Implications
The advances that have been made in breeding goals have been based on the needs of breeders; however, they are also influenced by consumers and societal needs related to food safety and efficient and environmentally sound production systems. New functional traits are growing in importance because of recent declines in animal health and fitness. There is also growing competition for high-quality, plant-based sources of energy and protein; thus, it is important to use those resources very efficiently in animal production. Successful programmes for animal improvement will require a balance between the effort needed to record data and the resulting benefits to farmers.
Introduction
As a consequence of negative genetic correlations between milk yield and fitness traits, a decline in many functional traits has been observed (e.g. Jones et al., Reference Jones, Hansen and Chester-Jones1994; Lucy, Reference Lucy2001). In recent years, there has been a stabilization or even increase in genetic trends of functional traits (e.g. Miglior et al., Reference Miglior, Chesnais and Van Doormaal2012; ZuchtData, 2014). An example for the increase in genetic trend for longevity is shown for Holstein Friesian in Figure 1 (Fuerst C., 2014, personal communication). Herd management has been challenged to compensate for antagonistic effects and to balance selection for production while maintaining fertility, udder health and resistance to metabolic diseases in order to maximize profit without compromising welfare. As a result, there is increasing demand around the world for novel traits that can be used for herd management and genetic improvement, as well as for monitoring parameters of public interest.

Figure 1 Average estimated breeding value for longevity by birth year and country for Holstein Friesian (Fuerst, Reference Schöpke and Weigel2014).
The work needed to record additional data must provide immediate benefits to the producer in order to motivate the extra effort required. Individual producers place particular value on tools that can help them better manage their herds. If other stakeholders, such as veterinarians, are to be encouraged to record, for example, treatments and diagnoses, they also need to accrue benefits from the effort that they put in. As it is more and more challenging to balance high levels of production with reproduction and health, there is a growing need for data that can be used to detect problems at an early stage so that early interventions can be made.
The inclusion of functional traits in genetic improvement programmes is important for the long-term development of dairy populations. Genetic gains are cumulative, and small improvements that build up over time will provide ongoing savings to all farmers, particularly those using selection indices to combine information from many different traits. In response to these needs, there has been a worldwide shift towards more emphasis on functional traits in selection programmes over the past 15 years. Complex breeding goals that include up to 43 functional traits/country are encompassed by total merit indices (TMI) according to a survey carried out by ICAR in 2012 (Stock et al., Reference Stock, Cole, Pryce, Gengler, Bradley, Andrews and Egger-Danner2012), and the responses from 23 countries showed that genetic evaluations for calving ease, fertility, longevity, feet and legs and indirect health traits are very common. However, a wider range of health traits are actually being recorded, and there are plans in a number of countries to further expand their recording programmes to include additional reproduction, metabolic and efficiency traits. Public interest in the use of parameters for monitoring food safety, tracking disease outbreaks and documenting animal welfare is increasing. National monitoring programmes may therefore be developed to meet the demands of producers, consumers and regulators. The objectives of this paper are to give an overview of expected developments and challenges related to the availability of novel traits, with emphasis on new health and fitness traits in the near future, and to suggest strategies for phenotyping of new traits.
Background
The world population is estimated to reach 9 billion people in 2050 according to the latest demographic reports from the United Nations (2014), which will require a big increase in food production. Demand for limited resources will increase and prices for energy and concentrates will rise. Disruptions in global environments due to climate change (see, e.g. Walthall et al., Reference Walthall, Hatfield, Backlund, Lengnick, Marshall, Walsh, Adkins, Aillery, Ainsworth, Ammann, Anderson, Bartomeus, Baumgard, Booker, Bradley, Blumenthal, Bunce, Burkey, Dabney, Delgado, Dukes, Funk, Garrett, Glenn, Grantz, Goodrich, Hu, Izaurralde, Jones, Kim, Leaky, Lewers, Mader, McClung, Morgan, Muth, Nearing, Oosterhuis, Ort, Parmesan, Pettigrew, Polley, Rader, Rice, Rivington, Rosskopf, Salas, Sollenberger, Srygley, Stöckle, Takle, Timlin, White, Winfree, Wright-Morton and Ziska2012) may also drive a greater emphasis on novel traits, especially those that optimize resource use efficiency. Cattle producers will be challenged to find ways to reduce greenhouse gas emissions throughout the production cycle, either by increasing production efficiency and reducing wastage or by using new technologies to decrease pollution. In this context, there is need for novel traits that can be used in herd management and breeding. Functional traits, such as direct information of cow health, have also increased in importance because of growing concerns about animal welfare and consumer demands for healthy and natural products. Consumers are also concerned about the impact of drugs used in veterinary medicine on the spread of antibiotic-resistant strains of bacteria that can negatively impact human health. These requirements are also a part of legal regulations in the European Union (Pavon, Reference Pavon2013).
According to a scientific report on the effects of farming systems on dairy cow welfare and disease (EFSA-Q-2006-113, 2009), the major diseases associated with poor welfare in dairy cows were leg disorders, mastitis and reproductive disorders. Pavon (Reference Pavon2013) stated that genetic selection of dairy cattle over many years is considered by the European Commission to be a major factor leading to poor welfare in dairy cows, which has driven a need for welfare indicators. However, only healthy cows can perform at high levels of production for a long time, and it is important to ensure that consumers and policy makers understand this.
A key requirement for the recording of data is the motivation of the stakeholders involved. Several countries have conducted surveys with farmers to understand their needs (Steininger et al., Reference Steininger, Fuerst-Waltl, Pfeiffer, Fuerst, Schwarzenbacher and Egger-Danner2012; Roessler et al., Reference Roessler, Herold, Weidele and Zarate2013; Schwarzenbacher, Reference Schwarzenbacher2013). A consistent observation in these surveys has been that farmers place priority on the genetic progress of functional traits, aiming at robust cows that are easy to handle. Increasing milk yield is no longer ranked among the most important traits to select for, but it is quite possible that survey respondents are indicating a desire for healthy, long-lived cows that are also highly productive. Growing emphasis on functional traits indicates that they are perceived as being more important than in the past – for example, because herd sizes and labour costs are increasing – but that does not necessarily mean that production is unimportant.
According to Bo (Reference Bo2009), a breeding goal should include the following aspects: increased income (higher production of milk/beef); reduced costs (better fertility, fewer diseases, reduced culling rates); ease of management (temperament, milking speed); and advantages regarding the sale of products (animal welfare, ethics, consumer concerns). Complex breeding goals also require information on a wide range of relevant traits that can be measured economically. In dairy cattle, these traits include efficiency, health, fertility and functional conformation. Many countries now use a diverse group of economically important traits in the TMI to rank cattle for genetic selection (Figure 2). However, recording has to have benefits beyond genetic improvement programmes, and the additional effort required for documentation must result in added value. Electronic systems that make data capture easy are a key to long-term success.

