A prominent set of studies in political science shows that long-deceased coercive institutions often continue to influence contemporary political attitudes and behavior (e.g., Acharya, Blackwell, and Sen Reference Acharya, Blackwell and Sen2016a; Lupu and Peisakhin Reference Lupu and Peisakhin2017; see Charnysh, Finkel, and Gehlbach Reference Charnysh, Finkel and Gehlbach2023; Simpser, Slater, and Wittenberg Reference Simpser, Slater and Wittenberg2018 for reviews). Reliably establishing legacy effects is challenging. It requires making robust causal inferences over a very long time span during which the treatment could have affected not only the outcome but also other variables relevant for the analysis. To deal with this challenge, most legacy studies explicitly address post-treatment bias by employing appropriate methods such as the sequential g-estimator.
Building upon the analytical strategies established in the legacies literature, Homola, Pereira, and Tavits (Reference Homola, Pereira and Tavits2020; HPT) explore the long-term political consequences of the Third Reich. The results show that current-day political intolerance, xenophobia, and voting for radical right-wing parties are associated with proximity to former Nazi concentration camps in Germany. This conclusion relies on a series of analyses using election results and data from two different surveys to measure contemporary attitudes and behavior.
Pepinsky, Goodman, and Ziller (Reference Pepinsky, Goodman and Ziller2024; PGZ) re-examine HPT and argue that state-level differences confound the relationship between distance to camps and out-group intolerance. To overcome this issue, PGZ add contemporary state fixed effects to HPT’s models and find that proximity to concentration camps is no longer a reliable predictor of intolerance. The authors posit that contemporary states, although mainly formed after the Third Reich, do not introduce post-treatment bias if the following assumptions hold: (a) contemporary cross-state heterogeneity is not in the causal path between camp proximity and contemporary attitudes, and (b) there are no unobserved variables that jointly explain contemporary state differences and contemporary outgroup intolerance or camp proximity.
We agree that it is important to think carefully about spatial heterogeneity in the historical legacies literature. HPT’s original analysis accounts for regional heterogeneity by including controls such as the local-level share of unemployment and foreigners, urban status, or a dummy for East vs. West Germany. While HPT considered these solutions sufficient and did not find a theoretical motivation to include state fixed effects, other scholars operating in good faith might find doing so important for theoretical or empirical reasons.
We also agree with the authors that adding variables observed post-treatment to a model does not always bias the estimates. However, PGZ’s conclusion that current-day state fixed effects do not risk inducing post-treatment bias rests on a dual and inconsistent interpretation of what regional fixed effects capture.
The authors emphasize the importance of accounting for regional heterogeneity by noting that “Länder fixed effects adjust for any factor (observable or not) that varies across German Länder” (520). Any political, economic, or social dynamic that varies across states is captured by geographical fixed effects. However, the meaning of this same construct shifts when PGZ describe the conditions for contemporary state fixed effects to induce post-treatment bias: “unless distance to concentration camps (T) causally affects postwar Länder boundaries (F), controlling for Länder fixed effects cannot create posttreatment bias” (521). This statement is incorrect. Following the definition that PGZ used earlier, contemporary state fixed effects induce post-treatment bias if “any factor (observable or not) that varies across German Länder” is a direct or indirect descendant of proximity to concentration camps. In other words, we need to assume that Nazi concentration camps had no effects whatsoever that vary systematically across states. Even with a perfectly random geographic distribution of the camps across Germany, this assumption only holds if camps had no effects at all on the economic and social structure around them—something that is not consistent with existing evidence (Charnysh and Finkel Reference Charnysh and Finkel2017; Hoerner, Jaax, and Rodon Reference Hoerner, Jaax and Rodon2019). In brief, the very reason why PGZ emphasize that state fixed effects are important is also the reason why we should be concerned about post-treatment bias.Footnote 1
In the remainder of this note, we first discuss the plausibility of the assumptions invoked by PGZ to support their empirical strategy. Next, we describe how it is possible to account for state-level heterogeneity in HPT’s analyses without risking post-treatment bias or M-bias and without requiring PGZ’s strong assumptions. By replacing PGZ’s contemporary state fixed effects with Weimar-era state fixed effects—the state boundaries in place at the time when the first camps were built—the results in HPT remain substantively unchanged. In the Supplementary Material (SM), we further assess the robustness of the findings to spatial correlation by replacing the geographic variable in HPT with spatially correlated noise, an appropriate alternative to assess spatial autocorrelation (Kelly Reference Kelly2019). The simulations suggest that camp proximity consistently outperforms spatial noise as an explanatory variable. Finally, we conclude with some general recommendations for future studies when scholars are concerned about spatial heterogeneity affecting their inferences.
