Implications
Behaviour is one of the important indicators in animal welfare assessments and can be considered in many dimensions, including the affective state, health and needs of animals. Technological development offers automated and continuous detection of poultry behaviour, so farmers can take actions to improve welfare. This review focuses on different sensor technologies to detect behaviours of broilers or laying hens, which are applied to analyse animal welfare conditions. It has been shown that sensor-based systems can monitor animal welfare and behaviour continuously, precisely and automatically with the potential to develop precision livestock farming systems.
Introduction
Currently, there is rising concern about poultry welfare, and poultry breeding should be conducted humanely with regard to feeding and slaughtering. Simultaneously, healthy poultry and product quality rely heavily on good welfare conditions, which can improve economic efficiency (Wathes et al., Reference Wathes, Kristensen, Aerts and Berckmans2008). A good state of welfare encompasses birds having a healthy body, a positive affective state and the opportunity to express natural behaviours (Sih et al., Reference Sih, Bell, Johnson and Ziemba2004). However, there are various indexes for the comprehensive assessment of animal welfare, such as behavioural indicators, physiological stress and proxies of productivity. Many of them interact together, making evaluation difficult and time-consuming, especially at the commercial level. Among these welfare indexes, behaviour is the most easily understood and commonly used welfare assessment index (De Jong et al., Reference De Jong, Hindle, Butterworth, Engel, Ferrari, Gunnink, Moya, Tuyttens and van Reenen2016), because behaviours which may be influenced by living conditions, emotions and diseases of animals can reflect physiological conditions. Therefore, monitoring animal behaviours and formulating feeding and management measures accordingly can not only promote the growth of animals and improve the production performance, but also protect animal welfare.
Precision livestock farming (PLF) is defined as the application of process engineering principles and techniques to livestock farming to automatically monitor, model and manage animal production (Tullo et al., Reference Tullo, Fontana, Diana, Norton, Berckmans and Guarino2017). The aim of PLF is to real-time monitor animal health and welfare automatically and continuously and prompt warning messages rapidly. Monitoring the resource use of chickens can improve the housing design and allocate resources optimally (Ringgenberg et al., Reference Ringgenberg, Frohlich, Harlander-Matauschek, Toscano, Wurbel and Roth2015; Li et al., Reference Li, Zhao, Oliveira, Verhoijsen, Liu and Xin2017). Through the location of chicken clustering, the quality of house facilities (e.g., feeders, drinkers) may be examined (Fraess et al., Reference Fraess, Bench and Tierney2016). Detecting the activity of poultry, such as eating, drinking, moving and perching, may estimate whether they are healthy and therefore provide early warning of diseases (Colles et al., Reference Colles, Cain, Nickson, Smith, Roberts, Maiden, Lunn and Dawkins2016; Dawkins et al., Reference Dawkins, Roberts, Cain, Nickson and Donnelly2017). Accordingly, the great potential of PLF relies on early warning, which allows farmers to take actions in the initial stages of welfare problems or diseases. However, to truly achieve PLF, it is necessary to monitor individual animals. Research on individual behaviour can control the health and production of every individual and improve the accuracy of welfare assessment. For example, detecting the movements of each chicken by a radio-frequency identification (RFID) system, the chickens can be classified into active, normal and sick level (Zhang et al., Reference Zhang, Hu, Chen, Guo, Duan and Wang2016). Therefore, each sick chicken can be found in time. Compared with large animals (e.g., cattle and pig), poultry are not only small, but raised in hundreds, thousands or hundreds of thousands in a commercial farm, making it difficult to monitor automatically and individually. However, despite that, PLF is able to provide new opportunities to promote animal husbandry with high-efficiency, low-cost, ecological and sustainable development and offers consumers some food safety assurance (Berckmans, Reference Berckmans2014).
The PLF system is based on sensors (e.g., RFID devices, accelerometer, camera, microphone and temperature sensor) and collects a variety of information (e.g., activity, body temperature, feed intake and weight) used for environmental monitoring, individual recognition and behaviour analysis. A range of sensor techniques are available that allow efficient and continuous data collection from multiple sources to monitor animal behaviour, health and production. Furthermore, advanced data processing techniques make it possible to analyse animal behaviour continuously, automatically, accurately and in real time (Valletta et al., Reference Valletta, Torney, Kings, Thornton and Madden2017; Van Hertem et al., Reference Van Hertem, Rooijakkers, Berckmans, Fernandez, Norton, Berckmans and Vranken2017). Ben Sassi et al. (Reference Ben Sassi, Averós and Estevez2016) reviewed the sensors and technologies for poultry welfare monitoring based on the literature prior to 2015. The review emphasised that the monitoring technologies could be implemented at the commercial level but did not analyse the difficulties of commercial application.
