Introduction
Precision farming or precision agriculture is based on using the inherent spatial and temporal variability in a field as a basis to manage farm operations (Goel et al., Reference Goel, Prasher, Landry, Patel, Bonnell, Viau and Miller2003). It is a management practice made possible by the advent of suitable information technologies (Ryu et al., Reference Ryu, Suguri and Umeda2009; Roslim et al., Reference Roslim, Juraimi, Che’Ya, Sulaiman, Abd Manaf, Ramli and Motmainna2021). Regarding time-effective methods, the practice of precision farming requires the development of accurate and reliable crop monitoring techniques to provide information on the spatial and temporal variations in key agronomic parameters (Steven, Reference Steven2004). A comprehensive review on status of various components for precision farming and application of information technology was presented by Plant (Reference Plant2001). He clearly highlighted the importance of high-altitude remote sensing to obtain information on crop growth condition. Remote sensing is a useful tool for monitoring spatial and temporal variation in crop morphology and physiological conditions and for supporting precision agriculture practices (Lu et al., Reference Lu, Dao, Liu, He and Shang2020).
Traditional methods for detecting and monitoring essential parameters of crops need detailed sampling, time, and expensive laboratory chemical analyses, which is neither economically viable nor environmentally acceptable on a large scale (Mahajan et al., Reference Mahajan, Pandey, Sahoo, Gupta, Datta and Kumar2017). Remote sensing can be used to collect information at vastly larger spatial extents more quickly and more cheaply per unit area than field sampling (Flynn et al., Reference Flynn, Frazier and Admas2020). It can also be combined with field data to more efficiently assess spatial and temporal distributions of crop parameters (Aneece et al., Reference Aneece, Epstein and Lerdau2017; Afrasiabian et al., Reference Afrasiabian, Noory, Mokhtari, Nikoo, Pourshakouri and Haghighatmehr2021). Over the past three decades, remote sensing techniques have been used as very useful tools to precisely monitor crops throughout their growing season to support decisions for good agricultural practices (Astor et al., Reference Astor, Dayananda, Nautiyal and Wachendorf2020).
Although the remote sensing technique is widely used for timely detection of variations in the spectral response of crops over large areas, the multispectral remote sensors exhibit serious limitations to accurately detect changes in crop because of coarse spectral resolutions that hide detailed information of signals from crop parameters (Hong and Abd El-Hamid, Reference Hong and Abd El-Hamid2020). Early multispectral images are limited by spectral resolution, which affects the accuracy of retrieval variables and leads to the inability to detect early signals of crop stress in a timely and effective manner (Ang and Seng, Reference Ang and Seng2021). Hyperspectral remote sensing (HRS), also known as imaging spectroscopy or hyperspectral imaging (HSI), involves hundreds of spectral narrow bands that are sensitive to distinct biophysical and biochemical characteristics masked by the broad bands of multispectral remote sensing (Goetz Reference Goetz2009; Slonecker et al., Reference Slonecker, Allen, Resmini, Rand and Paine2018; Gao et al., Reference Gao, Meng, Liang, Feng, Ge, Yin, Wu, Cui, Hou, Liu and Xie2019; Meivel and Maheswari, Reference Meivel and Maheswari2021). Thus, advances in HRS provide opportunities for detailed mapping, modeling, and biophysical characterization of crops (Nidamanuri and Zbell, Reference Nidamanuri and Zbell2011). It is more capable of detecting subtle changes in ground cover and its variation over time.
HRS can be used to address the above challenges and facilitate more accurate and timely detection of crop physiological states. The main research is on field and laboratory hyperspectral measurements for monitoring agriculture and vegetation, retrieval of plant traits in leaf and canopy layers from hyperspectral measurements (Berger et al., Reference Berger, Verrelst, Feret, Wang, Wocher, Strathmann and Hank2020; Dobrota et al., Reference Dobrota, Carpa and Butiuc-Keul2021); hyperspectral sensor calibration and product validation for agriculture and vegetation monitoring, product quality validation (Fahey et al., Reference Fahey, Hai, Gardi, Sabatini and Lamb2020; Jia et al., Reference Jia, Chen, Zheng, Wang and Chen2022); statistical and computational methods for agricultural and vegetation monitoring (Murphy et al., Reference Murphy, Boruff, Callow and Flower2020; Ma et al., Reference Ma, Rehman, Zhang, Maki, Tuinstra and Jin2021; Nansen et al., Reference Nansen, Murdock, Purington and Marshall2021); studies for agricultural and vegetation living environments, microbial load, surrounding species, etc. (Santos-Rufo et al., Reference Santos-Rufo, Mesas-Carrascosa, Garcia-Ferrer and Merono-Larriva2020).
HRS advances constitute a revolution in agriculture and in particular, crop monitoring. The widespread use of HRS for crop parameter acquisition suggests the necessity to review the progress of research in this field. The objectives of this paper are a) to discuss the different platforms and sensors of HRS, summarize the methods available for processing and analyzing hyperspectral information, and recent advances in HSI for agricultural applications; b) to discuss and summarize current developments in crop monitoring supported by HRS and to assess the performance of HRS under different applications; c) to further help agricultural researchers and practitioners better understand the advantages and limitations of HSI in agricultural applications and to promote the opportunities and challenges of using HRS for crop monitoring applications.
