Introduction
Awareness is the conscious perception of what is being learned and is an oft-used criterion of implicitness (Williams, Reference Williams, Ritchie and Bhatia2009). We define implicit knowledge as unconscious knowledge of linguistic regularities that can be gained mainly from ample input in an input-rich environment. Explicit knowledge is conscious-verbalizable linguistic knowledge that can be acquired, among other things, through instruction. These knowledge types may be the end-products of different processing activities at different stages in the acquisition process (Leow, Reference Leow2015). For instance, learners who focus more on either the linguistic aspects of a message (i.e., form-focused processing) or its meaning (i.e., meaning-focused processing) may build different knowledge bases. Sparked by the distinction between (explicit) learning and (implicit) acquisition (Krashen, Reference Krashen1981, Reference Krashen1985), scholars in second language acquisition (SLA) have advanced a range of hypotheses about how different knowledge types (i.e., explicit and implicit) and processes (i.e., form-focused and meaning-focused) relate. In this study, we examined the longitudinal associations between explicit and implicit knowledge and activity types that facilitate either form-focused or meaning-focused processing. The exploration of these associations will enhance understanding of the interface issue and the role of awareness in L2 acquisition.
Interface of L2 knowledge and processing: A single- and multiple-system view
The interface question concerns the scope of learning without awareness (Andringa, Reference Andringa2020; Godfroid, Reference Godfroid, Godfroid and Hopp2023). Although there is a long tradition of implicit learning research in both cognitive science and SLA, the exact nature of awareness, as a part of the interface question, remains widely debated. Two prevailing views may explain the underlying mechanisms behind awareness and learning: the single-and multiple-system views. The single-system view of explicit and implicit knowledge and learning is often recognized by cognitive scientists (in SLA: see Białystok, Reference Bialystok1982) as a theoretical account of how conscious rules emerge from implicit knowledge (hereafter, the implicit–explicit interface). Explicit knowledge, in a one-system perspective, is viewed as a “re-described form” of implicit knowledge (Hulstijn, 2015, p. 34) whereby the two knowledge types are indistinct but the development of awareness (explicit knowledge) is contingent upon the quality and stability of implicit knowledge (e.g., Cleeremans & Jiménez, Reference Cleeremans, Jiménez, French and Cleeremans2002; Goujon, Didierjean & Poulet, Reference Goujon, Didierjean and Poulet2014; Mathews, Buss, Stanley, Blanchard-Fields, Cho & Druhan, Reference Mathews, Buss, Stanley, Blanchard-Fields, Cho and Druhan1989). In other words, implicit knowledge gradually transitions to explicit knowledge when the quality of the implicit representation exhibits stability (O'Brien & Opie, Reference O'Brien and Opie1999).
In contrast to the cognitive science perspective, many SLA theorists maintain a multiple-system account, with research focused on how explicit knowledge impacts the development of implicit knowledge (hereafter, the explicit–implicit interface). Across different theoretical frameworks of L2 acquisition and perspectives of the interface hypothesis, theorists share three perspectives. First, explicit and implicit knowledge are not mutually transformative, but rather mutually cooperative. That is, explicit and implicit knowledge are two qualitatively distinct knowledge types subserved by different brain regions; as such, they do not directly interact with, transform, or convert into one another (DeKeyser, Reference DeKeyser, Long and Doughty2009, Reference DeKeyser, VanPatten and Williams2014, Reference DeKeyser, Loewen and Sato2017; N. C. Ellis & Larsen-Freeman, Reference Ellis and Larsen-Freeman2006; Hulstijn, Reference Hulstijn2002, Reference Hulstijn, Cummins and Davison2007; Krashen, Reference Krashen1981, Reference Krashen1985; Paradis, Reference Paradis and Ellis1994, Reference Paradis2004, Reference Paradis2009). Second, despite theoretical disagreements about its impact on acquisition and the underlying cognitive mechanisms, explicit practice generates implicit learning opportunities (DeKeyser, Reference DeKeyser, Loewen and Sato2017; Hulstijn, Reference Hulstijn2002; Krashen & Terrell, Reference Krashen and Terrell1983; Paradis, Reference Paradis2009), and explicit registration (noticing) of linguistic forms permits implicit fine-tuning (N. C. Ellis, Reference Ellis2002, Reference Ellis2005). Third, there is an inseparable link between the two types of processing (i.e., form-focused or meaning-focused) and their corresponding knowledge representations (i.e., explicit and implicit knowledge).
As Krashen (Reference Krashen1985) remarked, learned knowledge and explicit instruction enable monitoring and fine-tuning of linguistic accuracy, while acquisition entails the development of implicit knowledge. In his view, L2 acquisition requires ample comprehensible input focused on meaning, not grammar (Krashen, Reference Krashen1981), an idea that reinforces the link between the two types of processing and their corresponding knowledge representations. Although both Paradis (Reference Paradis and Ellis1994, Reference Paradis2004, Reference Paradis2009) and Hulstijn (Reference Hulstijn2002, Reference Hulstijn, Cummins and Davison2007, Reference Hulstijn and Rebuschat2015), and, to a limited extent, Krashen, recognize the influence of explicit knowledge on implicit knowledge, they maintain that this influence is indirect – that is, explicit knowledge guides learners to repeatedly practice constructions containing grammatical regularities, thereby indirectly influencing the establishment of implicit knowledge (Hulstijn, Reference Hulstijn and Rebuschat2015; Paradis, Reference Paradis2009). As such, their view that explicit and implicit knowledge do not interface “in the neurophysiological sense is by no means at variance with the practice-makes-perfect maxim” (Hulstijn, Reference Hulstijn and Rebuschat2015, p. 36).
Skill Acquisition Theory also emphasizes the relationship between processing types and knowledge types. Skill Acquisition theorists hold that explicit L2 knowledge, like other cognitive skills, is proceduralized, and eventually automatized, with deliberate practice through explicit learning (DeKeyser, Reference DeKeyser2020, p. 96). Explicit knowledge does not transform into implicit knowledge; rather, repeated use of one memory system enables “a gradual establishment of another memory system” (DeKeyser, Reference DeKeyser, Loewen and Sato2017, p. 19). As such, more explicit knowledge does not imply less implicit knowledge, nor can explicit knowledge somehow transform into implicit knowledge (e.g., DeKeyser, Reference DeKeyser, Long and Doughty2009, Reference DeKeyser, VanPatten and Williams2014, Reference DeKeyser, Loewen and Sato2017).
Lastly, in the Associative-Cognitive CREED (N. C. Ellis, Reference Ellis2006) account, language acquisition involves frequency-driven, statistical tallying of various input patterns. The more frequent the exposure to these patterns, the stronger the connections between constructions. Importantly, this adaptive fine-tuning occurs subconsciously (implicitly), but when it is hindered by a lack of salience of linguistic forms or prior knowledge blocking unfamiliar cues, explicit knowledge plays a role. As such, explicit knowledge enables the initial, conscious registration of L2 patterns, which in turn facilitates effective meaning-focused processing. This may lead to the development and strengthening of L2 implicit knowledge.
Interface of L2 knowledge and processing: Empirical evidence in SLA
Identifying direct empirical work on the explicit–implicit interface is challenging (DeKeyser, Reference DeKeyser, Loewen and Sato2017). This may be due partly to conceptual ambiguity (e.g., conceptual overlap across supposedly dichotomous terms) and methodological shortcomings (e.g., longitudinal nature of the interface question; validity of L2 knowledge measures). Nevertheless, existing studies on the interface hypothesis as a developmental phenomenon fall into three themes: (i) how L2 instruction types affect online L2 processing; (ii) how L2 instruction types influence L2 knowledge development; and (iii) how one type of L2 knowledge affects the development of the other type (Godfroid, Reference Godfroid, Godfroid and Hopp2023). Combined, these themes may illuminate the trajectory of the interface issue (instruction → processes → knowledge).
