Introduction
Global biodiversity is in decline, and intensive agricultural land use is one of the most important threats to species (Cole et al. Reference Cole, Elliott and Strobl2021; Dasgupta Reference Dasgupta2021). One policy option to conserve species in agricultural landscapes is the implementation of incentive-based instruments (Khanna et al. Reference Khanna, Swinton and Messer2018) such as payments for ecosystem services (PES) or – as they are often referred to in an agricultural context – agri-environment schemes (AES) (Chakrabarti et al. Reference Chakrabarti, Chase, Strong and Swallow2019; Dakpo et al. Reference Dakpo, Latruffe, Desjeux and Jeanneaux2021; Lichtenberg Reference Lichtenberg2021; Duke et al. Reference Duke, Johnston, Shober and Liu2022). An AES typically contains various conservation measures defined by restrictions on land use and measure-specific payments (Mennig and Sauer Reference Mennig and Sauer2020). The decision problem of the farmers is to select the land use that maximizes profits, which implies the decision of whether or not to participate in the AES and – if the AES offers the farmer the opportunity to select between different measures – which conservation measure to implement (Ohl et al. Reference Ohl, Drechsler, Johst and Wätzold2008).
For economists, a key criterion to assess the suitability of an AES is its cost-effectiveness (Messer Reference Messer2006; Armsworth et al. Reference Armsworth, Acs, Dallimer, Gaston, Hanley and Wilson2012; Duke et al. Reference Duke, Dundas and Messer2013; Bartkowski et al. Reference Bartkowski, Droste, Ließ, Sidemo-Holm, Weller and Brady2021). Previous research has examined the cost-effectiveness of conservation measures, taking into account that both the benefit and costs of a measure are spatially heterogeneous and differ between different conservation measures (Lewis et al. Reference Lewis, Plantinga, Nelson and Polasky2011; Duke et al. Reference Duke, Dundas, Johnston and Messer2014; Wätzold et al. Reference Wätzold, Drechsler, Johst, Mewes and Sturm2016). However, under climate change, initially cost-effective conservation measures may need to be adapted to remain cost-effective (Ando and Mallory Reference Ando and Mallory2012; Pecl et al. Reference Pecl, Araújo, Bell, Blanchard, Bonebrake, Chen, Clark, Colwell, Danielsen, Evengård, Falconi, Ferrier, Frusher, Garcia, Griffis, Hobday, Janion-Scheepers, Jarzyna, Jennings, Lenoir, Linnetved, Martin, McCormack, McDonald, Mitchell, Mustonen, Pandolfi, Pettorelli, Popova, Robinson, Scheffers, Shaw, Sorte, Strugnell, Sunday, Tuanmu, Vergés, Villanueva, Wernberg, Wapstra and Williams2017; Reside et al. Reference Reside, Butt and Adams2018). To develop recommendations for the necessary adaptations, the impact of climate change on both the species and on costs of conservation measures has to be considered (Gerling et al. Reference Gerling, Drechsler, Leins, Keuler, Radtke, Schulz, Sturm and Wätzold2022a).
Research so far has focused on impacts of climate change on the spatial dimension of conservation (Alagador et al. Reference Alagador, Cerdeira and Aráujo2014, Alagador et al. Reference Alagador, Cerdeira and Aráujo2016; Dasgupta Reference Dasgupta2021). Under changing climatic conditions, previously suitable habitat sites may no longer be so. However, other sites – typically sites further poleward or uphill – may become more suitable given these climatic changes (Oliver et al. Reference Oliver, Smithers, Beale and Watts2016). Additionally, the opportunity costs of conservation may change in a spatially heterogeneous manner, as some sites become more suitable for agricultural production (resulting in increasing opportunity costs if agricultural production is restricted for conservation purposes), while others become less productive (Rashford et al. Reference Rashford, Adams, Wu, Voldseth, Guntenspergen, Werner and Johnson2016; Ray et al. Reference Ray, West, Clark, Gerber, Prishchepov and Chatterjee2019; Lachaud et al. Reference Lachaud, Bravo-Ureta and Ludena2021). Previous research has hence focused on the cost-effective provision of habitat sites in this “new climate space” (Vos et al. Reference Vos, Berry, Opdam, Baveco, Nijhof, O’Hanley, Bell and Kuipers2008) and/or on providing migration pathways towards these areas (Alagador et al. Reference Alagador, Cerdeira and Aráujo2014, Alagador et al. Reference Alagador, Cerdeira and Aráujo2016; Gerling et al. Reference Gerling, Drechsler, Leins, Keuler, Radtke, Schulz, Sturm and Wätzold2022a, Gerling et al. Reference Gerling, Schöttker and Hearne2022b).