The aim of this paper is to discuss the possibility of accomplishing PLF though review of automatic methods implemented to monitor behaviours of broilers or laying hens at the experimental or commercial level in the last 5 years. The review expounds automated techniques, including sound analysis, RFID devices and image processing, which have been applied to poultry welfare and behaviour analysis, with discussion of the detection aspects of these techniques, advantages and disadvantages on a commercial scale, and feasibility for PLF.
Sound analysis
Vocalisations of animals contain a wealth of biological information (e.g., their social interactions, alarm signals and behaviour needs) and have the possibility to be used as a welfare indicator (Manteuffel et al., Reference Manteuffel, Puppe and Schon2004). When applied to poultry systems, the microphone is always put low near the animals and can capture sounds continuously and non-invasively, including pecking, vocalisations and environmental noises. The sound analysis techniques usually take the duration, energy distribution, frequency and formant amplitude as the characteristic parameters to estimate the behaviour and health of birds. It can be automated, in real time, and is capable of long-term monitoring without disturbing the birds.
Growth rate detection
Monitoring feed conversion, growth rate and BW is important to improve the efficiency and economic benefits of broiler farmers. Considering the relationship between pecking behaviour and eating, 90% of the feed intake of one broiler was correctly detected with pecking sounds in an experimental pen (Aydin et al., Reference Aydin, Bahr, Viazzi, Exadaktylos, Buyse and Berckmans2014). In another study, 86% of the feed intake of 10 broilers was detected accurately (Aydin et al., Reference Aydin, Bahr and Berckmans2015). Subsequently, short-term feeding behaviours of 10 broilers by pecking sound analysis were estimated in real time, and the accuracy of meal size reached 90%, meal duration reached 95%, the numbers of meals per day reached 84% and feeding rate reached 89% (Aydin and Berckmans, Reference Aydin and Berckmans2016). The above three papers were studied in an experimental pen of at most 10 chickens. However, the application to a commercial farm needs to be validated, including filtering out environmental noise and feasibility of individual detection with large bird numbers.
Broiler vocalisations are highly correlated with BW, and sound analysis could be a reliable method of measuring the growth rate of broiler chickens. In an intensive broiler farm, a study showed that with the growth of broiler chickens, the peak frequency of the sounds emitted by broilers decreased clearly during the entire life of the birds (Fontana et al., Reference Fontana, Tullo, Butterworth and Guarino2015 and Reference Fontana, Tullo, Scrase and Butterworth2016). Furthermore, a model to predict the weight of broilers was built and validated by the peak frequency analysis of the broilers’ vocalisations (Fontana et al., Reference Fontana, Tullo, Carpentier, Berckmans, Butterworth, Vranken, Norton, Berckmans and Guarino2017). Vocalisations could thus be used to assist in the automatic monitoring of the growth of broiler chickens.
Disease detection
There is a difference between the sound of healthy and unhealthy chickens. Analyses of the frequency domain features of 15 infected Clostridium perfringens type A chickens and 15 healthy chickens, classified by a supervised learning neural network, showed a classification accuracy of 66.6% 2 days after infection and reached 100% accuracy 8 days after infection (Sadeghi et al., Reference Sadeghi, Banakar, Khazaee and Soleimani2015). Using data-mining methods and Dempster–Shafer evidence theory, a device was made to detect whether a chicken was infected with Newcastle disease, bronchitis virus, avian influenza or healthy (Banakar et al., Reference Banakar, Sadeghi and Shushtari2016). The device could determine the type of disease in each chicken without surrounding noises.
