Implications
Why comparable animals reared in similar conditions have such different abilities to transform feed into food (i.e. animal feed efficiency) is a current relevant question for the beef industry. This review suggests that feeding and digestive-related factors are associated to animal variation in feed efficiency mainly because they co-vary with dry matter (DM) intake and that metabolic energy-consuming processes could be the true determinants. Finally, as some mechanisms related to feed efficiency could interact with other important animal traits, the information analysed and discussed in this review could help to better define future multi-trait breeding programmes.
Introduction
Ruminants can transform human-inedible feed (e.g. grasses, forages and by-products rich in cellulose) into high-quality human-edible food (e.g. meat, milk). However, this unique advantage of ruminants entails a low conversion efficiency, especially in growing cattle, compared with other livestock species and is associated with N pollution and greenhouse gas emissions. Given the growing human demand for animal protein-food, the scarcity of natural resources and the need to preserve our environment, improving the conversion of feed resources into animal products (i.e. animal feed efficiency) is becoming a greater challenge. Huge progress has been made to understand dietary factors influencing mean animal feed efficiency (e.g. feed conversion efficiency of a whole group), but there exists a considerable amount of animal-to-animal variation (phenotypic and genotypic) around this mean which remains to be fully understood.
Animal feed efficiency is a complex and multi-trait phenotype. Two different metrics, likely reflecting different underlying mechanisms, are currently used as measures of feed efficiency in growing beef cattle. First, the ratio between the amount of feed consumed and the animal weight gain, termed feed conversion ratio (FCR), was traditionally commonly used by the beef industry. However, despite the fact that FCR is a useful index to evaluate management practices on production efficiency, today it is a questionable trait for genetic selection because of its strong negative correlation with BW gain and thus, its undesirable effect on mature body size (and consequently on feed costs) of the breeding herd. In addition, selection based on ratio traits, such as FCR, may result in divergent and unpredictable response in the component traits (Zetouni et al., Reference Zetouni, Henryon, Kargo and Lassen2017) leading, for instance, to inconsistent relationships (Arthur and Herd, Reference Arthur and Herd2012) with feed intake (trait in the numerator). The second metric is the difference between actual feed intake and expected feed requirements for maintenance and weight gain, the main energy sinks in growing animals, termed residual feed intake (RFI). Animals with lower RFI are deemed to be more efficient since they eat less than expected. Residual feed intake has gained popularity in recent years, mainly among geneticists, because of its lack of phenotypic correlation with BW gain and animal size. Being a measure of feed efficiency that is independent of an animal’s performance has led to the belief that variation in RFI may represent inherent variation in basic metabolic processes that determine production efficiency (Archer et al., Reference Archer, Richardson, Herd and Arthur1999). However, in general RFI has been poorly accepted by industry mainly because animals ranked as superior in terms of RFI may be growing slowly (Berry and Crowley, Reference Berry and Crowley2013).
The biological determinants of animal-to-animal variation in feed efficiency are still far from being fully understood. However, they need to be comprehended for several reasons (Richardson et al., Reference Richardson and Herd2004): (i) it may provide insights into the undesirable side effects of selection for feed efficiency on other important animal traits such as robustness, reproduction and health (Rauw et al., Reference Rauw, Kanis, Noordhuizen-Stassen and Grommers1998) and thus help us to better design balanced breeding programmes; (ii) it may reveal novel management strategies aiming to improve the performance of feed inefficient animals and better matching diet formulation to individual animal requirements; and (iii) it may result in the discovery of cheap and rapid methods (biomarkers) for ranking individuals for genetic and management purposes without the need for feed intake measurement. The study of biological mechanisms underlying the animal-to-animal variation in feed efficiency has intensified over the past 15 years. Most of these studies aimed to identify the determinants and molecular bases explaining why comparable animals reared in similar conditions differ in feed efficiency, with some studies targeting the discovery of biomarkers to predict this phenotype. Although some excellent reviews are published in relation to the biological basis of feed efficiency in beef cattle (Richardson and Herd, Reference Richardson, Herd, Archer and Arthur2004; Herd and Arthur, Reference Herd, Arthur, Donoghue, Bird, Bird-Gardiner and Hegarty2009) extensive new data have emerged especially regarding the molecular basis of feed efficiency. The aim of this paper is to review the recent scientific literature on the biological determinants and the molecular basis of feed efficiency, with particular reference to growing beef cattle phenotyped for RFI. Previous research indicates that no single mechanism is responsible for determining animal feed efficiency (Herd et al., Reference Herd and Arthur2004). Furthermore, many observed differences between animals divergent in feed efficiency may be a direct consequence of a difference in intake. Therefore, this review also attempts to identify which set of plausible mechanisms might be causing variation in animal feed efficiency directly as opposed to traits whose change is simply a consequence of lower intake.
Feeding behaviour and physical activity
Feed intake is a function of meal size and frequency and is regulated by a combination of physical and metabolic mechanisms, with the relative importance of these two processes dependent on prevailing dietary characteristics (Fitzsimons et al., Reference Fitzsimons, McGee, Keogh, Waters and Kenny2017). Feeding behaviour is determined by the integration of central and peripheral signals in brain feeding centres (Allen, Reference Allen2014).
Considering the substantial phenotypic differences observed in DM intake between efficient and inefficient beef cattle (Kelly et al., Reference Kelly, McGee, Crews, Fahey, Wylie and Kenny2010; Fitzsimons et al., Reference Fitzsimons, Kenny, Fahey and McGee2013 and Reference Fitzsimons, Kenny and McGee2014), feeding behaviour likely makes an important contribution to the underlying variation in feed efficiency of beef cattle (Kelly et al., Reference Kelly, McGee, Crews, Fahey, Wylie and Kenny2010; Fitzsimons et al., Reference Fitzsimons, McGee, Keogh, Waters and Kenny2017). Nevertheless, activity associated with consumption, particularly for forage diets, is a significant energy sink in cattle (Fitzsimons et al., Reference Fitzsimons, McGee, Keogh, Waters and Kenny2017). Key feeding behaviour activities include frequency and duration of individual feeding events – collectively, termed daily feeding duration (min or h) – and eating rate (g/min). To quantify the effect of RFI status on daily feeding duration, Kenny et al. (Reference Kenny, Fitzsimons, Waters and McGee2018) conducted a meta-analysis of nine published studies with growing beef cattle offered energy-dense high-concentrate diets and found that high-RFI cattle spent proportionately 0.12 more time eating than their low-RFI contemporaries. The 0.17 proportionately higher DM intake of the high-RFI animals in that analysis implies that they also had a faster eating rate than the low-RFI animals. Published research evaluating the association between RFI status and daily feeding events is equivocal, and may be partly due to the diversity of diet types offered (Kenny et al., Reference Kenny, Fitzsimons, Waters and McGee2018). Physical activity is a non-productive function contributing to energy use in cattle and is interconnected with feeding-related activity behaviour, especially under grazing conditions. There is evidence that the occurrence of non-feeding events, where cattle are at the feed face but do not consume any feed, is less in low- compared with high-RFI beef cattle (Kelly et al., Reference Kelly, McGee, Crews, Fahey, Wylie and Kenny2010). The comparatively few studies published evaluating non-feed related activities – time spent standing, lying or active – in beef cattle varying in RFI status (Lawrence et al., Reference Lawrence, Kenny, Earley and McGee2012), have shown inconsistent behavioural effects between divergent RFI phenotypes under confined and/or grazing conditions (Kenny et al., Reference Kenny, Fitzsimons, Waters and McGee2018).
Most of the aforementioned research involved cattle in confinement, usually offered energy-dense diets, where individual animal intake and associated behaviour was relatively easily determined using automated feeding systems coupled with electronic animal identification sensors. In contrast, there is comparatively little published information pertaining to feed efficiency in grazing beef cattle despite the fact that the majority of beef production systems worldwide are largely based on pasture (Lawrence et al., Reference Lawrence, Kenny, Earley and McGee2012; Manafiazar et al., Reference Manafiazar, Basarab, Baron, McKeown, Doce, Swift, Undi, Wittenberg and Ominskik2015; Oliveira et al., Reference Oliveira, Ruggieri, Branco, Cota, Canesin, Costa and Mercadante2016). This is not surprising considering how challenging it is to accurately obtain equivalent measurements with individual animals grazing pasture (Lawrence et al., Reference Lawrence, Kenny, Earley and McGee2012).