Taken together, the note contributes to the growing literature on legacy studies by offering practical solutions for correctly dealing with regional heterogeneity in this challenging field. It also highlights the importance of thinking carefully about what statistical tools and concepts (e.g., fixed effects) represent and account for, as well as their underlying assumptions.
POST-TREATMENT BIAS AND HOW TO AVOID IT
Post-treatment bias occurs when an analysis conditions on a variable that is directly or indirectly affected by the treatment and also shares a common cause with the outcome of interest. Post-treatment bias is especially problematic because without very strong assumptions, it is impossible to know how it affects our estimates of interest. Neither the direction nor the magnitude of the bias are possible to anticipate (Elwert and Winship Reference Elwert and Winship2014; Montgomery, Nyhan, and Torres Reference Montgomery, Nyhan and Torres2018).
To overcome these difficulties in settings where we have strong theoretical reasons to include variables that are measured post-treatment, complex modeling strategies and additional assumptions are necessary. The sequential g-estimator is one such approach (Acharya, Blackwell, and Sen Reference Acharya, Blackwell and Sen2016b). The method starts by estimating a model with pre-treatment and post-treatment covariates (first stage). Next, it recalculates the outcome variable by removing from it the effects of the mediating variables of interest. Finally, it estimates the effect of the treatment on this “demediated” outcome (second stage). The model allows us to incorporate post-treatment confounders and mediators without incurring in post-treatment bias.
Figure 1 shows a causal relationship between different types of variables of interest, where the flow of causality runs from left to right. We have a set of pre-treatment variables X, the treatment variable A, a mediator M, and the outcome of interest Y. In addition, there are two types of confounders: Z, which is affected by the pre-treatment variables and the treatment, and W, which is not. Both Z and W confound the relationship between the mediator and the outcome.