Others
Sound analysis could identify the sex of 1-day-old chickens as well as different genetic strains (Cobb and Ross chickens) (Pereira et al., Reference Pereira, Naas and Garcia2015). Analysing four acoustic sound characteristics showed that the second spectral peak of the sound spectrum could identify the sex of chicks, and both the second spectral peak and the pitch could identify the genetic strain. Stress, such as temperature, fear and physical stress, can affect the health and production of commercial chickens. The sounds of chickens under heat or fear stress were detected in a commercial farm (Lee et al., Reference Lee, Noh, Jang, Park, Chung and Chang2015). The proposed system was able to classify different stresses by support vector machine (SVM) techniques automatically and non-invasively, and both the stress detection accuracy and the average classification accuracy were greater than 96%. The vocal variations of hens anticipating different types of rewards were investigated and showed that different sound cues made different vocal parameters produced by hens (McGrath et al., Reference McGrath, Dunlop, Dwyer, Burman and Phillips2017). It was possible to use the vocal communication of hens as indicators of welfare (e.g., health, stress, growth).
In summary, sound analysis is able to be used as an early warning system by interpreting the behaviours of chickens, predicting health conditions and detecting growth rates. The group sound analysis, which was tested commercially, can isolate the sounds of each bird, but it is impossible to find the exact bird which makes the sound. The sound collection and analysis of individual chicken, which can distinguish the chicken, is still at experimental stage due to the difficulty of collecting each individual sound in a commercial farm. Like voiceprint recognition, if the voice feature model of each bird could be established, the group sound collection can also match chickens to their sounds. Meanwhile, environmental noise is also a big challenge. With the maturation of voice recognition technology and the rapid development of artificial intelligence (AI), animal sound analysis has great potential as an online detection method to improve health and welfare in the poultry industry.
Tracking location and locomotion of individuals by radio-frequency identification
Wireless wearable sensors (e.g., accelerometer, RFID microchips) are mainly used to track the location and activity of individuals remotely. These sensors can distinguish the behaviours of individuals automatically, particularly when human observations are difficult. Siegford et al. (Reference Siegford, Berezowski, Biswas, Daigle and Gebhardt-Henrich2016) reviewed the technologies, especially RFID, which was applied as a research tool for tracking individual laying hens’ activity and location. However, RFID technology is rarely used in commercial poultry farms compared with farms producing larger animals (e.g., pigs and cattle), due to the small size and light weight of individual birds, the required size of the on-bird microchips and high financial investment cost. Radio-frequency identification technology is one method for automatic identification and data capture, which can be used mainly under experimental conditions to track the location of individual birds. An RFID microchip can also be injected to the leg or back of a bird produces a signal to the RFID reader when the antenna is within the magnetic field and the signal is sent to the central information system to identify and position the individual. Tracking the location of individual birds may allow observation of nesting, feeding, drinking, perching behaviour or ranging behaviour and, thus, prediction of health conditions.
Detecting nest use
Put RFID reader into nest and worn RFID tags on the leg of hens can detect a hen in and out of a nest. A smart nest box was designed to detect the laying behaviour of individual hens (Chien and Chen, Reference Chien and Chen2018). A low-frequency RFID system was used to detect whether hens were in the nest. An egg detection sensor could detect whether hens laid eggs and then weigh the eggs. Design-based Internet of Things enabled all data to be recorded simultaneously in the cloud database and a local SD card at the same time. Assigning each egg to an individual can achieve product traceability and analyse the laying performance of individuals. The system had the potential to monitor the underperforming hens in egg production due to welfare problems.
Establishing hens’ visits to the nest can improve the nest design. Using the Gantner Pigeon System GmbH (Schruns, Austria) RFID system, researchers found that the nest choice was more affected by the nest position, nest occupancy and age of hens than by the appearance of nest curtains (Ringgenberg et al., Reference Ringgenberg, Frohlich, Harlander-Matauschek, Toscano, Wurbel and Roth2015). Detecting laying behaviour could also examine the relationship between laying eggs and keel bone deviations and fractures (Gebhardt-Henrich and Frohlich, Reference Gebhardt-Henrich and Frohlich2015).
Tracking movement and feeding behaviour of individuals
Radio-frequency identification systems can track the position of individual birds to detect the movement and duration at a particular location. With a low-frequency RFID system, an environmental preference chamber with four compartments and two tiers was designed to study the movement in different compartments of four individual birds (Sales et al., Reference Sales, Green, Gates, Brown-Brandl and Eigenberg2015). Compared with video observation, successful detection rates were 62.6 ± 11.2%. The detection range of the antenna, the scan interval of the antenna and the RFID tags conflicts would affect the RFID system’s performance.