Figure 2 Traits included in the total merit indices of 17 countries. The data used to construct this figure were provided by F. Miglior of the Canadian Dairy Network.
Advances in technology will have an impact on the future definition and availability of phenotypes. The growing use of SNP-based genotyping may lead to routine genomic evaluations of new traits with relatively few daughter records, particularly if producers are willing to use breeding values with lower reliabilities than they are accustomed to. Phenotypes that are expensive to measure, such as direct feed intake, may be handled by establishing small reference populations with very thorough data recording. Those data could be used to develop predictors for the broader population based on traits that are recorded in most herds (e.g. Cole et al., Reference Cole, Waurich, Wensch-Dorendorf, Bickhart and Swalve2014). Recent technological developments will provide more knowledge about the biological background and genetic architecture of traits (RNAseq, genome sequencing, genomics, proteomics, metabolomics, etc.). Sequencing technologies can be used to identify causal mutations, rather than SNP in linkage disequilibrium with causal mutations, which should lead to increased accuracies of genomic prediction (Meuwissen et al., Reference Meuwissen, Hayes and Goddard2013). Metabolomics (the study of cellular metabolites present within an organism) and proteomics (the study of protein structure, function and patterns of expression within an organism) should lead to a better description of phenotypes, which could enhance selection opportunities.
Novel functional traits
Direct health traits
Several studies show that the use of direct health traits (e.g. mastitis diagnoses) is more effective than indicator traits alone in breeding programmes (Heringstad et al., Reference Heringstad, Klemetsdal and Steine2007; Egger-Danner et al., Reference Egger-Danner, Willam, Fuerst, Schwarzenbacher and Fuerst-Waltl2012a; Parker Gaddis et al., Reference Parker Gaddis, Cole, Clay and Maltecca2014). The Nordic countries have a well-established history of health recording in dairy cows. In Norway, each case of veterinary treatment has been registered on an individual cow basis since 1975 (Heringstad and Østerås, Reference Heringstad and Østerås2013; ICAR, 2013). Similar recording systems were established in Finland, Sweden and Denmark during the 1980s. Routine genetic evaluations for direct health traits have been in place in Austria and Germany since 2010, in France since 2012 and in Canada since December 2013. Systems for recording of diagnoses are presently being established in other countries as well and will be more widely available in the near future. Some countries will use veterinary diagnoses, whereas others will focus on producer-recorded data. Developments towards integrated use of health data require standardization of diagnoses to ensure that phenotypes are comparable. Gernand et al. (Reference Gernand, Rehbaein, von Borstel and König2012), Govignon-Gion et al. (Reference Govignon-Gion, Dassonneville, Baloche and Ducrocq2012), Parker Gaddis et al. (Reference Parker Gaddis, Cole, Clay and Maltecca2012) and Pryce et al. (Reference Pryce, Esslemont, Thompson, Veerkamp, Kossaibati and Simm1998) have shown that, for use in genetic evaluations, common health disorders recorded by farmers are of a similar quality as those documented by veterinarians. Combined use of health data from farmers and diagnosis documented by veterinarians may be an option to improve coverage of direct health data. In 2012, ICAR approved guidelines for Recording, Evaluation and Genetic Improvement of Health Traits (ICAR, 2012). A hierarchical system with a very comprehensive key of diagnoses (>900 entries), a reduced key of diagnoses (60 to 100 entries) and a simple key with about 10 diagnoses was described, enabling multidisciplinary use ranging from detailed information for veterinarians to simple recording of health-related observations by farmers (Table 1). International co-operation for comparability of results across countries is important.
Table 1 Example of use of the hierarchical health key published by ICAR (2012)
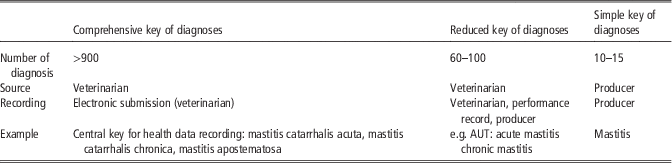
Udder health
Mastitis is the most common trait related to udder health. In most countries, somatic cell count (SCC) is obtained from samples processed in milk-recording programmes and used as an indirect measure of mastitis; the genetic correlation between mastitis and SCC is much lower than 1 (e.g. Heringstad et al., Reference Heringstad, Gianola, Chang, Ødegård and Klemetsdal2006; Negussie et al., Reference Negussie, Strandén and Mäntysaari2008). Heritability and genetic correlation estimates are presented in Table 2. Recent research has focussed on alternative definitions of SCC, such as prolonged elevated SCC (e.g. de Haas et al., Reference De Haas, Ouweltjes, ten Napel, Windig and de Jong2008; Koeck et al., Reference Koeck, Heringstad, Egger-Danner, Fuerst, Winter and Fuerst-Waltl2010a; Urioste et al., Reference Urioste, Franzen and Strandberg2010). These novel definitions showed higher correlations with mastitis and demonstrated that temporal changes in SCC provide additional information about mastitis resistance. However, such analyses may require more frequent collection of samples than is common in many milk-recording programmes. Genetic evaluations for clinical mastitis (CM) have been computed in the Nordic countries for >35 years, followed by Austria, and are now common in other countries as well (see the ‘Direct health traits’ section). Udder health indices combining SCC and CM (and udder conformation traits) are published in some countries.
Table 2 Heritabilities for novel traits

SSS=somatic cell count; AMS=automated milking systems; FPCM=fat and protein corrected milk.
