Highlights
-
• Self-regulated learning appears not to be hindered by L2 processing.
-
• Bilingual participants monitored the to-be-studied material correctly.
-
• Low-proficiency bilinguals could not compensate for the perceived language difficulty.
-
• L2 level may impact the resources available for metacognitive processing.
When we read, many cognitive and metacognitive processes operate so that we understand what is in a text. These processes include word recognition, information updating, inferencing, integration with previous knowledge, monitoring, and control of cognitive resources (Castles et al., Reference Castles, Rastle and Nation2018). For expert readers, most of these processes take place rapidly, automatically, and with minimal effort in their native tongue (L1). However, one might expect these processes to be more effortful when reading in a second language (L2), especially when L2 proficiency is not at native-like levels. This is relevant since in many countries, English as an L2 is increasingly used as the medium of instruction (Byun et al., Reference Byun, Chu, Kim, Park, Kim and Jung2011; Macaro, Reference Macaro2018; Pessoa et al., Reference Pessoa, Miller and Kaufer2014). Despite the clear benefits of L2 education in favoring transnational networking and communication in the long term (Dafouz & Camacho-Miñano, Reference Dafouz and Camacho-Miñano2016; Doiz et al., Reference Doiz, Lasagabaster and Sierra2013), many students with lower proficiency may struggle when they take courses in L2 and face the challenge of reading and studying text materials in English–L2. In this context, addressing how metacognitive and learning strategies unfold when studying in L2 is paramount. The focus of the present study is to investigate the consequences of studying texts in L2 in the interplay between monitoring and control strategies to achieve successful learning.
Previous studies have established that working in L2 is cognitively demanding and many resources might be directed to language control (e.g., Green & Abutalebi, Reference Green and Abutalebi2013; Ma et al., Reference Ma, Hu, Xi, Shen, Ge, Geng and Yao2014; Moreno et al., Reference Moreno, Bialystok, Wodniecka and Alain2010; Soares et al., Reference Soares, Oliveira, Ferreira, Comesaña, MacEdo, Ferré, Acuña-Fariña, Hernández-Cabrera and Fraga2019). For example, within a bilingual brain, both languages are active during production or comprehension even in contexts when only one language is being used (Bialystok, Reference Bialystok2017; Bialystok et al., Reference Bialystok, Craik and Luk2012; Chen et al., Reference Chen, Bobb, Hoshino and Marian2017; Iniesta et al., Reference Iniesta, Paolieri, Serrano and Bajo2021; Kroll et al., Reference Kroll, Bobb and Hoshino2014). To manage the interference from one language to the other, and to select the appropriate language for the context, language control processes need to be engaged (Beatty-Martínez et al., Reference Beatty-Martínez, Navarro-Torres, Dussias, Bajo, Guzzardo Tamargo and Kroll2020; Kroll et al., Reference Kroll, Dussias, Bice and Perrotti2015; Macizo et al., Reference Macizo, Bajo and Cruz Martín2010; Soares et al., Reference Soares, Oliveira, Ferreira, Comesaña, MacEdo, Ferré, Acuña-Fariña, Hernández-Cabrera and Fraga2019).
This might be even more so for unbalanced bilinguals whose asymmetrical language proficiency (Luk & Kroll, Reference Luk, Kroll, Dunlosky and Rawson2019) leads them to have weaker L2 semantic representations (Kroll & Stewart, Reference Kroll and Stewart1994), more interference from their L1 (e.g., Meuter & Allport, Reference Meuter and Allport1999), and slower and less accurate L2 processing and word recognition (Dirix et al., Reference Dirix, Vander Beken, De Bruyne, Brysbaert and Duyck2020). All this suggests that, for unbalanced bilinguals, L2 processing is more challenging and therefore might engage more cognitive resources than L1 processing (Hessel & Schroeder, Reference Hessel and Schroeder2020, Reference Hessel and Schroeder2022; Pérez et al., Reference Pérez, Hansen and Bajo2018; see Adesope et al., Reference Adesope, Lavin, Thompson and Ungerleider2010 for a review).
Research on second language acquisition suggests that the ability of L2 readers to construct the necessary inferences for forming situation models might be constrained by their proficiency in L2 reading and vocabulary (Joh & Plakans, Reference Joh and Plakans2017; Nassaji, Reference Nassaji2011; Sidek & Rahim, Reference Sidek and Rahim2015). Thus, whereas high-proficient readers are likely to effectively employ their prior knowledge to enhance their comprehension of the text, low-proficiency readers often struggle to construct accurate situation models, impeding their capacity to make inferences and to acquire the causal relations presented in the text (Hosoda, Reference Hosoda2017). High-proficiency readers have more knowledge of academic vocabulary, while low-proficiency readers have less extensive vocabulary and less low-frequency vocabulary, which can make it difficult for them to generate inferences and comprehend the text (Silva & Otwinowska, Reference Silva and Otwinowska2019). In this way, when L2 reading skills are limited, readers are compelled to prioritize fundamental reading processes (i.e., word decoding and syntactic parsing) over inferential processing, allocating their cognitive resources accordingly (Horiba, Reference Horiba1996; Hosoda, Reference Hosoda2014). This, in turn, may hinder their ability to internalize the causal relationships presented in the texts and to construct comprehensive situation models of the texts. Moreover, low-proficiency readers may not use their prior knowledge because they do not have the necessary vocabulary available (e.g., Sidek & Rahim, Reference Sidek and Rahim2015; Silva & Otwinowska, Reference Silva and Otwinowska2019), further limiting their ability to understand and integrate the information presented. Given the number of students that usually acquire contents in their L2, understanding the possible consequences that reading and studying in an L2 might have for learning strategies and more specifically, whether learning processes are impaired when studying in L2 has remarkable importance.