By monitoring the weight and moving time of individual hens using weighing sensors and RFID devices, poultry behaviours such as speed, ability to acquire food and resting time were detected, and by the K-means clustering method, chickens were classified as active, normal or sick (Zhang et al., Reference Zhang, Hu, Chen, Guo, Duan and Wang2016). An ultra-high-frequency RFID system was developed to monitor the feeding and nesting behaviours of 60 individual hens in enriched colony housing (Li et al., Reference Li, Zhao, Oliveira, Verhoijsen, Liu and Xin2017). Using the same experimental device, feeding behaviour was assessed with various feeder spaces (12.0, 9.5, 8.5 and 6.5 cm/hen) (Oliveira et al., Reference Oliveira, Xin and Wu2018). This type of research could contribute to housing system design, animal welfare and resource allocation.
Ranging behaviour in free-range housing
Currently, the free-range system is considered to be more natural and better for poultry welfare. However, each hen within a group has a different ranging behavioural pattern. To monitor the outdoor ranging behaviour of individual chickens, RFID systems have been used by attaching tags on chickens and putting antennas at pop holes, through which chickens move inside and outside (Gebhardt-Henrich et al., Reference Gebhardt-Henrich, Toscano and Frohlich2014; Campbell et al., Reference Campbell, Hinch, Dyall, Warin, Little and Lee2017; Larsen et al., Reference Larsen, Cronin, Gebhardt-Henrich, Smith, Hemsworth and Rault2017). The frequency and duration of visiting the outdoor areas are then calculated by analysing data from chickens walking over the antennas. These systems could be used in commercial farms and potentially track thousands of birds. In addition, an RFID system based on ultra-wideband technology was developed for monitoring 42 individual chickens’ locations in the 100 m × 100 m experimental field, which could calculate the position with 0.29 m of the median error and 68% of the registration rate (Stadig et al., Reference Stadig, Ampe, Rodenburg, Reubens, Maselyne, Zhuang, Criel and Tuyttens2018a). Monitoring of outdoor range use at the individual level allows for correlation between ranging and welfare indicators such as tonic immobility test, plumage damage, foot health and spatial cognition (Campbell et al., Reference Campbell, Hinch, Downing and Lee2016, Reference Campbell, Hinch, Downing and Lee2018a and Reference Campbell, Talk, Loh, Dyall and Lee2018b; Hartcher et al., Reference Hartcher, Hickey, Hemsworth, Cronin, Wilkinson and Singh2016; Larsen et al., Reference Larsen, Hemsworth, Cronin, Gebhardt-Henrich, Smith and Rault2018; Taylor et al., Reference Taylor, Hemsworth, Groves, Gebhardt-Henrich and Rault2018).
The impact of wearing a tag
Because the birds are small, the size, shape, weight and attachment method of tags might influence bird behaviour and the accuracy of the data. The behavioural and physiological responses of laying hens which wore backpacks containing automated monitoring devices were studied and showed that the backpacks only had a very minor effect on their behaviour after a short acclimation period (≤2 days) (Buijs et al., Reference Buijs, Booth, Richards, McGaughey, Nicol, Edgar and Tarlton2018). Furthermore, the effects on broiler behaviour, leg health, cleanliness and growth were evaluated with a tag worn on the back of birds for tracking location in a field (Stadig et al., Reference Stadig, Rodenburg, Ampe, Reubens and Tuyttens2018b). Six pens with 10 birds in each were observed with 5 birds fitted with a 36-g backpack and the other five with coloured markings to identify individuals. Footpad and hock dermatitis, gait and cleanliness were scored manually. These results indicated that birds wearing backpacks showed less activity early on, but no significant differences were observed in long-term behaviours between tagged birds and coloured birds. In summary, compared to the weight of chickens, a light monitoring tag has little impact on the behaviours of chickens after a period of adjustment. Wireless sensors are a reliable tool for detecting the location and activity of individual chickens.