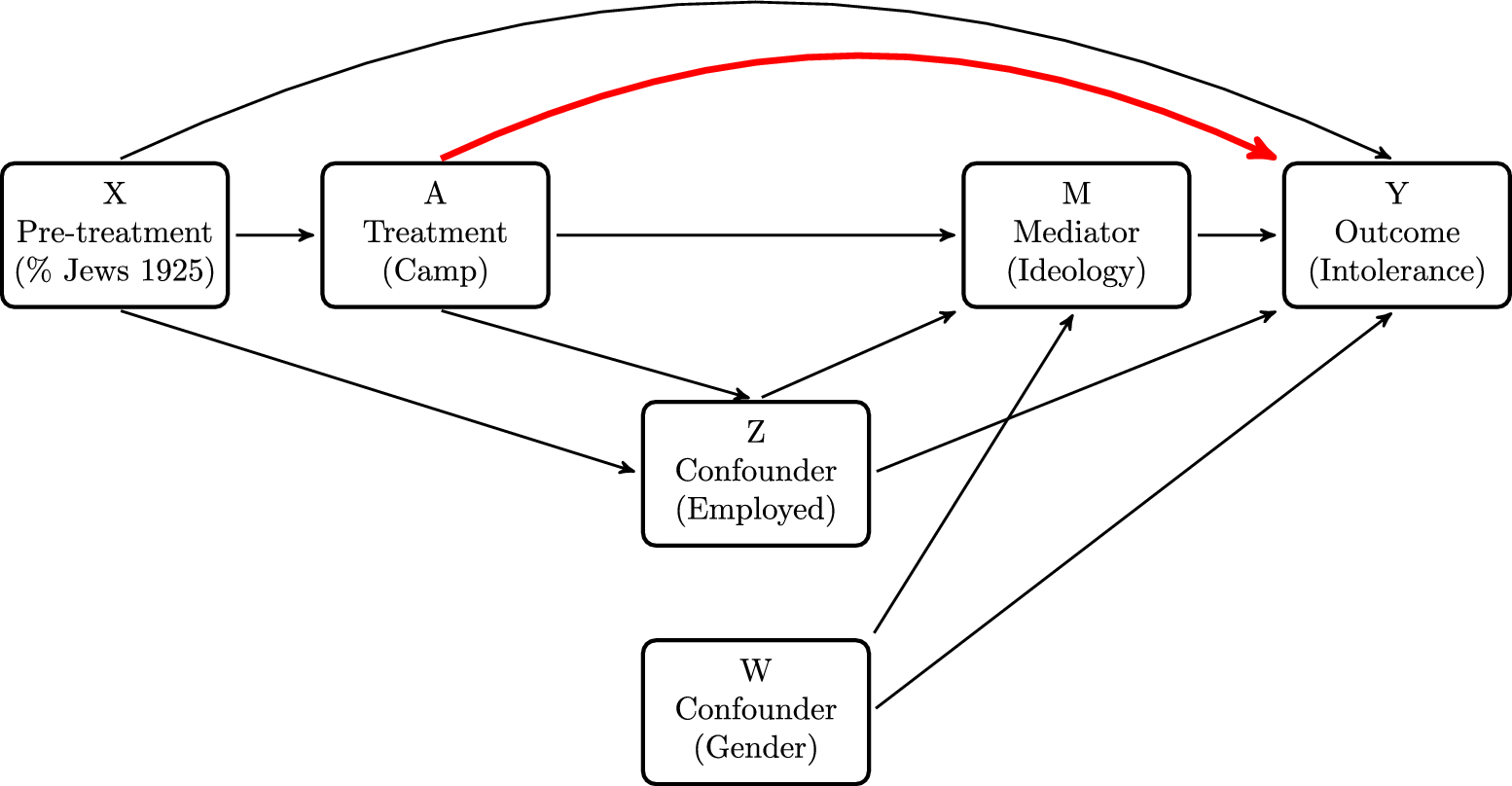
Figure 1. Directed Acyclic Graph Illustrating the G-Estimator
Note: The bold red line represents the controlled direct effect. Pre-treatment (i.e., Weimar-era) state-level fixed effects can be included as pre-treatment variables X. Post-treatment (i.e., contemporary) state-level fixed effects can be included as post-treatment confounders Z.
In HPT, the treatment A is the distance of a survey respondent (or area in the analyses with electoral data) to the closest former concentration camp, and the outcome of interest Y is captured by different indicators of out-group intolerance. The authors use variables like a district’s share of Jews in 1925 or unemployment rate in 1933 as pre-treatment variables X. We can think of an individual’s ideology as a mediator M, and of the control variables (e.g., a respondent’s employment status) as confounders Z that are likely to be affected by X and A. Other controls, such as age or gender, can be thought of as confounders W that are unlikely to be affected by X and A.
To analyze this data structure, HPT employ the sequential g-estimator (Acharya, Blackwell, and Sen Reference Acharya, Blackwell and Sen2016a; Reference Acharya, Blackwell and Sen2016b; Robins et al. Reference Robins, Blevins, Ritter and Wulfsohn1992). PGZ introduce contemporary state fixed effects into this setup. As recognized by the authors, decades passed between the construction of the camps and the creation of the German states we know today. Only 6 of the 16 current states already existed when the first camp was built.Footnote 2 PGZ’s decision to control for post-treatment state-level heterogeneity rests on two assumptions that we critically evaluate below.
PGZ’s Assumption 1: Contemporary State Heterogeneity Is Not Explained by Camp Locations
The first assumption for PGZ’s analyses to hold is that any contemporary cross-state differences (in attitudes, socioeconomic conditions, economic development, etc.) are not explained directly or indirectly by the location of camps. We rely on three pieces of qualitative evidence to demonstrate why this assumption is unlikely to hold.
