Introduction
The coronavirus disease 2019 (COVID-19) pandemic created an unprecedented disruption to most aspects of human life with over 400 million confirmed cases and 5 million deaths worldwide. Reference Hiscott, Alexandridi and Muscolini1 In response, governments, healthcare systems, and individuals mobilized resources to mitigate the spread of the virus and protect public health. Reference Ayouni, Maatoug and Dhouib2,Reference Talic, Shah and Wild3
Amidst the viral endemic, a parallel “pandemic” of misinformation and disinformation spread, challenging public health responses. Misinformation (unintended false information) and disinformation (designed to deceive) proliferated across social media, creating confusion and mistrust about the virus’s origin, prevention methods, and vaccine efficacy. Reference Saleh, McDonald and Basit4,Reference Saleh, Lehmann, McDonald, Basit and Medford5 This false information not only fueled conspiracy theories and unfounded claims Reference Enea, Eisenbeck and Carreno6 but also affected public behavior and attitudes significantly, undermining efforts to control the pandemic. 7
Given the serious negative effects of the COVID-19 pandemic on human morbidity and mortality and on economic recovery and given the ensuing false information exacerbating the pandemic’s effects, we determined that research into the extent and effect of false information was of critical importance. To further understand the extent and effect of false information in the context of the pandemic and in anticipation of future public health crises, we conducted a review of the scientific literature to provide a comprehensive overview of the current state of research on COVID-19 mis- and disinformation, including its frequency, the sources, and the effect on individuals and communities.
Methods
Data collection
Using selected search terms (Figure 1), we performed a PubMed query for English-language publications on false COVID-19 information published from March 1, 2020, to December 31, 2022. Reviewing the entire article, we screened all manuscripts by eliminating publications that did not discuss false information or were not related to COVID-19. We used the remaining articles for the manual topic modeling. Once that was completed, we eliminated manuscripts without abstracts, which were required to conduct the automated topic modeling and sentiment analysis. The Preferred Reporting Items for Systematic Reviews and Meta-Analyses (PRISMA) Reference Page, McKenzie and Bossuyt8 standards were applied to select the studies included in the analysis.

Figure 1. Search terms used for PubMed query.
Manual topic review
All remaining manuscripts were grouped manually into one or more topics and linked to a sentiment generated by automated sentiment analysis. The categories for manual topic review were selected a priori using an exhaustive framework designed to examine and sort pandemic disinformation and identify the complexities and repercussions of false information dissemination. To ensure inter-rater reliability, 10% of the abstracts were reviewed by 5 independent reviewers, who categorized the abstracts independently before comparing and discussing discrepancies to reach consensus. Prior to the manual screening, we identified 7 topics based on existing literature and expert input from authors experienced in misinformation research for the manual review of publications:
Source of misinformation
Persons/groups/entities/governments, who published the misinformation including individuals, private corporations, celebrities, anonymous sources, health professionals, educational institutions, etc.
Intent and motivation
The motive behind the false information, whether the intent was positive or negative, and if any conspiracy theories related to the pandemic were described.
Distribution routes
The platforms and networks used to spread false information related to the pandemic may have included social media platforms such as Facebook, Instagram, Twitter (now X), and YouTube and traditional media outlets such as newspapers, magazines, radio, television, and word of mouth.
Topic of misinformation
The specific topics or themes that were misrepresented or distorted through the spread of false information related to the pandemic were captured and included false advertising by companies, false information related to the etiology, source, treatment, or transmission of the virus, fears about the vaccine efficacy and side effects, and satirical or parody information.
Potential harm
The potential harm was described as short- or long-term harm that was associated with the spread of false information related to the pandemic including harm to public health, the economy, social cohesion, or individual rights and freedoms.
Mitigation and prevention
Strategies for preventing or mitigating the spread of false information related to the pandemic, which included fact-checking, improving media literacy, creating public awareness campaigns, and regulating social media platforms.
Censorship
Described means used to censor or suppress posted content and information and who suppressed and censored the information.
Topic modeling
We conducted both manual and automatic topic modeling. As a substantial set of studies did not include an abstract, which was used for the automated modeling, we wanted to evaluate our hypothesis that the omission of studies without an abstract would not result in a distortion of models.
For the manual topic modeling, we cleaned and reviewed 868 articles pertaining to COVID-19 mis/disinformation. After establishing the list of topics, reviewers collaboratively analyzed and categorized each study based on the primary topic it addressed or discussed. This approach ensured a consistent and comprehensive categorization of the literature.