Influence of L2 instruction on L2 knowledge
Findings from instructed SLA can inform the interface hypothesis, albeit indirectly, because the differential effects of instruction are often attributed to distinct cognitive processes (observed or assumed) mediating the observed end product – namely, explicit or implicit linguistic knowledge.
Four meta-analyses have synthesized findings from over 90 studies on different instructional types (i.e., Goo, Granena, Yilmaz & Novella, Reference Goo, Granena, Yilmaz, Novella and Rebuschat2015; Kang, Sok & Han, Reference Kang, Sok and Han2019; Norris & Ortega, Reference Norris and Ortega2000; Spada & Tomita, Reference Spada and Tomita2010). Here we focus on the three meta-analyses that explored the effectiveness of instructional treatments on performance on a free constructed task (Goo et al., Reference Goo, Granena, Yilmaz, Novella and Rebuschat2015; Norris & Ortega, Reference Norris and Ortega2000; Spada & Tomita, Reference Spada and Tomita2010). Since free constructed tasks, such as picture description, induce more spontaneous L2 use compared to controlled tests such as metalinguistic judgments, findings of a medium or large effect size for explicit instruction on free response tasks may lend support to the explicit–implicit interface. Across the three meta-analyses, researchers found explicit instruction to have medium effects (d = 0.55 in Norris & Ortega, Reference Norris and Ortega2000) to medium-large effects (d = 0.745 after averaging the effect sizes of complex and simple forms in Spada & Tomita, Reference Spada and Tomita2010; g = 1.443 in Goo et al., Reference Goo, Granena, Yilmaz, Novella and Rebuschat2015) on learners’ free production performance. These results suggest that processing strategies induced by form-focused instruction may lead to L2 implicit knowledge gains. Yet, for this conclusion to be valid, the free-response measures ought to be valid measures of L2 implicit knowledge. Given the synthetic nature of meta-analyses, this question remains open, but it nonetheless highlights some of the difficulties involved in empirically testing the interface hypothesis in its full scope.
Influence of L2 instruction on L2 processing
Recently, using eye-tracking technology, researchers have shown that learners’ online or real-time processing changes as a result of explicit focus-on-form instruction. Cintrón-Valentín and Ellis (Reference Cintrón-Valentín and Ellis2015), for instance, examined how different types of form-focused instruction affect native English speakers’ attentional foci during L2 Latin verb inflection processing. Given that native English speakers tend to attend more to adverb cues than verb cues as markers of temporality, a change in attentional processes (towards the verb cues) along with evidence that the attended cue is used for comprehension or production could be regarded as evidence for an interface. Eye-movement data revealed that all three focus-on-form groups attended more to the verb cues than the uninstructed control participants, who gradually focused less on the verb cues over the study duration. Moreover, the proportion of fixation time on either cue (i.e., the verb or the adverb) during training correlated with participants’ sensitivity to morphological cues during sentence comprehension and production.
Andringa and Curcic (Reference Andringa and Curcic2015), on the other hand, found no support for a knowledge interface. They examined whether learners instructed in the target grammar rule (i.e., differential object marking) exploited the knowledge gained from instruction during an online processing task that required them to use the grammar rule predictively. In this study, provision of grammar instruction played no role, resulting in similar eye movements for the instructed group and the group that did not receive explicit training. In a follow-up study, Curcic, Andringa, and Kuiken (Reference Curcic, Andringa and Kuiken2019) found that participants’ degree of awareness differentially predicted anticipatory processing of the target rule (i.e., gender-based determiner-noun agreement). In particular, learners who reported having gained prediction awareness – a group who described themselves as aware of gender distinctions and the determiners which predicted the picture-matching answers – showed higher levels of predictive processing than the learners who reported awareness of the gender rules (gender aware group) and pattern rules (pattern aware group). Such studies suggest that awareness is a graded concept and that instruction may not induce the same level of awareness and the same predictive processing effects among all participants.
Influence of L2 knowledge on L2 knowledge
Suzuki and DeKeyser's (Reference DeKeyser, Loewen and Sato2017) research is most closely related to the present study and represents an important attempt to study knowledge and knowledge influences. Using six linguistic knowledge tests and three aptitude measures, the authors attempted to examine the explicit–implicit knowledge interface, specifically whether automatized explicit knowledge contributes to the acquisition of implicit knowledge. They ran two structural equation models, termed interface model and noninterface model, which were identical except for an additional path in the interface model that extended from automatized explicit knowledge to implicit knowledge. The models did not differ meaningfully in terms of model fit; however, the path from automatized explicit knowledge to implicit knowledge in the interface model was positive and significant. The results provided suggestive evidence for an interface, which would need to be corroborated statistically in a future study presenting evidence for a better overall model fit of the interface than the noninterface model. Such a study would ideally be longitudinal rather than cross-sectional as in Suzuki and DeKeyser, to demonstrate a true causal relationship between the different knowledge types in L2 development.
Synthesis
The studies reviewed above represent important first steps toward testing the interface hypothesis directly. They also indicate some future research directions:
(i) To account for the developmental aspects of L2 acquisition, which are inherent to the interface hypothesis (DeKeyser, Reference DeKeyser, Loewen and Sato2017), longitudinal research is essential.
(ii) Unlike laboratory experiments with artificial or extinct languages (e.g., Andringa & Curcic, Reference Andringa and Curcic2015; Cintrón-Valentín & Ellis, Reference Cintrón-Valentín and Ellis2015; Curcic et al., Reference Curcic, Andringa and Kuiken2019), immersion in a naturalistic setting would represent an authentic context for research in which the L2 is used as a part of daily life.
(iii) Lastly, in Suzuki and DeKeyser's (Reference DeKeyser, Loewen and Sato2017) study, the three implicit knowledge measures (visual world eye tracking, a word-monitoring task, and self-paced reading) showed a weak convergence, with factor loadings failing to reach statistical significance. This highlights methodological concerns in measuring implicit and explicit knowledge and the importance of validating test measures prior to their utilization (Godfroid & Kim, Reference Godfroid and Kim2021).
Measures of L2 explicit and implicit knowledge
The theoretical importance of the explicit–implicit dichotomy has given rise to an era of test validation research, starting with R. Ellis's landmark study (R. Ellis, Reference Ellis2005). In his study, R. Ellis put forth a set of descriptors for L2 implicit and explicit knowledge, based on which he proposed a battery of five linguistic knowledge measures. Results indicated oral production (OP), elicited imitation (EI), and a timed written grammaticality judgment test (TGJT) loaded onto one factor, which he termed implicit knowledge. Conversely, the untimed written grammaticality judgment test (GJT) and metalinguistic knowledge test (MKT) loaded onto a different factor, which he labeled explicit knowledge (also see R. Ellis & Loewen, Reference Ellis and Loewen2007). More recently, Suzuki and DeKeyser (Reference Suzuki and DeKeyser2015, Reference Suzuki and DeKeyser2017) argued that implicit knowledge measures ought to invite a focus on meaning (as opposed to form) during online (real-time) language processing. As such, EI and the timed GJT may tap into automatized explicit (as opposed to implicit) knowledge because these measures are time-pressured (rather than real-time), and, in the case of the timed GJT, the focus is on form (not meaning). In an effort to address these conflicting views, Godfroid and Kim (Reference Godfroid and Kim2021) brought nine previously used explicit and implicit knowledge measures together, synthesizing 16 years of test validation research. The nine tests included a word monitoring test (WMT), self-paced reading (SPR), EI, OP, timed and untimed GJTs in the aural and written modes, and the MKT.