First, after World War II, southwestern Germany initially consisted of three states: Baden, Württemberg-Hohenzollern, and Württemberg-Baden. The Württemberg-Baden government wanted to unify all three into a single state, but Baden was against it. The new Basic Law from 1949 contained a specific article, which clarified that if the states could not come to an agreement, a referendum would be held. This referendum took place on December 9, 1951 and ultimately resulted in a merger of the three states into the new state of Baden-Württemberg.Footnote 3 The political discussion in the run-up to the referendum focused on economic and administrative issues but also on outgroup resentment, including anti-Baden attitudes and religious factions (Weber and Häuser Reference Weber and Häuser2008). In other words, in this specific instance, citizens themselves determined the shape of their states. If the concentration camps affected people’s beliefs during the Third Reich, as HPT argue (see also Charnysh and Finkel Reference Charnysh and Finkel2017; Hoerner, Jaax, and Rodon Reference Hoerner, Jaax and Rodon2019), and these same people decided the shape of states created post-war, then contemporary state differences are directly in the causal path between camp locations and current-day attitudes (as M or Z in Figure 1).
Second, and most importantly, contemporary states can induce post-treatment bias even without the direct or indirect influence of camps on state borders. Another example highlighted by PGZ helps illustrate this. The authors mention one specific difference across states that their fixed effects capture: the existence of “variation in school curricula” (520). While all state curricula include the discussion of the Nazi regime, there is systematic variation in whether or not students visit a concentration camp. This variation is driven in part by proximity to a camp. Schools are more likely to organize a camp visit if there is a camp close by. Some states even subsidize camp visits if they happen within the same state (Rathenow and Weber Reference Rathenow, Weber, Ehmann, Kaiser, Lutz, Rathenow, vom Stein and Weber1995; see Fouka and Voth Reference Fouka and Voth2023 for similar evidence in Greece). Therefore, policies determined by today’s states are shaped by proximity to camps and affect the likelihood that students will visit a camp. Contemporary state fixed effects, if treated as a pre-treatment confounder, pick up these state-level differences and induce post-treatment bias.
Finally, on a more abstract level, we know that not every state has the same number of camps. For example, Thuringia has two camps in HPT’s analysis although it is among the smallest states in Germany. On the other hand, North Rhine-Westphalia is one of the largest states but does not have any camps. If we assume that the camps had any effect at all on their surrounding areas, and we know that some small states have multiple camps whereas some large states have no camps, then these camp effects are necessarily leading to state-level differences that are (a) clearly post-treatment, and (b) would be picked up by fixed effects. The results of Charnysh and Finkel (Reference Charnysh and Finkel2017) demonstrate exactly this: the area surrounding the Treblinka camp in Poland experienced a real estate boom following the closure of the camp, and local communities in the area are subsequently more supportive of an anti-Semitic party. In other words, the camp had attitudinal effects that were concentrated in the region surrounding the camp and would be picked up by contemporary state fixed effects.
Figure 2 expands a section of Figure 1 to illustrate our points. This DAG corresponds to Figure 1b in PGZ. Contemporary fixed effects are likely to capture a collection of post-treatment confounders which induces post-treatment bias.

Figure 2. Violation of PGZ’s Assumption 1
Note: Adaptation of PGZ’s Figure 1b showing a violation of the assumption that any contemporary cross-state differences are not explained directly or indirectly by the location of camps. The bold red line represents the causal effect of interest.
PGZ’s Assumption 2: No Collider Bias
PGZ’s empirical strategy relies on a second assumption: that contemporary state differences are not explained simultaneously by (a) a variable that also predicts contemporary outgroup attitudes (the outcome) and (b) a variable that predicts camp proximity (the treatment). Violating this assumption leads to a form of collider bias (M-bias) and produces spurious causal inferences.
PGZ defend this assumption by stating, “there is no interpretation of German administrative history that matches any of the hypothetical causal structures [described by the authors]” (524). Once again, PGZ reduce state fixed effects to a matter of administrative borders. However, once we acknowledge what regional fixed effects capture, this assumption becomes considerably less plausible.
First, virtually all of the canonical predictors of outgroup intolerance in Germany are geographically clustered in certain areas and can therefore also drive cross-state differences. These include economic insecurity (Funke, Schularick, and Trebesch Reference Funke, Schularick and Trebesch2016), perceived cultural threat (Norris and Inglehart Reference Norris and Inglehart2018), globalization “winners” and “losers” (Kriesi et al. Reference Kriesi, Grande, Lachat, Dolezal, Bornschier and Frey2006), and perceived security threat (Ward Reference Ward2019). Second, there is, in fact, a plausible predictor of contemporary state differences and camp proximity: the Weimar-era states. As PGZ describe, the states in place prior to the creation of the camps overlap with contemporary states at least partly. Additionally, the geographical clustering of the camps described above means that Weimar-era states predict camp proximity. Figure 3 puts this in formal terms using the DAG presented by PGZ in Figure 2b. Weimar-era states (F
$ {}_{t-1} $
) are a predictor of the treatment (A) and PGZ’s fixed effects (F
$ {}_{t+1} $
); in turn, conventional predictors of outgroup intolerance (U
$ {}_2 $
) influence contemporary state heterogeneity and the outcome. This violates the implausible and untestable assumption that F
$ {}_{t+1} $
is not a collider.