However, in this context, there is evidence of genotype-by-environment interactions for RFI in growing beef cattle (Kenny et al., Reference Kenny, Fitzsimons, Waters and McGee2018). For example, beef heifers previously ranked as divergent for RFI when offered grass silage plus supplementary concentrates whilst indoors did not differ in herbage intake when subsequently grazing pasture (Lawrence et al., Reference Lawrence, Kenny, Earley and McGee2012). Similar findings were obtained by Oliveira et al. (Reference Oliveira, Ruggieri, Branco, Cota, Canesin, Costa and Mercadante2016). In contrast, Manafiazar et al. (Reference Manafiazar, Basarab, Baron, McKeown, Doce, Swift, Undi, Wittenberg and Ominskik2015) reported that RFI measured under drylot conditions was positively correlated (r=0.30) to RFI measured under grazing conditions. Discrepancies between grazing-based studies may be due to the very low precision of methods used to estimate pasture intake (Lawrence et al., Reference Lawrence, Kenny, Earley and McGee2012). To overcome this particular limitation and facilitate more accurate collection of grass herbage intake data, recent studies have used a zero-grazing regime instead (Coyle et al., Reference Coyle, Fitzsimons, Kenny, Kelly and McGee2016 and Reference Coyle, Fitzsimons, Kenny, Kelly and McGee2017). Research evaluating the within-animal repeatability of intake, growth and feed efficiency between two consecutive feeding periods in beef cattle offered grass silage followed by zero-grazed fresh grass (Coyle et al., Reference Coyle, Fitzsimons, Kenny, Kelly and McGee2016), or zero-grazed grass followed by a high-concentrate diet (Coyle et al., Reference Coyle, Fitzsimons, Kenny, Kelly and McGee2017), concluded that DM intake, and to a lesser extent RFI, were somewhat repeatable traits, whereas FCR and average daily live weight gain were not. This implies that there is a re-ranking in feed efficiency in beef cattle offered fresh grass herbage compared to other diets, especially for the measure FCR. With cattle offered grass herbage, ruminal distension is more likely to dominate control of feed intake rather than fuel-sensing by tissues when fuel supply is in excess of that required, such as occurs on high-energy concentrate-based diets (Allen, Reference Allen2014); thus, a strong relationship between ruminal fill and grazing dynamics is evident (Gregorini et al., Reference Gregorini, Gunter, Beck, Soder and Tamminga2008). Furthermore, under grazing conditions ingestive-digestive behaviours become significant due to variation in herbage nutrient supply and composition, and also differential energy expenditures associated with the harvesting and defoliation process (Gregorini et al., Reference Gregorini, Gunter, Beck, Soder and Tamminga2008). Genotype-by-environment interactions are particularly important if estimates of genetic merit for feed efficiency are derived under feeding and management systems that are different from commercial farm conditions.
Collectively, these results indicate that in confinement feeding, differences in feeding behaviour-related traits between low- and high-RFI cattle are largely a reflection of differences in intake; however, under grazing conditions the relative contribution of these traits to between-animal variation in feed efficiency is unknown. Much more research is required on feed efficiency of beef cattle grazing pasture, but precision in methodologies used to estimate grass herbage intake need to be improved upon in order to discern realistic differences in consumption between individual animals.
Digestibility and methane emission
Digestibility and methane emissions markedly limit the efficiency of capture of dietary energy in useful animal products. Since methane production (g CH4/day) is linearly related to DM intake, selection of cattle for low-RFI may substantially reduce methane production without reducing the growth of the animal (Basarab et al., Reference Basarab, Price, Aalhus, Okine, Snelling and Lyle2013). Lower methane production is not always seen in animals of lower RFI, however, because (1) divergent RFI cattle do not always exhibit differences in DM intake when digestibility is measured outside the RFI test period (Jones et al., Reference Jones, Phillips, Naylor and Mercer2011) and (2) low RFI cattle often exhibit up to a 4% points increase in whole tract DM digestibility, increasing the quantity of substrate available for fermentation and methanogenesis per unit feed (Bonilha et al., Reference Bonilha, Branco, Mercadante, Cyrillo, Monteiro and Ribeiro2017). Richardson and Herd (Reference Richardson, Herd, Archer and Arthur2004) concluded that the processes of digestion were responsible for at least 10% of variance in RFI. However, when available literature studies reporting concurrent feed intake and digestibility data in divergent RFI cattle were evaluated through meta-analysis, a negative correlation between DM digestibility and DM intake was found on average (Figure 1): the slope representing the assumed loss in digestibility as the DM intake increases (Sauvant and Nozière, Reference Sauvant and Nozière2016). This result suggests that overall higher DM digestibility in low RFI cattle might be mostly the consequence of lower DM intake and likely not the opposite.

Figure 1 Relationship between dry matter (DM) digestibility and DM intake in extreme residual feed intake (RFI) cattle from published data. Empty symbols refers to negative RFI animals, whereas full ones refer to positive RFI animals within the same study. A star beside the number means that significant differences (P<0.10) in digestibility between divergent RFI animals were originally reported in that particular study. Overall, a significant negative relationship was found between DM digestibility and DM intake once corrected for the effect of the study (dashed thick line: DM digestibility=76.0±1.79−0.72±0.15×DM intake; RSE=1.13; r 2=0.97). Four out of 17 studies did not show negative relationship between DM digestibility and DM intake across divergent RFI animals (square symbols). References are listed at the end of the Supplementary Materials.
Ideally, it is not just a lower methane production resulting from selection of low RFI cattle that should be targeted to increase efficiency of energy capture by the animal, but also a reduced methane yield (MY; g CH4/kg DM intake). Beef cattle divergent for RFI have not shown consistent differences in MY, and there is no obvious physiological reason why low RFI animals even if exhibiting reduced methane production, should also have a reduced MY. On the contrary, reducing an animal’s level of intake (relative to maintenance) often increases MY, as recently observed in extreme RFI beef cattle (Herd et al., Reference Herd, Velazco, Arthur and Hegarty2016). While reducing feeding level generally increases mean retention time of digesta in the rumen (Sauvant and Nozière, Reference Sauvant and Nozière2016), the limited data available for animals divergent in RFI fails to show longer mean retention time in animals of lower RFI, perhaps because of smaller rumens that are also associated with low RFI animals (Fitzsimons et al., Reference Fitzsimons, Kenny and McGee2014). Smaller rumen liquid volumes and a shorter mean retention time have been found in low RFI dairy cows (Rius et al., Reference Rius, Kittelmann, Macdonald, Waghorn, Janssen and Sikkema2012) and in low MY sheep (Goopy et al., Reference Goopy, Donaldson, Hegarty, Vercoe, Haynes, Barnett and Oddy2014). Differential responses in rumen volume and digesta retention time with divergence in RFI may account for some of the variability in effects of RFI on MY.
There is, however, evidence of genetically controlled between-animal variation in MY, giving scope for selection for reduced MY to improve efficiency of capture of energy consumed by the animal. Within a herd, there are cattle which display both low RFI and low MY (Figure 2). Selection pressure could be applied to MY in addition to selection for RFI, to provide downward pressure on both DM intake and MY. There remains the challenge of measuring methane production on a commercial scale and more data are required to confirm genetic relationships. Genetic variation in MY among cattle has recently been quantified (Donoghue et al., Reference Donoghue, Bird-Gardiner, Arthur, Herd and Hegarty2016) and, like RFI, can be selected against without impacting animal productivity, as no adverse genetic correlations with production traits are apparent. Interestingly, when comparisons were conducted in steers divergent in residual gain (gain adjusted to intake and BW), to overcome the problem associated with the inherent correlation between methane production and DM intake, no differences in daily methane production were noted (Freetly et al., Reference Freetly, Lindholm-Perry, Hales, Brown-Brandl, Kim, Myer and Wells2015). This finding may indicate that methane emissions are not always a contributor to animal-to-animal variation in feed efficiency.

Figure 2 Distribution of methane yield (g CH4/kg dry matter intake (DMI)) and residual feed intake (RFI) among 41 Angus cattle on a feedlot ration with methane measured by Greenfeed Emission Monitors. Data show some cattle exhibit both RFI and methane yield that are below the group means (displayed as solid lines). Data are raw values from Herd et al. (Reference Herd, Velazco, Arthur and Hegarty2016).