Several new methods for the detection of mastitis have been proposed. Electrical conductivity (EC) is available from some automated milking systems (AMS) and can be used as an indicator of mastitis. Collecting and implementing EC information in a breeding programme may be a challenge (Norberg, Reference Norberg2005), and recent literature on that topic is limited. Haeusermann and Hartung (Reference Haussermann and Hartung2012) analysed data from near IR spectroscopy, real-time PCR and IR thermography to determine whether they are useful indicators of mastitis. They concluded that the development and testing of new detection methods for mastitis will require further work. On-farm or in-line utilization of the data is not applicable for all of the techniques examined, and there is the challenge of defining a ‘gold standard’ for the prevention of mastitis. Recent advances in the capacity of large-scale prediction of lactoferrin (Soyeurt et al., Reference Soyeurt, Bastin, Colinet, Arnould, Berry, Wall, Dehareng, Nguyen, Dardenne, Schefers, Vandenplas, Weigel, Coffey, Théron, Detilleux, Reding, Gengler and McParland2012a) and mineral content indicator traits (Soyeurt et al., Reference Soyeurt, Bruwier, Romnee, Gengler, Bertozzi, Veselko and Dardenne2009), both known to be associated with mastitis, could also offer new opportunities to select for improved mastitis resistance (Soyeurt et al., Reference Soyeurt, Bastin, Colinet, Arnould, Berry, Wall, Dehareng, Nguyen, Dardenne, Schefers, Vandenplas, Weigel, Coffey, Théron, Detilleux, Reding, Gengler and McParland2012a and 2012Reference Soyeurt, Dehareng, Gengler and Dardenneb). Research has also been carried out on the use of pathogen-specific information in the genetic evaluation of mastitis (de Haas et al., Reference De Haas, Barkema and Veerkamp2002; Sorenson et al., Reference Sorenson, Madsen, Mark and Lund2009; Haugaard et al., Reference Haugaard, Heringstad and Whist2012). Different bacteria may cause different immune responses, and the severity of infection and response to therapeutics can differ between pathogens. It has been shown that prevention programmes for heifer mastitis can be more efficient when information about pathogens is known (De Vliegher et al., Reference De Vliegher, Fox, Piepers, McDougall and Barkema2012). The most significant challenge is in obtaining microbial cultures for a large number of animals because the data are expensive to collect relative to SCC or producer-reported diagnoses of CM.
Reproduction
Reproduction consists of several different traits. To establish a recording scheme for female fertility, the following data are desirable: (1) calving dates; (2) all artificial insemination dates as well as natural mating dates wherever possible; (3) information on fertility disorders; (4) pregnancy test results; (5) body condition score (BCS); and (6) hormone assays (ICAR, 2014). Routinely used fertility traits are mainly based on calving and insemination data. However, the use of fertility-related diagnoses is increasing, which envisions improved selection for reproductive health. Physical activity increases during oestrus. In addition, there are other behavioural changes, such as standing heat and mounting behaviour. These signs can be used to detect oestrus and can be used to calculate traits such as interval between calving and resumption of oestrus. It is probably unrealistic to routinely collect such data without automating heat detection. For example, pedometers and more sophisticated activity monitors are now being used routinely on many farms as part of a herd management package. The pedometer information needs to be compared with a baseline for the same cow, and algorithms have been developed to interpret the data collected. The efficiency of the oestrus detection rate has been reported to range between 50% and 100% depending on the criteria of success (At-Taras and Spahr, Reference At-Taras and Spahr2001). The ‘gold standard’ for oestrus detection is serum progesterone, and comparisons of pedometer – with progesterone-determined oestrus – has shown that activity monitors cannot detect silent behavioural oestrus (Lovendahl and Chagunda, Reference Lovendahl and Chagunda2010). However, an advantage of both progesterone- and activity-determined oestrus is that they do not require direct observations by farm workers. Novel predictors of fertility, such as pedometers and BCS, are growing in popularity (Fogh et al., Reference Fogh, Aamand, Hjorto and Lauritsen2013). Several researchers have shown that BCS has a favourable relationship with fertility (e.g. Pryce et al., Reference Pryce, Coffey and Simm2001; Berry et al., Reference Berry, Buckley, Dillon, Evans, Rath and Veerkamp2003). Research on the use of mid-infrared (MIR) predicted fatty acids as indicator traits for fertility (Bastin et al., Reference Bastin, Berry, Soyeurt and Gengler2012) and of MIR for pregnancy status testing is also underway (Gengler et al., 2013).
Labial position is associated with urovagina, a condition in which urine accumulates in the cranial portion of the vagina, which has harmful effects on fertility (Gautam and Nakao, Reference Gautam and Nakao2009).
Genomics offers new possibilities for the discovery of harmful recessive effects on fertility based on the absence of homozygous haplotypes (VanRaden et al., Reference VanRaden, Olson, Null and Hutchison2011). A number of causative mutations have been identified using SNP genotypes and whole-genome sequence data (Adams et al., Reference Adams, Sonstegard, VanRaden, Null, Van Tassell and Lewin2012; Fritz et al., Reference Fritz, Capitan, Djari, Rodriguez, Barbat, Baur, Grohs, Weiss, Boussaha, Esquerré, Klopp, Rocha and Boichard2013; Sonstegard et al., Reference Sonstegard, Cole, VanRaden, Van Tassell, Null, Schroeder, Bickhart and McClure2013; McClure et al., Reference McClure, Bickhart, Null, VanRaden, Xu, Wiggans, Liu, Schroeder, Glasscock, Armstrong, Cole, Van Tassell and Sonstegard2014), and a deletion with positive effects on milk yield and negative effects on fertility has recently been identified in Nordic Red cattle (Kadri et al., Reference Kadri, Sahana, Charlier, Iso-Touru, Guldbrandtsen, Karim, Nielsen, Panitz, Aamand, Schulman, Georges, Vilkki, Lund and Druet2014). In Fleckvieh (Simmental) and Brown Swiss, deleterious mutations compromising reproductive and rearing success in cattle have been discovered (Pausch et al., Reference Pausch, Schwarzenbacher, Burgstaller, Flisikowski, Wurmser, Jansen, Schnieke, Wittek and Fries2014a and Reference Pausch, Kölle, Wurmser, Schwarzenbacher, Emmerling, Jansen, Trottmann, Fuerst, Götz and Fries2014 Reference Pausch, Kölle, Wurmser, Schwarzenbacher, Emmerling, Jansen, Trottmann, Fuerst, Götz and Friesb; Schwarzenbacher et al., Reference Schwarzenbacher, Fuerst, Fuerst-Waltl and Dolezal2012).
These methods are amenable to automation, and in the United States and several other countries the process of searching for new recessives is now a part of the genomic evaluation system.
Feet and legs
Foot and leg conditions rank among the three most frequent reasons for culling after reproduction and udder health. The conformation of feet and legs is recorded routinely by linear-type classification systems that are often part of the services offered by breed societies. Feet and leg conformation traits may be useful indicator traits for claw health. They cannot replace direct measures of claw health because their genetic correlations with claw disorders are low, but they can be used to increase the reliability of EBVs (Häggmann and Juga, Reference Häggmann and Juga2013; Chapinal et al., Reference Chapinal, Koeck, Sewalem, Kelton, Mason, Cramer and Miglior2013). Koenig et al. (Reference Koenig, Scharifi, Wentrot, Landmann, Eise and Simianer2005 and Reference Koenig, Köhn, Kuwan, Simianer and Gauly2006), Koenig and Swalve (Reference Koenig and Swalve2006) and Linde et al. (Reference Linde, de Jong, Koenen and Eding2010) showed that the efficiency of breeding for improved claw health increases considerably when claw health data are included. Routine electronic recording systems for claw health at the time of trimming exist in the Nordic countries (Johansson et al., Reference Johansson, Eriksson, Nielsen, Pösö and Aamand2011; Häggmann et al., Reference Häggmann and Juga2013; Ødegård et al., 2013), and have been introduced in other countries as well (Kofler, Reference Kofler2013). Ødegård et al. (2013) showed, based on Norwegian data, that breeding for claw health is possible, and genetic evaluations based on data from claw trimming will be implemented in the breeding programme for Norwegian Red cattle in 2014.