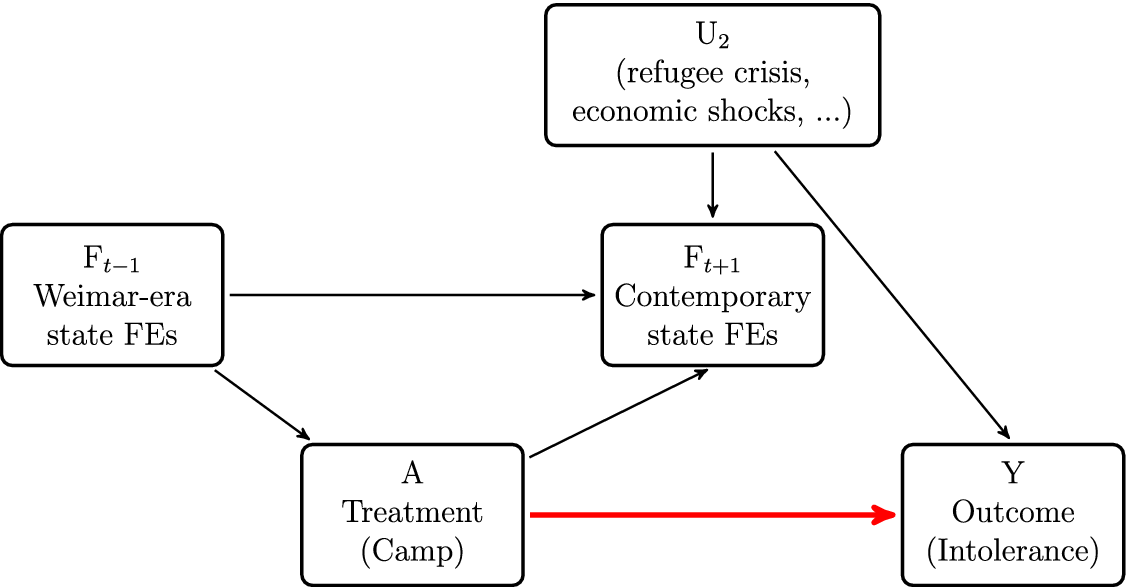
Figure 3. Violation of PGZ’s Assumption 2
Note: Adaptation of PGZ’s Figure 2b showing a violation of their assumption that there are no predictors of contemporary state differences (F
$ {}_{t+1} $
) and camp proximity (A). The bold red line represents the causal effect of interest.
These examples reveal the challenges of making assumptions about causal structures with variables that capture many sources of variation, such as regional fixed effects. By introducing in the causal model an amorphous cluster of variables that cannot be isolated, PGZ are forced to rely on untestable and implausible assumptions. Next, we describe two solutions to avoid the assumptions invoked by PGZ: (a) correctly specifying contemporary regions as post-treatment variables, or (b) using pre-treatment state fixed effects.
Two Solutions to Account for Regional Heterogeneity
Consider again the causal relationship in Figure 1. PGZ treat contemporary state fixed effects as pre-treatment variables X. Above, we described different ways in which this modeling choice induces post-treatment bias. However, g-estimation provides a way to overcome these flaws. Instead of treating contemporary states as pre-treatment variables, we consider them to be post-treatment confounders Z. This assumes that contemporary states are confounding the relationship between the mediator (e.g., ideology) and the outcome (e.g., out-group intolerance), which makes intuitive sense and is in line with the potential confounding effects of state heterogeneity that PGZ discuss (“unsynchronized policy environments” and “substantial variations in school curricula”). HPT adopt this same procedure to account for systematic differences between East and West Germany.