One objection to selecting on MY, like selecting for FCR, is the unpredictable consequences of selecting on a ratio trait (Zetouni et al., Reference Zetouni, Henryon, Kargo and Lassen2017), where response could be due to changes in the numerator, denominator or both. For this reason, the approach to selection has moved to residual methane (Herd et al., Reference Herd, Oddy and Richardson2014), with residual methane (methane production of the individual compared with its predicted emission) being strongly correlated with MY (r=0.82 to 0.95) but independent of DM intake and only weakly correlated with production traits.
In summary, the above considerations identify between-animal variation that exists in a number of genetically controlled traits and can be selected to reduce emission of the enteric greenhouse gas, methane. Effects of selection for RFI on MY are less certain but selection for residual methane (h 2=0.19; Donoghue et al., Reference Donoghue, Bird-Gardiner, Arthur, Herd and Hegarty2015) as a complementary trait to RFI offers a new means of reducing MY. Together, RFI and residual methane offer a significant opportunity to reduce the emission intensity (methane produced per unit of beef) whether considered on an individual animal or a whole enterprise basis. Despite a paucity of data, a trade-off between digestion and methane emissions might exist when improving animal feed efficiency, meaning that the positive impact for the animal of increasing digestibility could entail a higher MY and vice versa.
Rumen microbiota
The relationship between rumen digestion and feed efficiency has been investigated and there is emerging evidence of individualized variation in microbiome within a cattle population when animals are fed the same diet and raised in the same environment (Jami and Mizrahi, Reference Jami and Mizrahi2012). Guan et al. (Reference Guan, Nkrumah, Basarab and Moore2008) first proposed the linkage between the rumen microbiota (and its metabolites) and animal-to-animal variation in feed efficiency. Since then many efforts have been made worldwide further supporting that, although minor, there exist changes in the rumen microbiome associated with animal-to-animal variation in feed efficiency (Supplementary Table S1). For example, in beef steers, bacterial phylotypes (Succinivibrio sp., Eubacterium sp., and Robinsoniella sp.) were identified to be potentially associated with RFI (study#5; Supplementary Table S1), however, such a relationship is diet dependent. Succinivibrio bacteria, higher in high efficient animals (Study#4 (low FCR) and #5 (low RFI under a low-energy diet)), have been suggested to utilize hydrogen for the production of succinate, therefore lowering its availability for methanogenesis (Pope et al., Reference Pope, Smith, Denman, Tringe, Barry, Hugenholtz, McSweeney, McHardy and Morrison2011). Similarly, studies# 2, 3 and 6 (Supplementary Table S1) reported a less diverse methanogen community in efficient cattle (low RFI) with lower abundance of specific methanogenic species in less efficient animals (high RFI). For example, lower abundance of Methanobrevibacter sp. strain AbM4 and Methanosphaera stadtmanae (which synthesize methane through a non-CO2-based pathway) were found in the rumen of efficient animals (Study#2; Supplementary Table S1). These methanogens are known to consume substrates such as acetate, and limit their availability to the host. Recent metagenomics and metatranscriptomics based in-depth assessment of the rumen microbiome in beef (Study#14) and dairy (Study#11) cattle fed high-energy concentrate diets also suggest that the rumen microbiome is less genetically diverse and active in efficient cattle than inefficient ones. Both studies indicated that microbial nitrogen metabolism rather than carbohydrate metabolism is more related to variations in cattle feed efficiency (RFI and FCR). Although these findings contradict the prevailing dogma that greater diversity is always better, they shed light on the rumen microbiome and how their functional potential (genes) and activities (messenger RNAs (mRNAs)) can contribute to host feed efficiency. The less diverse microbial activity in efficient animals may reflect a simpler rumen microbiome, which uses less substrate and energy for microbial proliferation and directs more nutrients to the host. This suggests that intervention strategies to inhibit the ‘unnecessary rumen microbial activity’ (the enriched functions in inefficient animals) may lead to the improvement of feed efficiency. However, more studies are warranted to confirm that lowering microbial diversity does not impact resilience and health status.
From studies reported in Supplementary Table S1, it is evident that the overall microbial profiles do not differ across divergent feed efficiency phenotypes: all individuals under the same feeding condition have a ‘core’ bacterial structure and most dominant bacteria are not relevant for this trait (Hernandez-Sanabria et al., Reference Hernandez-Sanabria, Goonewardene, Li, Mujibi, Stothard, Moore and Leon-Quintero2010). However, the association of specific microbial phylotypes (bacteria and archaea) to RFI (Supplementary Table S1) may support the concept of a specific and individualized microbiome contributing to the animal variation in feed efficiency. This raises the question about what may drive such individualization and how such knowledge could be applied to the beef industry. A recent survey of the rumen microbiota of 732 beef cattle with genotypes by 50K bovine single nucleotide polymorphism panels (Li et al., Reference Liunpublished data) by University of Alberta team, further showcased that ~30% of the rumen microbial taxa are potentially heritable (h 2≈0.15), especially the members belonging to Firmicutes phylum, which play very important roles in fibre digestion and carbohydrate metabolism. In contrast, the taxa belonging to Bacteroidetes had a low heritability, in accordance with abundance shift when diet changes. Moreover, the same research group identified 19 single nucleotide polymorphisms located on 12 bovine chromosomes and associated with 14 rumen microbial taxa, and some of these rumen microbiota-associated single nucleotide polymorphisms were known as quantitative trait loci for feed efficiency in cattle (Li, Reference Li2017). These findings suggest that it may be possible to identify host genetic components that influence the rumen microbiome which can be used for future breeding strategies to improve rumen function and feed efficiency. Some speculations are that some traits differing across RFI groups, such as the size of the rumen (Fitzsimons et al., Reference Fitzsimons, Kenny and McGee2014; Meale et al., Reference Meale, Morgavi, Cassar-Malek, Andueza, Ortigues-Marty, Robins, Schiphorst, Laverroux, Graulet, Boudra and Cantalapiedra-Hijar2017), and/or ruminal tissue development and absorption capacity (Kong et al., Reference Kong, Guanxiang, Chen, Stothard and Guan2016) might be genetically regulated, which could directly or indirectly affect the ‘individualized’ rumen microbial population.
In summary, most studies converge to show that, although minor, there are significant differences in the rumen microbiome associated with animal variation in feed efficiency. It is not clear, however, whether the differences observed have causal effects on animal-to-animal variation in feed efficiency, or are rather the consequence of shifts in total intake, digesta biomass and rumen retention time, which may co-vary with changes in feed efficiency. To determine whether the rumen microbiome really contributes to animal-to-animal variation of feed efficiency or only co-varies with it, future efforts on the analysis of the rumen microbiome should include a higher number of animals and higher sampling frequency and representativeness with complementary measures such as physiological measures (rumen volume, passage rate, rumen pH, etc.), eating behaviour information and host genetic information. At present, our understanding of the impact of host physiology and genetics on the rumen microbiome is still a large ‘black box’.
Energy metabolism
Whole body level
Since the last comprehensive reviews by Richardson and Herd (Reference Richardson, Herd, Archer and Arthur2004) and Herd and Arthur (Reference Herd, Arthur, Donoghue, Bird, Bird-Gardiner and Hegarty2009), which suggested reduced maintenance energy requirements for low RFI lines, there is still a scarcity of data on energy expenditure and its underlying components of divergent RFI lines. In beef cattle, evidence is particularly limited as only two studies evaluated heat production from oxygen consumption measurements (Nkrumah et al., Reference Nkrumah, Okine, Mathison, Schmid, Li, Basarab, Price, Wang and Moore2006; Chaves et al., Reference Chaves, Nascimento, Tullio, Rosa, Alencar and Lanna2015). Interpretation of experimental results is generally hampered by the fact that major potential underlying mechanisms (e.g. digestibility) are often not simultaneously measured. Moreover, the statistical power of the experimental set up is often limited as regards to the small expected differences in heat production between animals identified as divergent for RFI in performance tests and the low precision of the methods. Despite those limits, evidence is emerging that animals showing higher feed efficiency (low RFI) are not only characterized by lower maintenance energy requirements, but also by higher partial efficiency of metabolizable energy (ME) utilization for growth leading to generally higher metabolic energetic efficiency. Only the most illustrative studies are reported here.