HRS monitoring platforms and sensors
Remote sensing platform is used to place various remote sensors to detect ground targets from a certain height or distance and to provide technical support and working conditions for the sensors (Zhong et al., Reference Zhong, Hu, Luo, Wang, Zhao and Zhang2020). According to the height of the platform from the ground, it can be divided into three categories: ground platform, airborne platform, and spaceborne platform (Table 1). These remote sensing platforms with different technical performances and working modes form a multilayer and three-dimensional modern remote sensing information acquisition system, which provides a technical guarantee for the completion of thematic or integrated, regional or global, and static or dynamic remote sensing activities. The working height of ground remote sensing platform is generally less than 100 m, which mainly includes vehicle, ship, handle, fixed or movable elevated platform, etc. Airborne remote sensing platform mainly includes balloon, plane, and other aircraft. Plane specially designed or modified as needed is the main platform of airborne remote sensing because of its flexibility, wide observation range, and high measurement accuracy. Spaceborne remote sensing platform mainly includes satellite, space shuttle, and spacecraft, etc. The prominent characteristics of spaceborne remote sensing are high altitude, large observation range, and fast monitoring speed (Wei et al., Reference Wei, Wang, Lu, Liang, Li, Wang, Wang and Cao2021). Satellite is the widely used platform of spaceborne remote sensing, which is suitable for large-scale surface accurate monitoring. With the continuous improvement of sensor resolution, the status of satellite monitoring platform will become more important. Existing or planned satellite HRS platforms are summarized in Table 2. It can be seen that not many satellites are currently in running and are about to be launched, but the progress of research and development has been significantly accelerated in recent years. Furthermore, sensors with higher spatial resolution and wider spectral range have become a trend.
Table. 1. The synoptic scheme chart showing different hyperspectral remote sensing platforms with diverse features
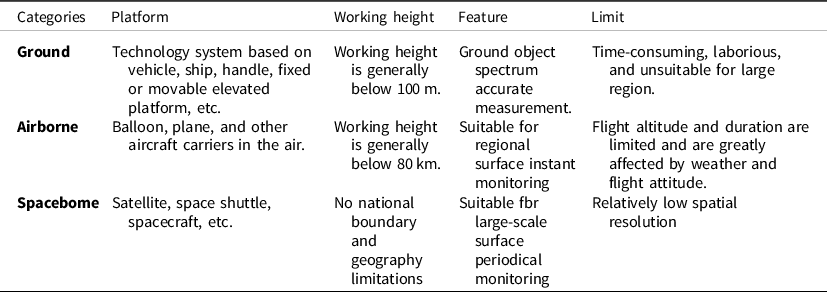
Table 2. Existing or planned satellite hyperspectral remote sensing platforms
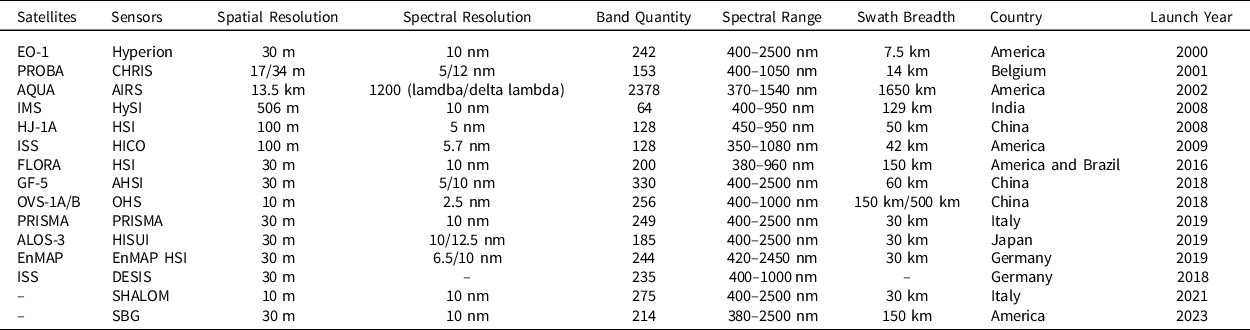
Application of HRS in monitoring diverse crop objects
A literature search was performed to examine if more research in using HSI for agricultural purposes had been published in recent years. It was found that there was an increasing number of publications in recent years that used HSI for agricultural applications (Table 3). During 2013–2019, we searched papers on the ‘Web of Science’ website using keywords containing ‘HRS’ and ‘crop’ to find that HRS has been widely used in monitoring of various crops which includes corn (Essayed and Darwish, Reference Elsayed and Darwish2017), wheat (Zhang et al., Reference Zhang, Ren, Zhou, Wu, He, Heng, Feng and Wang2018), rice (Krishna et al., Reference Krishna, Sahoo, Singh, Bajpai, Patra, Kumar, Dandapani, Gupta, Viswanathan, Ahmad and Sahoo2019), cotton (Marshall et al., Reference Marshall, Thenkabail, Biggs and Post2016), grapevine (Zovko et al., Reference Zovko, Žibrat, Knapič, Kovačić and Romić2019), Crambe abyssinica Hochst (Viana et al., Reference Viana, Mercante, de Andrade, Felipetto, Cattani, Bombarda and Boas2018), white bean, canola, peas (Pacheco et al., Reference Pacheco, Bannari, Staenz and McNairn2008), sugarcane (Mokhele and Ahmed, Reference Mokhele and Ahmed2010), soybean (Yuan et al., Reference Yuan, Yang, Li, Wang, Liu, Yu, Feng, Xu, Zhao and Yang2017), sugar beet (Inoue et al., Reference Inoue, Guerif, Baret, Skidmore, Gitelson, Schlerf, Darvishzadeh and Olioso2016), mustard (Kumar et al., Reference Kumar, Vashisth, Sehgal and Gupta2013), barley (Lausch et al., Reference Lausch, Salbach, Schmidt, Doktor, Merbach and Pause2015), blackgram (Prabhakar et al., Reference Prabhakar, Prasad, Desai, Thirupathi, Gopika, Rao and Venkateswarlu2013), and potato leave (Latorre-Carmona et al., Reference Latorre-Carmona, Knyazikhin, Alonso, Moreno, Pla and Yan2014). A chart showing the application domain of HRS in crop monitoring is provided for clear description. These monitoring work takes place in many different places such as North America, Asia, Europe, South America, and Africa, which means that specific crops are widely distributed in certain regions, or different regions have their own concerned crops. Corn, wheat, and rice are the three most widely distributed crops in the world. It also can be seen from these literatures that they are the three most common monitoring objects in the application of HRS technology. However, there are relatively few studies on many other crops, and even many crops which are important for regional economic development have not been studied, e.g., tobacco, tomato, pepper, cucumber, and carrot. There are many types of hyperspectral sensors at present, and similar studies still need to be further conducted in other areas for different crop species.