In terms of the estimation, this means that contemporary state fixed effects should appear in the first stage of the sequential g-estimator, but not in the second stage. Recall that the goal of the first stage is to accurately estimate the effect of the mediator on the outcome to successfully “de-mediate” the outcome before the second stage. The confounders W or Z (i.e., the contemporary state fixed effects) are only relevant for this part of the estimation and should not be included in the second stage.
An alternative solution to avoid post-treatment bias involves replacing contemporary state fixed effects with pre-treatment state fixed effects. We use Germany’s administrative map from 1932 as seen in Figure SM2.1 to identify the corresponding Weimar-era state for each present-day geographic location. We chose 1932 because it is the year before the first German camp was created (Dachau, March 1933). Theoretically, the use of the Weimar states means that we are now working with true pre-treatment variables X (cf. Figure 1). The Weimar states might affect camp locations (the treatment) through their policy environment or other unobserved factors that the other pre-treatment variables did not capture. They can also affect some of the contemporary confounders (Z) and the outcome variables (Y). Empirically, it means that the Weimar state fixed effects can now be included in both stages of the g-estimator without inducing post-treatment bias.Footnote 4 Crucially, because these are now pre-treatment variables, we do not have to make any assumptions about how they might be affected by the treatment.
Finally, we combine both approaches by including contemporary state fixed effects in the first stage and Weimar-era state fixed effects in both stages of the g-estimator. This third specification allows us to simultaneously account for historical regional differences that may explain camp location, and for any post-treatment confounder that varies systematically across contemporary states.
Together, HPT, PGZ, and the current note provide an extensive list of models with different data sources and model specifications. To help the reader follow this collective effort, Table SM1.1 summarizes the different main specifications modeling the effect of camp proximity on contemporary outcomes.
Results
We replicate the main analyses in HPT (Tables 2 and 4) while including (a) contemporary state-level fixed effects in the first stage of the g-estimator, (b) Weimar-era state-level fixed effects in both stages of the g-estimator, and (c) both contemporary states and Weimar-era states.Footnote 5