With the data from 131 non-native English speakers, Godfroid and Kim (Reference Godfroid and Kim2021) examined the predictive validity of implicit-statistical learning ability for knowledge types. We regressed a battery of four implicit learning aptitude tests (auditory statistical learning, visual statistical learning, alternating serial reaction time, and Tower of London) onto two confirmatory factor analysis models: a two-factor model (an extension of R. Ellis, Reference Ellis2005) and a three-factor model (an extension of Suzuki & DeKeyser, Reference DeKeyser, Loewen and Sato2017). We found that implicit-statistical learning ability (i.e., reaction time gains on the alternating serial reaction time task) predicted only the factors that included learners’ performance on timed, accuracy-based language tests. Importantly, implicit-statistical learning aptitude measured by the alternating serial reaction time task did not predict learners’ performance on reaction-time measures in the three-factor model. These results lend support to the validity of timed, accuracy-based language tests as measures of implicit knowledge. Based on these results, we include the same measures in the present study to assess implicit knowledge.
The current study
The following two research questions guided the current study:
RQ1. To what extent does explicit knowledge impact implicit knowledge development? And to what extent does implicit knowledge impact explicit knowledge development?
RQ2. To what extent do the types of activities (form-focused or meaning-focused) in which L2 speakers engage in a naturalistic context contribute to the development of different knowledge types?
Methods
Participants
The study participants were English L2 speakers studying at a large university in the American Midwest. They took part in three testing stages; one administered at the beginning of a semester (i.e., Time 1 [T1]: January-February, 2019), the second at the end of a semester (i.e., Time 2 [T2], April-May, 2019), and the third at the end of 2019 (i.e., Time 3 [T3], November–December, 2019). Data from T1 and T2 are analyzed and presented in the current paper. The mean interval between T1 and T2 was 78.78 days (min-max: 53–106 days).¹ Overall, there was 18 percent participant attrition: 149 participants participated in T1, and 122 participants remained in T2. The participants’ average TOEFL score, or the converted equivalent score from another standardized test, was 93.03 (SD = 13.11) in T1 and 93.61 (SD = 12.72) for those who remained in T2. The demographic information of participants enrolled at each time point is included in Appendix S1, Table S1, Supplementary Materials.
Materials
Target structures
The target structures included six grammatical features: (i) third person singular -s (e.g., *The old man enjoy watching many different famous movies.), (ii) mass/count nouns (e.g., *The girl had rices in her lunch box.), (iii) comparatives (e.g., *It is more harder to learn Chinese than to learn Korean.), (iv) embedded questions (e.g., *She wanted to know why had she studied for the exam.), (v) passive (e.g., *The flowers were pick last winter for the festival.), and (vi) verb complements (e.g., *Kim is told his parents want buying a new car.). These three morphological (1–3) and three syntactic (4–6) structures were selected to represent early and late acquired grammatical features (e.g., R. Ellis, Reference Ellis, Ellis, Loewen, Elder, Erlam, Philp and Reinders2009; Pienemann, Reference Pienemann1989), and were deemed appropriate to measure participants’ general English proficiency. These target structures are identical to those in Godfroid and Kim (Reference Godfroid and Kim2021) and Godfroid, Kim, Hui, and Isbell (Reference Godfroid, Kim, Hui and Isbell2018). A subset of these structures has also been used in previous studies such as R. Ellis (Reference Ellis2005) and Vafaee, Suzuki, and Kachinske (Reference Vafaee, Suzuki and Kachinske2017).
Instruments
We administered two questionnaires (i.e., background and motivation questionnaires), five linguistic knowledge measures and a self-reported Language Exposure Log (LEL). The linguistic knowledge measures were the same as in Godfroid and Kim (Reference Godfroid and Kim2021). Based on the results of this study, we used untimed written grammaticality judgement tests (GJT) and metalinguistic judgement tests (MKT) as measures of learners’ explicit L2 English knowledge. The timed written GJT, oral production (OP), and elicited imitation (EI) served as implicit L2 English knowledge measures.
The types of language engagement (i.e., form-focused activity and meaning-focused activity) were measured with a self-reported LEL. The background and motivation questionnaires and the two oral production tasks (i.e., OP and EI) were newly programmed on the web for participants to complete in the convenience of their homes.Footnote 1 These tasks were programmed on Java with Google Web Toolkit (see Procedure section for details on the administrative process) and were validated as explicit and implicit knowledge measures (Kim, Lu, Chen, & Isbell, Reference Kim, Lu, Chen and Isbell2023). The two GJTs were programmed on SuperLab 5.0, and the metalinguistic knowledge test was programmed on Qualtrics. For a summary, see Table 1.
Table 1. Summary of Measures

Note. G = grammatical; UG = ungrammatical
Oral production (OP)
In the web-programmed OP task, participants read a picture-cued short story, seeded with the target structures. The story consisted of 18 sentences (250 words) and 10 pictures on Mr. Lee's life (Godfroid & Kim, Reference Godfroid and Kim2021). Participants read the story (with picture prompts) twice with unlimited time, and were asked not to take notes but to rely on their memory.
A minimum of one sentence (10 words) to a maximum of four sentences (55 words) accompanied each picture. The custom-made pictures were carefully drawn to emphasize the main content and facilitate memory retrieval. When finished, the participants were asked to retell, in 2.5 minutes, the picture-cued story in as much detail as possible. They were informed that they could not go back to the previous picture once they had moved on (see Appendix S2, Figure S1, Supplementary Materials). During the retelling, the picture remained on the screen for participants to freely proceed to the next picture at their own pace. A progress indicator (e.g., 1 out of 10 pictures) was presented under each picture prompt (see Figure S2, Supplementary Materials).
At the two testing points (T1 and T2), the same story prompt was used because the time interval between the two time points (3–4 months) was sufficiently large to make it unlikely that participants would recall the exact wording of each sentence.
Scoring
Target morphosyntactic structures were coded on two features: the number of times (i) a target structure was required (obligatory contexts), and (ii) the number of times it was correctly applied. Obligatory contexts were defined relative to the participants’ own production. The number correct was divided by the number required to arrive at the overall accuracy score.
Elicited imitation (EI)
In the web-programmed EI task, participants were instructed to listen to sentences, judge their plausibility, and repeat each sentence in correct English after a beeping sound.
The task consisted of 32 sentences, 24 of which were target sentences (four sentences for each structure: two grammatical and two ungrammatical; Godfroid & Kim, Reference Godfroid and Kim2021). Each sentence was between six and 13 words. Eight practice sentences, four grammatical and four ungrammatical, and model responses to two practice sentences were played prior to the test for clarity in task instruction (Erlam, Reference Erlam, Ellis, Loewen, Elder, Erlam, Philp and Reinders2009). At no point did the model responses or instruction include explicit instruction or feedback on the linguistic structures. Furthermore, participants were not explicitly informed that the 32 sentences included ungrammatical trials.
Two counterbalanced lists of stimuli were created for the target sentences. In List 1, half of the sentences were grammatical, and half were ungrammatical; in List 2, the grammaticality was reversed from List 1. List 1 was given at T1, and List 2 was administered at T2.
Scoring
As with the scoring of the OP task, correct use of the target forms in obligatory contexts was used to calculate an overall accuracy score.
Grammaticality judgment tests (GJT)
In the lab-based computerized written GJT, participants were instructed to read the sentence, either under time pressure (timed written GJT) or without time pressure (untimed written GJT), and judge its grammaticality. Both tests consisted of 40 items (24 target sentences, four for each structure, half grammatical, half ungrammatical; Godfroid & Kim, Reference Godfroid and Kim2021). Part of the GJT items were from Vafaee et al. (Reference Vafaee, Suzuki and Kachinske2017), and were modified for sentence length and lexical choices to ensure comparable processing loads across sentences and to avoid lexical repetition across the nine tasks used in Godfroid and Kim (Reference Godfroid and Kim2021).
