The proliferation of social media—counter to the initial projection that it would usher in democratization across the globe (Diamond Reference Diamond2010; Tufekci and Wilson Reference Tufekci and Wilson2012)—has recently become a key ingredient in technological innovations that expand autocrats’ toolkits. Social media can be used to facilitate domestic and international goals alike by providing autocrats with platforms for monitoring popular attitudes toward a plethora of issues; interfering in public discussions at scale; reaching out to diverse groups of the population with promises, perks, or threats; collecting information about dissidents; or sowing discord and uncertainty among opponents inside and outside the country (Deibert Reference Deibert2019; Feldstein Reference Feldstein2019; Gunitsky Reference Gunitsky2015; Lorentzen Reference Lorentzen2014; Tucker et al. Reference Tucker, Theocharis, Roberts and Barbera2017). Here we investigate another way that modern nondemocracies employ social media to secure regime survival by analyzing how one competitive authoritarian regime, Russia, uses new artificial intelligence technologies (social media bots) to respond to both offline and online opposition activities.
Although social media trolls and bots are a new phenomena in politics, they have recently gained increasing scholarly attention (Bolsover and Howard Reference Bolsover and Howard2017; King, Pan, and Roberts Reference King, Pan and Roberts2017; Sanovich, Stukal, and Tucker Reference Sanovich, Stukal and Tucker2018; Stukal et al. Reference Stukal, Sanovich, Bonneau and Tucker2017; Tucker et al. Reference Tucker, Theocharis, Roberts and Barbera2017; Wooley Reference Wooley, Persily and Tucker2020), especially due to mounting evidence suggesting that the Russian government has employed them in pursuit of foreign policy goals (Leonnig, Hamburger, and Helderman Reference Leonnig, Hamburger and Helderman2017; Shane Reference Shane2017). Previous research has also shown that human trolls can be employed to spread discord (Golovchenko et al. Reference Golovchenko, Buntain, Eady, Brown and Tucker2020; Linvill et al. Reference Linvill, Boatwright, Grant and Warren2019; Phillips Reference Phillips2015), cause distrust in the political system of autocrats’ international opponents (Badawi, Lerman, and Ferrara Reference Badawi, Lerman and Ferrara2018), spread deceptive content (Lou, Flammini, and Menczer Reference Lou, Flammini and Menczer2019), or disseminate disinformation of various kinds (Shao et al. Reference Shao, Ciampaglia, Varol, Yang, Flammini and Menczer2018; Starbird Reference Starbird2019). Paid human trolls can also be used for domestic purposes in nondemocratic regimes—for example, for spreading positive sentiment toward the regime (King, Pan, and Roberts Reference King, Pan and Roberts2017) or diverting critical online discussions away from politically charged issues (Sobolev Reference Sobolev2018).
However, academic research on the ways authoritarian regimes use social media bots, defined as algorithmically controlled social media accounts, in the context of domestic politics is scarce. This is perhaps due to a lack of data about bots, which is in turn due to numerous challenges that arise when identifying bots, quantifying their behavior, and collecting very large relevant social media datasets. To mitigate this problem, previous research has mostly relied on publicly available general-purpose algorithms for detecting bots. This research has shown that bots can be used to promote human-generated political content (Stella, Ferrara, and Domenico Reference Stella, Ferrara and De Domenico2018) and are even capable of occupying a central role in online political discussions (Schuchard et al. Reference Schuchard, Crooks, Stefanidis, Croitoru, Aiello, Cherifi, Cherifi, Lambiotte, Lió and Rocha2019). Anecdotal evidence suggests that bots are employed to instill doubts about mainstream interpretations of political events or spread conspiracy theories (Kitzie, Karami, and Mohammadi Reference Kitzie, Karami and Mohammadi2018). Other scholarship has focused on evaluating the effect of bots on online network characteristics (Ghosh et al. Reference Ghosh, Viswanath, Kooti, Sharma, Korlam, Benevenuto, Ganguly and Gummadi2012; Zhang et al. Reference Zhang, Zhang, Zhang and Yan2016) or revealing specific strategies employed to manipulate social media algorithms (Benigni, Joseph, and Carley Reference Benigni, Joseph, Carley, Agarwal, Dokoohaki and Tokdemir2019). An alternative strategy of detecting bots has been to develop technologies based on new machine learning algorithms (Chavoshi, Hamooni, and Mueen Reference Chavoshi, Hamooni and Mueen2016; Davis et al. Reference Davis, Varol, Ferrara, Flammini and Menczer2016; Stukal et al. Reference Stukal, Sanovich, Bonneau and Tucker2017), although this body of research has been published outside of political science and has been largely disconnected from political science theories.
Here, we take the research on the political use of social media bots in authoritarian regimes a step further. We build on diverse strands of research about protest movements and authoritarian politics and theorize about the different ways in which political actors in a nondemocratic regime can use social media bots to prevent, suppress, or react to offline and online opposition activities. We choose to focus on the political strategies behind the use of bots in nondemocratic regimes due to the complex and nonlinear effects that social media can have on mass political protests and other types of political instability in authoritarian regimes. Periods of instability, or those leading to instability, are arguably the times when social media platforms can either facilitate activists’ access to broadcasting technologies (Rohlinger and Corrigall-Brown Reference Rohlinger, Corrigall-Brown, Snow, Soule, Kriesi and McCammon2019) or enable the government to manipulate public perception of the factors that are known to matter for protest mobilization, including grievances (Gurney and Tierney Reference Gurney and Tierney1982; Klandermans Reference Klandermans1997; Opp Reference Opp1988; Van Stekelenburg, Klandermans, and Walgrave Reference Van Stekelenburg, Klandermans, Walgrave, Snow, Soule, Kriesi and McCammon2019; Walsh Reference Walsh1981), group political efficacy (Bandura Reference Bandura1997; Finkel Reference Finkel1985; Wallace, Zepeda-Millan, and Jones-Correa Reference Wallace, Zepeda-Millan and Jones-Correa2014), emotions (Halfmann and Young Reference Halfmann and Young2010; Jasper and Poulsen Reference Jasper and Poulsen1995), social esteem (McClendon Reference McClendon2014), or an individual’s cost–benefit calculus (Hardin Reference Hardin1982; Oliver Reference Oliver1993; Olson Reference Olson1965). When this type of manipulation happens during or on the eve of protest rallies, it can be aimed at controlling the information environment and preventing rallies from growing. Alternatively, manipulation can seek to exercise online agenda control (McCombs Reference McCombs2014) in order to prevent the opposition from taking the initiative and dominating online political communications. What these two strategies have in common is that governments today can pursue both of them through the use of automated bots, which remains an understudied topic in political science.