Figure 4 replicates the four columns of Table 4 in HPT. Each panel shows the controlled direct effect of camp proximity on support for radical right parties in 2017 for four different model specifications. The first coefficient (Baseline) is the effect reported in HPT. The second coefficient (Current state FEs in 1st stage) reports the results from the same model specification when we also include contemporary state fixed effects in the first stage of the g-estimator. The third coefficient (Weimar state FEs) corresponds to the models including Weimar-era state fixed effects in both estimation stages. Finally, the last coefficient (Current state FEs + Weimar state FEs) corresponds to models simultaneously accounting for contemporary regional differences in the first stage and Weimar-era fixed effects in both stages.
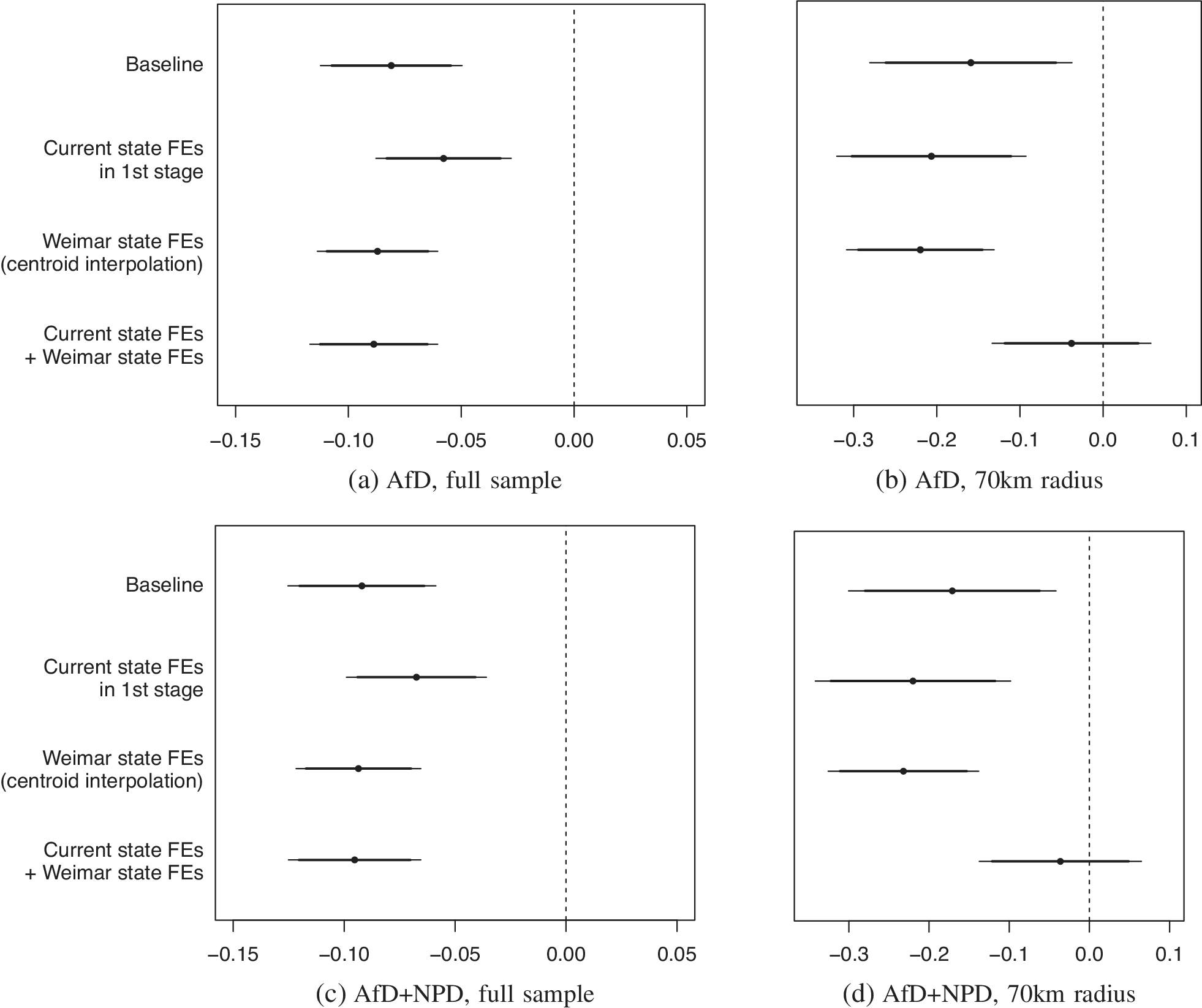
Figure 4. The Controlled Direct Effect of Camp Proximity on Support for Radical Right Parties in 2017, Accounting for State-Level Heterogeneity
Note: Plots depict estimates and 95%/90% confidence intervals from the sequential g-estimator for the controlled direct effects of distance to camps on support for radical right parties in 2017 (described in each panel label). Each estimate corresponds to a different model specification, described on the y-axis. The baseline specification corresponds to the results reported in Table 4 in HPT (
$ N\hskip0.3em = $
10,755 [a,c] and 3,949 [b,d]). Full model results for the remaining specifications in Tables SM3.1–SM3.3.
The results show that the main conclusions in HPT are robust to the inclusion of state fixed effects. Across the different specifications, we see that the effect of distance is always negative and statistically reliable at conventional levels except for the models within a 70-km radius and with pre- and post-treatment regional fixed effects.Footnote 6 We also do not observe any dramatic change in the uncertainty of the estimates.
The three panels in Figure 5 repeat this exercise for HPT’s EVS analysis. More specifically, each panel replicates the results in columns 2, 4, and 6 of Table 2 in HPT, respectively, corresponding to a different outcome variable.Footnote 7 We report the controlled direct effect of camp proximity for the same four model specifications as in Figure 4.

Figure 5. The Controlled Direct Effect of Camp Proximity on Outgroup Intolerance, Immigrant Resentment, and Support for Far-Right Parties (EVS), Accounting for State-Level Heterogeneity
Note: Plots depict estimates and 95%/90% confidence intervals from the sequential g-estimator for the controlled direct effects of distance to camps on contemporary attitudes (described in each panel label). Each estimate corresponds to a different model specification, described on the y-axis. The baseline specification corresponds to the results reported in Table 2 (models 2, 4, and 6;
$ N=1,376 $
) in HPT. Full model results for the remaining specifications in Tables SM4.1–SM4.3.