In the timed written GJT, participants were urged to make judgments as soon as possible. The time limit for each item was set based on the length of audio stimuli in the aural GJT. In particular, we computed the average audio length of sentences with the same sentence length and added 50% of the median. As a result, the time limit imposed for a seven-word sentence was 4.12 seconds, while that for a 14-word sentence was 5.7 seconds.
The procedure was the same for the untimed written GJT, but without a time limit. This extra time was designed to allow participants to draw on their explicit processing. Sentences appeared one at a time in their entirety (font: Helvetica; size: 44) on the computer screen. For each time point and each test, different sets of sentences were used.
Scoring
Correct responses were awarded one point.
Metalinguistic knowledge test (MKT)
Participants were given 12 sentences with grammatical violations, two for each structure (Godfroid & Kim, Reference Godfroid and Kim2021). The participants’ task was to (i) identify the error, (ii) correct the error, and (iii) explain why it was ungrammatical. Prior to the test, two practice questions were provided along with a good and bad response to illustrate the nature of the test. Participants were also allowed to use an online dictionary for translation purposes; for instance, when they knew metalinguistic English terms such as “articles” in their native language but not in English, participants were instructed to use the online dictionary. For each time point, different sets of sentences were used.
Scoring
Correct responses were awarded one point. For the final analysis, only the responses on the explanation component, which required the most explicit declarative knowledge of language, were used.
Language exposure log (LEL)
The self-reported LEL was used to elicit qualitative and quantitative information on participants’ engagement with languages. A modified version of Ranta and Meckelborg's work (Reference Ranta and Meckelborg2013), the log was designed to record daily language use in a fine-grained manner. Each daily log covered 21 hours, from 6 a.m. to 3 a.m., divided into one-hour blocks. For every hour, learners had to provide information in three categories: general language usage, specific activities in the general category, and form- and meaning-based usage. For the purpose of this study, we only used the first and last categories (see Kim, Reference Kim2020 for detailed information on all categories).
The first category, general language usage, collected whether participants used English, native or other languages, or no languages. Overall, six options were provided: speaking, writing, reading, or listening in English, using other languages, and no language use. They were instructed to choose all options they used within each one-hour block.
The last category elicited whether the chosen activity focused on form, meaning, or both. An activity was form-focused when its purpose was to learn the grammatical or lexical forms of the target language. This included learning language aspects in a language course or individually, consulting a dictionary to learn the meaning or forms of words, phrases, or idioms, reviewing papers to correct language usage, or analyzing language to understand a reading. On the other hand, activities were meaning-focused when the focus of language use was on content. Examples included watching television for pleasure, surfing the Internet to gain information, or reading or writing emails to convey messages. The average frequency counts of each category (i.e., form-focused activity and meaning-focused activity) across five logs (see Procedure) served as the scores for analysis.
Procedure
All participants started with the implicit knowledge measures to minimize the possibility of becoming aware of the target structures in the implicit tasks. As mentioned, participants completed the two oral production tasks (i.e., oral production [OP] and elicited imitation [EI]) prior to coming to the lab. The GJTs (untimed GJT [UGJT] and timed GJT [TGJT]) and the MKT were administered in the lab to prevent participants from relying on external resources when completing the tests on the web (e.g., referring to grammar books or browsing the Internet for answers).
With regard to the Language Exposure Log (LEL), participants recorded language usage activities on five days between the two test points (i.e., March and April, 2019). These five LELs represented different weekdays (e.g., Monday [log 5], Tuesday [log 4], Wednesday [log 3], Thursday [log 2], and Friday [log 1]). The five LELs were also distributed across the two months (i.e., three LELs given in March and two in April). On the day the LEL was due to be completed, reminders were sent once at 12 p.m. and again at 6 p.m. The questionnaire was developed using Qualtrics, which functioned in both web and mobile interfaces. See Table S2, Supplementary Materials, for the timeline and test sequence.
Analysis
We ran two sets of cross-lagged path models (CLPM) to examine the causal influences between knowledge types over time. The term cross refers to paths that cross over from one variable to another (e.g., paths c and d in Figure 1), and lagged indicates the temporary separation between the constructs. Thus, cross-lagged effects refer to the predictive effects of explicit knowledge at T1 on implicit knowledge development at T2 (path d), and implicit knowledge at T1 on explicit knowledge at T2 (path c). It is important that the cross-lagged effects accounted for the residual variance at T2 – that is, the amount of variance left unexplained after everything else in the model had been accounted for. Specifically, the CPLM also included two autoregressive paths (i.e., paths a and b) with which we controlled for the prior level of explicit (implicit) knowledge at T1 on explicit (implicit) knowledge at T2. These two autoregressive paths offset claims that cross-lagged effects were simply due to explicit knowledge and implicit knowledge at T1 being highly correlated.

Fig. 1. Path diagram for a two-wave, two-variable path model. T1 = Time 1; T2 = Time 2; a and b = autoregressive paths; c and d = cross-lagged paths.
The path models utilized factor scores obtained from two confirmatory factor analyses (CFAs) to represent the constructs of explicit and implicit knowledge. To that end, we performed two CFAs (CFA at T1 and T2) in which the explicit knowledge construct was composed of two linguistic tests (i.e., UGJT, MKT), and the implicit knowledge construct was modeled with three instruments (i.e., EI, OP, TGJT). The EI and UGJT served as the reference indicators for the implicit and explicit constructs, respectively, and the two latent variables were correlated in model specification. The extracted factor scores were composite variables which controlled for measurement error in our measurement instruments. We then used these extracted factor scores as observed variables (measures of explicit and implicit knowledge) in the CLPM.
We evaluated the CFA and CLPM models based on two major aspects: (i) global goodness of fit, and (ii) presence or absence of localized strain. The goodness of fit indices provided a global summary of the acceptability of the model – that is, whether the model was properly specified. The model fit indices considered were the χ2 statistic (and the corresponding df and p value), root mean square error of approximation (RMSEA, corrects for model complexity considering sample size) and its 90% confidence interval (CI), the standardized root mean square residual (SRMR), and comparative fit index (CFI, compares the fitted model to a base model with no parameter restrictions). We followed Hu and Bentler's (Reference Hu and Bentler1999) and Kline's (Reference Kline2016) guidelines for fit interpretation (i.e., RMSEA lower-bound confidence interval value <= 0.06, which yields a nonsignificant p value, SRMR values <= 0.08, and CFI values >= .95). All CFA and CLPM analyses were conducted in R version 1.2.1335 using the lavaan package.
If these model fit indices were poor (suggesting that the model significantly departs from the data), we used two statistics to identify localized areas of ill fit: residuals and the modification index (MI). The standardized residuals provide specific information about the differences between model-implied and sample covariances. Generally, larger differences suggest overestimation or underestimation of the difference between model-implied and sample covariances. Since standardized residuals are z-scores, indices above the value of |1.96| are flagged, as 1.96 corresponds to a statistically significant z-score at p < .05 (Brown, Reference Brown2015). The MIs reflect how well the model fit (χ2) would improve if a new path were added to the model. Indices of 3.84 or greater suggest that the addition of such a path will statistically improve the overall fit of the model (a value of 3.84 indexes χ2 at p < .05; Brown, Reference Brown2015).
Results
In this section we provide overall descriptive results of all linguistic measures at T1 and T2, with visualization of performance changes from T1 to T2 separately by tests. We then report a series of CFA and path analysis results. For a missing data analysis and missing value patterns by tests and time points, see Appendix S3, Supplementary Materials.