Table 3. Applications of hyperspectral remote sensing in crop monitoring during 2003–2019
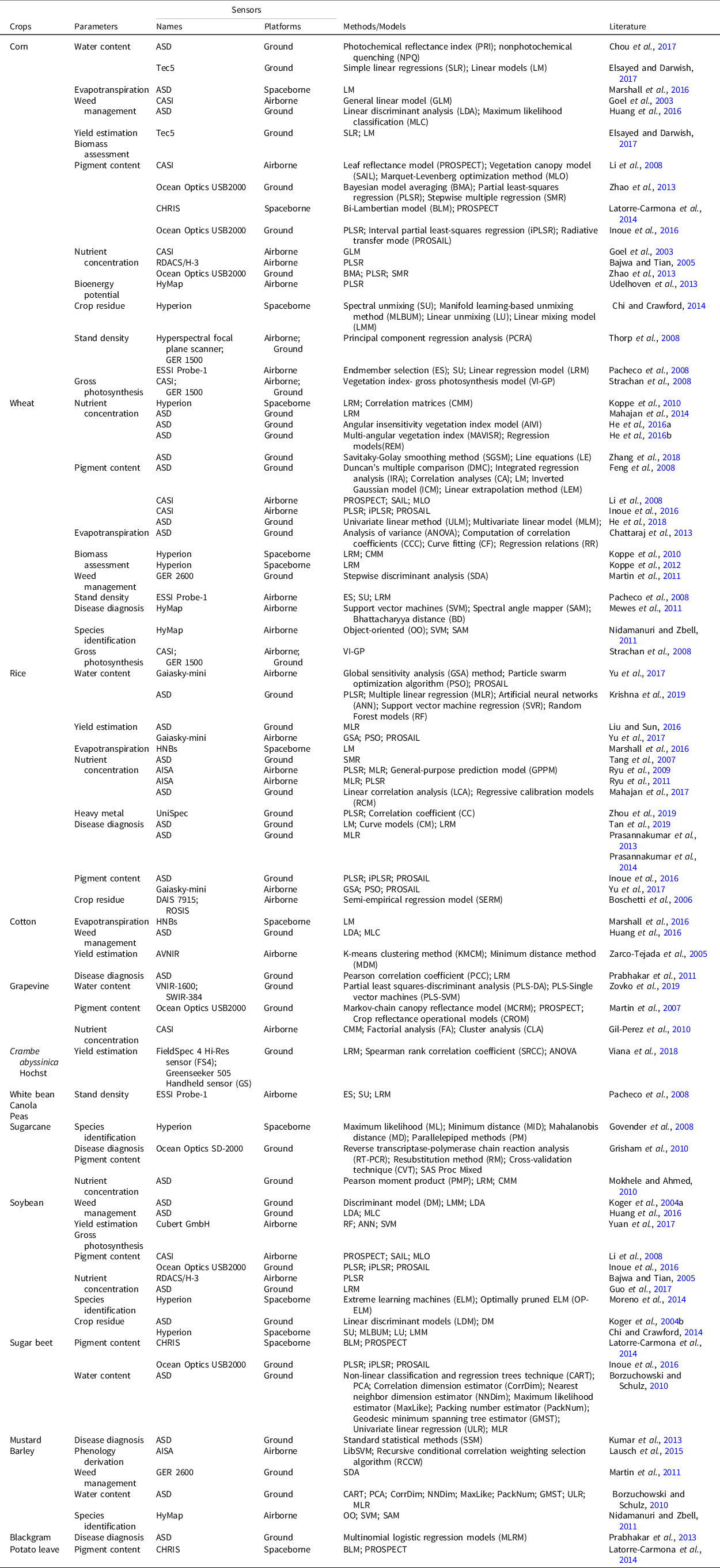
Application of HRS in retrieving key crop parameters
HRS provides an effective means for the extraction of plant parameters (Millan and Azofeifa, Reference Millan and Azofeifa2018; Yu et al., Reference Yu, Kong, Wang, Sun and Wang2018). It also has been widely used in retrieving crop parameters including water content (Chou et al., Reference Chou, Chen, Yu, Chen, Zhang, Croft, Khalid, Li and Shi2017), weed management (Huang et al., Reference Huang, Lee, Thomson and Reddy2016), evapotranspiration (Marshall et al., Reference Marshall, Thenkabail, Biggs and Post2016), yield estimation (Elsayed and Darwish, Reference Elsayed and Darwish2017), heavy metal (Zhou et al., Reference Zhou, Zhang, Zou, Liu, Du, Wang, Liu, Liu and Li2019), bioenergy potential (Udelhoven et al., Reference Udelhoven, Delfosse, Bossung, Ronellenfitsch, Mayer, Schlerf, Machwitz and Hoffmann2013), stand density (Pacheco et al., Reference Pacheco, Bannari, Staenz and McNairn2008), crop residue (Chi and Crawford, Reference Chi and Crawford2014), gross photosynthesis (Yuan et al., Reference Yuan, Yang, Li, Wang, Liu, Yu, Feng, Xu, Zhao and Yang2017), disease diagnosis (Prasannakumar et al., Reference Prasannakumar, Chander and Sahoo2014), phenology derivation (Lausch et al., Reference Lausch, Salbach, Schmidt, Doktor, Merbach and Pause2015), species identification (Moreno et al., Reference Moreno, Corona, Lendasse, Grana and Galvao2014), nutrient concentration (Mahajan et al., Reference Mahajan, Pandey, Sahoo, Gupta, Datta and Kumar2017), biomass assessment, and pigment content (Inoue et al., Reference Inoue, Guerif, Baret, Skidmore, Gitelson, Schlerf, Darvishzadeh and Olioso2016). In order to show the application of HRS in retrieving these parameters more clearly, a chronological diagram was drawn (Figure 1). In summary, pigment content and nutrient concentration were the most common theme in HRS for crop monitoring over decades (Table 3). Over time, the applications of HRS in crop monitoring have been growing and the contents have become more diversified and quantitative. Especially in recent years, the applications of HRS focus on emerging agricultural research domains, such as the heavy metal detecting and water content retrieving. However, most of the study cases were based on ground remote sensing platforms, and there were almost no airborne or spaceborne remote sensing platforms.
