sizes and selected the 1,000 × 1,000 m grid cell size for further analysis because it yielded the best model performance (Supplementary Table S3). We limited the range of model predictability on the basis of the distribution of the model training area cells in the principal component analysis scatterplot (Fig. 2). Twenty-three percent of cells were thus excluded, including cells with high percentages of human settlements (human) or arable land (acre), which differed most from cells inside the model training area.
Contributions of the specific environmental variables to the MaxEnt model are in Table 1. Response curves (Supplementary Fig. S1) provide more information on the relationship between environmental variables and the habitat suitability index. Anthropogenic open-space land-use types contributed most to the model and were negatively correlated with habitat suitability index. The occurrence of human settlements, industry or artificial surfaces (variable human; Supplementary Fig. S1) was linked to a comparably low habitat suitability index, and the response curve of variable distanc_hum demonstrated an increase in habitat suitability index with increasing distance to human settlements. Transitional woodland shrub and wetlands (variable woodshrub) and the three forest types broad-leaved, coniferous, and mixed provided comparatively suitable habitat, but for the variables forestmix (mixed forests) and forestconif (coniferous forest) the increase in habitat suitability index was marginal. High percentages of agricultural areas with significant natural vegetation or complex cultivation patterns (variable naturalagri) as well as high altitudes (variable altitude) resulted in a lower habitat suitability index. By projection of the trained MaxEnt model onto the model prediction area we created a habitat suitability map (Fig. 1b).
Model evaluation and threshold selection
Threshold-independent extrinsic model evaluation resulted in an AUCPO of 0.889. However, habitat suitability index values are also of interest. Lynx were observed in areas with higher habitat suitability index values (median = 0.488) than background locations (median = 0.083; Wilcoxon test: W = 5,554,179, P < 0.0001). The density plot (Fig. 3) displays similar information, namely that highest densities of observations were found in areas with habitat suitability index values of c. 0.4–0.6; in contrast, background locations with the highest densities of observations had habitat suitability values of 0.0–0.2. With the aim of quantifying available habitat we selected the intercept of the density curves, at a habitat suitability index of 0.35, as our threshold and defined habitat suitability index values ≥ 0.35 as suitable habitat. According to this threshold 89% of the Status and Conservation of the Alpine Lynx Population evaluation data comprise suitable habitat.

Fig. 3 Habitat suitability of 625 locations along the borders between Germany, the Czech Republic and Austria (Fig. 1a) where presence of lynx was confirmed, and 10,000 random background locations.
Habitat quantification, connectivity and occupancy
Mean home range sizes for male and female lynx were 445 ± SD 128 km2 and 122 ± SD 41 km2, respectively. Home ranges of males were significantly larger than those of females (W = 20, P = 0.016; Table 2). For the lynx named Patrik we only considered a 300-day period because he shifted his home range after that period.
Table 2 Home range estimations (based on minimum convex polygon, MCP) for radio-collared adult lynx in the Bohemian Forest Ecosystem (Fig. 1a) during 2005–2012, with name and sex of individuals, number of kittens, MCP90, MCP95, MCP100, time period of data collection, and number of locations recorded.

* Excluded because GPS locations were recorded only on 100 days
Percentages of suitable habitat cells within the home ranges (MCP95, Table 2) were 90% for females and 83% for males. We therefore assumed a habitat area of 110 km2 (122 × 0.9) for an average female and 369 km2 (445 × 0.83) for an average male. Consequently, we calculated a density of 1.18 resident lynx per 100 km2 of suitable habitat, resulting in a potential population size of c. 142 individuals (distributed among 13 patches; Fig. 1b, Table 3). The percentage of suitable habitat cells within MCP100 (Table 2) was 89% for an average female lynx and 80% for a male. The percentage of suitable habitat cells within MCP90 (Table 2) was 90% for an average female and 84% for a male, resulting in an estimated population of 93–160 individuals (Table 3).