Image processing
Image processing technology has been applied to automatically monitor animal behaviour for the analysis of animal welfare, health conditions, weight prediction and tracking. Image processing used to detect chicken flocks can be automatic, non-invasive and continuous. Using automated computer monitoring, it is possible to record and identify different behaviours of chickens, which is an inexpensive and cost-effective approach. Typical materials and methods of image processing used in chicken behaviour detection, such as camera type, software, algorithms and application area, are shown in Table 1.
Table 1 Typical materials and methods of automated image processing used in chicken behaviour monitoring
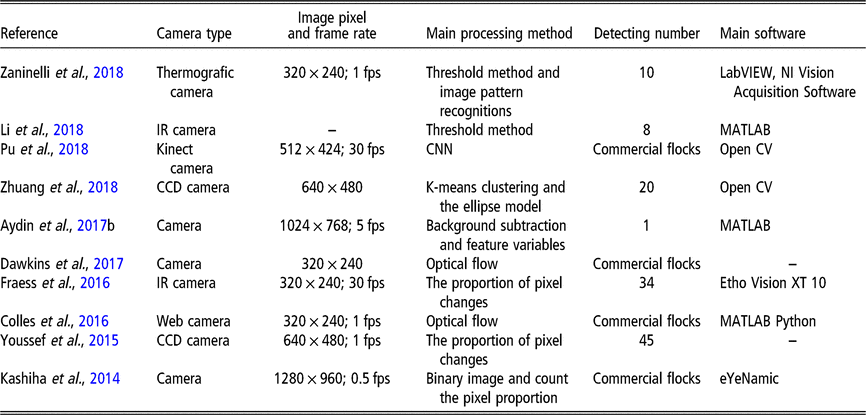
CCD = charge coupled device; CNN = convolutional neural network.
Flock activity analysis
Through the number of changed pixels between two adjacent frames divided by the overall number of pixels in the image, the flock activity level can be calculated (Youssef et al., Reference Youssef, Exadaktylos and Berckmans2015; Fraess et al., Reference Fraess, Bench and Tierney2016). The method is heavily influenced by plumage differences. Group activity around the food trough was assessed by the percent pixel change (Fraess et al., Reference Fraess, Bench and Tierney2016). The study showed that the method could identify changes in activity level, identify abnormal activity away from the feeder and serve as an early health warning system.
Optical flow is a method to find the corresponding relationship between the previous frame and the current frame by analysing the change in pixels in the time domain of the image sequence and the correlation between adjacent frames, to calculate the motion of objects between adjacent frames. It is suitable for monitoring flock activity in the commercial farm. Using the optical flow patterns of chicken movements, infection by the human pathogen Campylobacter was detected in the early stages (Colles et al., Reference Colles, Cain, Nickson, Smith, Roberts, Maiden, Lunn and Dawkins2016). Optical flow data were collected, and the mean, variance, skew and kurtosis were calculated. Infected Campylobacter flocks had low mean optical flow and an apparent high kurtosis, and infection was detected 7 to 10 days earlier compared with faecal sample testing. This system has the potential for early health and welfare warnings that can benefit farmers and chickens. Using the camera-based optical flow method, early-stage footpad dermatitis and hock burn were predicted in 50 flocks from a commercial farm (Dawkins et al., Reference Dawkins, Roberts, Cain, Nickson and Donnelly2017). Optical flow analysed the movements of flocks, and lower skew and higher kurtosis values indicated heterogeneity in flock movement, which had predictive power for footpad dermatitis and hock burn.
In commercial farms, the activity and sudden variation of flock could be related to abnormal conditions, such as the equipment failure and diseases. Converting images into animal distribution index, the equipment failure in a broiler house could be found out in real time by the sudden changes of the distribution index (Kashiha et al., Reference Kashiha, Bahr, Vranken, Hong and Berckmans2014). With a uniform moderate walking pace through the flock, four variables were derived from the changes in activity before and after walking, so that the gait score could be predicted automatically (Silvera et al., Reference Silvera, Knowles, Butterworth, Berckmans, Vranken and Blokhuis2017). The occupation index was used instead of the distribution index, and the activity and occupation of the flock had correlation with the welfare scores for the footpad lesions and hock burns by human experts (Fernandez et al., Reference Fernandez, Norton, Tullo, van Hertem, Youssef, Exadaktylos, Vranken, Guarino and Berckmans2018). Moreover, the precise fault location could be performed by these indices in specific areas such as feeding, drinking and resting.