To address this gap in the literature, we develop theory-based predictions about the political strategies behind the use of bots to counter domestic opposition in Russia and empirically test these predictions with a large collection of data on the activity of Russian Twitter bots from 2014 to 2018. We argue that not only government agencies, but also nongovernment actors, can deploy pro-government bots for policy and agency reasons. In both cases, the deployed bots can be focused on either demobilizing opposition supporters offline or exercising online agenda control. We develop observable implications of these two alternatives and empirically show that even though pro-government bots are involved in both types of activities, the ones we are able to identify are primarily employed as an online agenda control tool.
Our contribution is twofold. First, we bridge the gap between scholarship from the field of computer science on bot detection and research in political science on authoritarian politics by reverse-engineering the use of social media bots in a competitive authoritarian setting and identifying specific political strategies that can be pursued with the use of bots. Second, we develop testable hypotheses about the way social media bots can be used to counter domestic opposition activity either online or offline. We derive our hypotheses from diverse strands of political science literature, including previous research on trolls (King, Pan, and Roberts Reference King, Pan and Roberts2017; Roberts Reference Roberts2018), and show that some of those predictions do not hold for bots. Overall, our study advances previous research on the toolkit for undermining online opposition that is available in modern nondemocratic regimes (Sanovich, Stukal, and Tucker Reference Sanovich, Stukal and Tucker2018) by bringing together theoretical predictions and data.
RUSSIAN TWITTER BOTS
In this section, we provide some background information about the activity of Twitter bots in Russia by focusing on two questions: What is a Twitter bot? And who could be interested in deploying Twitter bots in contemporary Russia? Readers who are interested in a deeper understanding of the technological details of bot activity or illustrative examples of tweets posted by pro-government bots are referred to Online Appendices B–C.
What Is a Twitter Bot?
We define Twitter bots as algorithmically controlled accounts that can automatically perform a variety of actions including posting, retweeting, liking, responding, etc. In spite of this simple definition, bots represent very diverse types of social media accounts. In some trivial cases, a bot can be as simple as a small script that redirects updates from a website to someone’s Twitter page. In more sophisticated cases, bots can fully control a Twitter account and even communicate with other social media users (Freitas et al. Reference Freitas, Benevenuto, Ghosh, Veloso, Pei, Silvestri and Tang2015), thus resembling chatbots or recomender systems one could encounter when interacting with a large company online. Here, we focus on the latter case of the more sophisticated computer programs that are capable of maintaining Twitter activity, without a continuing intervention by a human operator. Figure 1 shows an example of such a Twitter bot that adopted the old Russian male name Yermolay as its screen name.
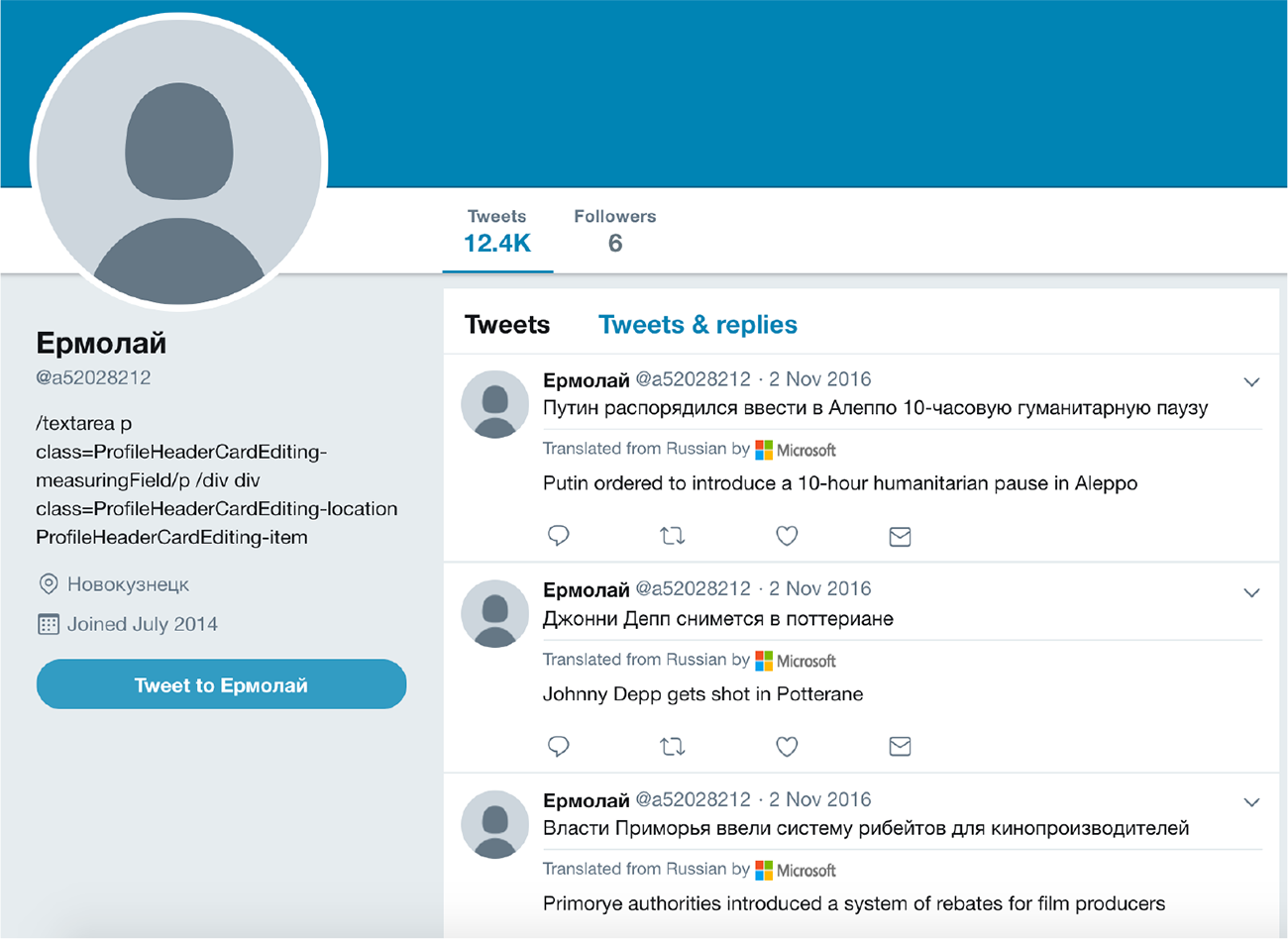
Figure 1. Russian Twitter Bot “Yermolay”
Yermolay is a bot that is not hard to identify for a number of reasons. First, this account has posted over 12,000 tweets, but there are only six other Twitter users who follow its tweets. Second, this account does not have a user picture and employs the default silhouette image instead, which is a common sign of a bot (Nimmo Reference Nimmo2017). Additionally, the bio section for this account does not contain any meaningful information and is merely a code snippet. Finally, the tweets posted by this account resemble news headlines, another behavior typical of bots (Sanovich, Stukal, and Tucker Reference Sanovich, Stukal and Tucker2018).