Metacognitive strategies are conceived as a feeling-of-knowing state that serves a self-regulatory purpose whereby one can observe the ongoing processing, assess one’s comprehension and/or learning, detect errors, and decide what strategies need to be employed to enhance the process. According to the classical model proposed by Nelson and Narens (Reference Nelson, Narens and Bower1990), metacognitive processes include two general functions: monitoring and control. Metacognitive monitoring refers to the online supervision and assessment of the effectiveness of cognitive resources while metacognitive control refers to the management and regulation of cognitive resources.
More recent theories on metacognitive regulation propose a close association between monitoring accuracy and control effectiveness, as monitoring facilitates control. Self-regulated learning theories, in particular, suggest that individuals rely on continuous monitoring to determine the best course of action to achieve their learning goals (e.g., Dunlosky & Ariel, Reference Dunlosky, Ariel and Ross2011; Koriat & Goldsmith, Reference Koriat and Goldsmith1996; Metcalfe, Reference Metcalfe2009; Metcalfe & Finn, Reference Metcalfe and Finn2008; Pieger et al., Reference Pieger, Mengelkamp and Bannert2016; for a review see Panadero, Reference Panadero2017). For instance, identifying the difficult parts of a text correctly leads to appropriate effort regulation and strategy selection, which, consequently, results in greater comprehension and better memory (Follmer & Sperling, Reference Follmer and Sperling2018). Thus, from a learning perspective, metacognitive strategies and self-regulation have been linked to academic achievement (Pintrich & Zusho, Reference Pintrich, Zusho, Perry and Smart2007; Zimmerman, Reference Zimmerman2008; Zusho, Reference Zusho2017), as they are critical for comprehending and memorizing information (e.g., Collins et al., Reference Collins, Dickson, Simmons and Kameenui1996; Fukaya, Reference Fukaya2013; Huff & Nietfeld, Reference Huff and Nietfeld2009; Krebs & Roebers, Reference Krebs and Roebers2012; Thiede et al., Reference Thiede, Anderson and Herriault2003).
Thus, Deekens et al. (Reference Deekens, Greene and Lobczowski2018) investigated the relationship between the frequency of metacognitive monitoring and the utilization of surface and deep-level strategies. Surface-level strategies usually imply investing minimal time and effort to meet the requirements (e.g., rote learning or memorizing key concepts, Cano, Reference Cano2007), whereas deep-level strategies involve paying attention to the meaning, relating ideas, and integrating them with previous knowledge, to maximize understanding. Deep-level strategies are regarded as more effective strategies for producing longer-lasting learning (Deekens et al., Reference Deekens, Greene and Lobczowski2018; Lonka et al., Reference Lonka, Olkinuora and Mäkinen2004; Vermunt & Vermetten, Reference Vermunt and Vermetten2004). Deekens et al. (Reference Deekens, Greene and Lobczowski2018) found that students who enacted more frequent learning monitoring also engaged in deep strategies more frequently than low-monitoring students, and this resulted in better performance on academic evaluations. This pattern suggests that the combination of metacognitive monitoring and deep-level learning strategies is intrinsically linked to successful academic achievement.
The interaction between monitoring, strategies used, and learning, is significantly relevant when the to-be-learned materials vary in difficulty. Previous research has proven that judgments of learning (JOLs), a measure of the monitoring process, are sensitive to different cues and item-based features such as font type, concreteness, and relatedness (e.g., Magreehan et al., Reference Magreehan, Serra, Schwartz and Narciss2016; Matvey et al., Reference Matvey, Dunlosky and Schwartz2006; Undorf et al., Reference Undorf, Söllner and Bröder2018). According to the cue utilization approach (Koriat, Reference Koriat1997), learners base their JOLs on different sources of information, namely intrinsic, extrinsic, and mnemonic cues. Intrinsic cues refer to features of the material that indicate how easy or difficult it will be to learn (e.g., word frequency, associative strength, text cohesion). Extrinsic cues concern the study environment (e.g., the use of interactive imagery, time constraints, and repeated study trials). Mnemonic cues are internal states that provide information about how well an item has been learned (e.g., the subjective experience of processing an item fluently, past experiences in similar situations or beliefs).