Table 3 Estimated area of suitable lynx habitat patches in the study area (Fig. 1a), with potential number of lynx, and whether occupancy is permanent or sporadic.
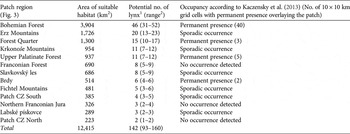
1 Based on 1.18 per 100 km2 suitable habitat
2 Based on 0.81–1.34 per 100 km2 suitable habitat
The results of the least-cost path analyses (Fig. 1b; Supplementary Table S4) depict the connectivity between these patches. The shortest least-cost path between these patches was 8 km; the longest was 52 km. Comparison of suitable habitat patches with reported lynx occurrence (Kaczensky et al., Reference Kaczensky, Chapron, von Arx, Huber, Andrén and Linnell2013) indicated permanent occupancy in four patches, sporadic occurrence in six patches, and no occurrence in three patches (Table 3).
Discussion
In our study area we estimated a habitat carrying capacity for a lynx population of c. 142 individuals (based on population estimates of 93 (MCP100) to 160 (MCP90) individuals), distributed across 13 patches (Fig. 1b, Table 3). This estimate refers to territorial lynx and does not include the population of subadults, which can be 5–35% of the resident population (Jędrzejewski et al., Reference Jędrzejewski, Jędrzejewska, Okarma, Schmidt, Bunevich and Miłkowski1996; Zimmermann & Breitenmoser, Reference Zimmermann and Breitenmoser2007). A spatial comparison of potential and actual lynx distribution with the latest status report (Kaczensky et al., Reference Kaczensky, Chapron, von Arx, Huber, Andrén and Linnell2013) revealed permanent lynx presence in four of these patches (Bohemian Forest, Upper Palatinate Forest, Brdy, Forest Quarter; Table 3). Of these, there is a permanent patch-wide distribution of lynx only in the Bohemian Forest. This represents the source of the population and includes the Bavarian Forest National Park and the Šumava National Park, which have been shown to be of significant importance for lynx conservation in the region (Müller et al., Reference Müller, Wölfl, Wölfl, Müller, Hothorn and Heurich2014). Nine patches (5,760 km2 in total) are not permanently occupied by lynx.
We analysed least-cost paths to assess connectivity between suitable habitat patches and considered available information on regional lynx distribution. In Bavaria there is evidence (Status and Conservation of the Alpine Lynx Population C1 and C2 categories) that lynx are able to reach the Fichtel Mountains and Franconian Forest in the north-west of our model prediction area (Fig. 1b). Connectivity to Brdy is not immediately apparent but VHF positions of a subadult lynx reveal that Brdy is accessible (Supplementary Fig. S2). Reported lynx distribution (Kaczensky et al., Reference Kaczensky, Chapron, von Arx, Huber, Andrén and Linnell2013) confirms accessibility of the remaining patches except for Northern Franconian Jura and Patch CZ North (Table 3). Moreover, data on lynx dispersal in Switzerland (2–97 km; Zimmermann et al., Reference Zimmermann, Breitenmoser-Würsten and Breitenmoser2005) and Scandinavia (3–428 km; Samelius et al., Reference Samelius, Andrén, Liberg, Linnell, Odden and Ahlqvist2012) indicate the ability of lynx to access suitable patches in our model prediction area. The roads located between suitable patches in our model prediction area are mostly main roads and municipal roads, which pose risks but not barriers for lynx. Highway A9, which separates the Franconian Forest from the other patches, may be more difficult to cross but a category C1 observation indicated that crossing is possible. Thus, we assume all patches are reachable for lynx, but highlight that fragmentation of patches needs to be considered in landscape planning to prevent degradation of connectivity. The habitat map (raster file available on request) may be used for this purpose by administrators and wildlife managers, and also as a basis for planning lynx reintroductions.