The automated topic modeling was completed by processing and cleaning 639 abstracts from the selected articles (229 articles lacked abstracts). After generating a corpus from bigrams and trigrams from the dataset, we used a latent Dirichlet allocation (LDA) model estimation algorithm from the Gensim library Reference Sojka and Řehůřek9 to train the model varying the number of topics from 1 to 20. To compare the models objectively, we computed a C_V coherence score, which measures the similarity between words within each topic. Reference Stevens, Kegelmeyer, Andrzejewski and Buttler10 Based on this measure, a model with 4 topics emerged as the most parsimonious using sample abstracts and the most common terms found in the abstracts. Subsequently, we labeled the topics in the automated model manually using the top 20 keywords and 10 random abstracts for each topic. This labeling was done by 2 individuals not involved in the topic development. The individuals collectively assigned labels or descriptions for each topic through a consensus method.
Sentiment and emotion analysis
To categorize feelings on informal text samples like abstracts more accurately, we used the SentiStrength library to score abstracts based on the emotion in the language of the abstract after stripping out symbols, URLs, and other irrelevant content. The scale to report sentiment ranged from −3 to +3, with −3 being an exceedingly negative sentiment and +3 representing an exceptionally pleasant sentiment. Reference Thelwall, Buckley, Paltoglou, Cai and Kappas11 Zero is considered neutral without positive or negative emotional connotations. We grouped the processed abstracts into categories based on the likelihood that they contained 1 of 5 emotions: joy, anger, sadness, surprise, and fear, using the text2emotion library. Reference Aman Gupta, Sharma and Bilakhiya12
Results