Descriptive statistics for language tests
Table 2 summarizes descriptive results of the five linguistic variables at T1 and T2. Unlike other tasks, markedly high OP mean scores are shown at both timepoints. These results are on par with previous studies: Godfroid et al. (Reference Godfroid, Kim, Hui and Isbell2018) reported 89% accuracy with 151 intermediate-to-advanced L2 English speakers (M = 96, iBT TOEFL); R. Ellis (Reference Ellis2005) reported 72% accuracy with beginning-to-intermediate L2 learners (M = 6.25, IELTS, which converts to 60–78 iBT TOEFL). Numerically, a general improvement in average scores was observed between the two time points for all tests. Different versions of multivariate normality tests (i.e., Mardia, Henze-Zirkler's, and Doornik-Hansen's MVN tests) jointly flagged the data as disproportionate (all p < .001). To accommodate multivariate normality assumption violations, we used the robust maximum likelihood (MLR) estimator method for the CFA.
Table 2. Descriptive Information of Five Linguistic Tests at T1 and T2

Note. OP = oral production; EI = elicited imitation; TGJT = timed written grammaticality judgment task; UGJT = untimed written grammaticality judgment task; MKT = metalinguistic knowledge test; T1 = Time 1; T2 = Time 2; aPearson r inter-rater reliability; bCronbach's alpha.
Figure 2 plots individuals’ performance changes from T1 to T2 separately by test for explicit knowledge measures (top two) and implicit knowledge measures (bottom three). As might be expected, bi-directional changes were observed across all tests, with some participants showing (steep) improvements from T1 to T2 while others performed poorly or maintained comparable scores at T2. A noticeable improvement was observed for the two explicit knowledge measures, which was statistically confirmed with medium within-subject effect sizes (0.85 < d < 0.93). For the implicit knowledge measures, participants performed significantly better for all three tests except for the timed written GJT at T2. The effects of the improvement of implicit knowledge were small (0.17 < d < 0.35). See Table S3, Supplementary Materials, for the summary of effect sizes.

Fig. 2. Spaghetti plots of explicit knowledge measures (top two) and implicit knowledge measures (bottom three) at T1 and T2. Plotted lines represent individual participants’ performance changes from T1 to T2.
Factor scores
We performed two two-factor CFA models on the linguistic tests, separately for T1 and T2. Overall, both models fit the data adequately. No modification indices were larger than 3.84 (largest = 1.73), and no standardized residual was greater than |1.96| (largest = 0.001), suggesting there were no localized areas of ill fit in our model specification. At the same time, a relatively high correlation was observed between the explicit and implicit latent variables in both time points (T1: r = 0.719, T2: r = 0.784). Factor correlations above .80 or .85 are typically flagged for showing poor discriminant validity (Brown, Reference Brown2015, p. 116). To examine if the data was better represented as reflecting a single underlying construct, we also ran a one-factor model. Goodness of fit and modification indices both suggested the two-factor model to be superior to a one-factor model at T1 (χ2 p value = .020; low CFI = .932; one modification index exceeding 3.84) and T2 (χ2 p value = .063; low CFI = .928; two modification indices over 3.84). In addition, we compared the AIC indices using aictab(). Both two-factor models had a lower AIC value at T1 (two- vs. one-factor: −554.37 vs. −548.77) and at T2 (two- vs. one-factor: −575.26 vs. −574.49). Based on the converging statistical and theoretical evidence, we concluded that the two-factor model fit the data best in both time points and was superior to the one-factor model. The global goodness of fit indices are summarized in Table S4, Supplementary Materials.
With an acceptable measurement solution established, we extracted factor scores, using the lavPredict() function in R with a regression method, and used the scores as proxies for latent variables of explicit and implicit knowledge at T1 and T2. For the activity measures, we used z-score converted, average frequency of form-focused and meaning-focused activity engagement across the five LEL logs. For descriptive results of the four factor scores and z-scores for the two activity measures, see Table S5, Supplementary Materials.
Path analysis
In this section, we report a series of path analyses to address the associations between explicit and implicit knowledge. To share the entire model selection process, we divided the results into two parts. In Part 1, we report the findings of two competing models:
I. Non-interface Model: No cross-lagged paths
II. Interface Model: One cross-lagged path from Explicit T1 to Implicit T2
In Part 2, we report an additional model that later proved to be a better-fitting model. The model was:
III. Reciprocal-interface Model: Two cross-lagged paths from Implicit T1 to Explicit T2 and from Explicit T1 to Implicit T2
Figure 3 represents the three models. Two pieces of evidence would count as support for the explicit–implicit interface position: (i) a better statistical fit of the Interface Model than the Non-interface Model, together with (ii) a positive, significant interface path from explicit knowledge (T1) to implicit knowledge (T2). Similarly, the superiority of the Reciprocal-interface Model would be evidenced by (i) a better statistical fit than the Non-interface and Interface Models, and (ii) positive, significant interface paths – that is, both the explicit–implicit and implicit–explicit paths between T1 and T2. In all models, we included two activity variables – meaning-focused and form-focused – as regression predictors of both types of knowledge at T2.
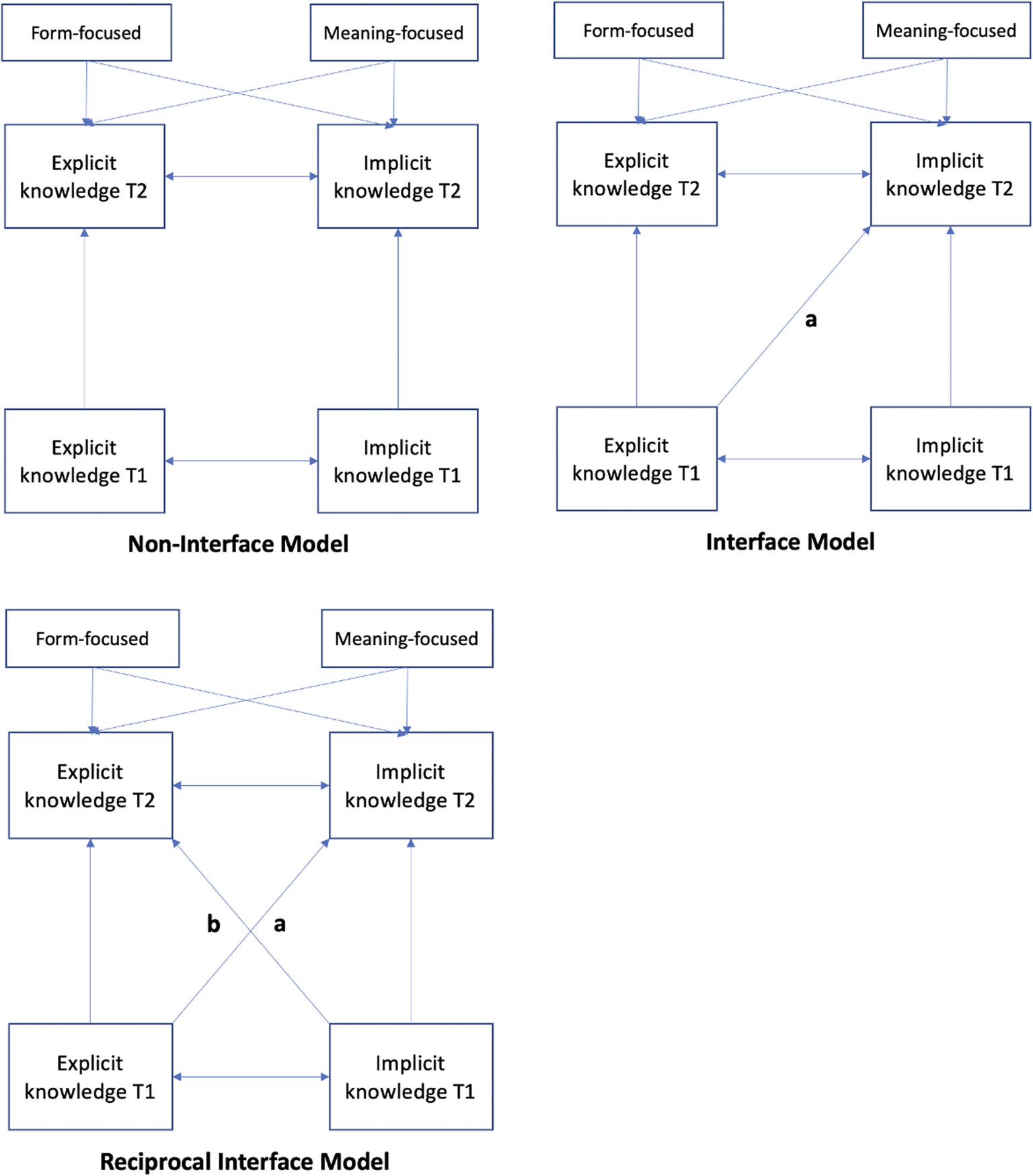
Fig. 3. The Non-interface Model (top left); The Interface Model (top right); The Reciprocal-interface Model (bottom left); Paths a and b represent the explicit–implicit interface and the implicit–explicit interface, respectively.