By combining a computer vision system with a novel convolutional neural network (CNN) method, the flock behaviour in a commercial farm was monitored automatically with a Kinect camera (Pu et al., Reference Pu, Lian and Fan2018). Three categories around feeders, not crowded, slightly crowded and fairly crowded, were labelled manually among 17 135 colour images and 17 135 depth images, and a supervised learning CNN was trained. The experimental results show that our proposed CNN-based method can recognise the flock behaviour of chickens with an accuracy of 99.17%.
Behaviours of individuals
Gait score of an individual hen can be done automatically. A digital video camera was mounted above the centre of a testing corridor to record images. The image processing method could classify the number of lying events (NOL) and latency to lie (LTL) of broilers (Aydin, Reference Aydin2015, Reference Aydin2017a and Reference Aydin2017b). The background subtraction method was applied to segment the shape, and then an elliptical shape model was used to define the size and orientation of the chicken (Aydin, Reference Aydin2015). Compared with the gait scores calculated manually from 250 broilers, the automatic monitoring system had 83% accuracy of identifying NOL. Subsequently, the image processing algorithm was improved by extracting feature variables (Aydin, Reference Aydin2017a). Automatically collected feature variables of broilers showed a clear correlation between feature variables and gait scores and were found to have a high level of accuracy starting from gait score three. Furthermore, instead of the 2D camera, a Kinect camera was used in the same experiment (Aydin, Reference Aydin2017b). Additional distance information between the broiler and the 3D camera was collected as broilers walked along a test corridor, and data were extracted to detect NOL and assess LTL with the back posture. The accuracy of NOL classification reached 93% compared to manual scoring.
In addition to RFID, image processing is another technology that has been considered to detect nest use. It can detect double nest occupation (Zaninelli et al., Reference Zaninelli, Costa, Tangorra, Rossi, Agazzi and Savoini2015 and Reference Zaninelli, Redaelli, Luzi, Mitchell, Bontempo, Cattaneo, Dell′Orto and Savoini2018), while RFID cannot. Photographs taken in a nest that was painted black, with the number of colour pixels counted and compared with the threshold double nest occupation, could detect whether there were two or more hens in a nest (Zaninelli et al., Reference Zaninelli, Costa, Tangorra, Rossi, Agazzi and Savoini2015). Later, with a thermographic camera to identify laying hens in a closed room (Zaninelli et al., Reference Zaninelli, Redaelli, Tirloni, Bernardi, Dell’Orto and Savoini2016 and Reference Zaninelli, Redaelli, Luzi, Bontempo, Dell’Orto and Savoini2017), this team developed a better ‘nest usage sensor’, which could count the number of hens in the nest (Zaninelli et al., Reference Zaninelli, Redaelli, Luzi, Mitchell, Bontempo, Cattaneo, Dell′Orto and Savoini2018). The thermographic images were processed by binarisation and coloured, and the number of colour pixels was counted to detect whether there were more than one hen in the nest. If there had a multiple nest occupation, pattern recognition was performed to make sure how many hens were in the nest.
The image processing algorithm could calculate the number of hens using an IR camera in an automatic lighting preference test system (Li et al., Reference Li, Li, Shi, Zhao and Ma2018). Original colour images were first cropped, converted into grey scale and then converted into binary images. The number of hens could be concluded by the number of white pixels. The accuracy of the image algorithm was 71.23%, due to the number of pixels changing significantly for one hen when feeding, wing flapping and preening.
The area required by each hen for the behaviours of standing, lying, perching, wing flapping and dust bathing was studied using ImageJ software and MATLAB (Riddle et al., Reference Riddle, Ali, Campbell and Siegford2018). However, it required manual selection of perfect images. Diseased broilers were predicted and classified automatically by the SVM model (Zhuang et al., Reference Zhuang, Bi, Guo, Wu and Zhang2018). To obtain the posture features, images were segmented by K-means clustering, and the skeleton structure was obtained by the thinning method. Superior to other machine learning (ML) algorithms, the SVM model obtained an accuracy rate of 99.47%.