Our initial query yielded 17,744 publications, 868 of which were included in our analysis and further analyzed to identify content categories and frames of reference. Duplicate publications were removed, and others were excluded if they were not COVID-19 related, not published within the study time frame, had designed protocols that were different from traditional ones, or had outcome evaluation methods that were uncommon. We removed 229 publications because they were found to have no abstract required for the automated topic modeling and sentiment analysis (Figure 2).
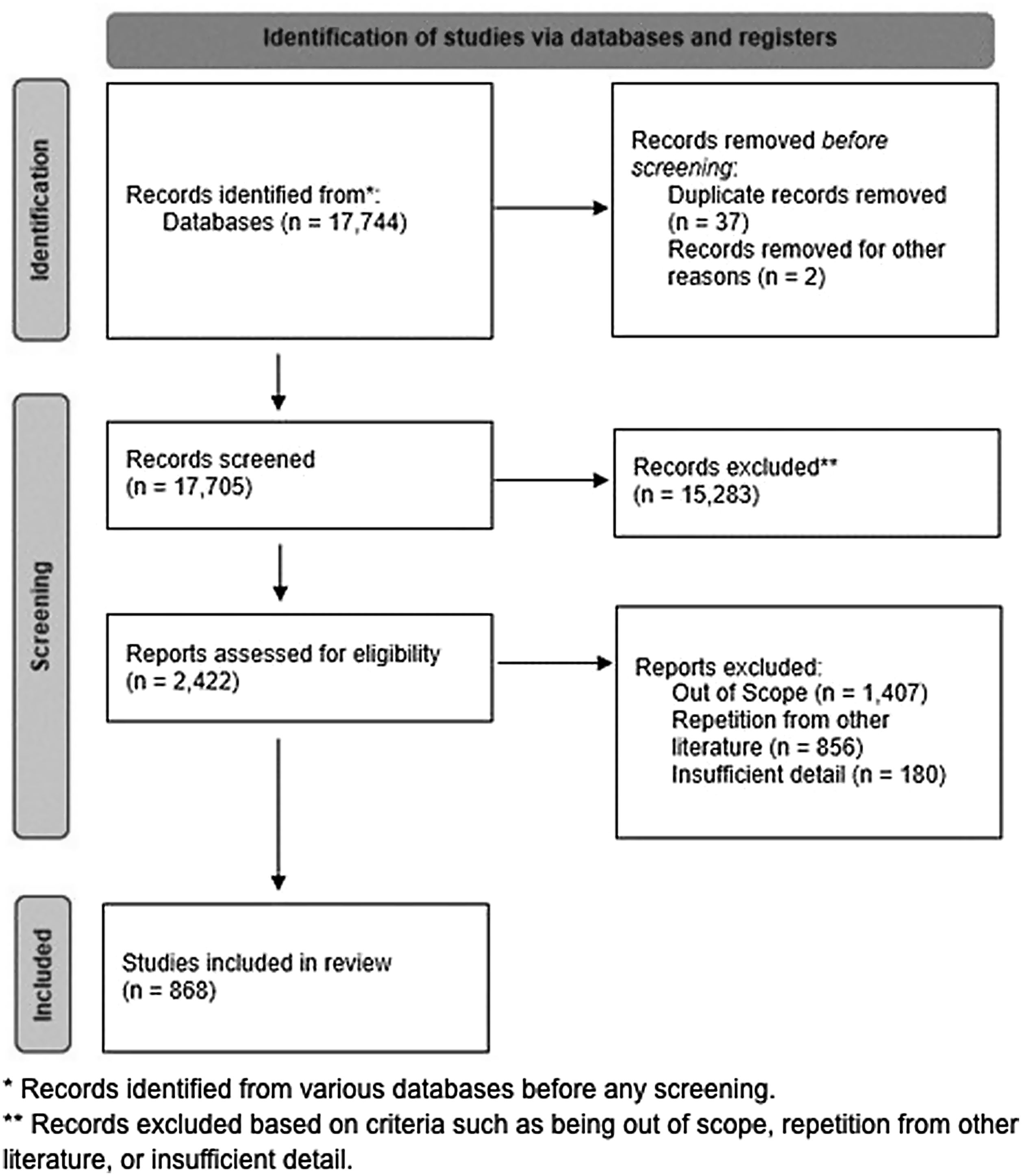
Figure 2. CONSORT diagram detailing the literature review process.
Of the 868 publications in our data set, the majority were published in 2021 (349), followed by 2022 (331), and 2020 (188).
Manual topic review
Of the 639 publications reviewed, most (298, 29%) were grouped in the “Mitigation and Prevention” category with 207 (20%) in the category “Topic of Misinformation,” 193 (18.8%) in “Potential Harm,” and 188 (18.3%) in “Distribution Routes” (Figure 3). The remaining categories, such as “Source of Misinformation” (34, 3.3%) and “Censorship,” (5, 0.5%) contained the lowest number of publications.
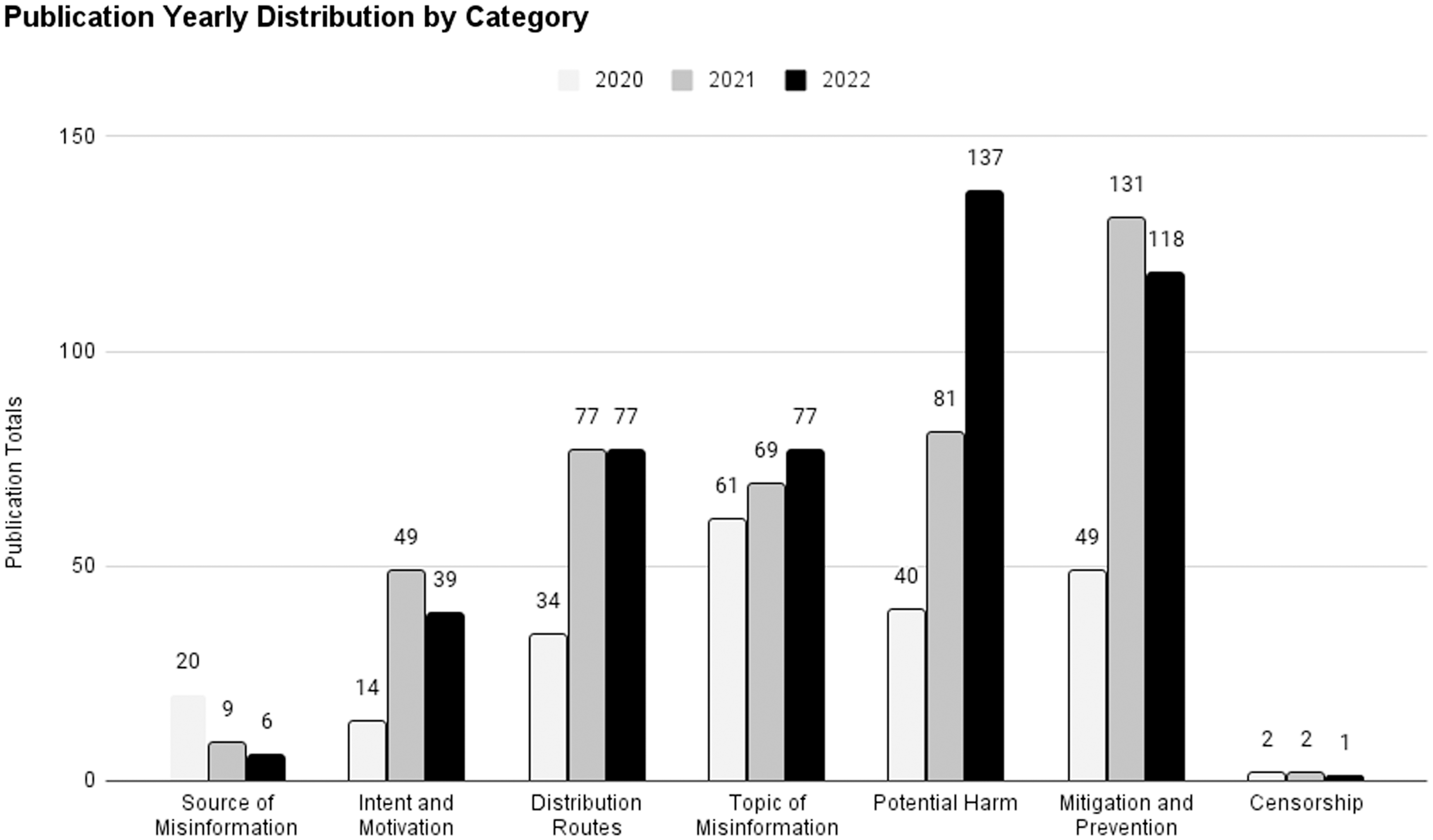
Figure 3. Publication counts per topic each year between 2020 and 2022.
When COVID-19 cases were reported in the United States in early 2020, the number of publications in all topic categories increased especially between 2020 (220) to 2021 (418), which was followed by a slight increase overall in categories in 2022 (455). The category of “Mitigation and Prevention” had a dwindling number of publications in 2020 (49) but saw a significant increase in 2021 (131) and remained high in 2022 (118). The category of “Intent and Motivation” had a low count of publications in 2020 (14) but saw a significant increase in 2021 (49) and remained high in 2022 (39). When we reviewed publications that focused on the distribution of false information, Twitter was the subject of the most publications with 67 (10.5%), followed by website/web searches with 34 (5.3%), and Google-related products with 31 (4.9%).