Lower maintenance requirements for ME have been demonstrated in pigs in an elegant study combining measurements of respiratory exchanges and a modelling approach to quantify the components of heat production (fasting, activity, short- and long-term thermic effect of feeding, Barea et al., Reference Barea, Dubois, Gilbert, Sellier, Van Milgen and Noblet2010). A 10% reduction in maintenance requirements was determined in low RFI piglets even after adjustment for differences in energy retention. These results in pigs support, despite species differences, calculations in beef cattle (e.g. Castro Bulle et al., Reference Castro Bulle, Paulino, Sanches and Sainz2007; even if differences were not significant because of low statistical power), including those obtained in heifers by regression for zero BW change (Lawrence et al., Reference Lawrence, Kenny, Earley and McGee2012; Fitzsimons et al., Reference Fitzsimons, Kenny, Fahey and McGee2013), of reduced maintenance ME requirements.
Most novel is the emerging evidence for a higher partial efficiency of ME use for growth. Nkrumah et al. (Reference Nkrumah, Okine, Mathison, Schmid, Li, Basarab, Price, Wang and Moore2006) rigorously measured heat production in phenotypically divergent RFI steers, each fed at two feeding levels above maintenance. Adaptation periods were unequal and sometimes short, but results indicated a considerable numerical, although not statistically significant, reduction in the increment of heat production between two feedings levels in low v. high RFI steers, and a significant negative correlation between heat production and partial efficiency of growth. Altogether, these results suggest a higher partial efficiency of ME use for growth in low RFI steers. This increased metabolic efficiency of ME use for growth measured at similar intakes highlights that the latter may be a true determinant of feed efficiency and not a passive result of lower intake. This effect associated with a small (5%) although significantly higher diet digestibility and a marked reduction (−24% to −28%) in methane emission, explained the improved energy retention when low-RFI steers were fed similar DM intake as high-RFI (Nkrumah et al., Reference Nkrumah, Okine, Mathison, Schmid, Li, Basarab, Price, Wang and Moore2006), and suggested changes in the composition of the BW gain. Variation in the partial efficiency of ME use for growth has been a matter of debate (e.g. Castro Bulle et al., Reference Castro Bulle, Paulino, Sanches and Sainz2007). A small meta-analysis conducted here (Supplementary Figure S1), which combined ME intake and retained energy calculated from published results in high- v. low-RFI steers (excluding Nkrumah et al., Reference Nkrumah, Okine, Mathison, Schmid, Li, Basarab, Price, Wang and Moore2006, because of controlled intakes during oxygen consumption measurements), indicated an average marginal efficiency of ME utilization for growth (Δ retained energy/ Δ ME intake) of 0.33±0.10, close to the value of 0.25 calculated from Basarab et al. (Reference Basarab, Beauchemin, Baron, Ominski, Guan, Miller and Crowley2003). This slope differs from the average theoretical kg value of 0.53 expected for animals, such as those in the current data set, which deposit 7% to 16% energy as protein (Geay, Reference Geay1984). It implies that kg may vary between RFI divergent animals, with a higher partial efficiency of ME use for growth in low-RFI steers, whether maintenance ME requirements are assumed to be unchanged or reduced.
Whether the two mechanisms of low maintenance ME requirements and improved partial efficiency of ME use for growth are combined or not remains to be determined. Indirect evidence for combined changes was provided by Basarab et al. (Reference Basarab, Beauchemin, Baron, Ominski, Guan, Miller and Crowley2003) after adjusting RFI for differences in composition of body gain. However, the dichotomy between reduced maintenance ME requirements and increased partial efficiency for ME use for growth is largely the result of mathematical conventions, experimental conditions and limited numbers of simultaneous measurements, and does not help in identifying the underlying biological mechanisms. In any case, more efficient (low-RFI) animals do appear to have a lower metabolic rate (i.e. heat production expressed by unit weight and unit time, at similar ME intake) than the feed inefficient (low-RFI) animals which can arise from reduction in maintenance or efficiency of ME use for growth, or both, and that needs to be confirmed in future studies.
The lower metabolic rate of more efficient lines (low RFI) may have multiple origins as each metabolic pathway will impact ATP use and production. At the tissue level, protein mass is important because of the high energy cost of protein metabolism. However, low-RFI steers often have leaner carcasses, so there may be a number of mechanisms that contribute to reducing the energy cost of protein-rich tissues. Reduced fractional protein degradation rate was associated with lower ME requirements for maintenance in steers (Castro Bulle et al., Reference Castro Bulle, Paulino, Sanches and Sainz2007). In addition, low-RFI animals showed changes in the distribution of muscle fibre types (hypertrophy of fast-twitch glycolytic fibres (Lefaucheur et al., Reference Lefaucheur, Lebret, Ecolan, Louveau, Damon, Prunier, Billon, Sellier and Gilbert2011) and generally had lower oxidative and glycolytic activities in muscles (Gilbert et al., Reference Gilbert, Bidanel, Gruand, Caritez, Billon, Guillouet, Lagant, Noblet and Sellier2007)). These are likely to result in a lower average metabolic rate of muscles. Modifications in the distribution of lean mass can also be involved. Gut and liver contribute 38% to total energy expenditure in growing cattle, which, if attributed to maintenance, represents on average 60% of theoretical maintenance ME requirements (Ortigues-Marty et al., Reference Ortigues-Marty, Cantalapiedra-Hijar, Vernet and Nozière2017). This is supported by a positive genetic correlation between the empty digestive tract weight and RFI (Renand and Krauss, Reference Renand and Krauss2002), but other studies failed to show differences in the mass of internal organs between RFI divergent animals as discussed further on in this review.
Cellular level
Between-animal variation in total energy expenditures by animals of similar biotype and management background may arise from a host of cellular energy-consuming processes, including ion pumping (e.g. Na+/K+ATPase), mitochondrial proton leakage, thyroid hormones, leptin, IGF-1, protein turnover, lipid/protein enzymes or sympathetic activity (Johnson et al., Reference Johnson, Ferrell and Jenkins2003). Of these physiological processes, it has been estimated that mitochondrial proton leakage, ion pumping, and protein turnover each contribute ≈20% to total between-animal variation in basal energy expenditures (Rolfe and Brown, Reference Rolfe and Brown1997). Mitochondria are responsible for producing over 90% of cellular ATP from acetyl CoA, which is generated upon digestion and catabolism of carbohydrates, protein and lipid derived from the diet and(or) body reserves. Through oxidative phosphorylation (OXP) involving a series of electron transfers through the electron transport chain (ETC), cellular respiration is coupled with the pumping of protons into the intermembrane space, which drives the synthesis of ATP as protons move back into the matrix via ATP synthase. In brown fat, this protomotive force is dissipated due to the presence of an uncoupling protein (UCP1), thereby generating heat rather than ATP. Inefficiency of OXP can also occur due to electron leakage across the ETC, which then generates reactive oxygen species (ROS; superoxide, H2O2) to cause oxidative stress in the absence of sufficient access to antioxidants (e.g. superoxide dismutase).
Bottje et al. (Reference Bottje, Tang, Iqbal, Cawthon, Okimoto, Wing and Cooper2002) was the first to demonstrate that differences in mitochondrial function were linked to between-animal variation in feed efficiency. In a series of studies, Bottje et al. (Reference Bottje, Tang, Iqbal, Cawthon, Okimoto, Wing and Cooper2002) found that mitochondria (isolated from multiple tissues) from broilers with efficient (low FCR) phenotypes exhibited a more tightly coupled respiratory chain that was associated with less electron leak and ROS production compared to mitochondria from inefficient (high FCR) broilers. Furthermore, the increase in ROS production of mitochondria from inefficient broilers was associated with lower activities of ETC complex proteins (I to IV) (Bottje and Carstens, Reference Bottje and Carstens2009). A greater degree of ETC coupling was also found in mitochondria isolated from muscle (Kolath et al., Reference Kolath, Kerley, Golden and Keisler2006) and liver (Lancaster et al., Reference Lancaster, Carstens, Michal, Brennan, Johnson and Davis2014) from beef cattle with low- than high-RFI phenotypes. Moreover, Sharifabadi et al. (Reference Sharifabadi, Zamiri, Rowghani and Bottje2012) reported that activities of ETC complex proteins were substantially higher in low- v. high-RFI lambs. These results indicate that mitochondria from feed-efficient animals have enhanced ETC coupling and generate ATP more efficiently from a given amount of substrate.