The most frequently available information is locomotion scoring (http://www.zinpro.com/lameness/dairy/locomotion-scoring). Weber et al. (Reference Weber, Stamer, Junge and Thaller2013) suggest that lameness might be a useful indicator for claw and leg health. Automated lameness detection based on activity sensors that measure lying time, number of lying bouts combined with milking and feeding data based on a cow-specific model could be a useful tool for dairy management, according to De Mol et al. (Reference De Mol, Andre, Bleumer, van der Werf, de Haas and Reenen2013). Giuliana et al. (Reference Giuliana, Kaler, Remnant, Cheyne, Abbott, French, Pridmore and Huxley2014) showed that lameness results in behavioural changes in dairy cows in AMS. Lame cows had less feeding time and visited the robot less frequently compared with sound cows. In the Nordic countries, Austria and Southern Germany, veterinary diagnosis of feet and leg traits is recorded routinely. Several studies found that genetic evaluations based on veterinary diagnoses are valuable (e.g. Fuerst-Waltl et al., Reference Fuerst-Waltl, Fuerst and Egger-Danner2012). As veterinarians are typically consulted only in the most severe cases, incidence rates based on veterinary diagnoses are much lower than those based on hoof trimming information. For effective improvement of the feet and legs complex, it is important to establish systems that allow the collection of data from hoof trimmers.
Metabolic status
To date, direct traits for metabolic disorders are only rarely used in genetic evaluation. Germany and Austria publish EBVs for milk fever for Fleckvieh (Simmental) and Brown Swiss. The Nordic countries include this information in the EBV for general health. In herd management, milk content traits such as fat and protein percentage, fat : protein ratio, and milk urea nitrogen are used for early detection of problems associated with metabolism. Recent research has focussed on using this information for genetic improvement as well (Koeck et al., Reference Koeck, Miglior, Jamrozik, Kelton and Schenkel2013; Negussie et al., Reference Negussie, Stranden and Mäntysaari2013). One of the limitations with this approach is that if the intervals between milk recording are too long, the predictive ability is limited. Automated milk-recording systems could fill this gap by providing more frequent observations (Nicolazzi et al., Reference Nicolazzi, Biscarini and Stella2012). Subclinical metabolic disorders like ketosis are thought to account for substantial economic losses; thus it may be important to detect subclinical signs at an early stage. These could be based on serum parameters like non-esterified fatty acids or ß-hydroxybutyric acid (BHBA) concentration (Robert et al., Reference Roberts, Chapinal, LeBlanc, Kelton, Dubuc and Duffield2012; Van der Drift et al., Reference Van der Drift, Jorritsma, Schonewille, Kmnijn and Stegeman2012). BHBA is the ‘gold standard’ of many cowside tests. The availability of such subclinical information will depend on the specificity and sensitivity of the tests as well as on ease and costs of implementation on-farm. Other potential indicator traits include MIR spectroscopy-based traits (Bastin et al., Reference Bastin, Soyeurt, Vanderick and Gengler2011b; McParland et al., Reference McParland, Banos, Wall, Coffey, Soyeurt, Veerkamp and Berry2011) or rumen activity, and BW change (Fogh et al., Reference Fogh, Aamand, Hjorto and Lauritsen2013). Melzer et al. (Reference Melzer, Wittenburg, Harwig, Jakubwoski, Kesting, Willmitzer, Lisec, Reinsch and Repsilber2013) showed that there might be potential in the identification of important metabolites that can help monitor the metabolic profile of a cow. Roche et al. (Reference Roche, Friggens, Kay, Fischer, Stafford and Berry2010) have shown a relationship between BCS and metabolic disorders. The risk of metabolic disorders is higher when BCS is below 2.5 or above 3.5.
Calving traits
Breeding values related to calving ease and stillbirth are commonly available (Interbull, 2013). Genetic evaluations usually differentiate between direct and maternal and first- and later calvings, and there is substantial variation among countries in the models used for evaluations. Although most countries use single-trait models (summarized in Cole et al., Reference Cole, Wiggans and VanRaden2007, Table 1), Eaglen et al. (Reference Eaglen, Coffey, Wooliams and Wall2012) showed that multiple-trait models may be better than single-trait models, and suggest that aggregate breeding values may be an effective way of ensuring that producers place emphasis on both direct and maternal traits. Breeds differ with respect to rates of dystocia and stillbirth, and evaluations are sometimes available only for some of the breeds in a country (e.g. Cole et al., Reference Cole, Goodling, Wiggans and VanRaden2005; Yao et al., Reference Yao, Weigel and Cole2014). Health disorders related to calving difficulties are recorded in some countries.
Recent studies showed the potential of decreasing losses during the rearing period. Fuerst-Waltl and Fuerst (Reference Fuerst-Waltl and Fuerst2010) and Fuerst-Waltl and Sorensen (Reference Fuerst-Waltl and Sorensen2010) investigated the genetic background of postnatal mortality in calves and replacement heifers in different age groups until first calving in Danish Holsteins and Austrian Simmentals. Genetic and phenotypic variation seems to be sufficiently high to genetically improve the trait of calf and heifer mortality. Some research also has been conducted on the health traits of Holstein calves in the United States (Mousa et al., Reference Mousa, Seykora, Chester-Jones, Ziegler and Cole2013), but heritability estimates were low, ranging from 0.01 to 0.06. In a genetic analysis of respiratory disease in Norwegian Red calves, Heringstad et al. (Reference Heringstad, Chang, Gianola and Østerås2008) found that reasonably precise genetic evaluations of sires for calf diseases could be calculated, despite the low frequency of those diseases.
In this context, information about raising stock is important. Heifers that cycle and do not get pregnant or heifers that are inseminated but are culled before calving are of interest. Reasons also may include conformational or behavioural issues (Bethard, Reference Bethard2008). The trait longevity is included in the total merit index in most of the countries. However, losses during rearing are generally not considered, or are included in a very simple manner. Mortality in dairy cattle is relevant not only with regard to economic losses but also with regard to animal health and welfare. McCorquodale et al. (Reference McCorquodale, Sewalem, Miglior, Kelton, Robinson, Koeck and Leslie2013) showed the potential of using calfhood diseases and survival in future dairy cattle breeding programmes.