Word frequency
After excluding the key terms used in our search query, the most frequently used word within our data set was “social” with 983 uses. The next 9 most frequently found words were “medium” (917), “study” (668), “public” (655), “news” (540), “result” (486), “relate” (458), “conspiracy” (390), “fake” (339), and “online” (336).
Topic modeling
Based on the 4 topics identified in the LDA model, 39.6% of the reviewed studies fell into the “COVID prevention and COVID vaccine misinformation,” whereas “Analyzing hoaxes, misinformation, and quality of content on COVID,” only accounted for 11.7% of the complete data set. The other topics included “An infodemic: describing the amount and content of misinformation” and “Surveying people on exposure to and knowledge of COVID-associated misinformation” accounting for 26% and 22.7% of the reviewed publications, respectively (Table 1).
Table 1. Topic modeling and sentiment analysis of 639 publication abstracts for each topic identified by the latent Dirichlet allocation model

Sentiment and emotion analysis
Overall, the sentiment for 56% of abstracts skewed negatively with a sentiment score <0 (mean sentiment score of −0.685) on the −3 to +3 scale. Neutral sentiments were (scores of zero) noted in 32% of all the abstracts, and 11% were classified as positive with a sentiment score >0 (Figure 4). The average sentiment scores of all the reviewed topics were negative, with the most negative topic being “An infodemic: describing the amount and content of misinformation” with a mean sentiment score of −0.78. The topic with the highest mean sentiment score was the topic covering “Analyzing hoaxes, misinformation, and quality of content on COVID,” with a mean sentiment score of −0.53.

Figure 4. Sentiment analysis of 639 publication abstracts between 2020 and 2022.