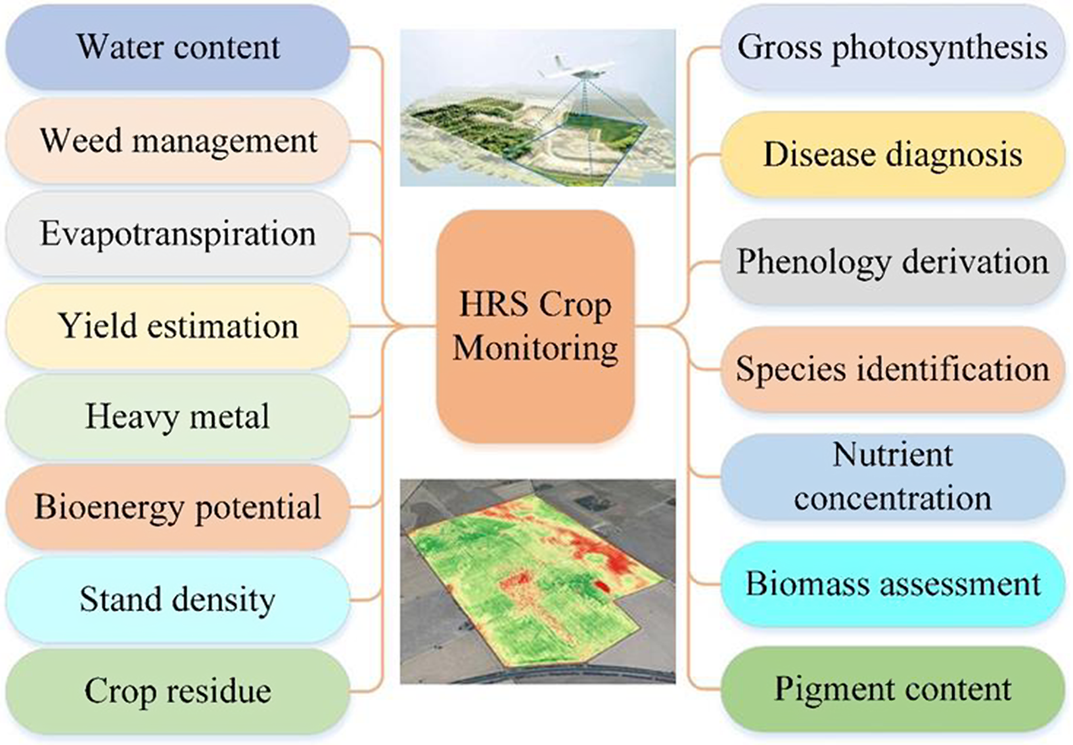
Figure 1. Applications of HRS in retrieving crop parameters (HRS: hyperspectral remote sensing).
Diversified extraction models and methods
It can be seen from the study cases in the past 20 years that various methods or models were used to extract crop parameters (Table 3). In order to clearly show the applications of methods or models, the network diagrams reveal the relationships between applied methods or models with monitoring objects, crop parameters, and monitoring platforms that were explored, respectively. As the three most widely distributed crops, corn, wheat, and rice had received extensive attention; therefore, many methods or models were involved (Xu et al., Reference Xu, Nie, Jin, Li, Zhu, Xu, Wang, Zhao and Feng2021) (Figure 2). A large number of methods or models had been applied to extract nutrient concentration, pigment content, and water content, which also showed that these parameters were concerned by researchers (Figure 3). Because most of the study cases were based on the ground monitoring platform, there were few methods applied to the airborne platform, especially to the spaceborne platform (Figure 4). These methods or models can be summarized into four main categories: linear analysis, non-linear analysis, image classification, and physical model. Linear analysis was the most commonly used method, but physical models were rarely used. A large number of methods reveal that the use of HRS technology for crop monitoring has received widespread attention (Figure 5). However, so many options confuse people in choosing models or methods, which indicates that there is still a need to explore universal quantitative models or methods and extensively apply them to practical work to test the actual effect.