Feed efficiency
An expected increase in prices for concentrates and energy will increase the focus on feed efficiency. Efficiency can be defined as output per input unit at the farm level, as well as for individual animals. Residual feed intake (RFI), also known as net feed efficiency, is growing in popularity as a measure of feed efficiency. RFI is generally calculated as the difference between actual and predicted dry matter intake (DMI). Efficient animals have lower DMI at the same level of milk production and the same weight. The greatest obstacle in the widespread use of feed intake and feed efficiency measures in breeding objectives is the availability of large quantities of feed intake data on individual animals (Berry and Crowley, Reference Berry and Crowley2013). There are two promising options for enabling selection for RFI: (1) predictor traits (Fogh et al., Reference Fogh, Aamand, Hjorto and Lauritsen2013) and (2) genomic prediction (Pryce and Berry, Reference Pryce and Berry2014; Pryce et al., Reference Pryce, Wales, de Haas, Veerkamp and Hayes2014a and Reference Pryce, Gonzalez-Recio, Thornhill, Marett, Wales, Coffey, de Haas, Veerkamp and Hayes2014b).
Rumen activity might be a future indicator trait for feed efficiency. Other measures in feed, faeces and urine samples are being developed – for example, metagenomic predictions using the rumen microbiome (symbiotic microorganisms) of enteric methane in cattle and body mass index in humans are showing promise (Ross et al., Reference Ross, Moate, Marett, Cocks and Hayes2013) and could possibly also be used for predicting RFI (De Marchi et al., Reference De Marchi, Toffanin, Cassandro and Penasa2014). In a recent review by Pryce et al. (Reference Pryce, Wales, de Haas, Veerkamp and Hayes2014a), which included seven studies of beef and dairy cattle, the accuracy of genomic predictions of RFI, energy balance and DMI ranged between 0.20 and 0.43. In theory, a genomic reference population can be assembled using a sub-population of animals with genotypes and phenotypes used to generate a genomic prediction equation (Berry et al., Reference Berry, Wall and Pryce2014; Pryce et al., Reference Pryce, Wales, de Haas, Veerkamp and Hayes2014a and Reference Pryce, Gonzalez-Recio, Thornhill, Marett, Wales, Coffey, de Haas, Veerkamp and Hayes2014b). The genomic prediction equation can then be applied to the rest of the population. However, farmers are unlikely to accept accuracies in the range currently estimated (<16%). This means that much larger populations need to be assembled. An international collaboration is endeavouring to build a data set of >6000 individuals with genotypes and phenotypes (from nine contributing partners) (Berry et al., Reference Berry, Wall and Pryce2014; Pryce and Berry, Reference Pryce and Berry2014; Pryce et al., Reference Pryce, Wales, de Haas, Veerkamp and Hayes2014a and 2014Reference Pryce, Gonzalez-Recio, Thornhill, Marett, Wales, Coffey, de Haas, Veerkamp and Hayesb). Selection for RFI, or RFI, has been reported to lead to reductions in methane emissions of ~13.45 g CH4/kg RFI (Hegarty et al., 2007) and 18.2 g CH4/kg RFI (Nkrumah et al., Reference Nkrumah, Okine, Mathison, Schmid, Li, Basarab, Price, Wang and Moore2006). A similar potential for abatement has been observed in Holstein dairy cattle, 17.5 g CH4/kg RFI (Pryce et al., unpublished data). Reducing RFI by 1 kg could lead to between 4% and 8% reduction in methane emissions. If RFI was to be included in the Australian national breeding objective, the Australian Profit Ranking, then it is predicted that RFI would reduce by 1.76 kg/cow per year (Gonzalez-Recio et al., Reference Gonzalez-Recio, Pryce, Haile-Mariam and Hayes2014a). Direct measurement of methane is expensive and is unlikely to be available in sufficient numbers for breeding in the near future, unless novel ways of measuring methane on large numbers of animals that are currently being tested prove to be successful. Chagunda et al. (Reference Chagunda, Ross and Roberts2009) reported that a laser methane detector can be used to accurately estimate enteric methane output in dairy cows without affecting their normal activity. Dehareng et al. (Reference Dehareng, Delfosse, Froidmont, Soyeurt, Martin, Gengler, Vanlierde and Dardenne2012) and McParland et al. (Reference McParland, Lewis, Kennedy, Moore, McCarthy, O’Donovan, Butler, Pryce and Berry2014) reported the potential capacity to provide MIR spectra-based methane indicators. Such systems may be an attractive way to collect many phenotypes if they are cost-effective and durable.
Other novel traits
Behavioural traits
Other traits, such as temperament, are of growing interest because farmers want cows that are easy to handle. From the aspect of animal welfare, these traits are gaining interest as well. So far, little information about heritabilities of behavioural traits is available, but commercial dairymen cull animals because of poor temperament (Berry et al., Reference Berry, Harris, Winkelman and Montgomerie2005). Temperament or behaviour is often subjectively scored. According to Kramer et al. (Reference Kramer, Erbe, Bapst, Bieber and Simianer2013), heritabilities based on phenotypes scored by independent people were lower than when scored by farmers (0.12 v. 0.20). Kramer et al. (Reference Kramer, Erbe, Bapst, Bieber and Simianer2013) analysed traits including general temperament, milking temperament, aggressiveness, rank order in herd, milking speed and position of labia for Brown Swiss. General temperament was scored by farmers using a 5-point scale (1=very nervous and 5=very calm) as described by Juga (Reference Juga1996). The heritability for general temperament was 0.38, that for aggressiveness was 0.12 and that for milking temperament was 0.04.
Traits out of data from AMS
AMS offer the possibility to record traits related to workability, which are described by Rinell (Reference Rinell2013). Temperament or behaviour, ease of handling and milkability are examples of traits that could be derived from measures routinely recorded in AMS. A measure of temperament from the AMS system could be teat cup attachment failures. Rinell (Reference Rinell2013) estimated genetic correlations of r=−0.38 and r=−0.50 between two teat cup attachment failure traits and temperament. Different approaches concerning milking speed are used. Data from AMS offer new possibilities to record such traits automatically (Byskov et al., Reference Byskov, Buch and Aamand2012; Carlström et al., Reference Carlström, Petterson, Johansson, Strandberg, Stålhammar and Philipsson2013a and 2013b). Carlström et al. (Reference Carlström, Petterson, Johansson, Strandberg, Stålhammar and Philipsson2013b) compared milkability from conventional milking parlours with AMS systems. They showed genetic correlations of 0.93 and 1.00 between the two systems and concluded that the inclusion of only one trait in breeding programmes is sufficient. Lovendahl et al. (Reference Lovendahl, Lassen and Chagunda2012) also discuss milking efficiency, in addition to milking speed. Milking efficiency describes the yield of ECM per minute in the milking box and is of interest for automated milking. This includes time for entry, cleaning, attachment, milking, exit and unit cleaning.