However, in agricultural landscapes, the temporal dimension also plays an important role as the timing of agricultural land use relative to the timing of a species’ critical life cycle stages has a strong impact on habitat suitability (Wätzold et al. Reference Wätzold, Drechsler, Johst, Mewes and Sturm2016). Changing climatic conditions have complex impacts on the temporal dimension of conservation as they may impact a species inhabiting agricultural landscapes both directly (by altering the climatic conditions of the habitat and hence, the timing of life cycle stages) and indirectly (by inducing changes to the timing of agricultural land use which in turn influence the species). For example, Santangeli et al. (Reference Santangeli, Lehikoinen, Bock, Peltonen-Sainio, Jauhiainen, Girardello and Valkama2018) examine the case of two ground-nesting farmland bird species which used to be well adapted to the timing of land use as they lay their eggs after the farmers have sown the fields. The nests are then not disturbed by any farming operations until the birds have left the nests. Under climate change, the species lay their eggs increasingly early and farmers have also advanced the timing of sowing. However, the adaptation of the birds is stronger than that of farmers, meaning that birds lay their eggs increasingly on unsown fields and nests are likely to be destroyed by the subsequent land use (Santangeli et al. Reference Santangeli, Lehikoinen, Bock, Peltonen-Sainio, Jauhiainen, Girardello and Valkama2018). When the species’ and farmers’ climate change adaptations are of a different magnitude, temporal desynchronizations thus pose an additional, indirect threat to species under climate change.
Temporal desynchronization processes may be relevant for the design of conservation measures as many measures in the context of AES focus on the timing and frequency of land use. A prominent example is biodiversity-enhancing mowing regimes which prohibit mowing during a certain time frame to protect species during their most susceptible life cycle stages (Johst et al. Reference Johst, Drechsler, Mewes, Sturm and Wätzold2015). Such schemes exist all over the world including the USA (Perlut et al. Reference Perlut, Strong and Alexander2011; Chakrabarti et al. Reference Chakrabarti, Chase, Strong and Swallow2019; Allen et al. Reference Allen, Lockwood and Burger2021) and Europe (Kleijn et al. Reference Kleijn, Baquero, Clough, Díaz, De Esteban, Fernández, Gabriel, Herzog, Holzschuh, Jöhl, Knop, Kruess, Marshall, Steffan-Dewenter, Tscharntke, Verhulst, West and Yela2006; Wätzold et al. Reference Wätzold, Drechsler, Johst, Mewes and Sturm2016; Cong et al. Reference Cong, Dupont, Søegaard and Eriksen2020). However, given the fixed timing of harvest restrictions in many AES in Europe (Wätzold et al. Reference Wätzold, Drechsler, Johst, Mewes and Sturm2016) and the USA (e.g., the “haying and grazing practices” according to primary nesting periods of the US Conservation Reserve Program (USDA n.d., USDA 2020)) and possible phenological adaptations of the target species under climate change, the resulting desynchronization may make AES less cost-effective. Regarding cost-effectiveness analysis, one also has to consider that climate change typically leads to a temporal advancement of the profit-maximizing land use (Cui and Xie Reference Cui and Xie2021), implying that the costs of a conservation measure with a fixed timing may change under climate change (Huber et al. Reference Huber, Snell, Monin, Brunner, Schmatz and Finger2017).
In this article, we develop a modelling approach which combines ecological-economic modelling (Polasky et al. Reference Polasky, Nelson, Pennington and Johnson2011; Jiang and Swallow Reference Jiang and Swallow2017, Drechsler et al. Reference Drechsler, Wätzold and Grimm2022) with a climate model to a climate-ecological-economic (CEE) model in order to design a cost-effective AES and determine its necessary adaptations under climate change. To the best of our knowledge, our approach is novel in two ways. It is the first to analyze how to design cost-effective AES under climate change, and it considers not only spatial but also temporal changes in conservation costs and impacts on species due to climate change. With our CEE model, we examine the cost-effective design of an AES consisting of different conservation measures with a specific focus on the timing of land use under recent and future climatic conditions. We apply the model to a case study in Northern Germany in which the conservation measures are extensive mowing regimes with specified mowing dates and frequencies and the conservation objectives are meadow-breeding bird species. We define cost-effectiveness as maximizing an ecological benefit indicator (here, effective habitat area generated for the target species, see section 3.1 for details) for a given (AES) budget constraint in a region that experiences climate change. We specifically model the impact of climate change on both the species and costs and consider two climate scenarios, RCP4.5 (moderate increase in greenhouse gas emissions) and RCP8.5 (large increase in greenhouse gas emissions). We determine the cost-effective set of conservation measures from a list of possible measures under recent (2000–2004) and future (2075–2079) climatic conditions.
We build on previous economic research on biodiversity conservation under climate change. So far, research has focused to a large extent on the cost-effectiveness of conservation measures and policy instruments. Gerling and Wätzold (Reference Gerling and Wätzold2021) analyzed the cost-effectiveness of conservation policy instruments on a conceptual level, and Gerling et al. (Reference Gerling, Drechsler, Leins, Keuler, Radtke, Schulz, Sturm and Wätzold2022a) examined cost-effective conservation measures under climate change for a specific species in a conservation planning context. Considering specific policy instruments, Schöttker and Wätzold (Reference Schöttker and Wätzold2022) investigated the cost-effectiveness of land purchase versus land lease, Huber et al. (Reference Huber, Snell, Monin, Brunner, Schmatz and Finger2017) simulated the outcomes of several conservation measures of an AES, and Gerling et al. (Reference Gerling, Schöttker and Hearne2022b) examined cost-effectiveness gains through increased flexibility of conservation agencies for land purchase and sale. Given the prominence of uncertainty in the context of climate change, another focus of economic research on biodiversity conservation under climate change has been on different approaches of how to deal with risk (Mallory and Ando Reference Mallory and Ando2014; Shah et al. Reference Shah, Mallory, Ando and Guntenspergen2016; Drechsler et al. Reference Drechsler, Gerling, Keuler, Leins, Sturm and Wätzold2021). Further research addressed auctions as an incentive-based instrument for the provision of ecosystem services under climate change (Lewis and Polasky Reference Lewis and Polasky2018) and people’s willingness-to-pay for species migrating under climate change (Lundhede et al. Reference Lundhede, Jacobsen, Hanley, Fjeldså, Rahbek, Strange and Jellesmark2014).