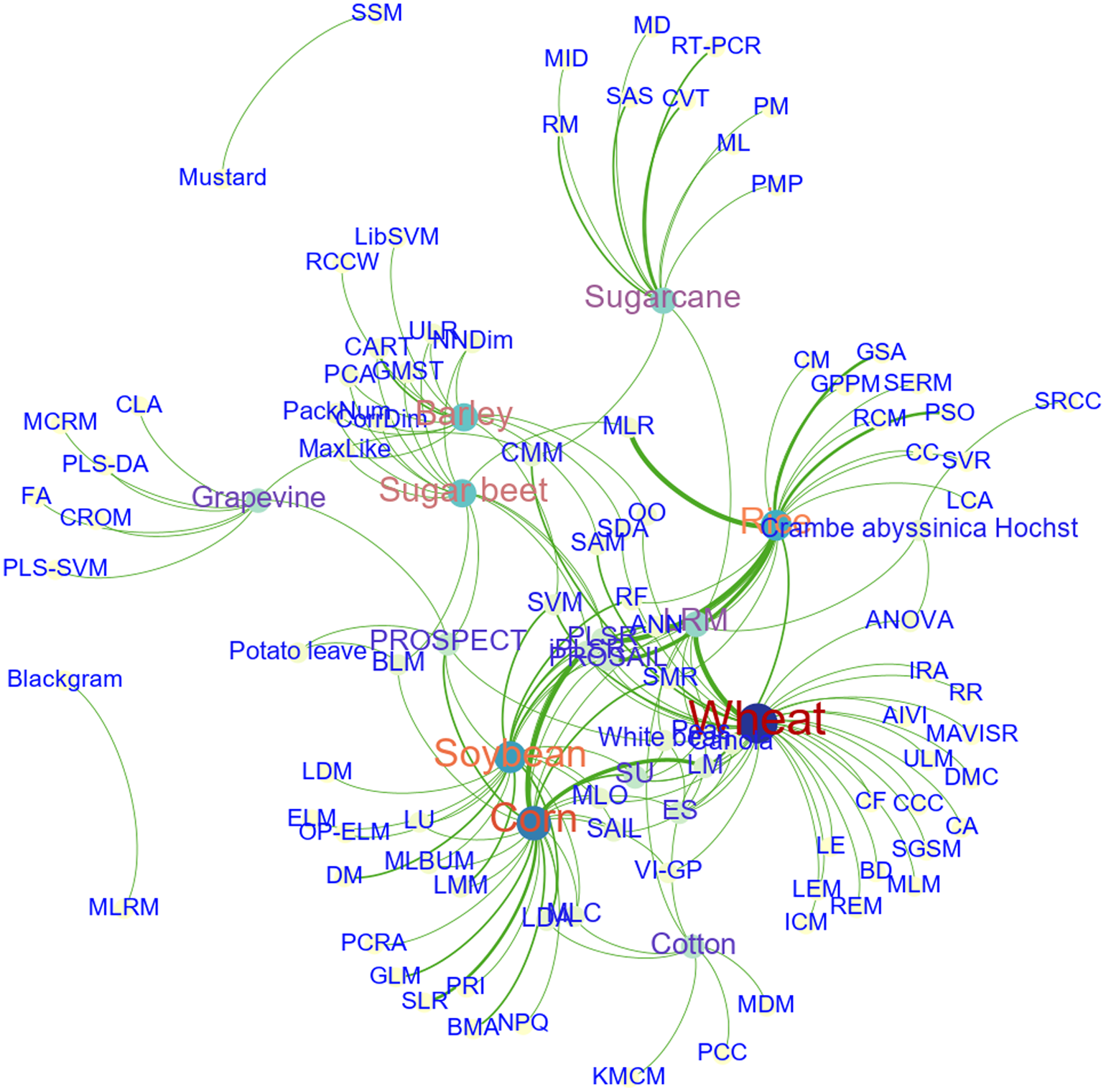
Figure 2. The relationships between objects and applied methods or models.
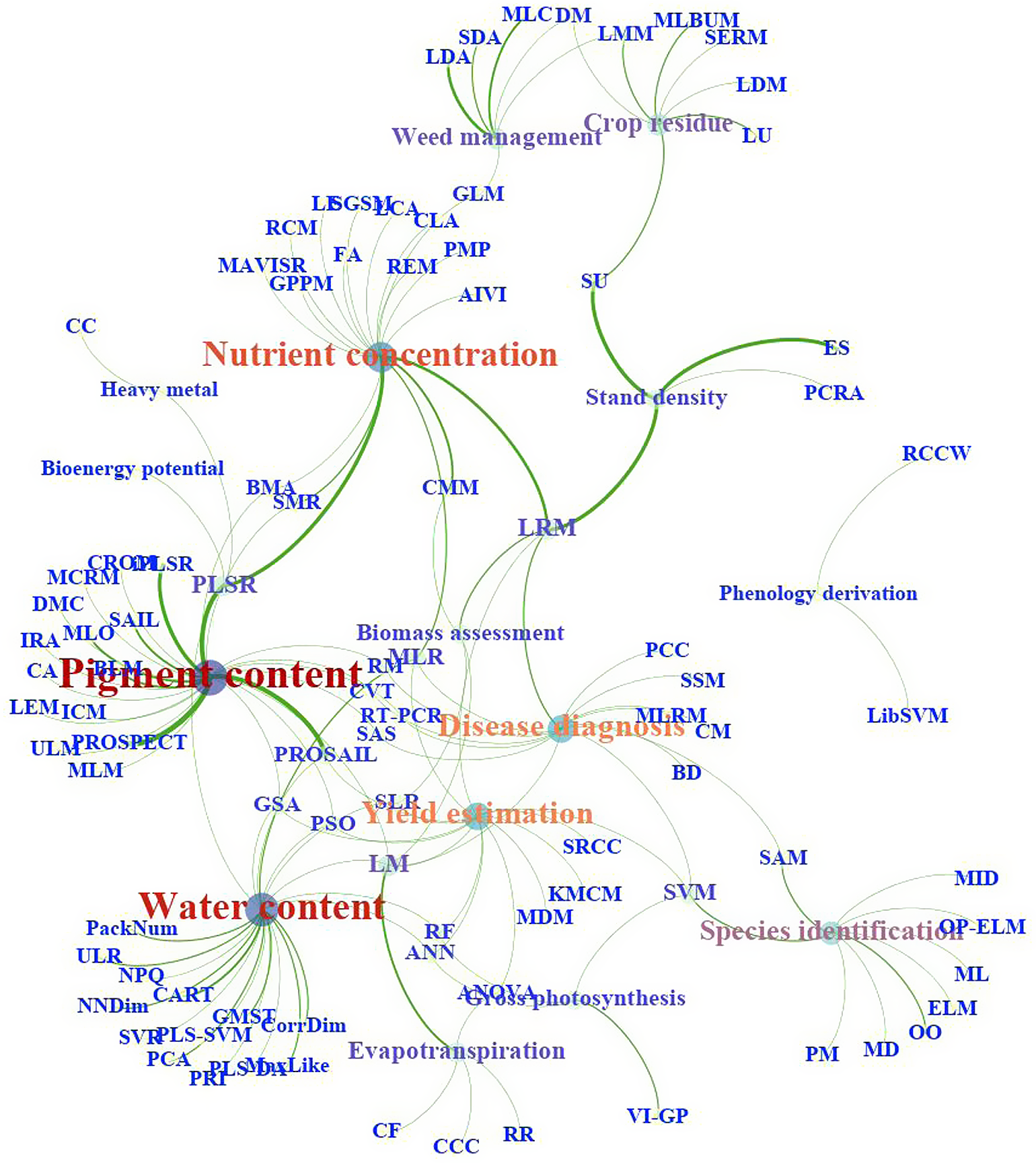