Why Deploy Bots in Russia?
In recent years, fake social media accounts similar to Yermolay have gained growing popularity in Russia. Bots stand out in the repertoire of digital information manipulation tools for a number of reasons. They are hard to trace, as—unlike humans, who need to work from a physical location, which facilitates tracking their IP addresses and linking them to certain organizations like the Internet Research Agency—bots can be deployed anywhere, including cloud computing environments, digital devices, and appliances (Boulton Reference Boulton2015; Kumar and Lim Reference Kumar and Lim2020). This feature makes bots specifically suitable for covert online operations that are aimed at affecting public opinion. Bots are also automated tools that can be deployed at scale (Hegelich and Janetzko Reference Hegelich and Janetzko2016), which makes them particularly useful for specific tasks including imitating regime support by ordinary citizens or manipulating social media and search engine algorithms to promote specific content online. Last, bots are relatively cheap to employ. Unlike paid human trolls—another novel tool available to autocrats in the digital era—bots typically involve minimal human intervention and do not require salary or overtime payments. Bots are pieces of software that can run online for indefinite periods of time (until they are detected and shut down by the platforms on which they operate, or by their creators) without requiring additional resources besides the initial production costs (Agarwal Reference Agarwal2017; Pickell Reference Pickell2018).
The ease of bot deployment partially explains the utility of bots and their observed wide use in autocratic regimes. Although our knowledge of government-sponsored bot deployment initiatives is generally scarce, previous research was relatively successful in identifying the use of bots by Russian authorities at the regional level. In particular, it has been revealed that most regional governors in Russia have official Instagram accounts followed by large numbers of bots, accounting for anywhere from 13% to 63% of governors’ followers (Center for Current Politics 2019). The underlying motivation for making bots follow governors’ accounts remains unknown, but anecdotal evidence suggests two potential explanations.
First, the deployment of bots can be motivated by policy-related concerns. A recent example comes from Moscow, where the regional government employed fake social media accounts supporting a controversial housing reconstruction program on the eve of the 2018 Moscow mayoral election (Chizhova Reference Chizhova2017). Abundant evidence also suggests that pro-government bots were employed during the 2019 regional governor elections to promote positive sentiment toward incumbent candidates in a number of Russian regions (Davidov Reference Davidov2019).
There are also multiple agency-related reasons for deploying pro-government bots. On the one hand, the Kremlin has required regional governors to pay more attention to their social media activity and has advised them to create regional government departments focused on managing political communication in social media (Antonova Reference Antonova2018). On the other, public employees at these departments often lack the necessary skills for effective social media communication and rely on bots to artificially inflate relevant activity indicators. Agency-related considerations are arguably also involved in the use of bots in the interests of other levels of the Russian government. Politicians and businessmen can deploy pro-regime bots in an effort to signal loyalty or develop stronger ties to the regime, which can result in extra perks and easier access to public resources (Pertsev Reference Pertsev2019). The attractiveness of bots as a loyalty-signaling tool is due to the imbalance of public and private information in nondemocratic settings. Although private actors can use nonpublic communication channels with the government to claim responsibility for bot activity, the difficulty of tracing bots back to their masters creates relatively low risks of popular condemnation or international reputation loss for these private actors.
Our analysis in this article remains largely agnostic about specific actors coordinating or funding the bots and bot-nets we analyze, the only assumption being that those actors are interested in maximizing the intended effects of bots on other Twitter users, either for policy-related reasons or due to agency considerations. In the next section, we develop two different but related theoretical frameworks for conceptualizing the political logic behind the use of pro-government Twitter bots by autocratic regimes in their own country’s domestic politics. We focus on bots’ responses to increases in offline or online opposition activity because these periods provide bot managers with a good opportunity to signal loyalty to the regime or justify potential funding requests. Additionally, this focus allows us to link different strands of literature from the fields of comparative politics and political communication to explain a novel phenomenon in the modern politics of authoritarian regimes.