Part 1: Non-interface vs. Interface Model
Table 3 summarizes the model fit indices of both the Interface and the Non-interface models.
Table 3. Model Fit Indices for the Interface and Non-interface Models

Note. χ2 = chi-square; χ2 p = chi-square test p-value; df = degree of freedom; CFI = comparative fit index; SRMR = standardized root mean square; RMSEA = root mean square error of association.
All fit indices in the Non-interface Model were clearly poor. The Interface Model, on the other hand, revealed a mixed picture: while the values of CFI and SRMR were acceptable, the lower bound of RMSEA was slightly higher than the recommended cutoff point of 0.05. Moreover, the chi-square test was significant, suggesting that the model significantly departed from the data. Typically, RMSEAs with low df (and sample size) generate artificially large values of the RMSEA and falsely indicate a poor-fitting model (Kenny, Reference Kenny2015). This is because the computational formula of the RMSEA is highly dependent on df and n size: √(χ2−df)/ √df(N-1) (for this reason, Kenny, Kaniskan & McCoach, Reference Kenny, Kaniskan and McCoach2015 proposed to not compute the RMSEA with low df models). Similarly, the significant chi-square shown in the Interface Model may be a combined result of a low df, smaller samples, and multivariate non-normality (Hayduk, Cummings, Boadu, Pazderka-Robinson & Boulianne, Reference Hayduk, Cummings, Boadu, Pazderka-Robinson and Boulianne2007), all of which contribute to a high Type 1 error rate (i.e., falsely concluding that the model significantly departs from the data; Kenny, Reference Kenny2015).
A scaled chi-square difference test suggested that the Interface Model was a significantly better-fitting model than the Non-interface Model: χdif = 37.339, df = 1, p = 0.001. The interface path from Explicit T1 to Implicit T2 was significant (p < .001). Taken together, these results suggest that explicit knowledge at T1 influences the development of subsequent implicit knowledge at T2.
Part 2: Non-interface vs. Interface vs. Reciprocal-interface Models
While the above results lend support to the explicit–implicit interface, we explored ways in which the Interface Model could be improved by inspecting the MIs. Of several respecification suggestions, one modification was theoretically sound: a path from Implicit T1 to Explicit T2, modeling that implicit knowledge may influence explicit rule discovery (e.g., Bialystok, Reference Bialystok and Ellis1994, Reference Bialystok2001; Cleeremans, Reference Cleeremans2007). No standardized residuals exceeded .10. Thus, a structural path from Implicit at T1 to Explicit at T2 was added to the model for model fit improvement.
As seen in Table 4, the addition of a new regression path improved the model fit of the Reciprocal-interface Model, yielding good fit indices within an acceptable range. A series of scaled chi-square difference tests suggested that the Reciprocal-interface Model was a significantly better-fitting model than the Non-interface Model, χdif = 54.126, df = 2, p < 0.001, and the Interface Model, χdif = 9.356, df = 1, p < 0.001.
Table 4. Model Fit Indices for the Reciprocal-interface Model

Note. χ2 = chi-square; χ2 p = chi-square test p-value; df = degree of freedom; CFI = comparative fit index; SRMR = standardized root mean square; RMSEA = root mean square error of association.
Having established that the Reciprocal-interface Model best described our data, we interpreted the parameter estimates only of this model (see Table 5). As expected, the results indicated that all autoregressive paths were significant. In particular, the strongest predictor of current implicit knowledge was prior implicit knowledge (Std. Est. = 0.483), and the strongest predictor of current explicit knowledge was prior explicit knowledge (Std. Est. = 0.385). This suggests that participants showed steady improvement in their explicit and implicit knowledge development, and that individual differences in both types of knowledge were stable over the lag between T1 and T2. Critically, the hypothesized interface path from Explicit T1 to Implicit T2 (the cross-lagged path) was also significant, suggesting that explicit knowledge led to an increase in implicit knowledge even after controlling for previous levels of implicit knowledge. The interface path had a standardized coefficient estimate of 0.329, a predictive magnitude that was as strong as the autoregressive impact of Explicit T1 on Explicit T2 (the CIs for the standardized coefficient estimate cross over across the pair). At the same time, the implicit–explicit cross-lagged path was also significant. This indicated that learners’ explicit knowledge at T2 was influenced by their previous levels of implicit knowledge at T1. Importantly, the predictive magnitude of Implicit T1 on Explicit T2 was comparable to the impact of Explicit T1 on Implicit T2. As such, we conclude that both knowledge types had a reciprocal relationship, mutually impacting each other bi-directionally with a comparable predictive strength.
Table 5. Model Parameter Estimates for the Reciprocal-interface Model

Lastly, none of the activity type measures predicted the development of implicit or explicit knowledge, suggesting a non-significant effect in their contribution to knowledge development.
Summary of results
In the current sample of L2 English speakers immersed in the target language environment for the pursuit of higher education studies,
• Finding 1: The strongest predictor of current explicit knowledge was prior explicit knowledge; the strongest predictor of current implicit knowledge was prior implicit knowledge.
◦ Evidence: The significance of the two autoregressive paths from explicit knowledge at T1 to T2 and implicit knowledge at T1 to T2.
• Finding 2: Previous explicit knowledge had a positive impact on the development of implicit knowledge.
◦ Evidence: A better statistical fit of the Reciprocal-interface model (which includes both cross paths) compared to the Interface and Non-interface models, together with a positive, significant path from explicit knowledge (T1) to implicit knowledge (T2).
• Finding 3: Explicit knowledge was highly influenced by the previous levels of implicit knowledge.
◦ Evidence: A better statistical fit of the Reciprocal-interface model (which includes both cross paths) compared to the Interface and Non-interface models, together with a positive, significant path from implicit knowledge (T1) to explicit knowledge (T2).
• Finding 4: Type of activity had no predictive impact on knowledge development.
◦ Evidence: The paths from form- and meaning-focused activity to either knowledge type were not statistically significant.
Discussion and conclusion
We compared the longitudinal causal impact of two types of L2 knowledge (i.e., explicit knowledge and implicit knowledge) and their relationship to different activity types (i.e., form-focused and meaning-focused). In a two-time-point longitudinal experiment, we demonstrated a mutually beneficial relationship between explicit and implicit L2 morphosyntactic knowledge. The best-fitting model revealed that both types of knowledge had a reciprocal association; that is, they mutually, bi-directionally affected each other and functioned as mutual causes and consequences. None of the activity types predicted knowledge development.