Adaptation to climate change
Exposure of animals to extreme weather conditions can be used as proxy for future climate change. Such exposure will trigger autonomous adaptations by the animals, but these adaptations are not perfect and therefore residual impacts or vulnerabilities will remain (Smit et al., Reference Smit, Burton, Klein and Street1999). By quantifying these non-adaptations, novel traits can be defined to assess the degree of resilience of an animal to climate change. Resilience can be defined in many ways, the simplest of which may be the individual response of an animal to a stressor variable linked to the weather. A commonly used stressor variable is the temperature heat index, initially developed by Thom (Reference Thom1959) as a heat index for human comfort, and is commonly used as indicator of heat stress. Reported research in this field addressed individual responses in both production and functional traits and reported clear trait differences, fertility and intake being often considered the most strongly affected (e.g. Kadzere et al., Reference Kadzere, Murphy, Silanikove and Maltz2002; De Rensis and Scaramuzzi, Reference De Rensis and Scaramuzzi2003; West, Reference West2003). Recent research also reported responses in some novel traits having a direct biological meaning. Gengler (Reference Gengler2014) reported, in this context, the reaction of MIR-predicted fatty acid C18:1-cis9 content in milk to heat stress. Several authors have shown that this trait reflects very well the equilibrium between mobilization and intake – heat-stressed animals eating less and mobilizing more (e.g. De Rensis and Scaramuzzi, Reference De Rensis and Scaramuzzi2003). This element is of large importance when considering both adaptation to and mitigation of climate change in climate conscious breeding objectives. More detailed physiologically based adaptation traits such as heat shock proteins have been proposed (Lewis et al., Reference Lewis, Handy, Cordi, Billinghurst and Depledge1999), but large-scale phenotyping is obviously more difficult to organize and these traits are therefore less useful for practical breeding.
Currently, despite promising research results, in cattle, no large-scale breeding programme directly includes adaptation traits. However, current breeding objectives are indirectly affecting adaptation given the unfavourable correlations between resilience and production traits (e.g. West, Reference West2003). Although profound scientific knowledge about the detailed relationships of resilience traits with other traits might still be missing, it can be hypothesized that more resilient animals are also more robust animals (i.e. more fertile) in general.
Sucking traits
Another trait of interest is suckling behaviour. Cross-suckling and inter-suckling are considered abnormal behaviours in cattle and constitute a common problem in dairy farming (Fuerst-Waltl et al., Reference Fuerst-Waltl, Rinnhofer, Fuerst and Winckler2010). De Passillé (Reference De Passillé2001) noted that deprivation of the opportunity to suck may interfere with digestive processes and used an artificial feeding system to examine the effects of milk flow rate on calf behaviour. In a review of dairy cow behaviour, Von Keyserlingk et al. (Reference Von Keyserlingk, Rushen, de Passillé and Weary2009) concluded that calf feeding systems that allow the expression of normal behaviours, such as sucking on a teat, are best for the animals. Studies of oral behaviours in calves are typically based on direct observations, and the general consistency of independent studies suggests that the methodology used to record those behaviours is adequately standardized (e.g. Margerison et al., Reference Margerison, Preston, Berry and Phillips2003).
Milk fatty acids
Fine milk composition traits, and in particular fatty acid profiles, can be considered another group of traits of interest (Gengler and Soyeurt, Reference Gengler and Soyeurt2010). The importance of this type of traits is double: first, directly as indicators of milk quality (Bauman et al., Reference Bauman, Mather, Wall and Lock2006) and, second, also as indicators of the physiological and health status of the animals (e.g. Bastin et al., Reference Bastin, Soyeurt, Vanderick and Gengler2011b). Some studies established a link between milk composition and the environmental footprint of cows for nitrogen (Jonker et al., Reference Jonker, Kohn and Erdman1998) and for methane (Mohammed et al., Reference Mohammed, McGinn and Beauchemin2011), these emissions being linked to fatty acid profiles. Unfortunately, measuring fine milk composition, and especially fatty acid composition, is not feasible on a large scale in an economical fashion as reference chemical analyses are very expensive and time-consuming. Recently, through the use of MIR spectroscopy, the prediction of most major fatty acids has become feasible on a large scale (Soyeurt et al., Reference Soyeurt, Dehareng, Gengler, McParland, Wall, Berry, Coffey and Dardenne2011), creating new opportunities for the recording and use of novel fine milk composition traits, in particular fatty acids.
Milk coagulation properties
Several physical characteristics of milk that are of importance in cheese manufacture have been described in the literature, including rennet coagulation time, curd firmness 30 min after rennet addition, and curd-firming time (Bittante et al., Reference Bittante, Penasa and Cecchinato2012). These traits are largely genetically independent of milk yield (Ikonen et al., Reference Ikonen, Morri, Tyrisevä, Ruottinen and Ojala2004), and there is interest in improving those traits in many breeds (Ikonen et al., Reference Ikonen, Ahlfors, Kempe, Ojala and Ruottinen1999; Cecchinato et al., Reference Cecchinato, Penasa, De Marchi, Gallo, Bittante and Carnier2011). One limiting factor in providing such evaluations is the time and expense involved in collecting the phenotypes, but recent research suggests that MIR spectroscopy may provide reasonable predictors that can be measured on many samples affordably (Cecchinato et al., Reference Cecchinato, De Marchi, Gallo, Bittante and Carnier2009).
Beef traits
Carcass traits are routinely recorded at slaughter houses and are available for genetic evaluation. This includes traits like dressing percentage, trading score and information about net daily gain. According to a survey carried out by Gene2Farm, tenderness is another trait that is more widely recorded for beef breeds. The availability of beef quality traits is still limited and under research (e.g. Gene2Farm). As the collection of beef quality traits is very expensive, genomics offers the chance to set up registration of these traits for a limited number of genotyped animals. A detailed review of this topic is outside the scope of this study.
Recording systems and sources of data for novel traits
For accurate breeding values there are two major possibilities: either cost-effective phenotypes are required on a large scale or investment must be made in the genotyping and collection of high-cost phenotypes for a limited number of females. Owing to advances in technologies, new traits based on lab data or automation are offering new possibilities. Many precision dairy farming technologies, including daily milk yield recording, milk component monitoring (e.g. fat, protein and SCC), pedometers, automatic temperature recording devices, milk conductivity indicators, automatic oestrus detection monitors and daily BW measurements, are already being utilized by dairy producers (Bewley, Reference Bewley2010). To invest in this equipment is expensive and will only pay for large farms. According to the review by Rutten et al. (Reference Rutten, Velthuis, Steeneveld and Hogeveen2013), sensor systems for mastitis and reproduction are brought to a high level of development, but still need to improve detection performance.