In support of these findings, Kong et al. (Reference Kong, Guanxiang, Chen, Stothard and Guan2016) found that genes coding for several mitochondrial proteins involved in OXP were upregulated in rumen epithelium of low- v. high-RFI steers. Of notable interest, Kong et al. (Reference Kong, Guanxiang, Chen, Stothard and Guan2016) also reported that relative mitochondrial genome copy number per cell was 26% lower in rumen epithelium of low- compared with high-RFI steers. This would indicate that feed-efficient steers had a greater rate of transcription of genes associated with OXP from a lesser mitochondrial genome in rumen epithelial tissue. Kelly et al. (Reference Kelly, Waters, McGee, Fonseca, Carberry and Kenny2011) examined the expression of genes associated with ETC proteins in muscle of steers with divergent RFI phenotypes, while fed both high-grain and high-forage diets. Differences in expression of complexes I, II and III were not detected between low- and high-RFI steers. However, a greater abundance of cytochrome c oxidase (complex IV) mRNA transcript was detected in low-RFI steers, but only when fed the high-grain diet. Kelly et al. (Reference Kelly, Waters, McGee, Fonseca, Carberry and Kenny2011) also reported that UCP3 mRNA levels were 2.2-fold higher in muscle of high-RFI steers, which is consistent with the finding that inefficient broilers had higher mRNA for avian UCP (equivalent to UCP3) compared with feed-efficient broilers (Ojano-Dirain et al., Reference Ojano-Dirain, Toyomizu, Wing, Cooper and Bottje2007). UCP3 in muscle acts to protect cells from oxidative damage by inducing mild uncoupling of OXP to reduced mitochondrial superoxide production. Thus, increased expression of UCP3 mRNA likely serves to attenuate ROS-mediated oxidative damage generated in mitochondria of less-efficient animals. In pigs divergently selected for RFI, several antioxidant genes/proteins (e.g. superoxide dismutase) were overexpressed in muscle of high-RFI pigs (Vincent et al., Reference Vincent, Louveau, Gondret, Tréfeu, Gilbert and Lefaucheur2015), suggesting that several adaptive mechanisms may have evolved to cope with higher rates of ROS production in mitochondria from less feed-efficient animals.
Kelly et al. (Reference Kelly, Waters, McGee, Fonseca, Carberry and Kenny2011) found that mRNA transcripts for the coactivator for peroxisome proliferator activated receptor (PGC-1) were 1.7-fold higher in low- compared with high-RFI steers. Increased expression of PGC-1 has been associated with increased mitochondrial biogenesis, enhanced mitochondrial respiration and the induction of several ROS-detoxifying enzymes. The fact that mitochondrial biogenesis may be elevated in low-RFI phenotypes by increased gene expression of PGC-1 appears to run counter to the reduction in mitochondrial content observed in rumen epithelium from low-RFI steers (Kong et al., Reference Kong, Guanxiang, Chen, Stothard and Guan2016). Moreover, utilizing both transcriptomic and proteomic approaches, Vincent et al. (Reference Vincent, Louveau, Gondret, Tréfeu, Gilbert and Lefaucheur2015) presented compelling evidence to show that genes/proteins associated with mitochondrial oxidative metabolism were downregulated, and that mitochondrial content was reduced in muscle of pigs selected for low RFI. Thus, selection for low RFI in pigs appears to have altered both mitochondrial biogenesis as well as intrinsic mitochondrial composition to affect the efficient mitochondrial phenotype. Interestingly, when high-RFI pigs were pair fed to match the intake of low-RFI pigs, differences due to divergent RFI selection were still evident, indicating that the molecular mechanisms associated with variation in mitochondrial energy metabolism were largely independent of differences in voluntary feed intake. The apparent discrepancies noted between some of the abovementioned studies no doubt reflect differences in species, tissue and methodologies, as well as the feed-efficiency phenotype (e.g. FCR v. RFI) used in experimental designs. Continued research that seeks to discover mechanisms controlling mitochondrial function and biogenesis will undoubtedly lead to better understanding of the cellular basis for between-animal variation in efficiency of feed utilization, as well as to the discovery of biomarkers to more accurately identify feed-efficient livestock.
Protein turnover
Protein turnover can be defined as the continuous process of degradation and synthesis of proteins leading to no net changes in mass but to a renewal. Thus, for growing animals, where protein accretion occurs because protein synthesis is greater than degradation, the protein turnover is equal to total protein degradation or alternatively an equal amount of freshly synthesized protein that replaces degraded protein. This means that in growing animals the protein turnover can be indirectly assessed through the intensity of protein degradation. The protein turnover in growing-fattening young bulls is huge compared with the net protein deposition with as high as 94% of the whole body protein synthesis only serving to counterbalance the protein degradation (Lobley et al., Reference Lobley, Sinclair, Grant, Miller, Mantle, Calder, Warkup and Maltin2000). This huge turnover is accompanied by a considerable individual genetic variation (Hawkins, Reference Hawkins1991; Oddy et al., Reference Oddy, Spec, Warren and Wynn1995).
Protein turnover is essential for life, providing the amino acid flux that enable maintenance services such as metabolic regulation, cellular repair and rapid adaptation against environmental changes, among other functions. However, protein turnover represents an apparently futile cycle that entails a high energy cost to the organism. The estimated energy expenditure associated with protein synthesis may account on average 23% of the total energy expenditure in ruminants (Caton et al., Reference Caton, Bauer and Hidari2000), a figure that agrees with theoretical estimates found in other species. This estimate represents, however, a minimal energy-cost related to total protein turnover since associated energy requirements for amino acid and associated ion transport, RNA turnover, metabolic regulation and protein degradation are not taken into consideration. Fractional protein turnover rate (i.e. protein degradation per unit of protein mass and time; %/day) is thus commonly regarded as a general index of individual variation in energy requirements for maintenance (Hawkins, Reference Hawkins1991), and it has been evoked as a potential determinant of between-animal differences in animal performance (Hawkins, Reference Hawkins1991), and RFI (Richardson and Herd, Reference Richardson, Herd, Archer and Arthur2004).
From a theoretical point of view, the most economical way of achieving higher growth efficiencies would be by a reduction in the protein degradation rate rather than by an increase in the synthesis rate. However, this is not always observed in the literature. Different relationships are obtained when analysing available data (Supplementary Table S2; Studies#16 to 32) from metabolic studies carried out in different livestock species in which changes in feed efficiency were measured at the same time as fractional protein degradation rate (assumed here to reflect changes in protein turnover rate for growing animals). Overall, in most of the evaluated studies, efficient individuals seemed to adopt a low protein turnover strategy (Studies #16 to 25). In contrast, other authors reported that efficient individuals show either no differences in protein fractional degradation rates or a concomitant increase (Supplementary Table S2). Despite its associated energy cost, an increase in protein turnover may be still compatible with higher feed efficiency provided that the proportion of total protein synthesis retained as net protein (i.e. efficiency of retention of synthesized proteins) increases, or in other words as far as protein synthesis increases at a higher extent than protein degradation. These somewhat controversial relationships shown in Supplementary Table S2 may stem from differences in methods used for measuring protein degradation (isotopic methods v. 3-methylhistidine marker v. postmortem enzymatic activities), the way to rank animals in terms of extreme feed efficiency phenotypes, the animal species and their stage of maturity. From data shown in Supplementary Table S2 it can be noted in some cases higher feed efficiency was associated with lower intakes (RFI), whereas in other studies lower intake was not observed. Feed intake, and especially protein intake, strongly affect protein turnover in several different ways (Waterlow, Reference Waterlow2006), and thus the association between feed efficiency and protein turnover rate should be assessed with caution in relation to concomitant changes in feed intake. Indeed, when efficient genetic lines consuming less feed were compared to unselected lines in poultry a lower protein turnover rate in muscle was reported (Study#16a in Supplementary Table S2). In contrast, in the same study when selection pressure was for higher growth rates, entailing both a higher feed intake and feed efficiency (lower FCR), no changes in protein turnover compared with unselected lines were observed (Study#16b). This highlights the importance of the choice of the feed efficiency index when assessing the role of potential biological determinants. This is well illustrated also by results reported within the same study ranking beef cattle through two different feed efficiency indices: protein turnover rate did not correlate with RFI (Study #27a) but a negative relationship was found with FCR (more efficient cattle showed higher protein turnover; Study #27b). When looking at the few studies in Supplementary Table S2 where feed efficiency was compared at similar DM intake, in an attempt to avoid the confounding effect of intake, results suggest a role of protein turnover rate beyond the effect of intake: two out three fish studies (Studies #17 and 18) found that whole-body protein turnover decreased in high growth-efficient animals, whereas the third did not find statistical differences (Study #26). In ruminants protein turnover rate has been shown to increase as the level of intake increases (Lobley et al., Reference Lobley, Sinclair, Grant, Miller, Mantle, Calder, Warkup and Maltin2000) but this relationship could be completely inverted in high growth-efficiency genetic lines (Studies #19 and 21). Interestingly and in the same direction, a different protein degradation response to feed intake was observed in healthy human adults classed as efficient v. non-efficient in terms of feed protein utilization: the most efficient individuals decreased their protein degradation as feed intake increased to a greater extent than less efficient ones (Fereday et al., Reference Fereday, Gibson, Malcolm and Millward1998). Overall, this could indicate that genetic variation might exist concerning the protein degradation response to feed intake, variations likely driven by hormonal regulation (Oddy et al., Reference Oddy, Spec, Warren and Wynn1995).