Figure 3. The relationships between parameters and applied methods or models.
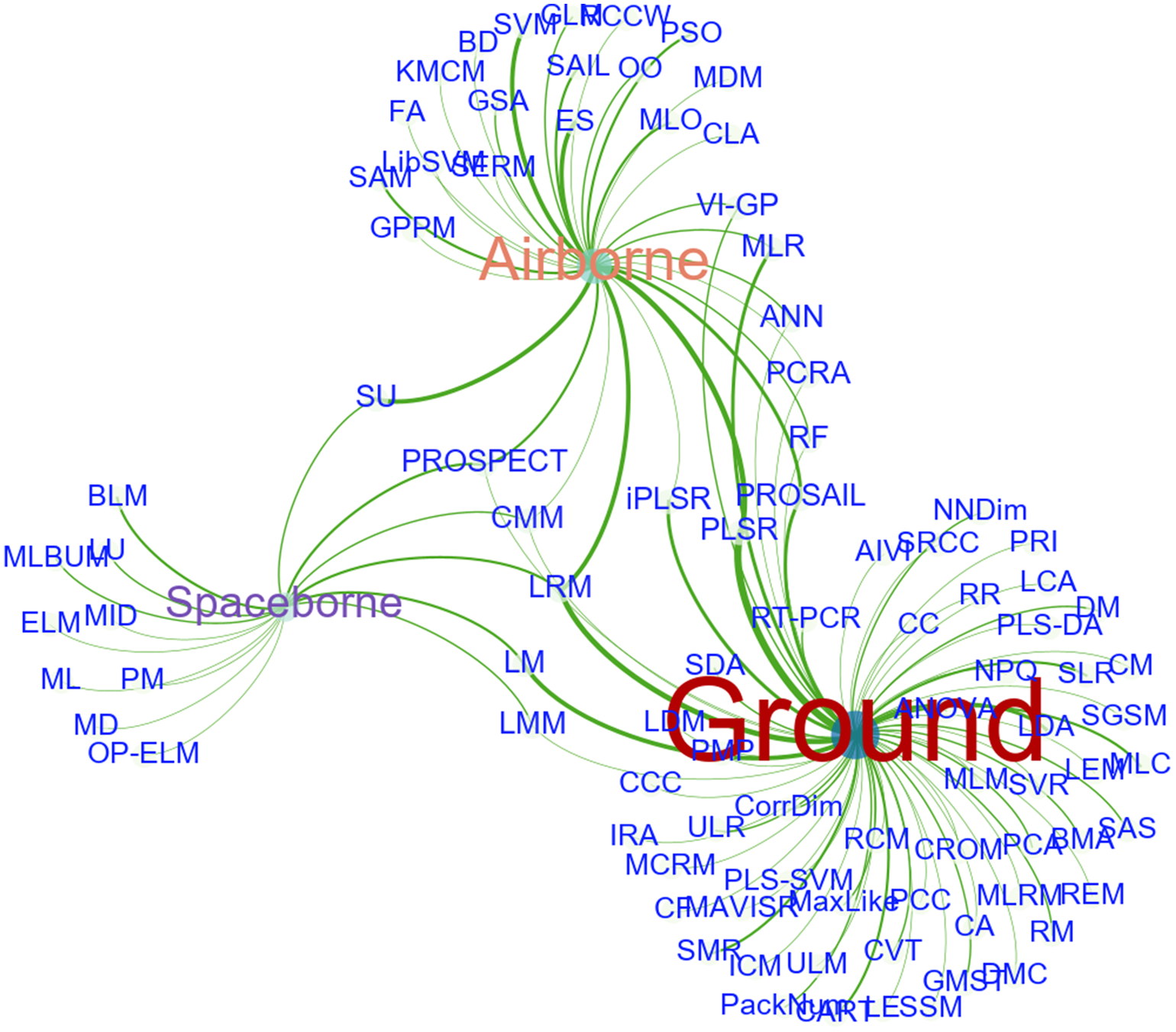
Figure 4. The relationships between platforms and applied methods or models.