Besides increasing numbers of lynx and thus raising the probability of connecting with isolated populations, reintroductions help to overcome deleterious effects of any potential inbreeding depression (Johnson et al., Reference Johnson, Onorato, Roelke, Land, Cunningham and Belden2010). The genetic status of the Bohemian–Bavarian population is unknown as genetic analyses are lacking. Nevertheless, camera-trap data (Supplementary Table S5; for methods see Weingarth et al., Reference Weingarth, Heibl, Knauer, Zimmermann, Bufka and Heurich2012) indicate substantial reproduction in the Bohemian Forest patch, and lynx dispersal is supported by sporadic occurrence in most patches. Despite this, lynx are apparently unable to establish permanent subpopulations there, and in line with other authors (Wölfl et al., Reference Wölfl, Bufka, Červený, Koubek, Heurich and Habel2001; Červený et al., Reference Červený, Koubek and Bufka2002; Molinari-Jobin et al., Reference Molinari-Jobin, Marboutin, Wölfl, Wölfl, Molinari and Fasel2010; Müller et al., Reference Müller, Wölfl, Wölfl, Müller, Hothorn and Heurich2014) we assume that illegal killing is the most likely factor limiting the distribution of the population.
Our home-range-based population estimate is higher than that of Schadt et al. (Reference Schadt, Revilla, Wiegand, Knauer, Kaczensky and Breitenmoser2002a,Reference Schadt, Revilla, Wiegand, Knauer, Kaczensky and Breitenmoserb), which extrapolated data for Swiss lynx to Germany and thus had predictive uncertainty. We estimated there was up to 12,415 km2 of suitable habitat in the prediction area. Schadt et al. (Reference Schadt, Revilla, Wiegand, Knauer, Kaczensky and Breitenmoser2002a,Reference Schadt, Revilla, Wiegand, Knauer, Kaczensky and Breitenmoserb) estimated a smaller total area of suitable habitat along the Austrian–German–Czech border but they did not consider the entire range of the Bohemian–Bavarian population but rather the different spatial requirements of lynx. Schadt et al. (Reference Schadt, Revilla, Wiegand, Knauer, Kaczensky and Breitenmoser2002b) assumed an overlap of one male per female lynx within 99 km2 and reported an approximate estimate of 100 individuals. Schadt et al. (Reference Schadt, Knauer, Kaczensky, Revilla, Wiegand and Trepl2002a) modelled 77 potential territories, considering the spatial requirements of Swiss lynx. Our analyses resulted in a higher potential population estimate of 142 (93–160). For further comparisons of population estimation, a habitat-based approach that does not require thresholding habitat suitability data (Boyce & McDonald, Reference Boyce and McDonald1999) would be informative. Such an approach has been applied in large carnivore conservation (Hebblewhite et al., Reference Hebblewhite, Zimmermann, Li, Miquelle, Zhang and Sun2012), but a reliable estimate of the size of the existing population as well as a detailed delineation of its range are needed as references.