The effects of some variables on JOLs have been extensively investigated at the word-unit level (e.g., Halamish, Reference Halamish2018; Hourihan et al., Reference Hourihan, Fraundorf and Benjamin2017; Hu et al., Reference Hu, Liu, Li and Luo2016; Li et al., Reference Li, Chen and Yang2021; Undorf et al., Reference Undorf, Zimdahl and Bernstein2017, Reference Undorf, Söllner and Bröder2018; Undorf & Bröder, Reference Undorf and Bröder2020; Undorf & Erdfelder, Reference Undorf and Erdfelder2015; Witherby & Tauber, Reference Witherby and Tauber2017) but also when learning larger chunks of information such as lists, paragraphs, and texts (Ackerman & Goldsmith, Reference Ackerman and Goldsmith2011; Ariel et al., Reference Ariel, Karpicke, Witherby and Tauber2020; Lefèvre & Lories, Reference Lefèvre and Lories2004; Nguyen & McDaniel, Reference Nguyen and McDaniel2016; Pieger et al., Reference Pieger, Mengelkamp and Bannert2016; see Prinz et al., Reference Prinz, Golke and Wittwer2020 for a meta-analysis). For example, text cohesion has been shown to influence JOL magnitude. Text cohesion refers to linguistic cues that help readers to make connections between the presented ideas. Examples of cohesion cues include the overlap of words and concepts between sentences and the presence of discourse markers such as because, therefore, and consequently (Crossley et al., Reference Crossley, Kyle and McNamara2016; Halliday & Hasan, Reference Halliday and Hasan1976). Poor cohesion texts impose higher demands on readers who would need to produce inferences to create a meaningful representation of the information in the text (Best et al., Reference Best, Rowe, Ozuru and McNamara2005). These texts are associated with poorer comprehension (Crossley et al., Reference Crossley, Yang and McNamara2014, Reference Crossley, Kyle and McNamara2016; Hall et al., Reference Hall, Maltby, Filik and Paterson2016).
Importantly, some studies show that participants can monitor text cohesion and adjust their JOLs accordingly (Carroll & Korukina, Reference Carroll and Korukina1999; Lefèvre & Lories, Reference Lefèvre and Lories2004; Rawson & Dunlosky, Reference Rawson and Dunlosky2002). For example, Lefèvre and Lories (Reference Lefèvre and Lories2004) manipulated cohesion by introducing or omitting a repetition of the antecedent to vary ambiguity in anaphoric processing. That is, they modified the complexity of resolving references to previously mentioned entities in the text. They observed that participants provided lower JOLs for low than for high-cohesion paragraphs. In addition, they found significant correlations between JOLs and comprehension scores. These results suggest that metacognitive monitoring is sensitive to the cohesion features of a text, as participants reported that they were poorly learning the low-cohesion texts which, indeed, were comprehended worse than high-cohesion texts. Similarly, Rawson and Dunlosky (Reference Rawson and Dunlosky2002) varied coherence by manipulating causal relatedness across sentence pairs and by altering the structure of sentences within paragraphs. They also found that both predictions and memory performance were significantly lower for low-coherence pairs than for moderate-to-high coherence pairs. Finally, in Carroll and Korukina’s (Reference Carroll and Korukina1999) experiment, sentence order was manipulated in narrative texts to create different coherence versions. They found a significant main effect of text coherence on both judgments and memory, as the ratings and the proportion of items that were immediately recalled were significantly greater for ordered texts than for nonordered texts.
Importantly, our study builds upon prior research by manipulating text cohesion within longer passages, contrasting with previous studies that primarily focused on cohesion within individual sentences. Overall, although JOLs have been proven to be sensitive to variations in the difficulty of the materials, people may in fact be less accurate in predicting their learning under circumstances with high cognitive load (Seufert, Reference Seufert2018; Wirth et al., Reference Wirth, Stebner, Trypke, Schuster and Leutner2020). Likewise, the language in which the learning is taking place might be a factor that mediates the cognitive and metacognitive resources that are devoted to the text (Reyes et al., Reference Reyes, Morales and Bajo2023).
1. Present study
In two experiments, we aimed to investigate whether studying in an L2 context has an impact on the self-regulation of learning and achievement. For this, we manipulated the cohesion of texts and examined to what extent unbalanced bilinguals monitored and controlled their learning both in L1 and L2 and whether they adjusted their judgments and strategies according to the characteristics of the materials.
Previous research on bilingualism provides reasons to hypothesize that learners might find L2 materials more difficult to process in comparison with the same content presented in L1 (Reyes et al., Reference Reyes, Morales and Bajo2023). At the same time, for the self-regulation of learning to occur, some processes must become automatic so that people can activate effective strategies (Winne, Reference Winne, Zimmerman and Schunk2011; Zimmerman & Kitsantas, Reference Zimmerman, Kitsantas, Elliot and Dweck2005). Hence, the nonautomatic processing and the potential extra cognitive demands imposed by L2 processing may have an impact on cognitive and metacognitive processes.
In a previous study, Reyes et al. (Reference Reyes, Morales and Bajo2023) reported that participants with intermediate L2 proficiency levels were able to correctly monitor their learning when studying in L2, adjusting their JOLs according to the difficulty of the to-be-studied material. That is, concrete words and semantically related word lists received higher JOLs, and were better remembered than abstract words and semantically unrelated word lists, respectively, both in L1 and L2. However, in this study monitoring and learning were assessed only for word lists and not for more complex materials such as expository texts, which align more with the materials used in academic and professional settings.
In the present study, we intended to extend previous results by studying the interaction among monitoring, strategies, and learning when the to-be-learned material was texts presented in participants’ L1 or L2. With this aim, we varied the difficulty of the text and assessed participants’ monitoring of the difficulty of the presented texts (i.e., JOL), their actual learning (i.e., open-ended questions), and the learning strategies used (i.e., self-report questionnaire). In Experiment 1, the tasks were presented to a sample of university students with an intermediate proficiency level of English–L2. In Experiment 2, higher and lower-proficiency groups were included.
