Book contents
- Downscaling Techniques for High-Resolution Climate Projections
- Downscaling Techniques for High-Resolution Climate Projections
- Copyright page
- Contents
- Preface
- 1 Impacts, Adaptation, Vulnerability, and Decision-Making
- 2 Global Climate Models
- 3 Assessing Climate-Change Impacts at the Regional Scale
- 4 Dynamical Downscaling
- 5 Empirical-Statistical Downscaling
- 6 Added Value of Downscaling
- 7 Uncertainty in Future Projections, and Approaches for Representing Uncertainty
- 8 Guidance and Recommendations for Use of (Downscaled) Climate Information
- 9 The Future of Regional Downscaling
- References
- Index
- References
References
Published online by Cambridge University Press: 05 February 2021
- Downscaling Techniques for High-Resolution Climate Projections
- Downscaling Techniques for High-Resolution Climate Projections
- Copyright page
- Contents
- Preface
- 1 Impacts, Adaptation, Vulnerability, and Decision-Making
- 2 Global Climate Models
- 3 Assessing Climate-Change Impacts at the Regional Scale
- 4 Dynamical Downscaling
- 5 Empirical-Statistical Downscaling
- 6 Added Value of Downscaling
- 7 Uncertainty in Future Projections, and Approaches for Representing Uncertainty
- 8 Guidance and Recommendations for Use of (Downscaled) Climate Information
- 9 The Future of Regional Downscaling
- References
- Index
- References
Summary
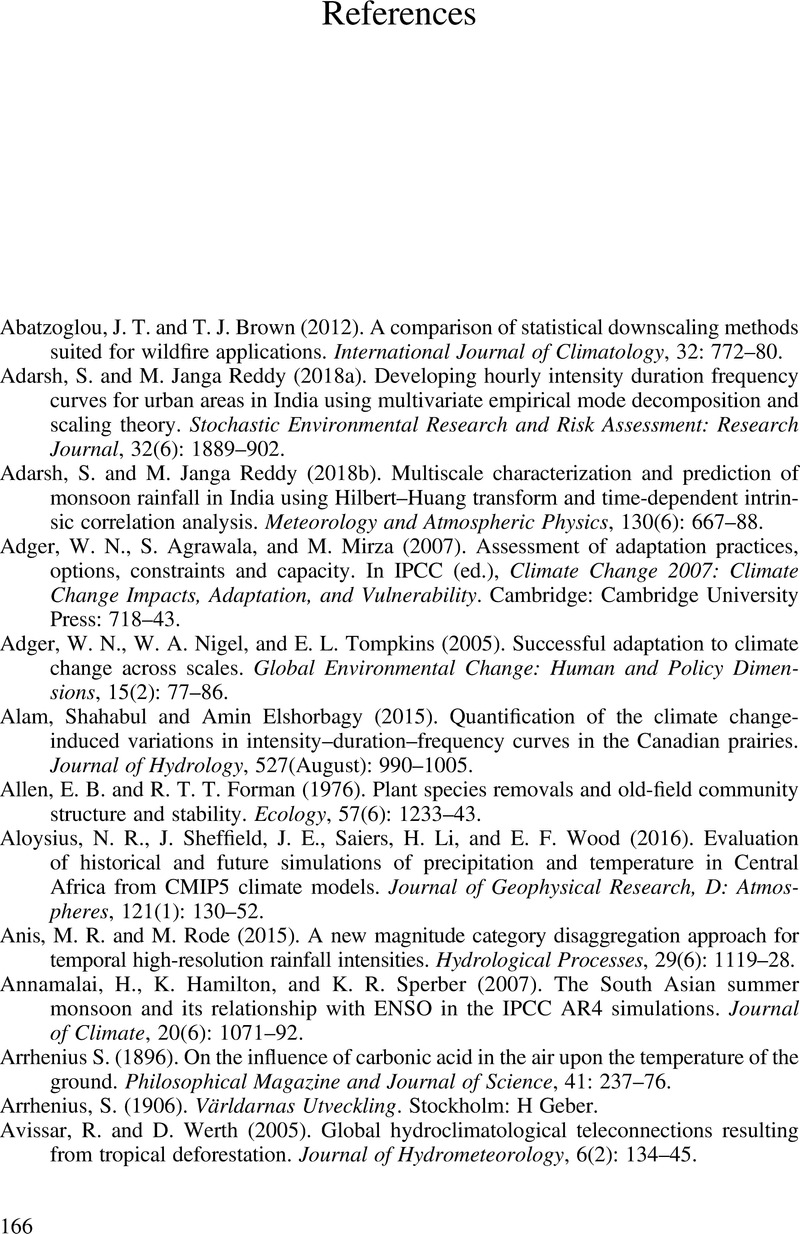
- Type
- Chapter
- Information
- Downscaling Techniques for High-Resolution Climate ProjectionsFrom Global Change to Local Impacts, pp. 166 - 187Publisher: Cambridge University PressPrint publication year: 2021