Conservation problem
Case study area
Our case study covers an area of 5,040 km2 in the federal state Schleswig-Holstein in Northern Germany (Figure 1). The area has a maritime climate with a mean annual temperature of 8.3°C between 1961 and 1990. Variation between mean summer and winter temperatures is relatively small, as mean monthly temperatures range from around 0°C (in January) to 16°C (in June). Compared to most other areas in Germany, there are fewer “summer days” (defined as having a maximum temperature of at least 25°C) and fewer “frost days” (defined as having a minimum temperature of less than 0°C). Climate change is expected to lead to a moderate temperature rise in comparison with other areas of Germany, and precipitation is expected to increase in winter and spring and decrease in summer (DWD 2017).

Figure 1. Illustration of the location of the case study area in the North of Germany and its division into 12 × 12 km² climate cells. Map of Germany created with mapchart.net.
The case study area is divided into climate cells of 12 × 12 km² (Figure 1). Furthermore, grassland areas that are used as meadows within the case study area are represented by grassland cells of 250 × 250 m². For each grassland cell, information such as the productivity of the land (measured by the German system of “grassland numbers” (GrünlandzahlFootnote 1 )) and the presence of structural elements such as water bodies is available. Taking into account the land productivity allows for a spatial differentiation of biomass growth (influencing the opportunity costs of conservation), while information on biomass growth and additional information like the presence of structural elements allow for a spatial differentiation of conservation impacts at the spatial scale of grassland cellsFootnote 2 .
Target species
We consider a list of eight bird species that are threatened or likely to become threatened in the near future according to the red list (Knief et al. Reference Knief, Berndt, Hälterlein, Jeromin, Kieckbusch and Koop2010): the black grouse (Tetrao tetrix), black-tailed godwit (Limosa limosa), common redshank (Tringa totanus), common snipe (Gallinago gallinago), meadow pipit (Anthus pratensis), northern lapwing (Vanellus vanellus), skylark (Alauda arvensis), and whinchat (Saxicola rubetra). All species are ground-breeding meadow birds but differ in their breeding period. Furthermore, the species differ in their habitat requirements such as humidity or grass length. We selected these species as they are of conservation interest (due to their red list status), and their habitat requirements are sensitive to the timing of agricultural land use. Moreover, both information on their breeding behavior in the recent past and on reactions to climatic changes were available. Details on the species’ habitat requirements and breeding period can be found in Appendix A1.
Under climate change, the species may adapt phenologically by advancing the timing of typical life cycle events. Regarding bird species, one critical parameter is the beginning of the breeding period (Kluen et al. Reference Kluen, Nousiainen and Lehikoinen2016). However, the phenological adaptations of different bird species are of different magnitude (Kluen et al. Reference Kluen, Nousiainen and Lehikoinen2016). We therefore consider species-specific values regarding the timing and advance of egg deposition of the eight species in the ecological model.
AES framework: selection of conservation measures and budget
In the first period (2000–2004), we consider 11 conservation measures which are candidates for the conservation measures included in the cost-effective AES. These 11 measures were selected by determining the most cost-effective conservation measures for the species using DSS-Ecopay, an existing software-based decision support system to design cost-effective grassland AES to conserve birds and butterflies (Sturm et al. Reference Sturm, Drechsler, Johst, Mewes and Wätzold2018). The measures differ in their frequency and timing of land use (Table 1). The timing of land use is given in quarter months (QM) by dividing each month into four equal parts consisting of approximately 7.5 days each.
Table 1. List of conservation measures. The measure name includes information on the timing and frequency of land use: for example, measure M19/6 allows for a first harvest in quarter month 19, followed by a break of 6 quarter months and a second harvest in quarter month 25

To account for possible phenological adaptations, we consider four additional conservation measures in the second period (2075–2079), M17/6, M17/8, M18/6, and M18/8, which represent shifts of the initially earliest measures (M19/6 and M19/8) by one and two quarter months. We thus have a list of 15 possible conservation measures for the second period.
The budget for each 5-year period is set to 3,725,000€, which would allow for approximately 15% of the grassland area to be conserved if on each grassland cell the most cost-effective conservation measure was implemented. We chose this value as in Schleswig-Holstein, 15% of permanent grasslands were managed with biodiversity-enhancing conservation measures in 2014–2020 (Sander et al. Reference Sander, Bathke and Franz2019) and 15% is also sufficiently large to have a clear visibility of any relevant effects.