Laboratory data include indirect health data with a spectrum of traits according to sampling protocols and testing requests (e.g. microbiological testing, metabolite analyses, hormone tests, virus/bacteria DNA, IR-based measurements) (Soyeurt et al., Reference Soyeurt, Bruwier, Romnee, Gengler, Bertozzi, Veselko and Dardenne2009). The advantage of lab data is that, in general, they are automated or semi-automated recording systems that produce objective measurements, and many data can be obtained at low cost. The disadvantage might be that, for example, for bacteriological findings or hormonal assays – data might be only from preselected animals.
To use synergies and enlarge the range of possible phenotypes for genetic collaboration with other organizations and institutions is an option (Figure 3). Data security issues have to be considered, and standardized protocols for ensuring security are necessary. Slaughter houses, dairy plants, health organizations and other entities are routinely recording data for their own purposes. The monitoring of welfare is gaining increasing importance. The central role of dairy cattle health in the context of animal welfare and consumer protection implies that farmers and veterinarians are obligated to maintain high-quality records, emphasizing the particular sensitivity of health data. The legal requirement to monitor the development of different indicator traits offers the possibility of synergies for recording of traits. One example is direct health data, which are partly recorded based on legal documentation requirements (Stege et al., Reference Stege, Bager, Jacobsen and Thougaard2003; Østerås et al., Reference Østerås, Solbu, Refsdal, Roalkvan, Filseth and Minsaas2007; Egger-Danner et al., Reference Egger-Danner, Fuerst-Waltl, Obritzhauser, Fuerst, Schwarzenbacher, Grassauer, Mayerhofer and Koeck2012b).

Figure 3 Pooled database with potential data sources and examples of use of data. grey=commonly used data; light grey=partly used data; white=data sources of interest.
Genotyping females in research herds
As several novel traits currently under investigation are expensive to record, using research herds for phenotyping in combination with genomics are an attractive source of data, as are herds that engage in intensive data collection. These farms are of particular interest for feed efficiency, methane emission and high-cost health traits. As several thousand phenotypes are required to accurately determine the characteristics of traits in terms of heritability and correlations to other traits of interest in the breeding goal (Calus et al., Reference Calus, de Haas, Pszczola and Veerkamp2013a), there is a clear need to invest in phenotyping of many animals for the new traits, as well as possible indicator traits (Gonzalez-Recio et al., Reference Gonzalez-Recio, Coffey and Pryce2014b).
In breeding programmes worldwide, more and more young bulls with genomic expected breeding values (GEBVs) are used. The reliability of the GEBVs depends to a very high extent on the size of the reference population. To achieve genetic response for the novel trait, the reliability of the trait is crucial. The limitation for novel traits where phenotyping has only recently started is that it will be very difficult or impossible to establish a large reference population based on bulls, as the number of bulls used within breeding programmes has been reduced because of genomic selection. Methods to increase the accuracy of genomic predictions are as follows: enlarging the reference population, using chips of higher density, imputing from lower densities to higher densities and the inclusion of ungenotyped cows in the reference population (Pszcola et al., Reference Pszczola, Veerkamp, de Haas, Wall, Strabel and Calus2013).
Enlarging the reference population by adding females is also a possibility. Simulation studies (e.g. Buch, Reference Buch2011; Pszcola et al., Reference Pszczola, Veerkamp, de Haas, Wall, Strabel and Calus2013; Gonzalez-Recio et al., Reference Gonzalez-Recio, Coffey and Pryce2014b) show that for novel traits and limited resources it is more effective to genotype females than only males. The impact of the cow reference population also depends on the sampling strategy. Including only elite dams will only result in minor increases of reliabilities and may lead to biased estimates. Exploring the variation of the trait by sampling extreme cows (top tails and bottom tails of distribution) will increase the benefit (Jimenez-Montero et al., Reference Jimenez-Montero, Gonzales-Rezio and Alenda2012). However, it is desirable to either randomly select cows or select those that will contribute the most data, which is the strategy being applied in the Australian genomic information nucleus known as Ginfo (Australia’s genomic information nucleus). Traits with medium to high heritability gain more from genotyping cows than direct health traits with low heritability (Egger-Danner et al., Reference Egger-Danner, Schwarzenbacher and Willam2014). Bolignon et al. (Reference Bolignon, Long, Albuquerque, Weigel, Gianola and Rosa2012) showed that reference population animals with extreme yield deviations are the most informative for genomic selection. De Haas et al. (Reference De Haas, Calus, Veerkamp, Wall, Coffey, Daetwyler, Hayes and Pryce2012) have shown that it is possible to increase the number of genotypes and phenotypes by merging data from various research herds worldwide. Validation of equations in completely independent populations showed promising results (Pryce et al., 2014b). Different studies stress the optimum design of female reference populations to use resources efficiently (Buch, Reference Buch2011; Pszczola et al., Reference Pszczola, Strabel, Mulder and Calus2012; Thomasen et al., Reference Thomasen, Sorensen, Lund and Guldbrandtsen2014). It is important that the variance of the trait can be explored and that the relationship within the reference population is low but closely related to the evaluated population. Therefore, it is important to update the reference population continuously.
Experiences from the United States showed that there is some gain from the inclusion of cows in the predictor population (Wiggans et al., Reference Wiggans, Cooper, VanRaden and Cole2011), but adjustments to their records may be needed to avoid potential biases (Dassonneville et al., Reference Dassonneville, Baur, Fritz, Boichard and Ducrocq2012; Wiggans et al., Reference Wiggans, VanRaden and Cooper2012). The use of cows in predictor populations for novel traits that lack a large group of bulls with high-reliability evaluations will be necessary. Table 3 gives an overview of gEBV reliabilities for novel traits. So far, experience is limited with real data. However, it is unclear whether or when low-reliability cow data should be removed from the predictor population as the reliability of sire EBV increases.
Table 3 Reliabilities of genomic EBVs for novel traits

GEVB=genomic estimated breeding value; RDC=red dairy cattle; DYD=daugher yield deviation; RFI=residual feed intake; *=accuracy.