Our main hypothesis was that studying texts in L2 may compromise the correct functioning of the processes implicated in self-regulated learning. On the one hand, participants might adjust their overall perception of learning according to the language context. If participants used language as a diagnostic cue (Koriat, Reference Koriat1997), we would expect them to provide higher JOLs in the L1 than in the L2 contexts, assessing learning in L1 as easier and more successful compared to learning in L2. Second, we expected to observe a less accurate assessment of other cues that influence the material’s difficulty in L2 compared to L1. Consequently, when participants studied in L2, they might not detect text cohesion as a useful cue for assessing their learning.
2. Experiment 1
2.1. Methods
2.2. Participants
We conducted a power analysis using G*Power (Faul et al., Reference Faul, Erdfelder, Lang and Buchner2007) to determine the sample size. We calculated it considering a mixed-factor analysis of variance (ANOVA) with language and cohesion as repeated measure variables, and order of the language block as a between-participant variable. We estimated 30 participants, assuming a small to moderate effect size (partial eta-squared of 0.07) to observe significant (α = 0.05) effects at 0.8 power. Due to an error in the text counterbalancing procedure, we had to recruit more participants to ensure a representative sample in each of the counterbalance lists. Participants had normal or corrected-to-normal vision and reported no neurological damage or other health problems. Participants gave informed consent before performing the experiment that was carried out following the Declaration of Helsinki (World Medical Association 2013). The protocol was approved by the institutional Ethical Committee of the University of Granada (857/CEIH/2019) and the Universidad Loyola Andalucía (201222 CE20371).
Sixty-eight psychology students from Universidad Loyola Andalucía (51.5%) and the University of Granada (48.5%) participated in this experiment. We removed from all analyses (1) a participant who did not vary the percentage given as a JOL in any of the texts and left it at the default value, and (2) two participants who gave answers in Spanish for both L1 and L2 block. We therefore had a total sample of 65 (18–28 years old, M = 19.92, SD = 1.76). Participants were tested remotely and individually in a two-session experiment and received course credit as compensation.
Participants were nonbalanced Spanish–English bilinguals although they started acquiring English as their L2 during childhood (M = 6.75, SD = 2.75). Subjective (Language Background Questionnaire, LEAP-Q; Marian, Blumenfeld, & Kaushanskaya, Reference Marian, Blumenfeld and Kaushanskaya2007) and objective (MELICET Adapted Test, Michigan English Language Institute College Entrance Test) language measures indicated that the sample had an intermediate proficiency level in English (M = 28.79). See Table 1 for descriptive statistics.
Table 1. Participants’ information for demographic and language measures.
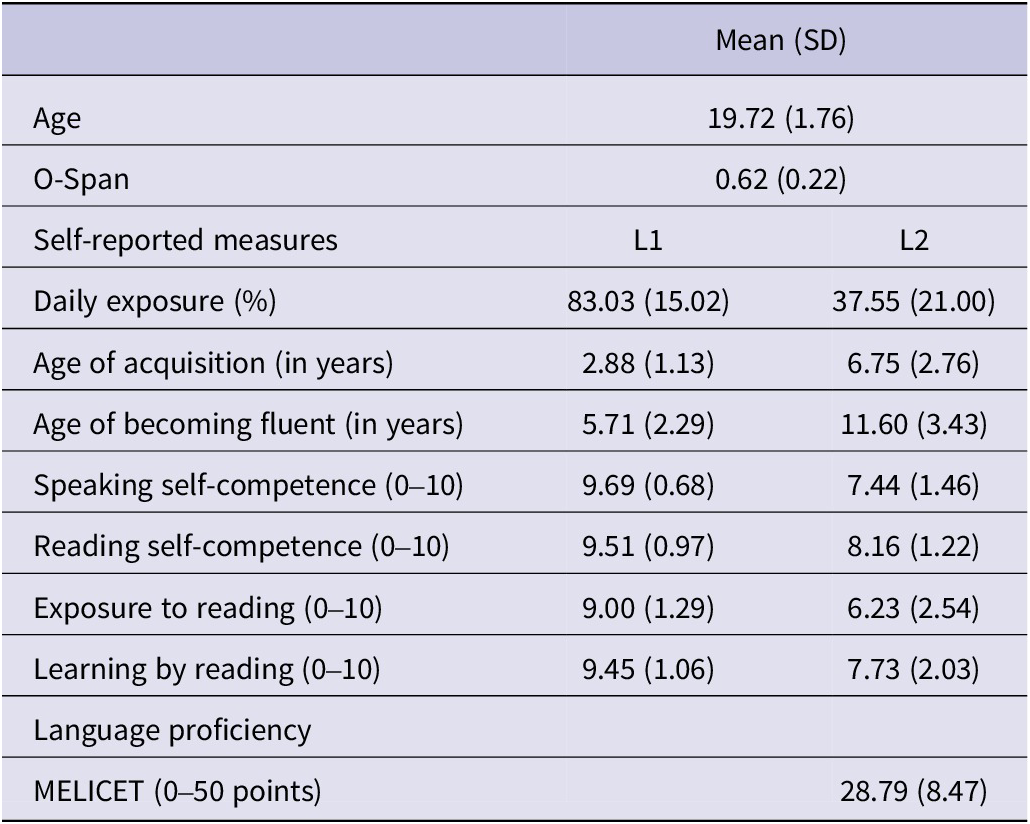
Note: T-tests for paired sample showed significant differences between languages in all the measures (all p-values < .001).