Climate-ecological-economic model
Overview of the CEE model
We adapt the CEE model by Gerling et al. (Reference Gerling, Drechsler, Leins, Keuler, Radtke, Schulz, Sturm and Wätzold2022a), which focuses on climate change impacts in a conservation planning context for a grasshopper species, and combine it with an ecological-economic modelling procedure to design cost-effective AES to conserve grassland birds developed by some of the authors in previous work (Wätzold et al. Reference Wätzold, Drechsler, Johst, Mewes and Sturm2016) in order to model an AES context and the mowing impact on bird species. As nearly all components of the applied CEE model are described in other papers, we restrict ourselves here to providing an overview of the model (Figure 2), explaining how we take into account climate change in the ecological-economic modelling procedure (Johst et al. Reference Johst, Drechsler, Mewes, Sturm and Wätzold2015; Wätzold et al. Reference Wätzold, Drechsler, Johst, Mewes and Sturm2016) and otherwise referring the interested reader to the literature. Keuler et al. (Reference Keuler, Radtke, Kotlarski and Lüthi2016) provides detailed information on the climate model, the vegetation model is based on Schippers and Kropff (Reference Schippers and Kropff2001), and details on the harvest module and the agri-economic cost assessment can be found in Gerling et al. (Reference Gerling, Sturm and Wätzold2020). The ecological model of the modelling procedure is explained in Johst et al. (Reference Johst, Drechsler, Mewes, Sturm and Wätzold2015), and the simulation and optimization module in Wätzold et al. (Reference Wätzold, Drechsler, Johst, Mewes and Sturm2016) and Sturm et al. (Reference Sturm, Drechsler, Johst, Mewes and Wätzold2018) (see also Appendix A2).

Figure 2. Structure of the CEE model (adapted from Gerling et al. Reference Gerling, Drechsler, Leins, Keuler, Radtke, Schulz, Sturm and Wätzold2022a). Boxes with dashed borders are taken from Gerling et al. (Reference Gerling, Drechsler, Leins, Keuler, Radtke, Schulz, Sturm and Wätzold2022a), and boxes with solid lines represent modifications.
The basis of the CEE model is the climate model, which generates high-resolution climate projections of parameters like temperature and precipitation using the regional climate model COSMO-CLM (Rockel et al. Reference Rockel, Will and Hense2008; Früh et al. Reference Früh, Will and Castro2016). Climate data is available at a spatial scale of 12 × 12 km² climate cells on a daily basis. We examine two scenarios, which differ in the emission pathways: a medium-emission scenario (RCP4.5) and a high-emission scenario (RCP8.5) (IPCC 2014). We do not consider a low-emission scenario as given current emission reductions, reaching a low-emission scenario seems increasingly unlikely (Sanderson et al. Reference Sanderson, O’Neill and Tebaldi2016). Climate data is used as an input for the vegetation model, which is a simplified model of grass growth based on Schippers and Kropff (Reference Schippers and Kropff2001). The vegetation model provides input to the harvest module and the agri-economic assessment and is needed to determine the costs of conservation measures. Grass growth is determined at the spatial scale of 250 × 250 m² grassland cells.
We then consider the business-as-usual (BAU) land use (i.e., the land use in the absence of an AES) and a list of alternative conservation measures defined by their timing of land use (cp. Table 1). Based on climatic conditions and grass growth, the harvest module estimates the yield-maximizing timing of harvest for the BAU land use and the different conservation measures on each grassland cell. However, the yield-maximizing timing of harvest may not be profit-maximizing. For example, while highly productive grassland may be harvested up to four times, the variable costs may exceed the value of the fourth harvest on less productive grassland.
We consider this in the agri-economic cost assessment, which determines the profit-maximizing timing of harvest for each grassland cell. Given this timing, the second output of the agri-economic cost assessment is grassland cell-specific cost estimates of each conservation measure for both periods (2000–2004 and 2075–2079), that is, the differences in profit between the BAU land use and the conservation measures. We consider changes in variable costs (such as the necessary machinery) and changes in the value of the yield of the BAU land use and the conservation measure. Changes in the yield value depend on the quantity and quality of the harvest (Mewes et al. Reference Mewes, Drechsler, Johst, Sturm and Wätzold2015) and may be impacted by changing climatic conditions (Ray et al. Reference Ray, West, Clark, Gerber, Prishchepov and Chatterjee2019; Gerling et al. Reference Gerling, Sturm and Wätzold2020; Lachaud et al. Reference Lachaud, Bravo-Ureta and Ludena2021). Moreover, we consider the impact of extreme events. In particular, changes in precipitation patterns may lead to increased frequency of flooding before the first harvest as precipitation shifts from summer towards spring (DWD 2017). However, when a meadow is flooded for an extended period of time, the quality of the harvest decreases rapidly (Gerling et al. Reference Gerling, Sturm and Wätzold2020).