Explicit knowledge impacts implicit knowledge development
A central aim of our project was to examine, using empirical data from a naturalistic language immersion setting, the extent to which explicit L2 knowledge plays a causal role in the development of implicit L2 knowledge. Results of a two-time-point CLPM confirmed that the Reciprocal-interface Model with two cross-lagged paths (explicit–implicit interface and implicit–explicit interface) fit significantly better than either the Interface or the Non-interface Models. Given that the explicit–implicit interface path in the Reciprocal-interface Model was positive and significant, we demonstrated a beneficial effect of explicit knowledge on the development of implicit knowledge in an authentic English-language environment.
Our study contributes to SLA research by providing support for the explicit–implicit interface in the form of initial empirical evidence based on (i) a natural language, (ii) a naturalistic context, and (iii) a longitudinal study design. These three combined components are important because the acquisition of implicit L2 knowledge, and acquisition of language in general, is a developmental process mediated by L2 exposure that is qualitatively and quantitatively different from lab-based experiments. Our findings therefore represent an important extension of those lab-based intervention studies with artificial or extinct languages (Cintrón-Valentín & Ellis, Reference Cintrón-Valentín and Ellis2015; Curcic et al., Reference Curcic, Andringa and Kuiken2019) by showing that similar developmental phenomena are at play in naturalistic language use contexts as in the lab.
While the exact mechanism underlying the explicit–implicit interface remains unclear, one theoretical explanation is that the conscious registration of linguistic patterns in the input, associated with (conscious) noticing and explicit knowledge, creates a conscious channel that enables further tallying of these patterns and their associated regularities implicitly (N. C. Ellis, Reference Ellis2005, Reference Ellis and Rebuschat2015). Alternatively, exposure to patterns, driven by conscious knowledge of their form, meaning, or both, could allow for more practice with and attention to the patterns, which in turn could facilitate the development of their implicit representations (DeKeyser, Reference DeKeyser, Long and Doughty2009; Hulstijn, Reference Hulstijn2002, Reference Hulstijn, Cummins and Davison2007, Reference Hulstijn and Rebuschat2015; Paradis, Reference Paradis and Ellis1994, Reference Paradis2004, Reference Paradis2009).
Our longitudinal results built on two CFAs that were specified based on R. Ellis’ measurement framework (R. Ellis, Reference Ellis2005), and replicated the empirical results of Godfroid and Kim (Reference Godfroid and Kim2021) for a subset of their tests. Nonetheless, in this study, at both T 1 and T 2, the factor correlations for explicit and implicit knowledge were relatively high, at r = .83, right at the border of what is considered poor discriminant validity between latent variables (Brown, Reference Brown2015). The explicit and implicit knowledge CFA constructs, however, certainly had some discriminant validity. The corresponding two-factor CFA models were the best fit for the data and were demonstrably (and statistically) superior to one-factor CFA models, which assumed that there was one type of linguistic knowledge only and hence no discriminant validity between explicit and implicit knowledge. Those one-factor models were shown to be a poorer fit to our data than the two-factor models. Recall also that in the CPLMs, we controlled for participants’ prior level of explicit (implicit) knowledge at T1 on their explicit (implicit) knowledge at T2, which allowed for a cleaner, more rigorous test of the interface paths.
These reassuring differences notwithstanding, we acknowledge that language performance is complex and no behavioral measure is likely to afford a pure measure of either explicit or implicit knowledge (R. Ellis, Reference Ellis2005); explicit knowledge is expected to make some (comparatively smaller) contribution to participants’ performance on implicit knowledge tests, and vice versa. Because our study was one of the first longitudinal examinations of the interface question, we do not yet know how this contribution changes over time and might impact any interface paths. Reassuringly, however, the changes in test performance over time for individual tests may provide some insights, given that only measures of explicit–declarative knowledge are likely to be susceptible to practice effects (e.g., DeKeyser, Reference DeKeyser2020) and show improvement over time. Consistent with this view, at T2 we observed strong practice effects for the explicit knowledge tests (i.e., MKT, UGJT; 0.90 < d < 0.93, see Table S3), which contrasted with small practice effects for the implicit measures (i.e., OP, EI, TGJT; 0.17 < d < 0.38). Hence, this comparison suggests a limited contribution of explicit knowledge to measures of implicit knowledge and bolsters our key finding that explicit knowledge facilitates the development of implicit knowledge over time. Simply put, our results support the notion of an explicit–implicit knowledge interface.
Implicit knowledge impacts explicit knowledge development
Another finding of this study was that implicit knowledge had a positive impact on explicit L2 knowledge development. In fact, the standardized coefficients of the two cross-lagged paths in the Reciprocal-interface Model demonstrated that the predictive impact of implicit knowledge on explicit knowledge was comparable in magnitude to the impact of explicit knowledge on implicit knowledge (see Table 5). While the interface debate in SLA centers primarily on the facilitative role of explicit knowledge in implicit knowledge development, the observed pattern of results (i.e., the reciprocal relationship between explicit and implicit L2 knowledge) suggests that awareness not only facilitates implicit learning and knowledge, but also can be a product of implicit learning and knowledge.
This idea of a reverse interface has a long history in the problem-solving and cognitive psychology literature.
The notion of “insight” is a term from problem-solving that refers to a sudden recognition of solutions (Mayer, Reference Mayer, Sternberg and Davidson1995, p. 3). In the context of L1 and L2 acquisition, an insight can manifest itself as the emergence of rule awareness from implicitly accrued linguistic knowledge (e.g., Bialystok, Reference Bialystok and Ellis1994, Reference Bialystok2001; Cleeremans, Reference Cleeremans2007), such as awareness of the abstract meaning of a verb argument construction in English. Evidence of rule discovery has been empirically tested in relation to memory consolidation where awareness arises after a period of sleep (e.g., Batterink, Oudiette, Reber & Paller, Reference Batterink, Oudiette, Reber and Paller2014; Fischer, Drosopoulos, Tsen & Born, Reference Fischer, Drosopoulos, Tsen and Born2006; Wagner, Gais, Haider, Verleger & Born, Reference Wagner, Gais, Haider, Verleger and Born2004; Wilhelm, Rose, Imhof, Rasch, Büchel & Born, Reference Wilhelm, Rose, Imhof, Rasch, Büchel and Born2013). Our study is one of the first to find the implicit–explicit interface in the L2 research context with a natural language. As such, the current findings suggest that claims from cognitive science about non-linguistic pattern discovery can also be extrapolated to L2 research context with natural language acquisition (also see Andringa, Reference Andringa2020, which used a miniature language).
Both the single- and multiple-system views may account for the mechanisms behind rule discovery. No qualitative differences are assumed, in a single-system perspective, between the two knowledge types; instead, development of awareness is contingent upon the quality and stability of implicit learning and knowledge. On the contrary, from a multiple-system view, the two knowledge types are qualitatively distinct; as such, implicit knowledge cannot become conscious by increased stability, and explicit processing does not have direct access to the contents of implicit knowledge (e.g., Esser & Haider, Reference Esser and Haider2017; Haider & Frensch, Reference Haider and Frensch2005; Rünger & Frensch, Reference Rünger and Frensch2008). Rather, rule knowledge emerges from a form of behavioral change; for instance, unexpected events during implicit learning might trigger explicit hypothesis testing. While this study was not a test of the underpinnings of consciousness, an investigation of this topic would represent a valuable avenue for future research aimed at understanding and theorizing the role of awareness in L2 acquisition (for a similar attempt, see Williams, Reference Williams2018).