2.3. Materials and Procedure
The experiment consisted of two online sessions that lasted 120 and 90 minutes respectively. We programmed tasks, presented stimuli, and collected data with Gorilla Experiment Builder (Anwyl-Irvine et al., Reference Anwyl-Irvine, Massonnié, Flitton, Kirkham and Evershed2020). Participants accessed the experiment remotely and individually. To ensure that participants did not open other windows in the computer while doing the tasks, they were forced to full-screen presentations. Recent research supports the validity and precision of experiments run online (Anwyl-Irvine et al., Reference Anwyl-Irvine, Massonnié, Flitton, Kirkham and Evershed2020, Reference Anwyl-Irvine, Dalmaijer, Hodges and Evershed2021; Gagné & Franzen, Reference Gagné and Franzen2023).
The main task in both sessions was a learn-judge-remember task with a study phase and a recognition test. It simulated a learning task in a classroom environment in which students needed to learn and remember information from texts either in Spanish–L1 or in English–L2, depending on the session. Additionally, we administered different tasks and questionnaires at the end of each session. Participants completed the MELICET Adapted Test (Michigan English Language Institute College Entrance Test) as an objective L2 proficiency measure.
At the end of the second session they fulfilled a customized metacognitive questionnaire regarding the strategies used when studying the texts in both languages, a language background and sociodemographic questionnaire (LEAP-Q, Marian et al., Reference Marian, Blumenfeld and Kaushanskaya2007), and the Spanish version of the Operational Digit Span task (O-Span) to assess that all participants ranged within normal standardized values of working memory capacity (Turner & Engle, Reference Turner and Engle1989). We used a shortened version adapted from Oswald et al. (Reference Oswald, McAbee, Redick and Hambrick2014) in which participants were presented with a series of math problems followed by a to-be-remembered target letter. We calculated a working memory index by multiplying the mean proportion of successfully recalled letters and the mean proportion of correctly solved arithmetic equations (Conway et al., Reference Conway, Kane and Al2005).
In the learn-judge-remember task, participants were instructed to give a JOL and to answer some questions about the text they read. We manipulated the language (Spanish–L1 vs. English–L2) and the cohesion of the texts (high vs. low cohesion) as within-subjects factors. Language was a blocked variable and the assignment of L1 or L2 to the first or second session was counterbalanced across participants. Both high- and low-cohesion texts appeared along the study phase for each language block so that half of the texts within a block were of high cohesion and the other half was low cohesion.
In each session, participants were informed to read comprehensively 10 short texts for a later learning assessment test. Texts of high and low cohesion were presented in a pseudo-random order one at a time in the middle of the computer screen and remained for 3 minutes for self-reading. Immediately after the presentation of each text, participants gave a JOL to predict the likelihood of remembering the information they have just read on a 0–100 scale (0: not all likely, 100: very likely) by moving a handle slider to the desired number. This screen advanced when participants pressed ENTER.
After studying and judging each text, participants answered three open-ended questions as an objective measure of their learning. Previous research exploring the consequences of studying in L1 vs. L2 on memory found different effects depending on the type of test. For example, Vander Beken et al. (Reference Vander Beken, De Bruyne and Brysbaert2020) and Vander Beken and Brysbaert (Reference Vander Beken and Brysbaert2018) found that essay questions hindered performance in L2 presumably due to difficulties in writing production while no differences between L1 and L2 performance were found with open-ended questions and true/false recognition items. This suggests that language proficiency and background would make the writing process more complex and challenging in L2 than in L1. To avoid confounding effects with writing complexity, we discarded the essay and chose an open-ended format to better discriminate and prevent a possible ceiling effect that may appear with true/false recognition items. Questions covered a range of information from general ideas to examples or brief descriptions. Participants could respond with a single word, a noun phrase, or a concise sentence (e.g., “What type of teeth do meat-eating mammals have?”; see Appendix 1 for a detailed example). Open-ended questions were corrected automatically using a Python script (available at https://osf.io/dw4y7/?view_only=4eeb04437db14d69b2269a8d19392df5) that matched a rubric criterion developed a priori. This script has been checked against a manual revision leading to a higher reliability in the final score/mark. We gave 1 point for fully correct answers in the language required and 0 points for incomplete or incorrect answers. We provided a full score if the key concepts in the rubric were included in the answer, accepting grammatical and spelling mistakes in both languages. We calculated the mean proportion of correct recall for each participant and condition.
We selected 22 English texts related to academic topics, which we retrieved from different books and previous studies: two of them were used as examples and the rest were testing materials (see Appendix 2 for references and find them available at https://osf.io/dw4y7/?view_only=4eeb04437db14d69b2269a8d19392df5). All texts included intermediate vocabulary, taught in secondary school. In this manner, we ensured that our university students-participants were familiar with it, given that understanding academic vocabulary is crucial for comprehending academic texts (Silva & Otwinowska, Reference Silva and Otwinowska2019). A Spanish native speaker translated texts into Spanish and manipulated their cohesion, both in English and Spanish texts, following norms from previous studies (see Table 2). Efforts were made to make the minimal changes possible and to ensure that the texts were comparable in terms of their difficulty (e.g., word frequency). Two judges, both native Spanish speakers, double-checked the translations and the cohesion manipulation. This resulted in four different versions for every text, one per language-cohesion condition: L1 high cohesion and L1 low cohesion, and L2 high cohesion and L2 low cohesion.
Table 2. Norms for manipulating text cohesion.