The ecological model builds on previous research (Johst et al. Reference Johst, Drechsler, Mewes, Sturm and Wätzold2015) regarding the impact of differently timed conservation measures on meadow bird species with differing habitat requirements. The model is the basis for the assessment of the impact of conservation measures on birds in the software-based decision support system DSS-Ecopay (Sturm et al. Reference Sturm, Drechsler, Johst, Mewes and Wätzold2018). In the ecological model, we determine the local habitat quality generated by each conservation measure for each species and for each grassland cell. The local habitat quality is given as a value between 0 and 1, with 0 representing very low habitat quality (i.e., reproduction is impossible) and 1 representing very high quality (ideal conditions). To determine the local habitat quality, we consider grassland-cell-specific conditions which are independent of the timing of egg deposition (such as the presence of structural elements and the grassland type) and factors that depend on the timing of egg deposition (such as mortality caused by mowing machines). We then sum up the local habitat qualities of all grassland cells in order to determine the total effective habitat area generated in the case study region. This value is thus an indicator of the overall conservation impact of a conservation measure on a species. DSS-Ecopay was used to determine the total effective habitat area.
The simulation and optimization module follows the same logic as the algorithm from Wätzold et al. (Reference Wätzold, Drechsler, Johst, Mewes and Sturm2016). The module is applied to the data sets from the ecological model and the agri-economic cost assessment. It combines information from the agri-economic cost assessment and the ecological model in order to estimate the expected conservation impact and the costs of each conservation measure for each grassland cell under recent (2000–2004) and future (2075–2079) climatic conditions. Using simulated annealing, the optimization tries different payments for each measure in a stepwise process in order to maximize the ecological outcome for a given budget constraint. For each step, the algorithm simulates the farmers’ profit-maximizing decision on which measure to apply considering the payments for the different measures and their costs. The ecological outcome is measured as the sum of the effective habitat areas generated for all species in the landscape, implying that each species is given the same weight.
The final output of the CEE model is the cost-effective AES consisting of a set of conservation measures and their respective payments for the case study area under recent (2000–2004) and future (2075–2079) climatic conditions. We take a period from the recent past (rather than current conditions), as the underlying ecological-economic model (Wätzold et al. Reference Wätzold, Drechsler, Johst, Mewes and Sturm2016) was parametrized based on data and studies from this time. The second period was chosen in order to be able to observe the effects of long-term climate change and due to data availability.
Phenological adaptations in the ecological model
In order to examine the impact of climate change on the bird species, we consider phenological adaptations of the species in terms of the timing of egg deposition. Importantly, the species react differently to climatic changes. Table 2 summarizes the expected adaptations for each species based on values published in the literature. In some cases, values are only available regarding changes in the arrival date of migratory birds. In these cases, we take the adaptation of the arrival date as an indicator for the adaptation of egg deposition.
Table 2. Species-specific phenological adaptations of egg deposition. Adaptations highlighted by an asterisk (*) refer to approximations based on changes in the arrival date

Results
In the RCP4.5 scenario, climate change has only minor impacts on the cost-effectiveness of the AES: in this scenario, the cost-effective AES consists of the same conservation measure in both periods (M27/0), but the payment is increased from 745€/ha to 800€/ha. Furthermore, the species’ phenological adaptations result in an increase in total effective habitat area generated. We therefore focus on the results of the RCP8.5 scenario here and present detailed results of the RCP4.5 scenario in Appendix A3.
In the RCP8.5 scenario, the cost-effective AES consists of only one measure in each period. While in period 1, the two-cut measure M25/6 is included, in period 2 only one cut is allowed (M28/0). The payment of M28/0 is higher (at 587€/ha) than that of M25/6 (374€/ha) as opportunity costs of this measure are higher. The increase in payment size is due to a combination of a less suitable timing (harvesting for the first time in QM28 is very late in comparison with the BAU land use) and a more restrictive land use, as only a single harvest is permitted.
Given that the payment per hectare is higher for the measure selected in period 2, the overall area conserved is smaller by 34%. Figure 3 shows our indicator for the conservation success of the AES, the effective habitat area for each species. Out of the eight species considered in the optimization, six species are conserved in both periods. However, the effective habitat areas for these species in period 2 is smaller than in period 1.

Figure 3. Effective habitat area (ha) generated for each species by each measure in period 1 (2000–2004) and 2 (2075–2079) (RCP8.5).
Figure 4 shows the relative losses in effective habitat area overall and for each species. Whereas 34% of the total effective habitat area are lost in the second period, some species experience larger losses than others. In particular the common redshank loses almost half its original habitat area, while the black-tailed godwit only loses 29%. This is due to the different timing of the reproduction phases of the two species, their habitat requirements and/or their phenological adaptations (see Appendix A5 for details).