In conclusion, although there is a scarcity of protein turnover data obtained in beef cattle through isotopic methods in vivo, most of reported studies in livestock species suggest a negative correlation between feed efficiency and protein turnover rate. Long-term studies are warranted in the future to evaluate in beef cattle the role of protein turnover rate on feed efficiency regardless of intake changes (e.g. residual gain).
Body composition
Nutrient requirement and energetic efficiency of cattle is complex due to differences in relative organ and tissue growth patterns. With increasing age and slaughter weight, the proportions of non-carcass parts, bone and muscle decrease, whereas the proportions of fat in non-carcass, carcass and muscle (marbling) all increase (Moloney and McGee, Reference Moloney and McGee2017). Due to the high metabolic cost associated with organs such as the gastro-intestinal tract and liver it is likely that between-animal variation in the size and functionality of these organs may influence energy requirements (Fitzsimons et al., Reference Fitzsimons, McGee, Keogh, Waters and Kenny2017; Meale et al., Reference Meale, Morgavi, Cassar-Malek, Andueza, Ortigues-Marty, Robins, Schiphorst, Laverroux, Graulet, Boudra and Cantalapiedra-Hijar2017). Although a positive genetic relationship between RFI and the empty digestive tract was noted in pure Charolais young bulls (n=946; r g : 0.42; Renand and Krauss, Reference Renand and Krauss2002), the limited published literature that has examined variation in visceral organ size amongst animals of divergent feed efficiency status is inconsistent (Kenny et al., Reference Kenny, Fitzsimons, Waters and McGee2018). For example, a number of studies have found that high-RFI cattle have a heavier gastro-intestinal tract, reticulo-rumen, liver, kidneys, bladder and/or heart compared their low-RFI counterparts, whereas other studies have failed to establish an effect of RFI status on the weight of these organs (Fitzsimons et al., Reference Fitzsimons, Kenny and McGee2014; Kenny et al., Reference Kenny, Fitzsimons, Waters and McGee2018; Meale et al., Reference Meale, Morgavi, Cassar-Malek, Andueza, Ortigues-Marty, Robins, Schiphorst, Laverroux, Graulet, Boudra and Cantalapiedra-Hijar2017).
Differences in the rates of protein and fat deposition influence feed efficiency and rate of BW gain primarily because fat has higher energy density than protein; however, although more ME is required for fat than for protein deposition (wet tissue basis), maintenance of protein requires more ME than maintenance of fat (Moloney and McGee, Reference Moloney and McGee2017). Currently, there is inconsistency in the published literature on the body compositional differences between cattle divergent in feed efficiency status (Fitzsimons et al., Reference Fitzsimons, McGee, Keogh, Waters and Kenny2017; Kenny et al., Reference Kenny, Fitzsimons, Waters and McGee2018). To evaluate the relationship between RFI status and measures of body composition Kenny et al. (Reference Kenny, Fitzsimons, Waters and McGee2018) conducted a meta-analysis of phenotypic studies that used growing beef cattle offered energy-dense diets. In relation to muscle accretion, they found no statistically significant differences in either live animal or carcass measures between cattle of high- or low-RFI status. Similarly, they failed to observe a statistically significant difference in ultrasonically measured back fat depth between cattle divergent in RFI. It was concluded that RFI rank in growing cattle was not obviously associated with final muscle area, carcass muscle area and change in back fat depth during the linear phase of the growth curve, typical of RFI test periods in many studies. Similarly, inconsistencies in the literature exist or relationships are absent for associations between RFI in beef cattle and systemic metabolic indicator traits for body composition and those connected with anabolic processes (Kenny et al., Reference Kenny, Fitzsimons, Waters and McGee2018); these include, creatinine (negative association, Lawrence et al., Reference Lawrence, Kenny, Earley and McGee2012; Fitzsimons et al., Reference Fitzsimons, Kenny, Fahey and McGee2013; no association, Fitzsimons et al., Reference Fitzsimons, Kenny and McGee2014), leptin (no association, Kelly et al., Reference Kelly, McGee, Crews, Fahey, Wylie and Kenny2010), and IGF-I and insulin (no association, Kelly et al., Reference Kelly, McGee, Crews, Fahey, Wylie and Kenny2010; Lawrence et al., Reference Lawrence, Kenny, Earley and McGee2012). Discrepancies amongst literature reports is certainly exacerbated by variation among studies in breed, gender and stage of physiological maturity of the cattle employed, coupled with huge disparity in methodologies used and reporting of results (Kenny et al., Reference Kenny, Fitzsimons, Waters and McGee2018). Theoretically, greater effects of body composition (fatness) would be expected in cattle with a relatively lower mature weight, especially when offered a high-energy diet. In addition, discrepancies between studies may be influenced by genotype-by-environment interactions. In contrast, a meta-analysis of genetic correlations between RFI and FCR, and body composition traits in growing beef cattle (Berry and Crowley, Reference Berry and Crowley2013) showed that, despite large variability across studies, in general there was a positive association of RFI and FCR with body fat in live animals or carcasses and a general tendency for RFI to be negatively correlated to lean body mass, muscularity and carcass conformation. Given this background, many studies include an adjustment for body fat in the statistical model for computing RFI (e.g. Kelly et al., Reference Kelly, McGee, Crews, Fahey, Wylie and Kenny2010). This adjustment can increase the R 2 of the statistical model from less than one to up to 5 percentage units; however, the significance and contribution of body composition per se to the accuracy of the model is not always stated (Kenny et al., Reference Kenny, Fitzsimons, Waters and McGee2018).
Nevertheless, due to the well-established influence of body fatness on key reproductive events, such as the onset of puberty and resumption of postpartum ovarian cyclicity (Kenny et al., Reference Kenny, Fitzsimons, Waters and McGee2018), female ‘robustness’, particularly associated with mobilization and deposition of body fat reserves, and potentially meat quality traits, this adjustment is prudent. In the context of beef cattle genetic improvement programmes, consideration for potential antagonism among commercially important traits needs to accounted for by using economically weighted, multiple-trait genetic selection indices to identify superior animals (Moloney and McGee, Reference Moloney and McGee2017).
In summary, body composition accounts for a relatively small proportion of the between-animal variation in feed efficiency.
Endocrine system
The endocrine system, an inter-organ communicator, could affect feed efficiency through its role in the regulation of both feed intake and nutrient utilization. Although this appears to be self-evident, most of the experiments looking into the specific role of hormones and neuropeptides in animal-to-animal variations in feed efficiency were not designed to evaluate causality, but rather to assess simple associations.
The gastro-intestinal tract with the attached glands is the first system that senses the diet and its energy, and it secretes hormones that play an important role in regulating both feed intake (i.e. insulin and ghrelin) and nutrient utilization (i.e. glucose-dependent insulinotropic polypeptide, insulin and IGF-1). Diet composition and energy concentration change gut hormone concentrations, regulating ME intake (Relling and Reynolds, Reference Relling and Reynolds2008) and energy utilization (Relling et al., Reference Relling, Crompton, Loerch and Reynolds2014). For example, the expression of some orexigenic (i.e. appetite stimulant) and anorexigenic (i.e. loss of appetite) neuropeptides such as neuropeptide Y or proopiomelanocortin, respectively, differs between high and low RFI animals (Perkins et al., Reference Perkins, Key, Garrett, Foradori, Bratcher, Kriese-Anderson and Brandebourg2014), but unfortunately the experimental design hampers any conclusion beyond a simple association.