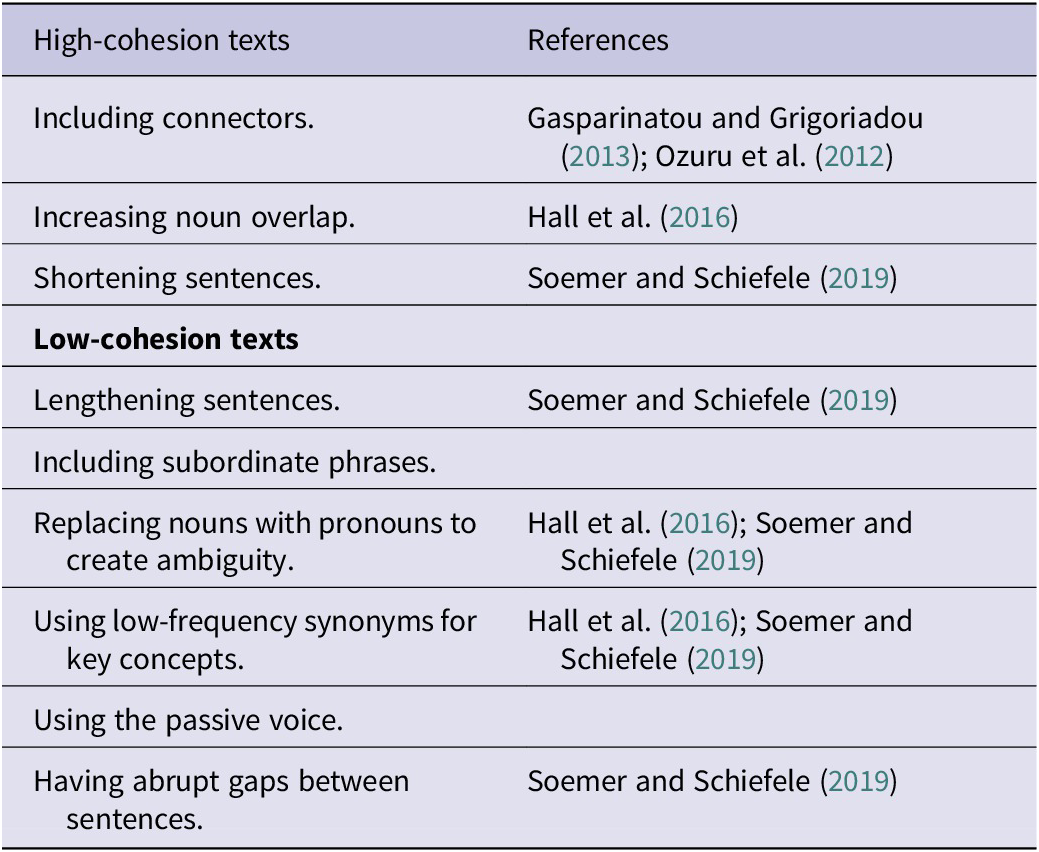
We created four counterbalanced text lists with each list containing five texts per language-cohesion condition. Therefore, each participant was presented with 20 different texts: 10 in the L1 session and 10 in the L2 session, of which five were low- and five high-cohesion texts. Repeated measures ANOVA (cohesion and language) showed that the texts were matched in length (number of words) between conditions, as no main effects or interaction were significant [all p-values > .05; L1: high (M = 142.6, SD = 22.8) and low cohesion (M = 141.0, SD = 28.4); L2: high (M = 141.0, SD = 26.4) and low cohesion (M = 140.3, SE = 29.6)]. See Appendix 1 for an example of a high- and low-cohesion version of a text in L2 and its open-ended questions.
For the learn-judge-remember task, we analyzed JOL responses in the study phase and the proportion of correct answers for the open-ended questions, grouped by condition (language and text cohesion).
In each language block, after the learn-judge-remember task, participants answered a customized metacognitive self-report questionnaire. We combined items from two different inventories into a single set of 8 questions and translated into Spanish by a Spanish native speaker, with subsequent double-checking by a second and third judge to ensure accuracy and consistency. We selected items from the NASA Task Load Index (NASA-TLX; Hart & Staveland, Reference Hart, Staveland, Hancock and Meshkati1988) and the Motivated Strategies for Learning Questionnaire (MSLQ; Pintrich et al., Reference Pintrich, Smith, García and McKeachie1991). Both are validated questionnaires designed to be modular and can be used to fit the needs of the researcher. Although we could not test the reliability of the Spanish translation of this ad hoc questionnaire (as it was beyond the purpose of our study) it could serve to understand the metacognitive processes engaged in our participants’ performance. Participants rated themselves on a seven-point Likert scale from “not at all true of me” to “very true of me.”
Thus, we assessed cognitive and metacognitive learning strategies, effort regulation, mental demand, and self-perceived performance. Originally, a few items were intended for a general learning context, so we modified some expressions for the specific task. We also translated the items into Spanish since we administered the questionnaire in the language the session was taking place. The item referring to metacognitive self-regulation was reversed and thus we inverted their punctuation. For this questionnaire, we compared the score for items in L1 and L2. See Appendix 3 to check the set of questions included into the questionnaire.
3. Results
We performed a 2 × 2 × 2 (language × text cohesion × block order) mixed-factor ANOVAs for JOLs in the study phase and for learning assessment test. We included language order in the analyses since previous research (Reyes et al., Reference Reyes, Morales and Bajo2023) suggests that it might influence monitoring and memory performance. Language (L1 vs. L2) and text cohesion (high vs. low cohesion) were within-subject factors and block order (L1-first vs. L2-first) was a between-subject factor. For all analyses, the alpha level was set to 0.05 and we corrected by Bonferroni for multiple comparisons. All effect sizes are reported in terms of partial-eta-squared (ηp2) for ANOVAs and Cohen’s d for t-tests.