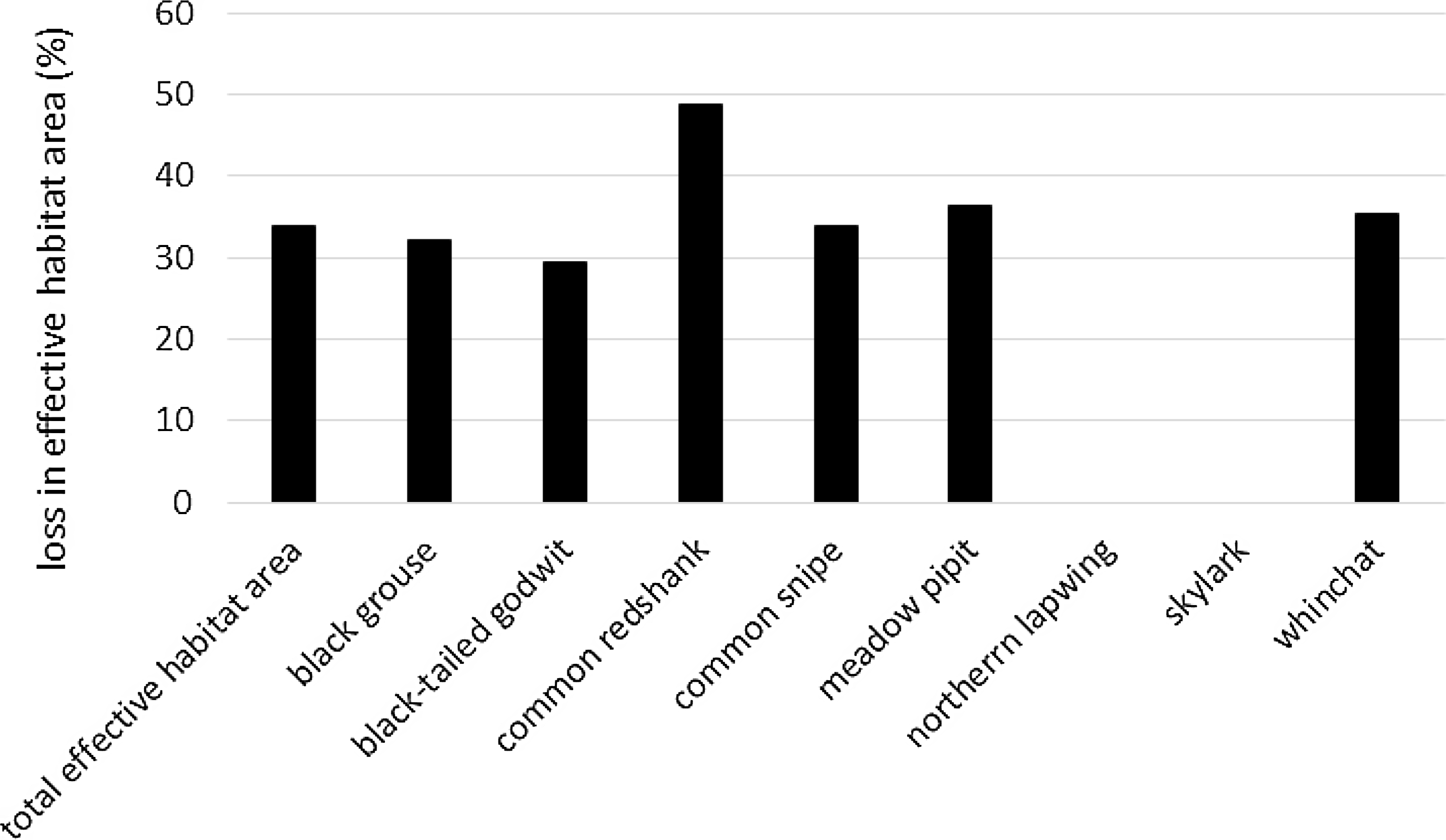
Figure 4. Loss in total effective habitat area (%) and in effective habitat area (%) generated for each species between period 1 (2000–2004) and 2 (2075–2079) (RCP8.5).
Figure 5 shows the spatial distribution of conservation sites in the two periods and the relative losses between the two periods. It can be seen that while in period 1, conservation activities occur more evenly throughout the landscape, they concentrate on fewer climate cells in the center of the case study area in period 2. In particular, climate cells that initially include only few conserved areas are lost (almost) completely in period 2.

Figure 5. Spatial distribution of conservation sites in periods 1 (a), period 2 (b), and relative losses between the two periods (c). Colors represent a continuous scale.
In order to gain a better understanding of the results, we additionally examine whether the change in the selected measure is driven by cost changes or by the species’ adaptations. Regarding costs, Figure 6 shows that the marginal costs of conserving an additional grassland cell with measure M25/6 are markedly higher in period 2 than in period 1. In period 1, this measure is the lowest cost measure (see Appendix A4 for details). Considering measure M28/0, costs in period 2 are only slightly higher than in period 1. Most conservation measures generate higher costs in period 2 than in period 1. However, cost changes differ strongly between measures (see Appendix A4). The reason for these differences lies in the occurrence of extreme events, in particular flooding. In period 2, measure M28/0 is a measure of medium costs and has the lowest costs of those allowing only a single harvest. Moreover, the measures allowing only a single harvest have comparatively low costs in the RCP8.5 scenario (see Appendix A4 for details). Further analyses show that cost changes are relatively homogeneous throughout the landscape (Appendix A4). Overall, cost analyses thus support the shift from measure M25/6 to M28/0: while measure M25/6 is the lowest cost measure in period 1, it has higher costs in period 2. Measure M28/0, which is chosen in period 2, has medium costs but low costs in comparison with other measures that allow only a single harvest.

Figure 6. Marginal costs of conservation measures M25/6 and M28/0 in period 1 (2000–2004) and 2 (2075–2079) (RCP8.5).