Activity types
Lastly, frequency of activity types made little contribution to the acquisition of explicit and implicit knowledge. The standardized coefficients of both form-focused and meaning-focused activity did not predict explicit and implicit knowledge development in a significant manner (see Table 5), although at a descriptive level, the effects of form-focusing activity and meaning-focused activity went in opposite directions. The directional paths of meaning-focused activity to both knowledge types were negative, whereas those from form-focused activity were positive. Although the interpretation of these non-significant paths warrants caution, the positive predictive power of form-focused activity, compared to meaning-focused activity, on the acquisition of both explicit and implicit knowledge aligns with the superior effectiveness of explicit over implicit instruction reported in meta-analytic reviews. For instance, Goo et al. (Reference Goo, Granena, Yilmaz, Novella and Rebuschat2015) reported medium effect size differences on learner performance on free production (g = 0.454) and constrained production (g = 0.584), with explicit instruction being superior – a finding consistently reported since Norris and Ortega (Reference Norris and Ortega2000), and reaffirmed in Spada and Tomita (Reference Spada and Tomita2010). Perhaps L2 speakers, including more advanced learners, may benefit more from focused attention to linguistic forms, at least for some morphosyntactic structures and in certain contexts.
While the above interpretation may partially explain the stronger effects of form-focused activity, why more meaning-focused activity induces less acquisition of L2 knowledge types remains unclear. It may be that the relationship between meaning-oriented input and language development is linear until a certain point, beyond which the effects of language exposure may diminish. It might therefore be interesting to extend this study with beginning or intermediate L2 speakers to explore whether the same pattern of results manifests itself. Another reason for the null association may relate to the measurement. In particular, the “meaning-focused activity” category may be somewhat crude, and perhaps certain types of meaning-focused activity – for instance, meaningful engagement during L2 comprehension or L2 production – may be associated more with language gains. On top of this, narrowing the time-segments to 30 minutes or even 15 minutes (as in Ranta & Meckelborg, Reference Ranta and Meckelborg2013) may provide researchers with a more comprehensive report on learners’ L2 engagement patterns. In essence, to better understand the association between processing and knowledge, a more fine-grained approach to analyzing different types of meaning-focused activity combined with learner characteristics may be needed.
Methodological innovations in the study
Methodologically, we introduced notable technological advances in this study. First, the web-programmed language production tasks (OP and EI) were efficient tools for remote data collection even before the COVID-19 global health crisis increased the demand for reliable online data collection alternatives. Technical errors in the platform were minimal. For instance, in OP, the task that had the most missing data, 4.96 percent (at T2) to 9.40 percent (at T1) of data were missing due to poor-quality recordings or missing files. These numbers are relatively trivial considering the need for good online data collection tools and the overall volume of data that can be collected online. With no commercial software available to support remote oral recording functions at the time of data collection, the current project showed that such a program can be a viable alternative to in-person data collection (see Kim et al., Reference Kim, Lu, Chen and Isbell2023).
Second, the self-recorded language exposure log (LEL), which recorded participants’ L2 usage data in real time, hour by hour, functioned as a good alternative to offline retrospective questionnaires. The participant logs collected through this device revealed substantial variation in how much (and for what reasons) international college students in the U.S. engaged with English in an immersion context (Kim, Reference Kim2020). Additionally, a host of other research topics can be addressed using this device, including: language engagement patterns of L2 speakers in EFL and ESL contexts; the quality and quantity of L2 use by cultural background; or conceptual issues such as whether a linear relationship exists between input and language development; or whether there is a threshold beyond which the effects of language exposure diminish.
Limitations and future directions
To our knowledge, this study was one of the first to investigate the explicit–implicit interface question longitudinally. Despite its significance, some limitations of the study must be considered when interpreting the results.
First, from a statistical standpoint, the analyses in this study were based on factor scores rather than longitudinal structural equational modelling (LSEM). The complex nature of LSEM, combined with our fairly small sample size, would have necessitated imposing unjustifiable and non-theory-driven constraints on the data (e.g., fixing covariances of latent variables to zero or error covariances of the same instruments across time to zero). As such, we used a simpler path model with factor scores. The path analysis accounted for measurement error in the different linguistic measures, just like LSEM, but at the same time it imposed an unwarranted – or at least untested – assumption of factorial invariance, which is the notion that instruments measure the same constructs over time. Hence, because we did not test for factorial invariance, the longitudinal effects should be interpreted with caution.
Second, practice and retest effects in our study results cannot be overlooked. This may apply to the OP task, which utilized the same story prompt at both time points, and, to some extent, the EI task, which differed only in the grammaticality of items across the two sets of sentences at T1 and T2. Generating more stories with picture prompts for OP was not possible with the current budget, but future researchers could vary the OP prompts at each time point to ensure a comparable difficulty/complexity of the stories. Regarding EI, researchers could also employ different sets of items after carefully controlling for comparability. Of note, we did not observe a clear improvement in OP performance (T1 = 89% and T2 = 93%) or EI (T1 = 64% and T2 = 69%), which would have occurred if practice effects were notable. Nevertheless, it might be better to use different test forms at each time point if their comparability can be assured.
Lastly, our findings were based on participants who had a wide range of L2 proficiency levels and lengths of residence in L2-speaking countries. Eighteen participants were beginning-to-intermediate L2 users (TOEFL score between 60–78), 80 were intermediate-to-advanced (TOEFL score between 79–100), and 43 were advanced. While the inclusion of a wide range of L2 English speakers allowed us to examine the developmental changes across a large L2 learner population, the current results may not be replicated with different subsamples (e.g., beginning-to-intermediate L2 speakers with limited experience abroad). Future researchers could thus seek to replicate the present study's design with a more tightly controlled sample to examine the robustness of the results at different proficiency levels.
Conclusion
To our knowledge, this study, with its large-scale, longitudinal design, was the first to capture the developmental aspects of the interface hypothesis in an ecologically valid setting. We therefore invite more research testing the reproducibility and generalizability of our findings in different learning contexts and on wider subsamples to advance and refine our understanding of the interface question. Longitudinal research has much to gain from newer approaches to data collection, including the web-based and mobile-supported collection of data. We would welcome more collaborations between SLA researchers and computer scientists to this end in order to effectively and efficiently track learners' language development in society.
Supplementary Material
Supplementary material can be found online at https://doi.org/10.1017/S1366728922000773
Acknowledgements
This study was supported by the National Science Foundation Doctoral Dissertation Research Improvement Grant (NSF BCS-1844694), the International Research Foundation for English Language Education (TIRF) Doctoral Dissertation Grant, a National Federation of Modern Language Teachers Associations (NFMLTA) Dissertation Support Grant, and several internal funds from Michigan State University, including a Dissertation Completion Fellowship (DCF) from the College of Arts and Letters and the Second Language Studies program.
We would like to express our appreciation to Xiaobin Chen who helped with web programming the oral production and elicited imitation tasks; Nick Ellis, Susan Gass, Shawn Loewen, Yuichi Suzuki, and Paula Winke for their constructive feedback on this project; Dylan Burton, Brittany Carter, Steven Gagnon, Sanguk Lee, Marisol Masso, and Amr Rabie for their help in data coding; Dustin Crowther, Stella He, Bronson Hui, Elizabeth Huntley, Daniel Isbell, Ryo Maie, Kiyo Suga, and Russell Werner for their assistance in piloting; and Brittany Finch for her editorial assistance. Hope Akaeze, Frank Lawrence, and Steven Pierce from the Center for Statistical Consulting at Michigan State University provided valuable insights on data analysis. Lastly, endless thanks go to all our participants, who remained with us and this project for one entire year.
Data Availability
The Data that support the finding will be deposited in Dataverse with a one-year embargo period from the date of publication to allow for commercialization of research findings.