In accordance with results of previous studies (Schadt et al., Reference Schadt, Revilla, Wiegand, Knauer, Kaczensky and Breitenmoser2002b; Niedziałkowska et al., Reference Niedziałkowska, Jędrzejewski, Mysłajek, Nowak, Jędrzejewska and Schmidt2006) our MaxEnt model of lynx distribution indicated that lynx avoid areas close to human settlements or with intense anthropogenic disturbance. Similarly, Basille et al. (Reference Basille, Herfindal, Santin-Janin, Linnell, Odden and Andersen2009) reported that lynx avoid agricultural areas, but in contrast to our study they did not find that lynx avoided areas close to artificial areas. Previous studies have emphasized the importance of forest for lynx (Schadt et al., Reference Schadt, Revilla, Wiegand, Knauer, Kaczensky and Breitenmoser2002b; Niedziałkowska et al., Reference Niedziałkowska, Jędrzejewski, Mysłajek, Nowak, Jędrzejewska and Schmidt2006; Basille et al., Reference Basille, Herfindal, Santin-Janin, Linnell, Odden and Andersen2009; Müller et al., Reference Müller, Wölfl, Wölfl, Müller, Hothorn and Heurich2014). Our model was not primarily determined by variables linked to forest, possibly because of the comparatively high availability of forest (66% of the model training area). Our results also indicated that in the study area lynx preferred areas of lower altitude, which is in accordance with the results of Basille et al. (Reference Basille, Herfindal, Santin-Janin, Linnell, Odden and Andersen2009) and matches the increasing density of roe deer with decreasing altitude (Heurich et al., Reference Heurich, Brand, Kaandorp, Šustr, Müller and Reineking2015). Prey density could not be included in the model because no transnational data were available, but we expect prey densities in the model prediction area to be equivalent to or higher than within the forest-dominated model training area. We base this assumption on the roe deer density index in Bavaria (Hothorn et al., Reference Hothorn, Brandl and Müller2012) and on previous records of high roe deer densities in less-forested areas (Melis et al., Reference Melis, Jędrzejewska, Apollonio, Bartoń, Jędrzejewski and Linnell2009). An advantage of the use of coarse transnational data was the ability to consider the entire Bohemian–Bavarian population, unlike the study of Müller et al. (Reference Müller, Wölfl, Wölfl, Müller, Hothorn and Heurich2014), which was restricted to Bavaria. However, we are aware that we disregarded fine-scale habitat characteristics of forests, such as essential cover for den sites, daily resting sites (Podgórski et al., Reference Podgórski, Schmidt, Kowalczyk and Gulczyńska2008), and cover from hunting (Dickson & Beier, Reference Dickson and Beier2002). Considering the intentions of our study, however, we assume these characteristics to be negligible as forest should provide them sufficiently.
We used MaxEnt to assess habitat suitability, a presence-only method that is designed to make predictions based on incomplete data (Elith et al., Reference Elith, Phillips, Hastie, Dudík, Chee and Yates2011). The predictive accuracy of MaxEnt is high, which makes it consistently competitive among the highest-performing methods (Guisan et al., Reference Guisan, Graham, Elith and Huettmann2007; Phillips & Dudík, Reference Phillips and Dudík2008). MaxEnt was not developed primarily for use with telemetry data (Baldwin, Reference Baldwin2009), which are characterized by repeated sampling of one individual. However, as reception of GPS data on lynx locations did not vary with habitat the detection probability of telemetry data is consistent across a study area and the data represent a random sample of presence locations, thereby meeting the basic assumptions of MaxEnt (Yackulic et al., Reference Yackulic, Chandler, Zipkin, Royle, Nichols, Campbell Grant and Veran2013).
The model was complex because lynx presence could not be associated with a particular variable, but evaluation revealed good model performance according to the AUCPO. Qualification of the AUC as a measure of model accuracy has been criticized (Yackulic et al., Reference Yackulic, Chandler, Zipkin, Royle, Nichols, Campbell Grant and Veran2013); nevertheless, our approach of thresholding based on model evaluation categorized 89% of the Status and Conservation of the Alpine Lynx Population data correctly. Our approach therefore equals a cut-point probability at 89%, compared with the cut-point probabilities of Hebblewhite et al. (Reference Hebblewhite, Zimmermann, Li, Miquelle, Zhang and Sun2012), who chose 85% with Amur tiger Panthera tigris altaica tracks, and Hebblewhite et al. (Reference Hebblewhite, Miquelle, Murzin, Aramilev and Pikunov2011), with 90% for Far Eastern leopards Panthera pardus orientalis.