We now consider whether ecological outcomes also support the shift from measure M25/6 towards measure M28/0. Figure 7 shows the ecological benefit per measure for both periods. It can be seen that the differences between the two periods are small and spatial analyses show that differences over time remain small throughout the landscape (Appendix A4). However, early measures (with a first harvest until quarter month 20) have a higher ecological benefit in period 2 than in period 1, while measures with a medium timing (with a first harvest between quarter months 21–25) have a higher ecological benefit in period 1. This is due to the phenological adaptations of the species. The measures allowing only a single harvest have a very late cut (starting from quarter month 26), and their impact on the breeding period for the species is equal in both periods (see Appendix A5 for details).

Figure 7. Total effective habitat area if each conservation measure was implemented on all sites in period 1 (2000–2004) and 2 (2075–2079) (RCP8.5).
Moreover, the measures allowing only a single, late harvest have the largest ecological benefits. In particular, measure M28/0 (which is chosen in period 2) has the highest ecological benefit, while it also has the lowest costs out of all conservation measures allowing only a single harvest.
In summary, in period 1, the measure with lowest costs and medium-high benefits is the cost-effective one, whereas in period 2, a measure with medium costs but the highest benefit is chosen. The results are thus triggered by an interplay of economic and ecological parameters, and in particular by the impact of extreme events on costs and the impact of the species’ phenological adaptations on species’ benefits from a conservation measure with a fixed timing of harvest. Extreme events play a smaller role in the RCP4.5 scenario, and hence, there are no changes in the conservation measures selected for the cost-effective AES for the two periods.
Discussion and conclusion
We developed a CEE model to examine the cost-effectiveness of an AES and its necessary adaptations under climate change. To the best of our knowledge, this is the first model investigating the cost-effective design of AES for biodiversity conservation under climate change. A further novelty is that the focus of our paper is on the timing of the conservation measure, whereas most other work concentrates on spatial adaptations. We applied the model to determine the cost-effective AES for a set of meadow birds in Northern Germany. The model considers the impact of climate change on both the species and costs of conservation. Our results show that while under the RCP4.5 scenario, the cost-effective AES changes only in terms of the payment size, in the RCP8.5 scenario the cost-effective AES also changes in terms of the conservation measure included in the AES.
This change occurs due to the impact of climate change on both conservation costs and benefits. Regarding costs, we find a high variability of conservation costs due to extreme events (inundations) to play a key role in causing relative cost advantages for the selected conservation measure under climate change. Moreover, this conservation measure also provides large ecological benefits due to the late timing of land use, and the phenological adaptations of the species further increase the relative benefit of this measure compared to other measures of similar costs. The change in conservation measures in the cost-effective AES may therefore be explained by the impact of extreme events on conservation costs and the species’ phenological adaptations to climate change. Apart from the specific results for the case study, our research also allows us to derive two general insights and policy recommendations regarding the design of AES under climate change.
First, our results provide an indication that conservation measures need to be adapted to climate change with respect to the temporal dimension. Current policy documents such as the Strategic Plan for Responding to Accelerating Climate Change (US Fish and Wildlife Service 2010), the EU Biodiversity Strategy for 2030 (The European Commission 2021), and the German Adaptation Strategy to Climate Change (Die Bundesregierung 2008) focus on the spatial dimension, emphasizing the need to adapt the location of conservation sites due to species’ range shifts. However, the need to also adapt the temporal restrictions of conservation measures is so far rarely considered in policy strategies. Our results show that neglecting the temporal dimension reduces the cost-effectiveness of conservation given climatic changes. This is relevant for any species that would be negatively affected by mechanical land use directly – apart from ground-breeding meadow birds, this may also include species such as butterflies (Johst et al. Reference Johst, Drechsler, Mewes, Sturm and Wätzold2015) and grasshoppers (Leins et al. Reference Leins, Banitz, Grimm and Drechsler2021) among others. Additionally, the timing of land use may influence species indirectly by creating certain habitat types (Johst et al. Reference Johst, Drechsler, Mewes, Sturm and Wätzold2015), ensuring the availability of feeding plants (e.g., the availability of milkweed for monarch butterflies (Thogmartin et al. Reference Thogmartin, López-Hoffman, Rohweder, Diffendorfer, Drum, Semmens, Black, Caldwell, Cotter, Drobney, Jackson, Gale, Helmers, Hilburger, Howard, Oberhauser, Pleasants, Semmens, Taylor, Ward, Weltzin and Wiederholt2017)), or providing suitable habitat during migration phases (e.g., for migrating shorebirds along the Pacific Flyway in California (Golet et al. Reference Golet, Low, Avery, Andrews, McColl, Laney and Reynolds2018)). One simple way of adapting conservation measures automatically may be by defining their timing phenologically (e.g., in relation to the phenological beginning of spring), rather than setting fixed dates. Gerling et al. (Reference Gerling, Drechsler, Leins, Keuler, Radtke, Schulz, Sturm and Wätzold2022a) have shown that such measures may remain cost-effective under changing climatic conditions for the conservation of the large marsh grasshopper in Northern Germany, but whether this finding can be generalized requires further analysis.