Cost efficiency is an important criterion for the success and sustainability of breeding programmes. Therefore, it might be too expensive to continuously record data extensively on research herds. Indictor traits with known genetic correlation to the direct trait of interest may be used instead. According to Pszcola et al. (Reference Pszczola, Veerkamp, de Haas, Wall, Strabel and Calus2013), easily recorded predictor traits could be used to increase the reliability of scarcely recorded traits – for example, fat- and protein-corrected milk yield and live weight for DMI – and the bias of genomic breeding values of scarcely recorded traits could be reduced. As described under the various trait complexes, this approach could be applicable also for metabolism, feet and legs and other traits (Axelsson et al., 2013). Genomic selection programmes for indicator traits also benefit from the inclusion of cows in the reference population (Calus et al., Reference Calus, de Haas, Pszczola and Veerkamp2013a and Reference Calus, Bijma, van Arendonk and Veerkamp2013b).
Challenges
Predictive biology
Biomarkers, such as metabolites or milk components, may be rich sources of information about novel phenotypes (Gengler et al., Reference Gengler, Berry and Bastin2013). Standard analyses undertaken by milk-recording laboratories, such as by MIR spectroscopy, generate spectral data that reflect many characteristics of milk. Research is currently underway to investigate the use of this data for prediction of indicator traits (RobustMilk, OptiMIR, PhenoFinlait, GplusE, etc.). The main challenge posed by these data is the lack of reference data for the detection of disease, such as mastitis. A reliable pool of ‘healthy’ and ‘sick’ animals is required. For traits with low heritability and limited reliability and repeatability, this is even more difficult. The reference data set has to account for different production circumstances. There is the need for comparability of spectra from different instrument manufacturers (Foss, Bentley, Delta, etc.), models within brands, and stability over time. The repeatability of results across different breeds and production environments, as well as logistical challenges related to data collection and transfer, must be considered. This includes extracting data from spectrometers and storing of MIR spectra, standardizing spectra, complex computation of indicators and finally the implementation into routine milk-recording work flow (Soyeurt et al., 2012Reference Soyeurt, Dehareng, Gengler and Dardenneb and Reference Soyeurt, Dehareng, Romnée, Gengler and Dardenne2012c; Gengler et al., Reference Gengler, Berry and Bastin2013).
Predictive biology is also a topic for other sensor techniques. According to a review by Rutten et al. (Reference Rutten, Velthuis, Steeneveld and Hogeveen2013), research is still needed. In the future, genomic data could be integrated to improve the prediction. Different environmental effects, such as feeding and management, can influence the prediction equations. Therefore, there is also a need for calibration across breeds and countries (De Marchi et al., Reference De Marchi, Toffanin, Cassandro and Penasa2014). Prediction equations are derived using reliable phenotypes from a large sample of animals, with the ultimate goal of predicting phenotypes directly from the MIR spectra. In Figure 4, the system of prediction is described. The prediction equation is derived based on reliable phenotypes for novel traits (e.g. mastitis) from a larger sample of animals. The ultimate goal is to predict phenotypes directly from, for example, MIR spectra. Advances could be that these equations are improved by taking the genomic information into account.

Figure 4 Overview about the system of predictive biology to determine traits based on prediction equations.
Standardization and integration of relevant data sources
There are many mechanical and electronic systems on dairies that can be used as sources of new phenotypes (Figure 5). Automatic milking and feeding systems, for example, routinely generate many observations. Among them, which data are actually stored is again different. There is a need for harmonization in trait definitions across systems and devices. An additional challenge is the availability of data. Many systems use process computers that do not communicate with external databases. This results in data that are ‘stranded’ in different systems that cannot easily be integrated into a single database for analysis. When such data are available in a central database, they can also be used for benchmarking, which offers an opportunity to compare performance both between and within farms over time. A single parameter does not provide an appropriate benchmark, and indices based on a basket of parameters may provide a better marker of overall performance (Bradley et al., Reference Bradley, Breen, Hudson and Green2013). Benchmarks also provide farmers with rapid feedback about the effect of management changes on farm performance, and may encourage ongoing participation in data collection programmes.
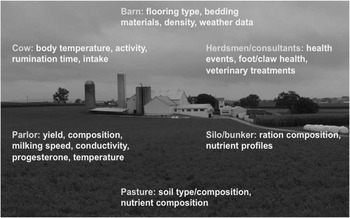
Figure 5 Sources of on-farm information that can be used to collect health and fitness phenotypes (source: http://commons.wikimedia.org/wiki/File:Amish_dairy_farm_3.jpg).
Simplicity and ease-of-use for farmers
The future availability of phenotypic data will depend heavily on the motivation of the farmers. It is expected that the traits and data available will further increase. The challenge is to present those data in a way that does not overwhelm farmers when providing useful tools. Easy handling and simple access to data is essential because time is a limiting factor on most farms.
Conclusions
Conditions of production, as well as consumer demands, will have an impact on the future availability of phenotypes. Traits connected with animal health, animal welfare, food safety and efficiency will become more important. Advances in technology will enable more precise trait definitions, with phenotypes closer to the genotype. Technological advances will permit the closer integration of existing data sources. New technologies are expected to provide better indicator traits for fertility, mastitis, metabolism and energy efficiency. There are still challenges to solve before some technologies can be widely applied on a routine basis, including the predictive biology of indicator traits, the harmonization of data from automated systems, the motivation of farmers to record high-quality phenotypes and the genomic gap for novel traits. To overcome the phenomic gap between traditional traits and novel traits, expanded genotyping of cows should be considered. International co-operation will ensure that trait definitions are consistent across countries and will support the research necessary to deliver new management and selection tools to farmers.
Acknowledgements
This review is based on an invited presentation at the 64th Annual Meeting of the European Association for Animal Production held in Nantes, France, August 2013. Excellent cooperation within ICAR Working Group Functional Traits. Federal Ministry of Agriculture, Forestry, Environment and Water Management (BMLFUW) in Austria and the Federation of Austrian Cattle Breeders for the support within the projects ‘Efficient cow’ and ‘OptiGene’. Gene2Farm (EU-FP7-KBBE-2011-5-PNr.: 289592) for provision of information. J. B. Cole was supported by appropriated project 1265-31000-096-00, ‘Improving Genetic Predictions in Dairy Animals Using Phenotypic and Genomic Information’, of the Agricultural Research Service of the United States Department of Agriculture. N. Gengler was supported by the INTERREG IVB NWE Project 190G OptiMIR: new tools for a more sustainable dairy sector and the Ministry of Agriculture of the Walloon Region of Belgium (Service Public de Wallonie, Direction générale opérationnelle ‘Agriculture, Ressources naturelles et Environnement’ - DGARNE) for the support within the project ‘MethaMilk’ (D31-1248 and D31-1304). J. E. Pryce acknowledges financial support from the Department of Environment and Primary Industries (Melbourne, Australia).