As the scale of predictor variables affects the model prediction, we evaluated model performance for various grid cell sizes. Our results indicated that the habitat model performed best using a grid cell of 1 km2 and that, in line with Guisan et al. (Reference Guisan, Graham, Elith and Huettmann2007), a lower resolution does not necessarily imply poorer performance. In contrast, the model performed best at a grid cell size five times coarser than the smallest resolution tested. From an ecological point of view, a comparably coarse grid cell size makes sense when modelling habitat of a species with large spatial requirements, such as the lynx, and facilitates the necessarily wide-ranging conservation planning (Chapron et al., Reference Chapron, Kaczensky, Linnell, von Arx, Huber and Andrén2014). Overly coarse grain is not effective, however; model performance decreased when a grid cell size of > 1 km2 was used. A study on the sensitivity of MaxEnt to scale differences indicated that the maximum grain size should be c. 1.5 km (Song et al., Reference Song, Kim, Lee, Lee and Jeon2013).
We assume our model predictions are reasonable as we did not extrapolate our model beyond the ranges of variable values used to train it. Others have cautioned against going beyond the calibration range (Pearson et al., Reference Pearson, Thuiller, Araújo, Martinez-Meyer, Brotons and McClean2006; Hirzel & Le Lay, Reference Hirzel and Le Lay2008), yet this is rarely considered in model applications. We benefited from lynx data originating from a part of the model prediction area, and by defining a range of predictability we were able to predict 77% of cells within the study area.
The applied least-cost path method estimates the distances that lynx have to overcome between suitable habitat patches, but assumes that an individual has a planned destination when leaving a patch. We acknowledge that a more complex stochastic movement simulator without this assumption could map dispersal paths more precisely (Palmer et al., Reference Palmer, Coulon and Travis2011). As we intended to estimate the distances rather than determine the exact paths, we believe the least-cost path method is sufficient.
From our results we draw some conclusions regarding the conservation of a characteristic Central European lynx population with the potential to connect small and isolated populations. Firstly, despite connectivity, the current distribution of the Bohemian–Bavarian lynx population is not in equilibrium with available habitat, which in line with Chapron et al. (Reference Chapron, Kaczensky, Linnell, von Arx, Huber and Andrén2014) indicates that factors other than habitat limit the distribution, with illegal killing being the most likely factor. We thus recommend focusing future research on dispersing subadult lynx, with the aim of increasing acceptance of lynx by people. Secondly, the potential carrying capacity of habitat in the study area is not sufficient for a long-term viable population, which according to expert opinion should comprise c. 500 individuals (Linnell et al., Reference Linnell, Salvatori and Boitani2008). Thus, the population requires connectivity to other populations (i.e. Czech Carpathian, German Harz, and Austrian Alpine populations). Thirdly, patches of suitable habitat are fragmented; their distribution needs to be considered in landscape and road development to prevent further fragmentation, and when planning and locating sites for lynx reintroduction.
Acknowledgements
This study is part of the Eurasian lynx project of the Bavarian Forest National Park, Department of Research and Documentation. Financial support was provided by EU Programme Interreg IV (EFRE Ziel 3). We thank O. Vojtěch, J. Mokrý, H. Burghart, M. Gahbauer, and other staff of Šumava National Park and Bavarian Forest National Park for their help with field work, and F. Gutkowski for analyses of GPS collar performance. We also thank Karen A. Brune for improving the writing of the manuscript, and Miha Krofel and three anonymous reviewers for their helpful comments.
Biographical sketches
Nora Magg develops guidelines for conservation of faunistic species in managed forests. Jörg Müller is a conservation biologist interested in the effects of varying management intensities on forest biodiversity. Christoph Heibl works on data analyses in ecology and evolutionary biology. Klaus Hackländer focuses on wildlife ecology and develops management strategies for sustainable use, conservation or control of wildlife species. Sybille Wölfl works on lynx conservation and management. Manfred Wölfl works on wildlife management, with a focus on human dimensions and conflict mitigation. Ludêk Bufka is a zoologist specializing in research and conservation of large carnivores. Jaroslav Červený focuses on wildlife biology and the ecology of mammals. Marco Heurich is interested in the ecology and conservation of natural forest ecosystems.