Second, our results highlight that the increase of extreme events under climate change needs to be considered in the design of cost-effective AES. In our case, extreme events, specifically flooding, have a substantial impact on costs as the harvest during certain periods of the year becomes less valuable or unusable. Previous research has considered the impact of climate change on opportunity costs due to changes in the vegetation period (Huber et al. Reference Huber, Snell, Monin, Brunner, Schmatz and Finger2017), while our results show that extreme events may also play an important role. However, climate projections only inform about the increasing probability of the occurrence of extreme events, while the exact years in which they occur are uncertain. One way of dealing with this uncertainty are robustness analyses, which allow to identify robust conservation strategies given uncertain climate change (Mallory and Ando Reference Mallory and Ando2014; Shah et al. Reference Shah, Mallory, Ando and Guntenspergen2016; Drechsler et al. Reference Drechsler, Gerling, Keuler, Leins, Sturm and Wätzold2021). In this way, one could identify conservation measures which are relatively cost-effective even in years of extreme events, rather than choosing conservation measures with the highest expected outcomes, which, however, may have a very low cost-effectiveness in certain years. This would reduce the variability of ecological outcomes and/or costs over time. The important influence of extreme events on the cost-effective design of the AES in our case study thus provides further indications for including robustness considerations in the design of conservation schemes.
In order to simplify complex processes and due to data limitations, we had to make some assumptions which require a discussion. Regarding the ecological model, we had to reduce the complexity of the possible adaptations of the species to climate change by not considering phenological changes in life cycle parameters driven by climate change other than the beginning of the breeding period (e.g., changes in the reproductive success of birds due to a mismatch between the hatching timing and peak nutritive quality of plants (Reséndiz-Infante and Gauthier Reference Reséndiz-Infante and Gauthier2020)). Given that we focus on the desynchronizations between the timing of harvest and the species’ breeding period, we expect our results to nonetheless highlight valuable trends. Further simplifications within the ecological model include the assumptions that changes to the arrival date are an indicator for the timing of egg deposition and that estimates from studies from a range of countries also hold for Northern Germany.
Furthermore, we simplified the interactions between the ecological model and other submodels to limit computation time: local humidity and grass length influence the suitability of a grassland cell for the species, and we consider spatial differences in these parameters between different grassland cells. However, climate change may also influence these parameters over time, for example, by changing the dynamics of grass growth (and thus, grass length at any chosen point of time) or humidity due to changes in precipitation patterns. While we consider climate-induced changes in local humidity and grass growth dynamics in the agri-economic cost assessment, we exclude it in the ecological model to limit the dependencies between the ecological model and the climate and vegetation models in order to reduce computation time. This implies that we take these parameters as a general indicator of the suitability of a grassland cell and assume that the values are stable over time.
Finally, the impact of climate change on conservation costs and species is uncertain. For example, the uncertain impact on species is visible by different studies reporting different expected phenological adaptations for the same species. Regarding our target species specifically, we expect the black-tailed godwit to not adapt its timing of egg deposition based on two studies (Schroeder et al. Reference Schroeder, Piersma, Groen, Hooijmeijer, Kentie, Lourenço, Schekkerman and Both2012; Kentie et al. Reference Kentie, Coulson, Hooijmeijer, Howison, Loonstra, Verhoeven, Both and Piersma2018). However, Gunnarson and Tómasson (Reference Gunnarsson and Tómasson2011) did find phenological adaptations of the arrival date.
Despite these limitations, we believe our CEE model to be a useful approach to obtain an understanding of necessary adaptations for cost-effective AES under climate change. However, when translating these results into concrete recommendations for the adaptation of actual AES, the underlying assumptions and uncertainties need to be considered. More detailed recommendations that go beyond the general recommendations drawn from this research would therefore require a deeper understanding of the impacts of climate change on the target species (see e.g., Gerling et al. (Reference Gerling, Drechsler, Leins, Keuler, Radtke, Schulz, Sturm and Wätzold2022a) on the large marsh grasshopper) and the development of recommendations that explicitly take into account uncertainty (Drechsler et al. Reference Drechsler, Gerling, Keuler, Leins, Sturm and Wätzold2021).
Our research has shown how CEE modelling may be used for the analysis of AES under climate change and has highlighted the need to adapt the timing of conservation measures to climate change to maintain the cost-effectiveness of AES. Given the prominence of PES or AES, and the large amount of public resources spent on such schemes, we believe further research on the “climate-smart” design of AES to be highly valuable.
Supplementary material
To view supplementary material for this article, please visit https://doi.org/10.1017/age.2023.4
Data Availability Statement
The following data underlying the article is available:
Regarding the climate model, the data underlying the article are available at https://esgf-data.dkrz.de/projects/esgf-dkrz/. The code for the climate model requires a licensing agreement with the DWD or membership in the CLM community. This is possible after signing a community agreement.
Regarding the harvest module and agri-economic cost assessment, the data underlying the article are available at http://www.inf.fu-berlin.de/DSS-Ecopay/software_eng.html (files “Schleswig_Holstein_Dateien_fuer_Ecopay.zip”). The code for the two models can be cloned through the command line with git clone at git@85.214.153.232:ecoclimb.git.
Regarding the ecological model, the database including the bird species and the decision support system DSS-Ecopay are available from the DSS-Ecopay website: http://www.inf.fu-berlin.de/DSS-Ecopay/software_eng.html
Funding Statement
This work was supported by the German Federal Ministry of Education and Research (grant number 01LA1803A).
Competing interests
The author(s) declare none.