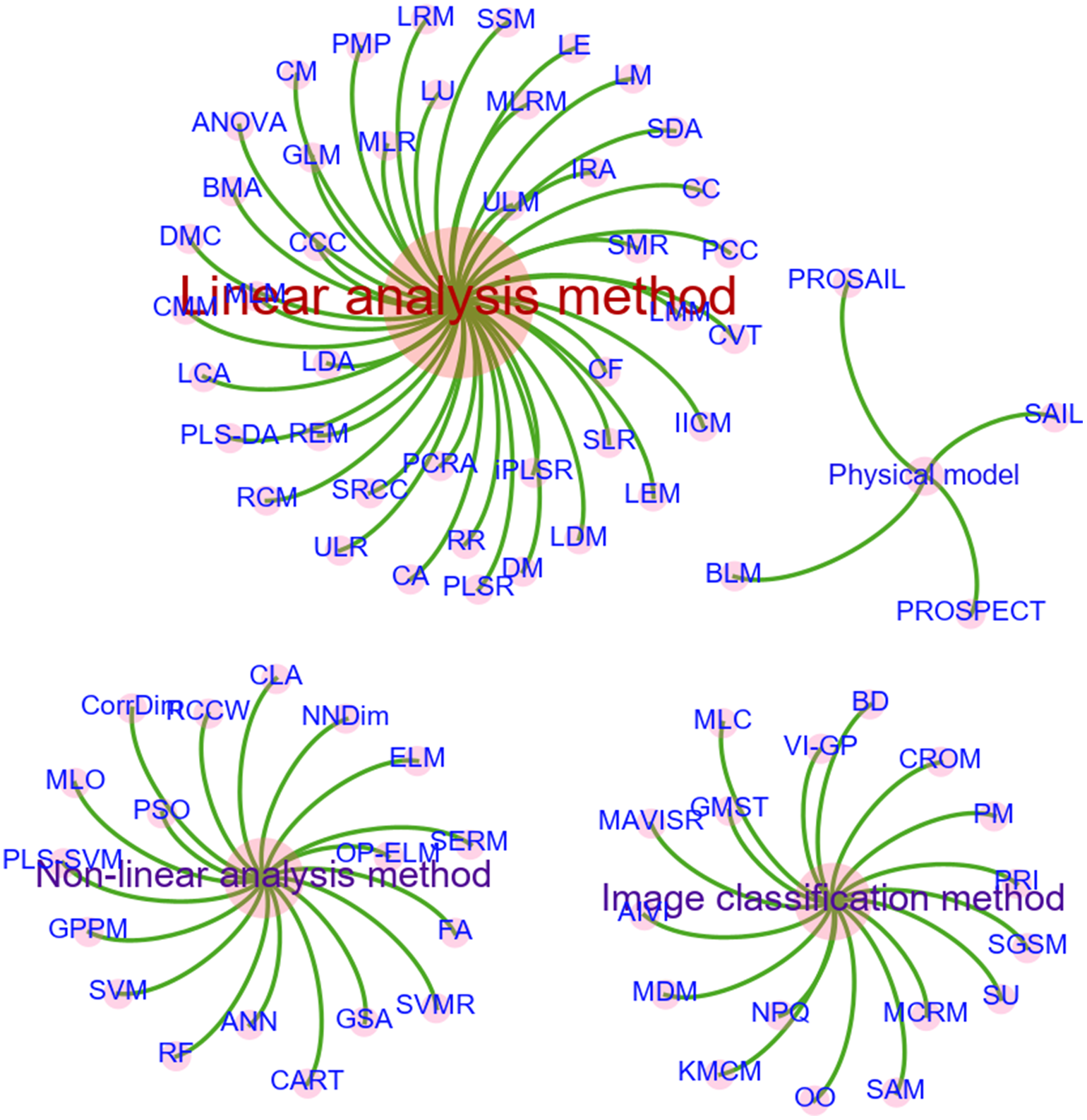
Figure 5. Classification of methods or models by features.
Opportunities and challenges
Although HRS provides new insights of its theory and methodology for studying the crop parameters, there is much work that has to be done both experimentally and theoretically before we can really understand the physical and chemical processes predicting these crop parameters. For example, in terms of data acquisition, the limitations are sensor calibration required; changes in ambient light conditions influence signal and need frequent white reference calibration; and canopy structure and camera geometries or sun angle influence signal. How to effectively achieve HRS data mining, information extraction, high-efficiency data compression and high-speed data transmission is one of the important issues to be solved in the future.
As the resolution of HSI remote sensing sensors increases, more and more dimensions of information are obtained and the volume of data shows significant growth. The limit of employing HRS is the large volume of data that can be generated from spectral images. The large amount of data can make data management particularly important. While acquiring large amounts of hyperspectral image data, one is faced with the problem of how to maximize the use of these massive data. Although some progress has been made in the technology of hyperspectral data classification and information extraction, it still lags behind the development of sensors in general. Therefore, there is still a long way for research on hyperspectral data classification and information extraction.
