23 results
Chapter 4 - Neuropsychological Assessment in Dementia Diagnosis
- from Section 1 - Essential Background Knowledge
-
- Book:
- The Neuropsychology of Dementia
- Published online:
- 25 October 2024
- Print publication:
- 17 October 2024, pp 45-58
-
- Chapter
- Export citation
6 - Central Tendency
- from Part 2
-
- Book:
- Elementary Statistics for Public Administration
- Published online:
- 01 November 2024
- Print publication:
- 12 September 2024, pp 115-132
-
- Chapter
- Export citation
Chapter Three - Measures of Location, Spread, and Skewness
-
- Book:
- Statistics Using R
- Published online:
- 07 December 2023
- Print publication:
- 07 December 2023, pp 72-109
-
- Chapter
- Export citation
Chapter Three - Measures of Location, Spread, and Skewness
-
- Book:
- Statistics Using Stata
- Published online:
- 26 January 2024
- Print publication:
- 30 November 2023, pp 75-118
-
- Chapter
- Export citation
2 - Basics
-
- Book:
- Introduction to Environmental Data Science
- Published online:
- 23 March 2023
- Print publication:
- 23 March 2023, pp 19-64
-
- Chapter
- Export citation
10 - Statistical Methods in Cosmology and Astrophysics
- from Part III - The Later Universe
-
- Book:
- A Course in Cosmology
- Published online:
- 31 March 2023
- Print publication:
- 09 March 2023, pp 229-266
-
- Chapter
- Export citation
1 - Basic Concepts in Probability and Statistics
-
- Book:
- Statistical Methods for Climate Scientists
- Published online:
- 03 February 2022
- Print publication:
- 24 February 2022, pp 1-29
-
- Chapter
- Export citation
3 - Static Systems: Probabilistic Input Uncertainty
-
- Book:
- Large-Scale System Analysis Under Uncertainty
- Published online:
- 17 January 2022
- Print publication:
- 17 February 2022, pp 54-90
-
- Chapter
- Export citation
Bounds on the mean and squared coefficient of variation of phase-type distributions
- Part of
-
- Journal:
- Journal of Applied Probability / Volume 58 / Issue 4 / December 2021
- Published online by Cambridge University Press:
- 22 November 2021, pp. 880-889
- Print publication:
- December 2021
-
- Article
- Export citation
Chapter 9 - The Ethos of a Theologian
- from Part II - Prominent Ethical Views of the Time
-
-
- Book:
- The Reception of Greek Ethics in Late Antiquity and Byzantium
- Published online:
- 15 June 2021
- Print publication:
- 24 June 2021, pp 161-173
-
- Chapter
- Export citation
5 - Means
-
- Book:
- Entropy and Diversity
- Published online:
- 21 April 2021
- Print publication:
- 22 April 2021, pp 133-168
-
- Chapter
- Export citation
Introduction
-
- Book:
- Entropy and Diversity
- Published online:
- 21 April 2021
- Print publication:
- 22 April 2021, pp 1-14
-
- Chapter
- Export citation
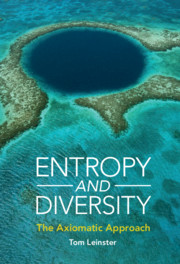
Entropy and Diversity
- The Axiomatic Approach
-
- Published online:
- 21 April 2021
- Print publication:
- 22 April 2021
3 - Models of Central Tendency and Variability
-
- Book:
- Statistics for the Social Sciences
- Published online:
- 24 December 2020
- Print publication:
- 17 December 2020, pp 36-61
-
- Chapter
- Export citation
Chapter 11 - Harmonics as Theological Paradigm in Proclus
-
-
- Book:
- Music and Philosophy in the Roman Empire
- Published online:
- 27 November 2020
- Print publication:
- 17 December 2020, pp 242-261
-
- Chapter
- Export citation
1 - Basic Statistical Terms, Sample Statistics
-
- Book:
- Biostatistics with R
- Published online:
- 18 September 2020
- Print publication:
- 30 July 2020, pp 1-18
-
- Chapter
- Export citation
Chapter 10 - Description and Preliminary Analysis of Quantitative Data
- from Part III - Implementation
-
- Book:
- Research Methods in Business Studies
- Published online:
- 17 January 2020
- Print publication:
- 12 March 2020, pp 182-209
-
- Chapter
- Export citation
Chapter 10 - Description and Preliminary Analysis of Quantitative Data
- from Part III - Implementation
-
- Book:
- Research Methods in Business Studies
- Published online:
- 17 January 2020
- Print publication:
- 12 March 2020, pp 182-209
-
- Chapter
- Export citation
4 - Dealing with Uncertainties
-
- Book:
- Experimental Methods for Science and Engineering Students
- Published online:
- 24 August 2019
- Print publication:
- 05 September 2019, pp 59-84
-
- Chapter
- Export citation
DECADAL CLIMATE VARIABILITY IMPACTS ON CLIMATE AND CROP YIELDS
-
- Journal:
- Journal of Agricultural and Applied Economics / Volume 51 / Issue 1 / February 2019
- Published online by Cambridge University Press:
- 13 September 2018, pp. 104-125
-
- Article
-
- You have access
- Open access
- HTML
- Export citation