Ghrelin concentration has been associated with energy intake/partitioning in ruminants (Jennings et al., Reference Jennings, Wertz-Lutz, Pritchard, Weaver, Keisler and Bruns2011). However, current data does not discern whether ghrelin is a true regulator of DM intake. Foote et al. (Reference Foote, Hales, Lents and Freetly2014) showed an association between plasma total ghrelin concentration and FCR. However, this was dependent on the time when plasma was sampled in relation to the feeding process, because this association was not found in studies with multiple samplings (Foote et al., Reference Foote, Tait, Keisler, Hales and Freetly2016). Moreover, if ghrelin affects feed intake and animal growth, it may depend on the physiological state and type of diet (Jennings et al., Reference Jennings, Wertz-Lutz, Pritchard, Weaver, Keisler and Bruns2011; Foote et al., Reference Foote, Hales, Lents and Freetly2014). The role of glucose-dependent insulinotropic polypeptide on beef cattle has not been studied in detail with no available data evaluating its role on feed efficiency. However, in dairy cows a positive association was found between plasma glucose-dependent insulinotropic polypeptide and milk energy output when data were corrected for ME intake (Relling et al., Reference Relling, Crompton, Loerch and Reynolds2014).
Specific hormones related to nutrient metabolism may have a role in between-animal variation in feed efficiency, especially hormones involved in the somatorophic axis (composed by growth hormone, IGFs and their binding proteins), leptin and cortisol. IGF-1 has been proposed as a biomarker of RFI by some authors because of its stimulation of protein synthesis (Davis et al., 2012), and the fact that protein turnover is one of the main determinants of feed efficiency evoked in the present review. However, given the potential confounding factors, IGF-1 concentration are not always associated with variations in RFI (Supplementary Table S3). Important potential factors responsible for equivocal results could be the differences in diets or in physiological stages of the animals and interactions between the somatorophic axis and other hormones and metabolic pathways. Indeed, IGF-1 has been negatively associated with RFI in most of the studies using low-concentrate or low-energy diets (as described in Supplementary Table S3; Studies #28, 29, 30, 32a, 33a). In contrast, on high-concentrate diets, the response is not always consistent. In some studies there is no association between IGF and RFI, whereas in others it is negative (Supplementary Table S3; Studies #31, 32b and 35) or dependent on the time of sampling (Supplementary Table S3, Study #34). Another hormone whose function has been associated with feed efficiency is leptin. The association of leptin and feed efficiency is inconsistent as well (Supplementary Table S3). For example, while in some studies there is no association between leptin and RFI (Supplementary Table S3; Studies #30, 36a, 40) in others there was either negative (Supplementary Table S3; Study #41) or positive (Table S3; Studies #36b, 37, 38, 39) association. In contrast to the IGF-1 results, literature results do not indicate a different leptin response according to the diet. However, it has been shown within the same studies (Supplementary Table S3, Study #36a,b and 39) that the association of leptin and RFI or FCR depends on the physiological stage of the animal. When leptin was measured in young animals, there was no association with RFI, whereas an association was noted when the analysis was conducted near the end of a fattening period. Multiple sampling during the growing and finishing period will help to remove some of the confounded effects and allow a greater understanding of the role of the hormones on efficiency (Supplementary Table S3, Study #39). Finally, cortisol has been also proposed as a marker of RFI because of its role in increasing protein turnover (Davis et al., 2012). As for IGF-1 and leptin, the association is not always present (Supplementary Table S3). This difference could be attributed to multiple factors, such as stress or sampling time in relation with the circadian cycle of the animal. For example within the same study (Supplementary Table S3, Studies #36c and 36d), there was an association between cortisol and RFI at the end of the animal house confinement (more stress), whereas it was absent at the end of the feedlot period (less stress). Furthermore, while there were no differences when samples are taken in a daily basis, significant associations between RFI and cortisol were found at specific times (evening) when multiple samples were obtained within the same day (Supplementary Table S3, Studies #41a and 41b).
As mentioned earlier, there are many partial and contradictory data on the association of plasma hormone concentrations and feed efficiency in beef cattle (RFI and FCR). Many of the apparent contradictions are due to interactions among plasma hormone concentrations, diet, physiological stage, age of the animals or even the specific sampling time. With the available data and experimental designs it is impossible to conclude that the improvements of RFI or FCR are hormone-driven. In addition, since feed efficiency changes are unavoidably associated with changes in feed (energy) intake when it is measured as RFI (and to a lesser extent to FCR) it is impossible to disentangle the true cause and effect relationships.
Molecular basis of animal-to-animal variation in feed efficiency
As with other complex traits (also referred to as quantitative or polygenic traits), feed efficiency is controlled by many genes which are involved in several molecular mechanisms and pathways causing animal-to-animal genetic variation in different biological functions.
Although traits such as RFI are usually determined to be phenotypically independent of growth and body size, as well as fatness when phenotypes are adjusted for fat, this is not the case from a genetic standpoint as revealed by genomic analysis (Saatchi et al., Reference Saatchi, Schnabel, Taylor and Garrick2014; Ceacero et al., Reference Ceacero, Mercadante, Cyrillo, Canesin, Bonhila and de Albuquerque2016). This is perhaps to be anticipated as analysis of genetic parameters reveal correlations between these traits (e.g. r g ranged from 0.22 to 0.71; Ceacero et al., Reference Ceacero, Mercadante, Cyrillo, Canesin, Bonhila and de Albuquerque2016) and it is expected that some genes would have a pleiotropic effect on these traits (Saatchi et al., Reference Saatchi, Schnabel, Taylor and Garrick2014) and are involved in complex gene networks (see Supplementary Figure S2). This adds another level of complexity to the understanding of biological mechanisms contributing to animal-to-animal variation in feed efficiency and may result in co-selection for other traits when selection is applied for feed efficiency. Nonetheless, recent advances in Omics approaches, sequencing, genotyping, statistical methods and bioinformatics tools have led to new information on the molecular basis of feed efficiency traits.
For this review, and after a detailed analysis of genes, proteins and metabolites associated with RFI (summarized in Supplementary Materials SM1 to SM3 and Supplementary Table S4), we conducted an overall gene interaction network analysis using Ingenuity Pathway Analysis (IPA; Ingenuity Systems, www.ingenuity.com) of the various results described in Supplementary Table S4. Only the most recent and representative studies at each functional level (genes, RNA, proteins and metabolites) are here analysed. In total, 334 genes, proteins and metabolites were identified from literature and used in IPA. Approximately 24 networks and their linked functions involved in the molecular basis of feed efficiency were obtained (see Supplementary Table S5). Combining these networks identified five main biological functions involved in feed efficiency (summarized in Supplementary Figure S2): (1) growth and proliferation, cell cycle and carbohydrate metabolism, (2) energy production and lipid metabolism, (3) cell signalling and transport, (4) organ development and cell and organ morphology, (5) cancer, organismal injury and abnormalities and disease (i.e. genes, proteins, and metabolites involved in inflammation, immunity, disease as well as oxidative stress). These gene interaction networks and their linked functions are in agreement with the general biological mechanisms proposed by Richardson and Herd (Reference Richardson, Herd, Archer and Arthur2004). Nonetheless, they provide more detail about each mechanism as well as the interaction among these mechanisms. For example, the energy production and lipid metabolism networks showed connection between important genes such as ACSL6, UCP2, Peroxisome Proliferator-Activated Receptor Gamma, Coactivator 1 Alpha, and mechanistic target of rapamycin complex 1 (Supplementary Figure S2c). Peroxisome Proliferator-Activated Receptor Gamma, Coactivator 1 Alpha is a key transcriptional coactivator that regulates genes involved in adipogenesis, muscle fibre type determination and mitochondrial biogenesis. The mechanistic target of rapamycin gene is involved in protein synthesis regulated by growth hormone receptor and thyroid-stimulating hormone. Thus, selection for RFI could optimize energy production as well as lipid and protein metabolism.