THEORY AND HYPOTHESES
Tension between incumbents and the opposition is a—if not the—primary component of domestic politics in competitive authoritarian regimes, whose survival largely depends on the regime’s ability to manage the informational environment (Guriev and Treisman Reference Guriev and Treisman2019) and maintain the widespread belief in low numbers of opposition supporters (Kuran Reference Kuran1991; Lohmann Reference Lohmann1994), their lack of coordination (Kuran and Romero Reference Kuran, Romero, Congleton, Grofman and Voigt2019), and the high expected costs of engagement in opposition activities (Rubin Reference Rubin2014). These goals are often achieved by silencing adverse information and emphasizing positive agendas (Roberts Reference Roberts2018) or selectively attributing good and bad news to the government and other actors, respectively (Rozenas and Stukal Reference Rozenas and Stukal2019). However, the effectiveness of these techniques is questionable in times of large-scale collective actions that can not only pose an immediate threat to political elites but also send broad communities strong antiregime signals, thereby creating and popularizing alternative public agendas. Offline collective action and online political campaigns (for example, high-profile anticorruption investigations targeting senior officials) organized by opposition leaders can therefore motivate nondemocratic regimes to mobilize extra resources and demonstrate the full potential of their propaganda machines in mass and social media.
In addition, these are also the times that can provide the managers of social media bots, who are not necessarily affiliated with the government, with a good opportunity to signal their loyalty to the regime. They could also use this opportunity to justify the need for further funding and access to government resources by deploying their networks of social media bots at full scale. Given these considerations and the growing political science literature on the online aspects of offline political mobilization (Aytaç, Schiumerini, and Stokes Reference Aytaç, Schiumerini and Stokes2018; Steinert-Threlkeld Reference Steinert-Threlkeld2017; Sullivan Reference Sullivan2016), we focus on analyzing the political strategies behind the use of pro-government bots in times of both offline and online opposition mobilization to study the differential response of bots to these two types of events.
Theoretically, bot responses can be conceptualized in terms of their attempts to change the cost–benefit analysis of a potential opposition supporter weighing the costs and benefits of taking actions in support of the opposition in a competitive authoritarian environment (Kuran Reference Kuran1991; Oliver Reference Oliver1993). One way to push citizens away from getting involved in opposition initiatives is to increase the actual costs of staying informed about opposition activities and plans for collective action. In some countries, including China, this is achieved by large-scale censorship that makes it harder for ordinary people to get access to off-limits information (King, Pan, and Roberts Reference King, Pan and Roberts2017). However, censorship is not the only tool available to nondemocratic regimes. An alternative technique is to increase—rather than decrease via censorship—the volume of available information (Roberts Reference Roberts2018). Swamping news consumers with massive flows of irrelevant information can make it harder and more time consuming to find antiregime news.
Alternatively, bots might seek to change the public perception of regime popularity. Under an unpopular government, even a small disorganized rally can stir up popular grievances and spark a cascade of large-scale protests capable of overthrowing the regime. However, the expectations can be dramatically different when there is a widespread belief that the regime enjoys high levels of popular support. Attempting to make the regime and its leaders look stronger and more popular is therefore one way bots could affect the expected costs and benefits of supporting the opposition.
Another way for bots to affect the perceived costs of joining opposition activities online or offline is to act as automated trolls that publicly harass and threaten opposition activists, thereby raising additional concerns about the physical security and potential future persecution of opposition supporters. Even though one could argue that actual human trolls might be more effective in arguing with activists and emphasizing weaknesses in their agenda, automated bot accounts could be better at inducing fear via posting a slew of threats to opposition leaders or slandering them at scale—for example, by publicizing compromising information hacked from their email accounts (Sanovich Reference Sanovich, Woolley and Howard2018).
Taken together, the goals of decreasing the expected benefits and increasing the expected costs of getting involved in offline or online opposition activities shape the space of possible political strategies available to pro-government bots.
Observable Implications and Hypotheses
In this paper, we focus on four observable implications for bot behavior during offline protests and increased online opposition activity that we derive from our theoretical cost–benefit analysis framework.
One potential strategy that bots can employ is to deemphasize the protest-related agenda by distracting social media users and augmenting informational noise in their social media feeds. As a result of this strategy, if bots are activated—for whatever reason—in times of street protests or online opposition mobilization, then the volume of content posted by pro-government bots should go up during these periods. We refer to this generic observable implication as the volume implication.
Another observable implication of the same strategy is to amplify a more diverse set of news. Some of the news might be positive for the regime, others could be neutral or negative but unrelated to the cause of the protest. The goal is to distract social media users, expose them to an information environment that is rich in news of every kind, and make it harder for them to focus on the protest-related agenda (Munger et al. Reference Munger, Bonneau, Nagler and Tucker2018). We thereby expect bots to increase in the diversity of the accounts they retweet as a response to offline or online opposition mobilization, which we refer to as retweet diversity.
Another possible strategy for decreasing opposition supporters’ expected benefits is to program bots to adopt the tactics similar to what paid human trolls do in China. As King, Pan, and Roberts (Reference King, Pan and Roberts2017) show, Chinese trolls act as cheerleaders that express positive sentiment about government activities. Bots can post similar content automatically and on a large scale. More specifically, we measure this behavior with the number of tweets that pro-government bots post about Vladimir Putin on a given day. We refer to this response as cheerleading.
Finally, the automated trolling and harassment of opposition leaders constitutes an alternative strategy aimed at increasing the expected costs of supporting opposition. We measure the use of this strategy, referred to as negative campaigning, with the number of pro-government bot-produced tweets that mention Alexey Navalny, a prominent Russian opposition leader, who is also known for his charisma and ability to bring large numbers of protesters to the streets (Nechepurenko Reference Nechepurenko2018).
These four observable implications provide us with a set of hypotheses that can be tested empirically to both shed light on the logic behind the use of bots in domestic politics in modern nondemocratic regimes and contrast the use of bots during mass street protests and their deployment as a response to opposition mobilization online. Are bots primarily used as yet another tool for demobilizing citizens in times when opposition is trying to bring people onto the streets, or are they mainly employed as an online agenda control, or gatekeeping, mechanism (McCombs and Shaw Reference McCombs and Shaw1972) tailored to regulating information flows on social media?