Study phase (JOLs). To evaluate the effect of language and text cohesion on the magnitude of JOLs, we computed the mean across participants’ JOLs for each condition (see Table 3 for partial means).
Table 3. Mean (and standard deviations) for JOL scores (1–100 scale) and learning performance (proportion of correct responses) across language, cohesion, and block order conditions.

None of the interactions were significant (all p-values > .05). We did find significant main effects of language, F(1, 63) = 20.13, p < .001, η p2 = .24, and cohesion, F(1, 63) = 15.35, p <.001, η p2 = .20. Texts in L1 (M = 68.8, SE = 1.58) received higher JOLs than texts in L2 (M = 61.6, SE = 1.95). Similarly, high-cohesion texts received higher JOLs (M = 66.5, SE = 1.60) than low-cohesion texts (M = 63.8, SE = 1.64). The main effect of block order was not significant, F(1, 63) = 0.06, p =.808, η p2 = .001. Texts received comparable JOLs regardless of the language order (L1 first: M = 64.8, SE = 2.22; L2 first: M = 65.5, SE = 2.26).
Learning assessment test (accuracy). To evaluate learning performance, that is, how much participants remembered from the texts, we computed the proportion of correct responses in the open-ended questions across participants (see Table 3).
None of the interactions were significant (all p-values > .05). Yet, the analysis showed a significant main effect of language, F(1, 63) = 6.41, p = .014, η p2 = .10, and cohesion, F(1, 63) = 24.66, p < .001, η p2 = .28. That is, participants remembered information from texts in L1 (M = 0.71, SE = 0.02) better than from texts in L2 (M = 0.65, SE = 0.02); and from high-cohesion texts (M = 0.71, SE = 0.02) better than from low-cohesion texts (M = 0.65, SE = 0.02). The main effect of block order did not reach significance, F(1, 63) = 1.62, p = .21, η p2 = .03. Participants’ accuracy in the learning assessment tests did not depend on which language block they performed on the first or second place (L1 first: M = 0.65, SE = 0.03; L2 first: M = 0.70, SE = 0.03).
Language metamemory accuracy—resolution. To examine participants’ metamemory accuracy—resolution—across languages (i.e., to check whether participants’ JOLs discriminate between the information recall of one text relative to another), we used a Goodman–Kruskal (GK) gamma correlation (Nelson, Reference Nelson1984) and a language-accuracy index correlation.
GK gamma correlation is a nonparametric measure of the association between JOLs and subsequent recall. We calculated one gamma correlation for each participant in each of the language conditions. We then ran a t-test to examine whether the GK Gamma correlations differed across languages. No significant effects were found, t(64) = −0.76, p = 0.45, d = −0.09.
We also performed a language-accuracy index correlation as an additional measure of metamemory accuracy, which allows us to further explore participant’s overall resolution in L1 and L2. To do so, we first calculated a language index for JOLs and for learning accuracy, by subtracting the mean scores in L2 from the mean scores of L1 of JOLs and learning accuracy respectively, and then performed correlation analyses of the two indexes. Interestingly, the JOLs index correlated with the accuracy index (r = 0.6), suggesting that participants’ predictions during the study phase about what they would remember later agreed with what they actually recalled in the learning assessment test.
Customized metacognitive self-report questionnaire. We analyzed the questionnaire regarding the participants’ learning strategies in the study phase (see Table 1S in Supplementary Materials for partial means in the questionnaire). We conducted t-tests to compare the frequency of each strategy in L1 and L2. Overall, we found that participants employed some strategies more frequently in L1 than in L2, namely, elaboration (“When reading the texts, I tried to relate the material to what I already knew”), t(64) = 2.17, p = .033, d = 0.27, metacognitive self-regulation (“When studying the materials in the texts, I often missed important points because I was thinking of other things”), t(64) = 2.71, p = .008, d = 0.34, and effort regulation (“I worked hard to do well even if I didn’t like what I was studying in the texts”), t(64) = 2.06, p = .044, d = 0.26. No differences were found in terms of critical thinking strategy, t(64) = −0.60, p = .55, d = −0.07, or rehearsal, t(64) = −0.59, p = .56, d = −0.07. No strategy was more frequently used in L2 than in L1 either. As expected, and consistent with JOLs, participants reported that they experienced significantly higher mental demand in L2 than in L1, t(64) = −8.81, p < .001, d = −1.09. Similarly, participants felt their performance had been better in L1 than in L2, t(64) = 2.17, p = .034, d = 0.27.
3.1. Discussion
In this first experiment, we focused on investigating two potential outcomes. Initially, we wanted to observe if a cue such as text cohesion yielded variations in JOLs and learning. We also sought to ascertain whether these differences depended on the language environment (L1 or L2) within which the task was executed. Additionally, we aimed to evaluate whether that linguistic context exerted an influence on the global perception of task complexity.