The results from pathway analyses using Database for Annotation, Visualization and Integrated Discovery v6.8 (Supplementary Table S6) provided several enriched biological pathways and their corresponding genes associated with feed efficiency (Figure 3). Based on the number of genes involved in these pathways, phosphatidylinositol-4,5-bisphosphate 3-kinase and protein kinase B singnalling pathway (PI3K-Akt), mitogen-activated protein kinases (MAPK) and rat sarcome proteins related to signal transduction pathways (Ras) signalling pathways, involved in protein synthesis, cellular growth and proliferation and apoptosis are very important in understanding the molecular basis of feed efficiency. Nonetheless, a study to calculate the variance explained by each and together in total for these pathways is required.
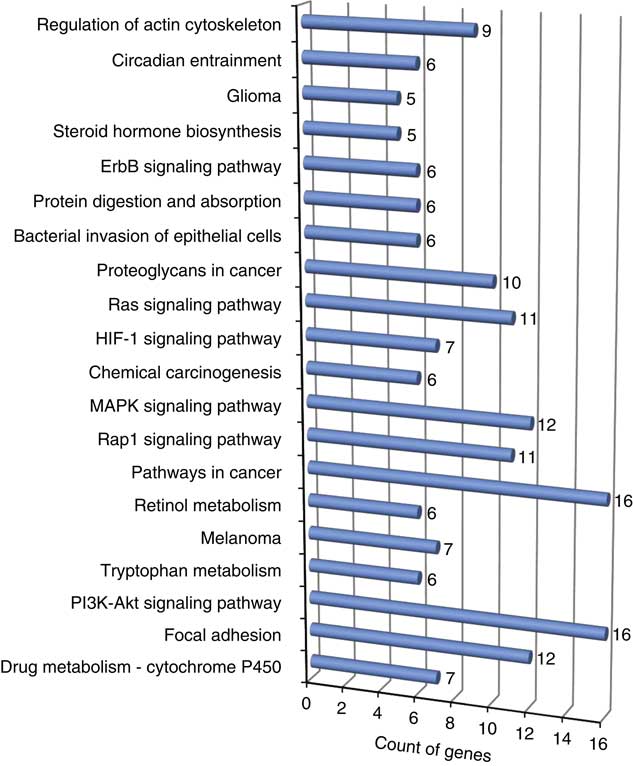
Figure 3 Enriched (P<0.05) biological pathway linked to the discussed gene list associated with feed efficiency in beef cattle. ErbB is the epidermal growth factor family of receptor tyrosine kinases, Ras is the rat sarcome proteins related to signal transduction pathways, MAPK is the mitogen-activated protein kinases, Rap1 is a Ras-related protein 1, PI3K-Akt is the phosphatidylinositol-4,5-bisphosphate 3-kinase and protein kinase B singnaling pathway, and HIF-1 is an hypoxia inducible factor protein (colour online).
Implications for knowledge of molecular pathways and biomarkers
The physiological determinants of feed efficiency or putative biomarkers could be used as a cost-effective and rapid tool for genetic selection or management decisions. Blood (plasma) concentrations of IGF-I, β-hydroxybutyrate, leptin and glucocorticoids (e.g. cortisol) might be indicators for feed efficiency, although no marker has successfully explained enough of the variability in RFI that they were used as part of routine improvement program (Huang et al., Reference Huang, Hines and Davis2006). Other putative biomarkers such as creatine, hipurate and carnitine were proposed to be used as a selection tool for feed efficiency (Karisa et al., Reference Karisa, Thomson, Wang, Li, Montanholi, Miller, Moore and Plastow2014). Furthermore, using putative causative mutations in genes associated with feed efficiency in a customized genetic marker panel may improve breeding value prediction and result in more consistent prediction across breeds and management groups. It is anticipated that the continued development of such markers will result in a cost-effective genomic tool to select efficient and productive crossbred animals in the near future.
Selection for efficient animals for optimal processes (activation or inhibition) of different biological or physiological functions will be possible. However, other traits including growth, meat quality, fertility, life-time productivity and robustness as well as behavioural traits can be affected with different magnitude and direction when selecting for feed efficiency. These effects may result from genes with pleiotropic effects or from linkage between genes. For example, genetic correlations were found between RFI and subcutaneous fat thickness (r=0.37) and rump fat thickness (r=0.32) even after accounting for these traits in the model (Ceacero et al., Reference Ceacero, Mercadante, Cyrillo, Canesin, Bonhila and de Albuquerque2016). This may be explained by the down-regulation of genes involved in fat deposition in adipose and muscle tissue in efficient animals (Weber et al., Reference Weber, Welly, Van Eenennaam, Young, Porto-Neto, Reverter and Rincon2016). Body fatness and actual body composition play critical roles in male and female fertility traits. As a result efficient bulls had delayed sexual maturity as a strong relationship exists between the onset of puberty and RFI in cattle even after adjustment for body composition (Huang et al., Reference Huang, Hines and Davis2006). Although selection of efficient animals based on FCR was not found to affect female fertility traits, selection of efficient animals based on RFI had an unfavourable effect on days to calving (Mu et al., Reference Mu, Vander Voort, Abo-Ismail, Ventura, Jamrozik and Miller2016). Genes involved in the MAPK signalling pathway and linked to tissue development especially ossification and adipose tissue were found to have pleotropic effects on calving ease and birth weight (Saatchi et al., Reference Saatchi, Schnabel, Taylor and Garrick2014). Regulating protein degradation through the gene networks linked to growth and proliferation may explain the association between RFI and carcass and meat quality traits including tenderness but also a possible trade-off between feed efficiency (which benefits from low-protein turnover) and adaptability and robustness (which benefits from high-protein turnover) of animals (Rauw et al., Reference Rauw, Kanis, Noordhuizen-Stassen and Grommers1998). In conclusion, selecting efficient animals may result in genetic change in growth, decrease in feed intake, subcutaneous fat thickness, delayed sexual maturity as well as lean meat and lower animal robustness. Incorporating these traits or their putative biomarkers within a selection index should avoid these potential negative effects to result in desired improvement in efficiency and other important economic traits.
Conclusions
As anticipated, the number of potential mechanisms involved in animal-to-animal variation in feed efficiency is huge and inter-connected. However, several conclusions can be drawn from this review. First, feeding and digestive-related mechanisms (i.e. feeding behaviour, digestion, methane emission and rumen microbiome) seem to be associated with RFI mainly because they co-vary with DM intake. Their role as true determinants of animal variability in feed efficiency could be minor. However, this should be confirmed in future experiments by (i) controlling DM intake in the analysis of biological determinants (e.g. animal ranking based on residual gain), (ii) combining different physiological measures in the same study and (iii) testing the relationship in grazing conditions or with high-forage diets. Concerning the metabolic-related mechanisms, despite the scarcity of studies using reference methods it seems that efficient (low-RFI) animals have a significantly lower energy metabolic rate regardless of the associated intake reduction. Energy metabolism could thus be a true determinant of animal-to-animal variation in feed efficiency. The lower heat production (from maintenance and production) in efficient (low-RFI) animals may stem from both a decreased protein turnover and a higher efficiency of ATP production in mitochondria, as both mechanisms were also identified in the molecular network analysis conducted in the present review. In contrast, hormones and body composition could not be conclusively related to animal-to-animal variation in feed efficiency. The analysis of potential biological networks underlying RFI variations highlighted other significant pathways such as lipid metabolism and immunity and stress response. The relevance of these pathways should be confirmed in further studies combining information gathered at different molecular levels (genes, RNA, protein and metabolites). Finally, emerging knowledge suggests that metabolic functions underlying genetic variation in feed efficiency (protein turnover, lipid metabolism, immunity) could be associated with other important traits in animal production. This emphasizes the relevance of understanding the biological basis of relevant animal traits to better define future balanced breeding programmes.
Supplementary materials
To view supplementary material for this article, please visit https://doi.org/10.1017/S1751731118001489
Acknowledgements
The authors would like to thank Dr Zhou and Dr Morgavi for their advices on the rumen microbiota section. G.C.-H. thanks the scientific committee of the 10th International Symposium on the Nutrition of Herbivores for proposing him to make this review.
Declaration of interest
Authors declare no conflicts of interest.
Ethics statement
Not applicable.
Software and data repository resources
Not applicable.