Weight prediction
An automatic weight prediction system based on a 3D camera was developed and assessed on a commercial farm (Mortensen et al., Reference Mortensen, Lisouski and Ahrendt2016). The algorithm consisted of a range-based watershed algorithm and was predicted by a Bayesian artificial neural network. The system was able to weigh several chickens simultaneously. Due to the overlapping of broilers in the image, better prediction could be improved by a better segmentation method. Moreover, to estimate the weight of live broiler chickens, a machine vision method with an artificial neural network (ANN) was proposed (Amraei et al., Reference Amraei, Abdanan Mehdizadeh and Salari2017). Compared to Levenberg–Marquardt, scaled conjugate gradient and gradient descent, Bayesian regulation was the best network for the prediction of broiler weight. The maximum error of the system was less than 50 g. The aforementioned systems weighed individual broilers.
Discussion
Over the last few years, the prominent emergence of sensor technology, AI and big data analysis has made it possible to implement PLF. In this review, there are a total of 50 papers using automated techniques to detect poultry welfare. Of these, 14 papers including 3 of sound analysis, 4 of RFID and 7 of image processing were tested on a commercial level (even if just experimentally in a commercial flock). These techniques all have the ability to monitor the behaviour of birds under commercial conditions. Figure 1 shows the number of papers classified by detection contents and sensors.
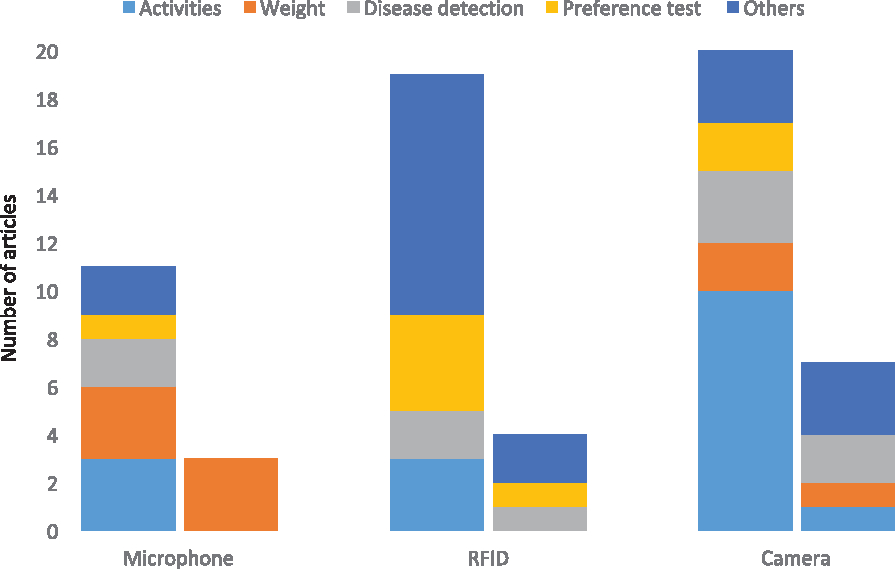
Figure 1 The articles, which monitored behaviour and welfare of broilers and laying hens automatically, are classified by detection contents and sensors. The height of the bars denotes the number of articles. Each sensor, including microphone, RFID and camera, has two bars. The left bar shows all the articles used in the sensor which are divided into five categories. The right bar shows the commercially tested articles which also have five categories. Activities refer to basic behaviours, such as feeding, drinking, perching, walking ability (including gait score) and laying behaviours (multiple nest occupation). Preference test includes environment preference, lighting preference, nest choice and free-range use. Others mean not belonging to the other four categories (e.g., equipment failure, welfare assessment). RFID, radio-frequency identification.
The use of sound and image processing technologies offers a comparatively non-invasive way to monitor the health and behaviour of animals over the long term. Due to the increasing size in commercial production systems for broilers and laying hens, it is common to observe and assess welfare at the group level. At the group level, sound analysis technology can be used to detect weight and stress of chickens on a commercial farm (Lee et al., Reference Lee, Noh, Jang, Park, Chung and Chang2015; Fontana et al., Reference Fontana, Tullo, Carpentier, Berckmans, Butterworth, Vranken, Norton, Berckmans and Guarino2017). Other detections based on sound analysis are at the experimental stage with a small number of birds, but they have a great potential to be applied in commercial chicken houses. Due to the environmental noise and simultaneous vocalisations, the accuracy of algorithms may be affected in complex environments. Using image processing, the activity of chicken flocks can be monitored on commercial farms and in real time (Colles et al., Reference Colles, Cain, Nickson, Smith, Roberts, Maiden, Lunn and Dawkins2016; Dawkins et al., Reference Dawkins, Roberts, Cain, Nickson and Donnelly2017). Mainly defined as the pixels change in the whole image, the activity means the flock is active in general but the specific behaviours of the flock are not classified. Moreover, some cameras have blind spots that cannot cover the entire chicken house. Another problem is that cameras, except IR cameras, cannot monitor the activity of chickens when lights are off.