Like the proportion of articles abstracts with negative mean sentiment scores, our analysis of abstract emotions found most to convey negative emotions. Of the 5 emotions included in our study (happy, anger, surprise, sadness, and fear), 82.2% (525) of included studies portrayed negative emotions (anger, sadness, or fear emotions), with fear, found in 58.9% (376) of the abstracts reviewed (Figure 5). Happiness and surprise emotions that were identified in the publications reviewed only comprised 7% and 4.6%, respectively.

Figure 5. Emotion analysis of publication abstracts.
Discussion
The COVID-19 pandemic generated an unprecedented surge in the dissemination of dis- and misinformation, fueling confusion and sometimes panic among the general population. Reference Clemente-Suárez, Navarro-Jiménez and Simón-Sanjurjo13 Automatic topic modeling of 639 scientific studies analyzing the surge published between 2020 and 2022 demonstrated that over 80% of the publications were associated with the emotions of anger, sadness, or fear suggesting how concerned researchers were about this infodemic. Social media platforms played a significant role in amplifying the spread of false information during this period, and the sentiment associated with these studies reviewing this trend was negative 52% of the time. Reference Dang14 Our study supports the concerns that the rapid dissemination of information through social media platforms enabled the swift circulation of unverified claims and conspiracy theories, which fueled fear and anger emotions among researchers and public health officials.
Our study combined manual and automated topic modeling to analyze COVID-19-related dis/misinformation. The manual topic review provided nuanced insights and context-specific categorization, identifying themes like conspiracy theories, false treatment claims, and effects on public behavior. Inter-rater reliability assessments ensured objective and consistent evaluations, minimizing bias. Automated topic modeling, using machine learning algorithms, analyzed a larger corpus of publications efficiently, identifying trends and patterns in misinformation dissemination over time. This dual approach validated the thematic overlap between human expertise and machine learning, highlighting areas of agreement and divergence. The manual review focused on thematic categorization, while the automated model provided large-scale sentiment and emotion analysis.
Additionally, the manual topic selection was expert-informed, and its categories were defined a priori, drawing on the expertise of various subject matter specialists. This played a crucial role in identifying key research questions and designing topic categories that were relevant to the socio-behavioral aspects of misinformation during the pandemic. Reference Ahmed, Sadri and Amini15 Expert involvement ensured that the manual topic selection was rooted in a deep understanding of the dynamics of misinformation and its potential effect on public health and behavior. Reference Ivanov, Tacheva, Alzaidan, Souyris and England16 Interestingly, despite employing different analytical approaches, our study found significant overlap in the thematic outputs of manual and automated topic modeling. Manual topic modeling, which relies on knowledge and predefined categories, and automated topic modeling using the LDA method both highlighted themes like “Mitigation and Prevention,” “Misinformation Topics,” “Potential Harm,” and “Distribution Routes.” This thematic congruence indicates that the fundamental topics of COVID-19 misinformation research remained consistent regardless of the approach taken. The agreement between automated methodologies not only enhances the credibility and validity of our findings but also underscores the effectiveness of our analytical framework in capturing the complexities of misinformation during the pandemic. Reference Hameleers, Humprecht, Möller and Lühring17
We observed in this large group of articles that captured the main part of the pandemic’s mis- and disinformation that fear was the main emotion. The COVID-19 pandemic created an environment of uncertainty and anxiety with significant information needs for the public. The copious amounts of mis- and disinformation generated during this period created concern and fears among individuals analyzing the pandemic of false information. Reference Bavel, Baicker and Boggio18 The combination of a highly contagious virus overwhelmed healthcare systems, and the rapid spread of false information created a perfect storm for the proliferation of fear-inducing narratives. Reference Joffe and Elliott19 Other investigators described that misinformation is spread by text and images and hence may have amplified the fear. Reference Brennen, Simon and Nielsen20 The lack of stringent content moderation mechanisms on some social media platforms allowed false information to flourish unchecked. Consequently, individuals were exposed to misleading information regarding COVID-19 transmission, prevention, and treatment, which may have resulted in misguided behaviors and compromised public health efforts. Reference Escandón, Rasmussen and Bogoch21
The categories of “Mitigation and Prevention (298, 29%),” “Topic of Misinformation (193, 18.8%),” and “Potential Harm (188, 18.3%)” in our manual review garnered significant attention from researchers as indicated by the number of studies. The publication counts for “Mitigation and Prevention” showed substantial growth over the 3 years, rising from 49 (out of 220, 22.3%) publications in 2020 to 131 (out of 418, 31.3%) publications in 2021 and 118 (out of 455, 25.9%) publications in 2022 despite a drop in pandemic cases. This trajectory suggests a heightened focus on developing strategies and interventions to counteract the spread of false information. The increased research interest reflects concern about the effect of false information, particularly within the context of social media platform proliferation and the challenges they pose to public discourse. By overlaying sentiment analysis onto the identified topics, we gained insight into the overall tone and attitude of the discourse. Notably, the sentiment analysis revealed a pervasive negative leaning sentiment within the study abstracts, reflecting the heightened anxiety and concern inherent in discussions surrounding misinformation during a global health crisis.