In Table 1, we concisely summarize our hypotheses that are drawn from the two different—but not mutually exclusive—theoretical frameworks for explaining the use of pro-regime political bots in comparative authoritarian regimes. The first is that bots are used in the interest of offline demobilization—that is, to reduce participation in offline protests. The second is that bots are used to control the online agenda, which we refer to as the online agenda control framework, and therefore will be mobilized in response to opposition online activity.Footnote 1 These two theoretical perspectives do not differ in the hypothesized tactics that will be used by pro-government bots but only in the events (offline protest vs. online activity) that will trigger the use of these bots. Thus, in Table 1, the four observable implications that start with H1 (H1a, H1b, H1c, and H1d) are derived from the offline demobilization theoretical framework, whereas the four implications that start with H2 (H2a, H2b, H2c, and H2d) are the predicted empirical observations from the online agenda control theoretical framework.
Table 1. Summary of Hypotheses

Note: The observable implications that start with H1 (H1a, H1b, H1c, and H1d) together make up the tests of the offline demobilization theoretical framework, whereas the implications that start with H2 (H2a, H2b, H2c, and H2d) make up the tests of the online agenda control theoretical framework.
DATA AND METHODS
To conduct our analysis, we rely on approximately 32 million tweets about Russian politics in Russian that we collected via the Twitter Streaming API using a list of keywords related to different aspects of Russian politics (see the Online Appendix A for further details on data collection). These tweets were posted during 2015—2018 by about 1.4 million Twitter users using the Russian-language Twitter account interface. As Twitter does not automatically label users as humans or bots, our first task was to identify bots in our collection.
Detecting Bots
As social media bots are gaining growing attention in the social sciences, tools for detecting them are continually developing.Footnote 2 Most existing research relies on publicly available general-purpose software like Botometer (Heredia, Prusa, and Khoshgoftaar Reference Heredia, Prusa and Khoshgoftaar2018; Stieglitz et al. Reference Stieglitz, Brachten, Ross and Jung2017; Vosoughi, Roy, and Aral Reference Vosoughi, Roy and Aral2018) or DeBot (Bello, Heckel, and Minku Reference Bello, Heckel and Minku2018; Schuchard et al. Reference Schuchard, Crooks, Stefanidis, Croitoru, Aiello, Cherifi, Cherifi, Lambiotte, Lió and Rocha2019) that are not tailored to the specific contexts under study here. This is particularly problematic, as bots can exhibit dramatic differences in patterns across national borders, periods, and campaigns (Uyheng and Carley Reference Uyheng, Carley, Thomson, Bisgin, Dancy, Hyder and Hussain2019). Additionally, the use of tools such as Botometer or DeBot does not allow researchers to retrospectively analyze historical data—both approaches rely on a real-time call to the Twitter API, which attempts to assess the bot versus human status of the account at the time of the study. For many studies we need to classify bots at the time of the account activity in question—which is of course crucial for many research tasks in the social sciences.Footnote 3
Another approach involves researchers using leaked lists of bots, as has been previously applied when studying paid human trolls (Sobolev Reference Sobolev2018). However, this technique requires both the existence of such a leak and confidence in its veracity and representativeness.
To avoid the concerns with the aforementioned approaches and to enable better quantification of error, we make use of a tool that is designed specifically for detecting bots on the Russian political Twitter and shows high out-of-sample performance (Stukal et al. Reference Stukal, Sanovich, Bonneau and Tucker2017). This approach to bot detection relies on a machine learning algorithm that is trained on human-labeled data of Russian Twitter accounts and enables researchers to scale up human coding of Russian bots through training an ensemble of supervised classifiers. This method can also be trained and implemented for distinct periods as needed.
More specifically, this bot detection algorithm involves four main steps. First, it takes 10 random samples of the accounts that were annotated by a group of trained human coders who labeled the accounts as bots or humans. Then, for each of the sampled subsets of the data, it uses a variety of features measured at the account level to predict the assigned labels via training four different supervised classifiers (a ridge-penalized logistic regression, a support vector machine, an extreme gradient boosted tree, and an adaptive boosting binary classifier). Third, it uses a unanimous voting rule to aggregate the results of the four classifiers for each subset of the data. And fourth, it aggregates the results across all the subsets by applying a majority voting rule.
As discussed in much more detail in Stukal et al. (Reference Stukal, Sanovich, Bonneau and Tucker2017), the architecture of this bot detection tool is specifically designed to maximize precision over recall—that is, to minimize the probability that a human account would be predicted to be a bot (for more details, please refer to Online Appendix D). From a substantive perspective, this implies that we might miss some of the most sophisticated bots in this study, especially the ones that could deceive human coders. On the other hand, Stukal et al. (Reference Stukal, Sanovich, Bonneau and Tucker2017) have shown that the relatively unsophisticated bots detected by this method make up around half of all Twitter accounts that regularly tweeted about Russian politics in Russian during 2014–2016. Therefore, the types of bots we are able to study here comprise not only a sizeable portion of all bots but also a decent share of all Russian-language Twitter accounts—bots and humans—that discuss Russian politics. An additional reason for us for being conservative with bot detection is to ensure that the activity we analyze in this article is in fact automated and our findings properly characterize the usage of a large set of bots in Russia, thereby shedding new light on the information manipulation strategies available to modern nondemocratic regimes. Nevertheless, the limitations of the bot-detection algorithm we employ in this article also highlight the importance of continued research in this area, as the type of bots we identify and track during this period may or may not be the same types of bots that are prevalent in the 2020s.
Measuring the Political Orientation of Bots
Identifying Russian Twitter bots is not sufficient for studying the strategies of their deployment in the interests of the government in that previous research has shown that pro-government bots are not the only type of Twitter bots that are active in Russia. In fact, according to Stukal et al. (Reference Stukal, Sanovich, Bonneau and Tucker2019b), only 27% of bots operating in Russian political Twitter during this period were pro-government; the remaining 73% were either neutral (49%) or appeared to be friendly to regime opponents (24%).
For this reason, we moved beyond mere bot detection and employed another tool specifically designed to measure Russian bots’ political orientation via a multilayer feed-forward neural network (for details on this classifier, please refer to Online Appendix E). This orientation classifier demonstrated high performance in separating pro-government and antigovernment bots (Stukal et al. Reference Stukal, Sanovich, Bonneau and Tucker2019a).Footnote 4 As our primary interest lies in understanding how Russian pro-government actors use bots during periods of increased opposition activity (either offline or online), we focus on 1,516 pro-government Twitter bots (with over one million tweets in our collection in total) detected with high confidence in Stukal et al. (Reference Stukal, Sanovich, Bonneau and Tucker2019a). Although this is only a subset of all the detected pro-government bots in Russia, we focus on these accounts, as we can be confident that they are bots tweeting pro-Kremlin messages and that this set is large enough to inform us about patterns of bot activity.