However, PLF systems should be based on individual welfare assessments, because the average group welfare level may not accurately predict specific individual welfare. Monitoring individual behaviour, especially monitoring multiple individuals in a large group, requires the recognition and tracking of animals. Radio-frequency identification technology has been utilised in PLF systems to identify, track and register animals to detect behaviour and welfare in a reliable, automated and inexpensive way. While the RFID system can collect a large amount of data, it estimates behaviour by indirectly tracking the position of individuals. Additionally, the accuracy and tracking of this system depends on the number of antennas installed and the number of chickens wearing transponders. Because of the huge number of birds on commercial farms, it is currently unrealistic for each bird to wear an RFID tag. Typically, a random sample of birds is selected to wear a tag. Although RFID systems can be applied to hundreds of chickens, fitting and recycling tags is a time-consuming and laborious problem. Image processing technology can be used for target detection and tracking. With image target tracking and identification algorithms, an individual bird was tracked and identified in a group of six birds (Wang et al., Reference Wang, Chen, Zhang and Meng2016). Due to the high breeding density on commercial farms, it is difficult to automatically identify and track individual birds in a long time based on image processing with currently technology. Occlusion is another consideration. By combining image processing with RFID, individual birds can be tracked and behaviour can be detected more accurately (Nakarmi et al., Reference Nakarmi, Tang and Xin2014). The combination of multiple methods is such a way to implement PLF.
Since the advent of cheaper sensors and advanced sensor technology used for the observation of animal behaviour, huge data sets have been collected. These data sets are large, complex and diverse; thus, it is difficult to analyse by classical statistical methods. Unlike classical mathematical statistics, ML is a branch of AI that can analyse complex model data sets with unknown relationships between measured variables. ML attempts to mine the hidden variables from large collections of historical data for future prediction or classification. Many ML methods, such as decision trees, SVMs (Banakar et al., Reference Banakar, Sadeghi and Shushtari2016; Zhuang et al., Reference Zhuang, Bi, Guo, Wu and Zhang2018) and ANNs (Pu et al., Reference Pu, Lian and Fan2018), have been used in welfare assessments. Therefore, ML can provide strong support in developing an early warning system for PLF systems. On the other hand, multi-sensor integration system has a trend made for PLF (Van Hertem et al., Reference Van Hertem, Rooijakkers, Berckmans, Fernandez, Norton, Berckmans and Vranken2017). It is a hard work to perform a meta-analysis of the collected data of control units and behaviour detect devices such as climate control units, feed distribution units, camera-based system and microphone-based system. For farmers, only systems which are simple, easy to understand and easy to operate are been willing to use, for example, a visualisation tool. The stability of the system and the accuracy of measurement and prediction are also other factors that affect the use of farmers.
Conclusion
The development of modern technology offers powerful tools to automatically detect poultry health and welfare. However, most applications have the limitations of only being used in experimental conditions, although they have the potential to be used in commercial systems. The assessment of animal welfare is being transformed from the group level to the individual level. Consequently, the full implementation of the PLF system remains a great challenge. Further research is required to improve the accuracy of data processing and modelling, as well as to improve device detection before commercial validation. Once available, PLF systems will create added value to improve animal welfare, health and efficiency for farmers.
Acknowledgements
The authors would like to thank American Journal Experts for editing the English language in this paper. This work was also supported by the Key Science and Technology Research and Development Program of Hebei Province (16236605D-2 (2018)). The authors also thank the anonymous reviewers whose critiques and comments greatly improved the manuscript.
Declaration of interest
The authors declare no conflicts of interest.
Ethics statement
Conducting this review involved no animal handling or procedures.
Software and data repository resources
No new software or original data resources were generated as part of this study.