Again, we find support for the original conclusions. Across the different outcome variables and fixed effects specifications, we see that the effect of distance is always negative and reliable. When we introduce contemporary state fixed effects as post-treatment variables, the main results are virtually unchanged. When Weimar-era fixed effects are introduced, the effect sizes decrease slightly in all models. However, for both attitudinal measures (panels a and b), the effects remain statistically significant. Only the estimate for self-reported far-right support (panel c, coefficient 3) is no longer distinguishable from zero at conventional levels (p-value = 0.11), although the estimated effect is indistinguishable from the result obtained in the baseline model without state fixed effects. When using contemporary and Weimar-era fixed effects, the main results remain unchanged. Overall, the evidence reveals that once we account for spatial heterogeneity in a way that avoids post-treatment bias, the results uncovered in HPT remain unchanged.
DISCUSSION
Our goal with this research note is to contribute to the discussion of how to deal with regional heterogeneity in studies of historical legacies. We discuss the specific challenges that the inclusion of fixed effects may pose in work that tries to estimate the impact of historical events. We identify two obstacles that scholars need to overcome in order to avoid post-treatment bias. The first challenge is theoretical. Informed by theory and qualitative evidence, scholars need to decide what type of confounding they want to correct while recognizing that regional fixed effects capture any source of variation across units. This determines which regional units to control for: historical or current ones. In the context of legacy studies, this choice is crucial given that borders are often redrawn throughout history and capture an amorphous set of heterogeneity that may be directly or indirectly on the causal path of interest. The second challenge entails making the correct modeling choices, for example, correctly specifying the g-estimator to avoid post-treatment bias. The types of regional units used (pre-treatment or post-treatment) define how they can be incorporated in the analysis and, in turn, whether the results are biased.
We show that these obstacles are real and consequential using the example of PGZ’s criticism of HPT. PGZ failed to overcome both of the obstacles listed above, which led to post-treatment bias in their analysis. Properly introducing regional fixed effects in HPT’s original analysis—without inducing post-treatment bias—confirms our original results.
As a general recommendation for future studies, if there are concerns about regional confounding along administrative borders that are justified based on the researcher’s background knowledge of the case, we suggest using fixed effects based on borders established pre-treatment given the amorphous nature of factors captured by regional fixed effects. However, two other methods are better equipped to deal with geographical heterogeneity and treatments that have localized effects: (1) subsetting the analysis into small (and varying) radii around the source of effects and (2) sensitivity analyses to spatial autocorrelation. The former solution is already adopted in HPT. We perform the second method in Section SM6 and show that spatial correlation is not a relevant threat to inference in this specific context. We believe these approaches offer a more principled solution than using fixed effects to deal with spatial auto-correlation because they reduce researcher degrees of freedom and allow scholars to move beyond arbitrary administrative borders (see Fouka and Voth Reference Fouka and Voth2023 for a similar approach).
Our note offers practical reminders about the problem of post-treatment bias and guidance on how to avoid it in historical analyses. We highlight that, while commonly used, the choice of whether or not to include fixed effects is not straightforward in this context. It pays to pause and think whether fixed effects are warranted at all, and if yes, how to properly include them without introducing further bias into the analysis. Ultimately, we hope to highlight the important interplay of theory and empirics, especially in the inherently complicated assessment of the present-day consequences of events that took place decades ago.
SUPPLEMENTARY MATERIAL
To view supplementary material for this article, please visit https://doi.org/10.1017/S0003055423001351.
DATA AVAILABILITY STATEMENT
Research documentation and data that support the findings of this study are openly available at the American Political Science Review Dataverse: https://doi.org/10.7910/DVN/YTXZ6X.
Acknowledgments
We would like to thank Avidit Acharya, Volha Charnysh, Vicky Fouka, Anselm Hager, Soeren Henn, Florian Hollenbach, Connor Huff, Christopher Lucas, Noam Lupu, Jacob Montgomery, Leonid Peisakhin, Arturas Rozenas, Anton Strezhnev, Michelle Torres, Rick Wilson, and Yuri Zhukov for comments and helpful discussions about earlier versions of the article.
CONFLICT OF INTEREST
The authors declare no ethical issues or conflicts of interest in this research.
ETHICAL STANDARDS
The authors affirm this research did not involve human subjects.
Comments
No Comments have been published for this article.