Identifying Offline Protests
Since 2011, when the largest political protests in Russia under Putin started, there have been a number of important and prolific academic efforts to track and describe Russian protests (Gabowitsch Reference Gabowitsch2016; Greene Reference Greene2014; Lankina and Tertytchnaya Reference Lankina and Tertytchnaya2019; Lankina and Voznaya Reference Lankina and Voznaya2015; Robertson Reference Robertson2011; Volkov Reference Volkov and Ross2015). However, studying protest in almost real time is still a challenging research task that requires real-time data analytics and automated event detection technologies that are still open areas of research in computer science (Hasan, Orgun, and Schwitter Reference Hasan, Orgun and Schwitter2018). We make use of the database of the Integrated Crisis Early Warning System (ICEWS) project that automatically extracts information about events from news articles based on the pattern “who did what to whom, when, and where” (Boschee et al. Reference Boschee, Lautenschlager, O’Brien, Shellman, Starz and Ward2015). Different types of events have different codes in the database, including a variety of codes for subtypes of protests, which allows researchers to extract potential protest-related data for different countries without substantial time gaps. We extract information on all protests in Russia during 2015–2018 from the ICEWS database and manually validate the results by searching for any mentions of protest events on a given date in Russian and English-language mass media. We also augment the ICEWS database with additional protest events that it clearly missed. Finally, we restrict our attention to protests with at least 1,000 participants, as we believe these events are large enough that the Russian government would be motivated to take online action against them. The full list of protest rallies in Russia we analyze is reported in the Online Appendix I.
A potential limitation of the ICEWS data is its reliance on media reports. This might be problematic for studying protest in government-controlled media systems like Russia, as media might be required to underreport political protests. To address this concern, we conduct a set of robustness checks using an extended version of the protest data set that includes the augmented ICEWS data, data from Lankina and Tertytchnaya (Reference Lankina and Tertytchnaya2019), and the Mass Mobilization in Autocracies Database (Weidmann and Rød Reference Weidmann and Rød2019). We find substantively similar results (see Online Appendix J).
Identifying Spikes in Online Opposition Activity
To identify periods of increased online opposition activity, we count the total number of tweets that prominent opposition leaders or related organizations posted on their Twitter accounts. Specifically, we collected data on 15 Twitter accounts that belong to activists, independent journalists, or mass media with extensive and benevolent coverage of the Russian opposition (Table 2).
Table 2. Opposition and Independent Journalists

To identify spikes, we use a robust measure that compares daily numbers of tweets from these 15 accounts with the respective median over a two-month period. More specifically, we define a spike as a day with at least five times as many tweets from opposition accounts as they posted on a median day a month before and after that day. This measurement instrument identifies 24 days with spikes in the opposition tweeting activity. As shown in the Online Appendix G, only two of these spikes coincide with days of offline protests.
Measuring Effects
To measure the effect of protest rallies and spikes in opposition activity on bot strategies, we use maximum likelihood to estimate a hierarchical varying-intercept varying-slope Poisson regression model with the following parameterization of the conditional mean function:

where
$ {Y}_{it} $
is a count variable for the number of tweets pro-government bot i posted on day t; Protest
t
, Online
t
, and Placebo
t
are binary independent variables that equal 1 if there is a street protest, spike in the online opposition activity on day t, or day t is a randomly selected placebo day, respectively; and
$ {\gamma}_{m\left[t\right]} $
are month-year random effects.
This flexible parameterization (for alternatives, please refer to the Online Appendix N) allows us to overcome the limitation of constant-slope models that can fail to capture data heterogeneity by constraining regression coefficients to be the same across different groups of observations (Gelman and Hill Reference Gelman and Hill2007).
For the ease of interpretation, we focus on SAPD, simulated average predictive differences (Gelman and Hill Reference Gelman and Hill2007, Chapter 5.7), defined as follows:

where I is the total number of pro-government bots, M is the total number of year-months, s is the index for a simulated coefficient vector, and S is the total number of simulations.
SAPD is a discrete analogue of the simulated average marginal effects that shows the average change in the expected value of the dependent variable as the value of a binary independent variable shifts from zero to one. We make 1,000 draws from the sampling distribution of coefficient estimators (S = 1,000). Then, for each simulated coefficient vector, each pro-government bot, and each year-month, we compute the difference in the expected values of the dependent variable if there is a protest or online spike on a given day or if there is none and we average these differences across bots and month-years. Finally, we average the computed average differences over all simulated coefficients.
Finally, to accommodate the fact that we perform a large number of statistical tests in this study and control the probability of Type I errors, we employ the Bonferroni correction for 191 tests. In other words, we use the nominal confidence level of
$ 1-\frac{\alpha }{191} $
, where
$ \alpha \hskip1.5pt =\hskip1.5pt 0.05 $
.
Causality
The causal attribution of the observed SAPD to protest rallies or spikes in online opposition activity requires making certain assumptions. First, it requires that bots could change their behavior as a result of an intervention (e.g., via an intervention of a bot manager). We call this the manipulability assumption. This assumption would be violated if bots were preprogrammed to exhibit no temporal volatility. In that case, their tweeting activity would be constant over time. Our tweet volume dimension provides a simple test for this assumption, as it shows whether bots change the intensity of their tweeting during specific events.
Alternatively, this assumption would be violated if bots were preprogrammed to react to activists’ tweets.Footnote 5 In this case, bots and activists would show similar—if not identical—patterns of activity. However, as we show in Figure 2 (Section 1), a fivefold increase in the number of activists’ tweets per day (the online spikes dummy goes from 0 to 1) is on average associated with only a one-tweet increase in the activity of bots. Thus, there is little evidence of bots being preprogrammed to react to activists.

