46 results
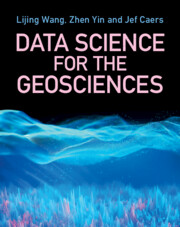
Data Science for the Geosciences
-
- Published online:
- 25 August 2023
- Print publication:
- 17 August 2023
-
- Textbook
- Export citation
Integrating Data Across Misaligned Spatial Units
-
- Journal:
- Political Analysis / Volume 32 / Issue 1 / January 2024
- Published online by Cambridge University Press:
- 23 March 2023, pp. 17-33
-
- Article
-
- You have access
- Open access
- HTML
- Export citation
Spatial relationship between clay content and geophysical data
-
- Journal:
- Clay Minerals / Volume 45 / Issue 2 / June 2010
- Published online by Cambridge University Press:
- 09 July 2018, pp. 197-207
-
- Article
- Export citation
Spatial Relationships Between Seedbank and Seedling Populations of Common Lambsquarters (Chenopodium album) and Annual Grasses
-
- Journal:
- Weed Science / Volume 44 / Issue 2 / June 1996
- Published online by Cambridge University Press:
- 12 June 2017, pp. 298-308
-
- Article
- Export citation
The nature and consequence of weed spatial distribution
-
- Journal:
- Weed Science / Volume 45 / Issue 3 / June 1997
- Published online by Cambridge University Press:
- 12 June 2017, pp. 364-373
-
- Article
- Export citation
Analysis of Spatial Distribution of Common Lambsquarters (Chenopodium album) in No-Till Soybean (Glycine max)
-
- Journal:
- Weed Science / Volume 43 / Issue 2 / June 1995
- Published online by Cambridge University Press:
- 12 June 2017, pp. 258-268
-
- Article
- Export citation
A Web-based GIS Decision Support Tool for Determining Corn Aflatoxin Risk: A Case Study Data from Southern Georgia, USA
-
- Journal:
- Advances in Animal Biosciences / Volume 8 / Issue 2 / July 2017
- Published online by Cambridge University Press:
- 01 June 2017, pp. 718-723
- Print publication:
- July 2017
-
- Article
- Export citation
Determining Corn Aflatoxin Risk within Counties in Southern Georgia, USA using Remotely Sensed Data
-
- Journal:
- Advances in Animal Biosciences / Volume 8 / Issue 2 / July 2017
- Published online by Cambridge University Press:
- 01 June 2017, pp. 640-644
- Print publication:
- July 2017
-
- Article
- Export citation
Infestation and spatial dependence of weed seedling and mature weed populations in corn
-
- Journal:
- Weed Science / Volume 50 / Issue 1 / February 2002
- Published online by Cambridge University Press:
- 20 January 2017, pp. 54-63
-
- Article
- Export citation
Exploratory data analysis to identify factors influencing spatial distributions of weed seed banks
-
- Journal:
- Weed Science / Volume 52 / Issue 6 / December 2004
- Published online by Cambridge University Press:
- 20 January 2017, pp. 936-947
-
- Article
- Export citation
Spatial distribution and mapping of crenate broomrape infestations in continuous broad bean cropping
-
- Journal:
- Weed Science / Volume 49 / Issue 6 / December 2001
- Published online by Cambridge University Press:
- 20 January 2017, pp. 773-779
-
- Article
- Export citation
Multi-species weed spatial variability and site-specific management maps in cultivated sunflower
-
- Journal:
- Weed Science / Volume 51 / Issue 3 / June 2003
- Published online by Cambridge University Press:
- 20 January 2017, pp. 319-328
-
- Article
- Export citation
Spatial Distribution and Temporal Stability of Prostrate Knotweed (Polygonum aviculare) and Corn Poppy (Papaver rhoeas) Seed Bank in a Cereal Field
-
- Journal:
- Weed Science / Volume 57 / Issue 5 / October 2009
- Published online by Cambridge University Press:
- 20 January 2017, pp. 505-511
-
- Article
- Export citation
Sampling strategy is important for producing weed maps: a case study using kriging
-
- Journal:
- Weed Science / Volume 50 / Issue 4 / August 2002
- Published online by Cambridge University Press:
- 20 January 2017, pp. 542-546
-
- Article
- Export citation
Sampling to make maps for site-specific weed management
-
- Journal:
- Weed Science / Volume 53 / Issue 2 / April 2005
- Published online by Cambridge University Press:
- 20 January 2017, pp. 228-235
-
- Article
- Export citation
Spatial and temporal stability of weed populations over five years
-
- Journal:
- Weed Science / Volume 48 / Issue 3 / June 2000
- Published online by Cambridge University Press:
- 20 January 2017, pp. 366-377
-
- Article
- Export citation
Spatial dependence of weed seed banks and strategies for sampling
-
- Journal:
- Weed Science / Volume 50 / Issue 5 / October 2002
- Published online by Cambridge University Press:
- 20 January 2017, pp. 595-606
-
- Article
- Export citation
Spatial distribution, temporal stability, and yield loss estimates for annual grasses and common ragweed (Ambrosia artimisiifolia) in a corn/soybean production field over nine years
-
- Journal:
- Weed Science / Volume 54 / Issue 2 / April 2006
- Published online by Cambridge University Press:
- 20 January 2017, pp. 380-390
-
- Article
- Export citation
An 11-year record of mass balance of Brewster Glacier, New Zealand, determined using a geostatistical approach
-
- Journal:
- Journal of Glaciology / Volume 63 / Issue 238 / April 2017
- Published online by Cambridge University Press:
- 01 December 2016, pp. 199-217
-
- Article
-
- You have access
- Open access
- HTML
- Export citation
Construction of maps for soil recycling in regional infrastructural works integrating soil-quality laws
-
- Journal:
- Netherlands Journal of Geosciences / Volume 79 / Issue 4 / December 2000
- Published online by Cambridge University Press:
- 01 April 2016, pp. 449-457
-
- Article
-
- You have access
- Export citation