In summary, the combined approach of manual and automated topic modeling that used sentiment and emotion analysis created a cohesive analytical framework that captured the multifaceted nature of COVID-19-related misinformation. The alignment between negative sentiment and the prevalence of fear as the dominant emotion emphasized the emotional toll of misinformation on individuals and public health efforts. This integrated methodology represents a significant step forward in unraveling the intricate dynamics of misinformation, offering valuable insights for future research, policymaking, and communication strategies in times of crisis.
There were several limitations to this study. First, we used PubMed as the sole data source for publications and limited our search to the English language. This could result in a biased sample, as it primarily includes scientific and medical literature and may not capture the full extent of COVID-19 false information or its research from a variety of sources. We also limited our search to the most active time of the pandemic, and hence our findings may overrepresent the negative spectrum of sentiment and emotions. The choice of specific search terms used in PubMed also might inadvertently exclude certain relevant publications or bias the selection toward specific topics or types of false information. To rectify this, we used a combination of broad and specific search terms to ensure wider coverage of relevant publications to refine the search strategy and maximize inclusivity within the studies identified in PubMed when addressing this potential issue.
Determining and categorizing identified studies can be subjective and different researchers might have varying interpretations. This subjectivity could introduce bias in the analysis and potentially affect the validity of the findings. The identification and categorization of specific topics covered during different periods might be limited by the available data and the ability to accurately classify publications into distinct topics. When addressing this issue, we developed explicit guidelines and criteria for identifying and categorizing false information to minimize subjectivity. We also involved multiple researchers or experts in the categorization process and ensured inter-rater reliability assessments to enhance the validity and reliability of the findings.
Our findings may not represent the broader landscape of COVID-19 false information, as other sources or platforms might have different patterns or labeling of false information, which could make the screening process more difficult to complete accurately. We combined automated or machine learning techniques with manual content analysis to increase efficiency, objectivity, and accuracy in categorizing and analyzing the large volume of publications.
We examined 900 studies on misinformation and disinformation related to COVID-19 released from 2020 to 2022 and discovered widespread negative feelings and emotions. This highlights the pressing need to tackle the public health effects of information. Our study indicates an emphasis on creating strategies to curb and stop the dissemination of details understanding its potential dangers and delving into the reasons behind its creation. It is essential for the scientific and infectious disease communities to ramp up their efforts in conveying information to the public. This goal can be accomplished through fact-checking procedures, public education campaigns, and enhanced media literacy initiatives. By taking steps, the scientific community can play a role in ensuring that accurate evidence-based facts dominate in public discourse, thus lessening the detrimental effects of misinformation and nurturing a more knowledgeable and resilient society.
Acknowledgments
None.
Financial support
This study was supported by grant funding from the Centers for Disease Control and Prevention (U01CK000590), the National Institutes of Health (1R01AI178121), the Texas Health Resources Clinical Scholars Program (R.J.M.), and the National Center for Advancing Translational Sciences of the National Institutes of Health (UL1 TR003163) (C.U.L.).
Competing interests
None.
Publishing ethics
The work presented in this study did not require Institutional Review Board approval. This exemption is justified on the basis that all data utilized in our study is open source and publicly accessible, ensuring transparency and reproducibility of our research findings.
Disclaimers
None.
Data
This review is predicated on an exhaustive analysis of data concerning COVID-19-related misinformation and disinformation. The foundational data set for our investigation was meticulously curated through a comprehensive search of published literature within the PubMed database. We limited our selection to publications that specifically addressed the phenomena of mis- and disinformation related to COVID-19, ensuring a focused and relevant corpus of study. The temporal scope of our data collection spanned from March 1, 2020, through December 31, 2022. This period was chosen to capture the evolving landscape of COVID-19 discourse from the initial stages of the pandemic through to the end of the year 2022.