Figure 2. Volume of Tweets: Effect Size
Another assumption needed to causally interpret SAPD requires that the effect of protest rallies or spikes in online activity be separable from the effects of other events on the ground. We refer to this as the identifiability assumption. Although it is challenging to fully verify this assumption, we address the issue in the Online Appendix G by applying a structural topic model to the texts of the tweets and identifying topics that stand out as prevalent on days of offline protests and show that all these topics are related to protests or protest causes. This finding supports the validity of the identifiability assumption.
In addition, as we show in Online Appendix G, the effects of street protests are separable from the effects of spikes in the online activity of the Russian opposition, as only two of these spikes overlap with street protests.
RESULTS
We report the results for the offline demobilization and online agenda control hypotheses on each dimension separately. These dimensions refer to different strategies that could be employed for different purposes. Thus, juxtaposing empirical evidence for each hypothesis dimension-wise can provide additional insights about the potential motivation driving bot managers.
As the offline demobilization hypothesis implies bot activation either during or before protests without providing any further guidance about proper lag size, we address the lag issue empirically and consider cumulative lags from 0 to 3. Lag 0 means that the protest dummy equals one only for protest days, whereas for lag k (
$ 0<k\hskip1.5pt \le \hskip1.5pt 3 $
), the protest dummy equals one both for the day of the protest and for k previous days.
Finally, to provide a benchmark with which we can compare our findings, we also report the results for 10 placebo days randomly selected from outside of the seven-day periods around the actually observed protest days.
Volume Dimension
We start assessing the empirical support for the offline demobilization and online agenda control hypotheses by testing them along the volume dimension (H1a and H2a in Table 1). Figure 2 summarizes the results for the volume dimension with the 95% Bonferroni-corrected confidence intervals for SAPD.
As one can see from Figure 2, both hypotheses receive moderate empirical support, as pro-government bots increase their tweeting activity during street protests and on days of increased online opposition activity. The latter effect is 2.7 times as strong as the former.
Interestingly, the effect of online spikes drops significantly if the days leading up to or following the spikes are also included into the analysis, whereas the effects of street protests are rather stable on days before and after offline mobilization.
From a substantive perspective, the identified effects are relatively small, as each pro-government bot posts on average one additional tweet during online opposition mobilization and only around one-fourth of a tweet during protests. However, given their large share in the overall political activity on Twitter in Russia, the cumulative effect of an army of bots is much more sizable. Clearly, the benefit that bot managers can get from launching bots is due to scalability and their ability to produce large volumes of desired content in a concerted manner.
Retweet Diversity Dimension
On the retweet diversity dimension (H1b and H2b in Table 1), both the offline demobilization and online agenda control hypotheses predict that pro-government bots will retweet a wider range of accounts during specific days, which corresponds to what Roberts (Reference Roberts2018) refers to as the “flooding” strategy. Figure 3 presents the findings with the 95% Bonferroni-corrected simulated confidence intervals for SAPD.

Figure 3. Retweet Diversity: Effect Size
As one can see from Figure 3, there is evidence that pro-government bots do indeed employ the flooding strategy and increase the diversity of the retweeted sources on protest days and days with high online opposition activity. Again though, the effect is more pronounced for the online agenda control hypothesis and is around twice as large as the offline demobilization effect. The effect magnitude here should also be interpreted in terms of the concerted activity of a large number of bots rather than a potential contribution of a single bot.
Cheerleading Dimension
Figure 4 reports the results for the cheerleading dimension (H1c and H2c in Table 1). Contrary to our expectations, pro-government bots do not systematically increase their tweeting about the Russian president during mass political protests. Instead, the expected number of tweets that mention Vladimir Putin is smaller on protest days than on days without a protest.

Figure 4. Cheerleading: Effect Size
In contrast, for days of high online activity of opposition leaders we do find a statistically significant effect in the predicted direction. In other words, pro-government bots tend to tweet more about President Putin of Russia on these days, although the bot-level effect is of limited magnitude and equals around half a tweet per bot.
Negative Campaigning Dimension
Finally, we model the dependent variable that equals the number of tweets posted by pro-government bots mentioning Alexey Navalny (H1d and H2d in Table 1). As the pro-government bots we study in this article include the most explicitly partisan automated accounts (Stukal et al. Reference Stukal, Sanovich, Bonneau and Tucker2019a), we assume that all of their mentions of Navalny are critical. We focus on Alexey Navalny because he is both a prominent Russian opposition leader and known for his charisma and ability to bring large numbers of protesters to the streets (Nechepurenko Reference Nechepurenko2018).
Figure 5 reveals two interesting findings. First, the negative campaigning dimension is the only observable implication of our theories for which we find more activity in response to offline protests than online spikes. That being said, the size of these effects is also significantly smaller than those for any of the other three observable implications. Indeed, the online spike effects are barely distinguishable from the placebo effects, whereas the offline protests effects—while distinguishable from the placebo effects—are much smaller than any of the online spike effects from the previous three tests.

Figure 5. Negative Campaigning: Effect Size
CONCLUSION
Although there has been growing anecdotal evidence that nondemocracies use social media bots for a variety of different purposes, no previous attempts have been made to systematically analyze the strategies behind the political use of bots on a large scale. In this article, we seek to fill this gap by building on two distinct strands of literature in the areas of political behavior and political communication to develop and empirically test hypotheses about the strategic deployment of Twitter bots for fighting Russian domestic opposition. One hypothesis views bots as a tool for responding to opposition activity on the ground and demobilizing Twitter users from joining street protests, whereas the other conceptualizes the role of bots in Russian domestic politics as algorithms designed to exercise agenda control over the online information flows.
Empirically, we make use of a large collection of Russian-language Twitter data and apply bot detection tools that were previously designed to study Russian Twitter bots. Although our hypotheses are rejected on the negative campaigning dimension and results are mixed on the cheerleading dimension, we confirmed our hypotheses on the volume and retweet diversity dimensions. In other words, pro-government Twitter bots tweet more and retweet a more diverse set of accounts when there are large street protests or opposition activists post an unusually large number of tweets.