The current HRS processing methods are particularly rich. On the one hand, new data mining technologies such as deep learning provide opportunities for efficient processing of large amounts of HRS data. Deep learning proposes a method for computer to automatically learn pattern features and incorporates feature learning into the process of model building, thus reducing the incompleteness caused by artificial design features. The findings show that machine learning applications using deep learning as the core method have achieved excellent recognition or classification performance beyond existing algorithms. On the other hand, in view of the huge amount of HRS data, we must extract more effective feature parameters for crop monitoring from the original measurement data. There are two ways to realize this process: band selection and feature extraction. We all know that we should choose those bands with more information, less data correlation, large spectral difference of crop parameters and good separability as the best working bands, and develop feature extraction indexes or methods that are easy to implement with higher extraction accuracy. However, due to the different research objects and regions, the best spectral parameters or characteristic indices of the same crop attribute are also different. Most of the existing spectral indices are based on limited data sets, which makes the monitoring model still lack universality in the selection and application of characteristic parameters.
Specific methods are widely used in building models, including principal component regression analysis, multiple linear regression, partial least-squares regression, stepwise multiple regression, univariate linear method, multivariate linear model, linear correlation analysis, etc. Some studies have explored the methods of nonlinear mathematical analysis, such as support vector machines, nonlinear classification and regression trees technique and artificial neural networks technology. These nonlinear methods can compensate for the shortcomings of linear methods to some extent and improve the prediction accuracy of the model. On the basis of these studies, we should try new artificial intelligence modeling methods such as linear and nonlinear coupling, machine learning and explore their application in crop monitoring. Furthermore, the development from empirical model to physical model will improve the universality and robustness of the model. In addition, in the application of HRS for acquiring crop parameters, more attention should be paid to the application of multivariate models involving multiple parameters, rather than to the single-factor model.
Crop spectra have not only high similarity and spatial variability, but also strong temporal dynamics. The spectral variation of crop parameters with time will be more distinct due to the influence of seasonal characteristics of vegetation. Specifically reflected in the vegetative growth and reproductive growth stages, as well as the staggered stage of vegetative and reproductive growth will cause changes in physical and chemical components and their contribution to spectral reflectance differences. Therefore, by making full use of the advantages of HRS in distinguishing subtle differences of the surface and combining with the temporal and dynamic characteristics of crop parameters, the accuracy of detecting and monitoring of crop parameters will be greatly improved. Further data accumulation and methodological exploration are, however, required to achieve monitoring of crop parameters considering the dynamic process of vegetation growth due to the redundancy of the spectral resolution and the limitation of the temporal resolution of the HRS data, as well as the complexity of the associated models.
With the successful development and launch of ground-based, airborne and spaceborne hyperspectral sensors worldwide, it is easier to obtain reliable and time-sensitive hyperspectral data of the land surface. Moreover, the new features of high spatial resolution, high spectral resolution, and high temporal resolution of HRS technology have become more and more obvious. However, optical HRS means may be affected by many factors such as dust, rust, plowing, particle size distribution, and vegetation coverage, which makes single type of optical HRS data is rather limited and problematic when striving for quantitatively accurate information. Sensors with different working modes and wavelength ranges can provide a variety of detecting means and methods, which can form complementary information to improve monitoring accuracy. For this reason, multidata fusion and multiscale data assimilation will become another research hotspot in HRS monitoring of crop parameters. Fusing hyperspectral data with other types of sensor data, such as Li DAR, SAR, and high spatial resolution images, will have wide application prospects if it can expand the monitoring range or improve the detection accuracy. In addition, with the continuous improvement of temporal and spatial resolution of HRS data and the diversity of data acquisition ways (e.g., ground-based, air borne, and spaceborne), HRS data assimilation has great potential for application. Multiscale data assimilation will improve the monitoring accuracy of land surface process and promote the comprehensive application of multiresolution (temporal, spatial, and spectral) HRS data in agricultural science.
Spaceborne hyperspectral sensors are clearly less used than airborne hyperspectral sensors. Since remote sensing satellite hyperspectral data provide simultaneous views and repeat coverage, two important advantages over ground-based observations and airborne hyperspectral data, research into the potential of satellite hyperspectral data for crop monitoring has become an important issue. Furthermore, the estimation of crop parameters using airborne and satellite hyperspectral sensors is currently limited mainly to small agricultural areas and is still in the testing phase, mainly because the relatively high cost of HSI cameras does not allow for widespread use, which is one of the important issues to be addressed by HRS applications. In addition, the modeling technique used for crop monitoring in most of the previous studies was the simple statistical method, which has several drawbacks related to the physical interpretation of the results and the complexity of transferring the models from one sensor to another (Gomez et al., Reference Gomez, Rossel and McBratney2008; Peón et al., Reference Peón, Fernández, Recondo and Calleja2017). Laboratory and airborne imaging spectroscopy of crop have shown to have considerable potential for the estimation of crop parameters with promising results. However, only a few studies exist that determine crop parameters directly from satellite hyperspectral imagery. Although this approach holds great potential for digital crop mapping with satellite hyperspectral imagery, crop parameter assessment from image data acquired by spaceborne systems is a more difficult issue, mainly due to atmospheric distortions and lower spatial and spectral resolution of the sensors (Mulder et al., Reference Mulder, de Bruin, Schaepman and Mayr2011). In order to be able to fully exploit data from forthcoming hyperspectral satellites, information on several issues related to sensor spatial and spectral resolution and range, as well as on calibration and validation issues, is still required. The development of more physically based models in this context would offer a real step forward towards the generalization of the estimation approaches, but at present still seems an elusive objective (Casa et al., Reference Casa, Castaldi, Pascucci, Palombo and Pignatti2013).
Acknowledgements
This research was funded by the National Natural Science Fundation of China (grant no. 41971226; 41871357), National Basic Research Program of China (2017YFC1503103), and the Strategic Priority Research Program of the Chinese Academy of Sciences (XDA19030303, XDA28110503).
Conflict of Interest
The authors have declared no conflict of interest.