Even though the bot-level effects we detected are relatively small, bots are able to produce substantial shifts in the volume and sentiment of political tweets by acting en masse. A precise estimate of the overall effect of bots is still beyond the current state of bot-detection technologies, as multiple issues related to precision, recall, temporal and geographical consistency, and generalizability of existing bot-detection tools remain unsolved (Rauchfleisch and Kaiser Reference Rauchfleisch and Kaiser2020). In this paper, we measure the changes in the activities of 1,516 Russian bots that were previously identified with high confidence as pro-government. Thus, in cumulative terms, these bots produced on average around 1,500 extra tweets every day with increased opposition activity, and around 750 daily tweets were cheerleading for President Putin. However, these numbers should only be treated as a lower estimate for the overall effects of bots, as the existing literature also features other estimates of around 30,000 Russian pro-government bots on Twitter during 2014—2018 (Stukal et al. Reference Stukal, Sanovich, Bonneau and Tucker2019b).
Our findings are limited to the use of pro-government Twitter bots in Russia, and further research is required to better understand how generalizable these results are from the cross-platform or cross-national perspectives. Addressing the generalizability issue in further research is particularly important given the peculiarities of the Russian-language Twitter that largely remains a social media platform for activists and highly specialized communities (RIA-Novosti 2020). The outsized presence of activists and political journalists on Russian Twitter suggests that Russia follows the global trend of indirect but strong political influence of Twitter through its centrality to all stages of news production from sourcing, selection, and judgment to dissemination and amplification (Ingram Reference Ingram2018; Lopez-Rabadan and Mellado Reference Lopez-Rabadan and Mellado2019; Lukito et al. Reference Lukito, Suk, Zhang, Doroshenko, Kim, Su, Xia, Freelon and Wells2020; McGregor and Molyneux Reference McGregor and Molyneux2020). Uncovering the strategies behind the use of Russian-language pro-government bots remains highly important given abundant evidence that their use in democratic countries, including the United States, follows the templates tested within Russia (Sanovich Reference Sanovich, Woolley and Howard2018).
The results of this paper show that social media bots constitute a sort of “universal soldier” that can be activated for various purposes and in a variety of situations. They are however by no means the only digital tool nondemocratic regimes can use against domestic and international rivals (Sanovich, Stukal, and Tucker Reference Sanovich, Stukal and Tucker2018). Disinformation campaigns involving coordinated human activity (King, Pan, and Roberts Reference King, Pan and Roberts2017; Sobolev Reference Sobolev2018; Varol et al. Reference Varol, Ferrara, Davis, Menczer and Flammini2017), denial-of-service attacks (Lutscher et al. Reference Lutscher, Weidmann, Roberts, Jonker, King and Dainotti2019), special regulations on Internet service providers to block or remove online content (Deibert and Rohozinski Reference Deibert and Rohozinski2010; King, Pan, and Roberts Reference King, Pan and Roberts2013)—among other techniques employed to increase what Roberts (Reference Roberts2018) dubbed as fear, friction, and flooding—are other tools that can complement or substitute the deployment of social media bots. Although very little is known to date about the specific factors that drive autocrats’ choice of digital tools, bots—as we argue in this paper—are an easy-to-use and cheap technology that can be deployed not only down the chain of command inside the bureaucratic hierarchy but also in a decentralized manner by a plethora of rent-seeking agents.
Further research is required at the intersection of political and computer science to better understand the cross-national diversity of social media bots and their strategies and, thereby, to better understand how generalizable our results are. Additional research is also required to measure the effects of bots on how people perceive and evaluate information on social media (Edwards et al. Reference Edwards, Edwards, Spence and Shelton2014). Future scholarship should also advance our knowledge of the characteristics of bots that make them more or less appealing and trustworthy for different groups of the population (Freitas et al. Reference Freitas, Benevenuto, Ghosh, Veloso, Pei, Silvestri and Tang2015; Savvopoulos, Vikatos, and Benevenuto Reference Savvopoulos, Vikatos, Benevenuto, Brandes, Reddy and Tagarelli2018). This would help us to measure the effectiveness of bots and their future role in information manipulation and disinformation campaigns.
Supplementary Materials
To view supplementary material for this article, please visit http://doi.org/10.1017/S0003055421001507.
DATA AVAILABILITY STATEMENT
Research documentation and data that support the findings of this study are openly available at the American Political Science Review Dataverse: https://doi.org/10.7910/DVN/H8SIXG.
ACKNOWLEDGMENTS
We want to thank four anonymous reviewers. We are also very grateful to Andrey Akhremenko, Neal Beck, Noah Buckley, Charles Crabtree, Olga Gasparyan, Holger Kern, Tomila Lankina, Umberto Mignozzetti, Molly Roberts, Alexander Petrov, Arturas Rozenas, Zachary Steinert-Threlkeld, and the participants of the Carnegie Post-Communist Politics Workshop at NYU and Columbia (May 10, 2019) for great feedback and helpful suggestions. Sanovich gratefully acknowledges NYU Jordan Center for the Advanced Study of Russia Visiting Fellowship (May–June, 2019). Stukal acknowledges support from the Russian Science Foundation grant No. 21-78-00079. Tucker gratefully acknowledges the support of the National Science Foundation (Award #1756657). The NYU Center for Social Media and Politics (csmapnyu.org), which supported this research, is generously funded by the John S. and James L. Knight Foundation, the Charles Koch Foundation, the Hewlett Foundation, Craig Newmark Philanthropies, the Siegel Family Endowment, and NYU’s Office of the Provost. Stukal conducted all of the analysis and prepared the first draft of the manuscript. Stukal and Tucker developed the research design. Bonneau and Tucker oversaw the data collection process. Sanovich designed and oversaw the human labeling of Twitter accounts. All of the authors participated in revising and editing of the manuscript.
CONFLICT OF INTEREST
The authors declare no ethical issues or conflicts of interest in this research.
ETHICAL STANDARDS
The authors declare the human subjects research in this article was deemed exempt from review by NYU.
Comments
No Comments have been published for this article.