Regarding text cohesion, we found a cohesion effect both in JOLs and in the learning assessment test. Participants predicted better performance for high-cohesion texts and, correspondingly, learning rates were higher for them compared to those with low cohesion. Thus, we replicated what had previously been reported in the monolingual text monitoring and comprehension literature (Carroll & Korukina, Reference Carroll and Korukina1999; Crossley et al., Reference Crossley, Yang and McNamara2014, Reference Crossley, Kyle and McNamara2016; Hall et al., Reference Hall, Maltby, Filik and Paterson2016; Lefèvre & Lories, Reference Lefèvre and Lories2004; Rawson & Dunlosky, Reference Rawson and Dunlosky2002). More importantly, we expanded these findings to a bilingual sample, suggesting that the processes operating in L2 are similar to those in L1 concerning text monitoring and comprehension.
As to the language effect, participants judged L1 materials as easier to learn—giving higher JOLs—than materials in L2. As shown by the learning assessment test, participants encountered significantly more difficulty in remembering information from texts in L2. Previous research exploring the consequences of studying in L1 versus L2 on memory observed different effects depending on the test type used. For example, Vander Beken et al. (Reference Vander Beken, De Bruyne and Brysbaert2020) and Vander Beken and Brysbaert (Reference Vander Beken and Brysbaert2018) found that essay questions hindered performance in L2 presumably due to difficulties in writing production, while no differences between L1 and L2 performance were found with open-ended questions and true/false recognition items. Nevertheless, our participants did show an L2 recall cost despite the fact that we chose an open-ended format to avoid confounding effects with writing complexity and that our rubric accepted grammatical, syntactic, or orthographic errors (note that language mistakes are not punished—as long as they do not obscure meaning—in international reading comprehension assessments like the Programme for International Student Assessment, PISA). Hence other factors related to the type of processing or strategies used during L1 and L2 may have produced differences in L1 and L2 memory performance.
In sum, the results of Experiment 1 indicate that participants with an intermediate level of English–L2 were able to use intrinsic cues such as text cohesion and language simultaneously, to monitor their learning both in L1 and L2. However, in Experiment 1 we did not manipulate participants’ L2 proficiency, and it was plausible that differences in language proficiency and exposure could have influenced the monitoring behavior of the bilingual individuals. To explore this possibility, we conducted a second experiment to investigate the effects of L2 proficiency levels on monitoring and control processes of self-regulated learning. For this, we intentionally recruited participants with higher and lower L2 proficiency.
4. Experiment 2
In the second experiment, our aim was to investigate the influence of L2 proficiency on the dynamic relationship between monitoring and control during text-based learning. To achieve this, we recruited a sample that included both lower and higher English–L2 proficiency levels. We hypothesized that individuals in the lower-proficiency group might encounter challenges in effectively monitoring their learning due to allocating a greater share of cognitive resources to language control compared to their higher-proficiency counterparts (Francis & Gutiérrez, Reference Francis and Gutiérrez2012; Sandoval et al., Reference Sandoval, Gollan, Ferreira and Salmon2010). As a result, the cohesion effect in JOLs, which manifests as higher values with well-cohesive texts, could potentially diminish within the lower-proficiency group. This attenuation might arise from the substantial cognitive load imposed by learning in a demanding L2 context, potentially overshadowing the sensitivity to nuanced differences in text cohesion (see Magreehan et al. Reference Magreehan, Serra, Schwartz and Narciss2016, who did not find the font type effect on JOLs when other cues were available). In essence, we posit that texts in L2 may present inherent challenges for individuals with lower proficiency, regardless of their cohesion status.
In addition, we introduced two further modifications: (1) as block order was not significant in Experiment 1, we eliminated this variable from the procedure, and high- and low-cohesion texts in L1 and L2 appeared along the study phase in a pseudorandom order; (2) participants attended an in-person session at the laboratory for the second part of the experiment. The remaining conditions were held constant, mirroring the setup employed in Experiment 1.
4.1. Methods
4.1.1. Participants
Instructions for recruitment indicated that participants needed to have some English knowledge, although we did not specify the threshold for participation. Fifty-seven psychology students from the University of Granada (63.17%) and Universidad Loyola Andalucía (36.84%) enrolled in the experiment. Participants were tested individually in two sessions (a remote and an in-person session) and received course credit as compensation. We divided our sample into two independent groups by scores in MELICET. Based on previous studies (Kaan et al., Reference Kaan, Kheder, Kreidler, Tomíc and Valdés Kroff2020; López-Rojas et al., Reference López-Rojas, Rossi, Marful and Bajo2022), we established scores of 30 or above as the criteria to be included in the higher-proficiency group (upper-intermediate level, n = 23, 18–23 years old, M =19.05, SD = 1.36), and scores of 25 or below were classified into the lower-proficiency group (pre-intermediate level, n = 24, 18–49 years old, M =21, SD = 6.81). Participants with in-between scores were not included in the analyses. In addition, we removed two participants who did not vary the percentage given as a JOL in any of the texts and left it at the default value, so this resulted in a total sample of 49. No differences were found in the O-Span index (following the same calculation described in Experiment 1) between groups t(45) = 1.49, p = .14, d = 0.43 (higher-proficiency group: M = 0.60, SE = 0.04; lower-proficiency group: M = 0.51, SE = 0.05) suggesting that any possible difference between groups were not due to differences in working memory capacity. Comparisons between languages for all self-reported linguistic measures within groups showed that participants in both groups were unbalanced and significantly more fluent in L1 than in L2. All p-values were below .05. See Table 4 for further details.
Table 4. Participants’ information for demographic and language measures divided by proficiency group (higher- and lower-proficiency).
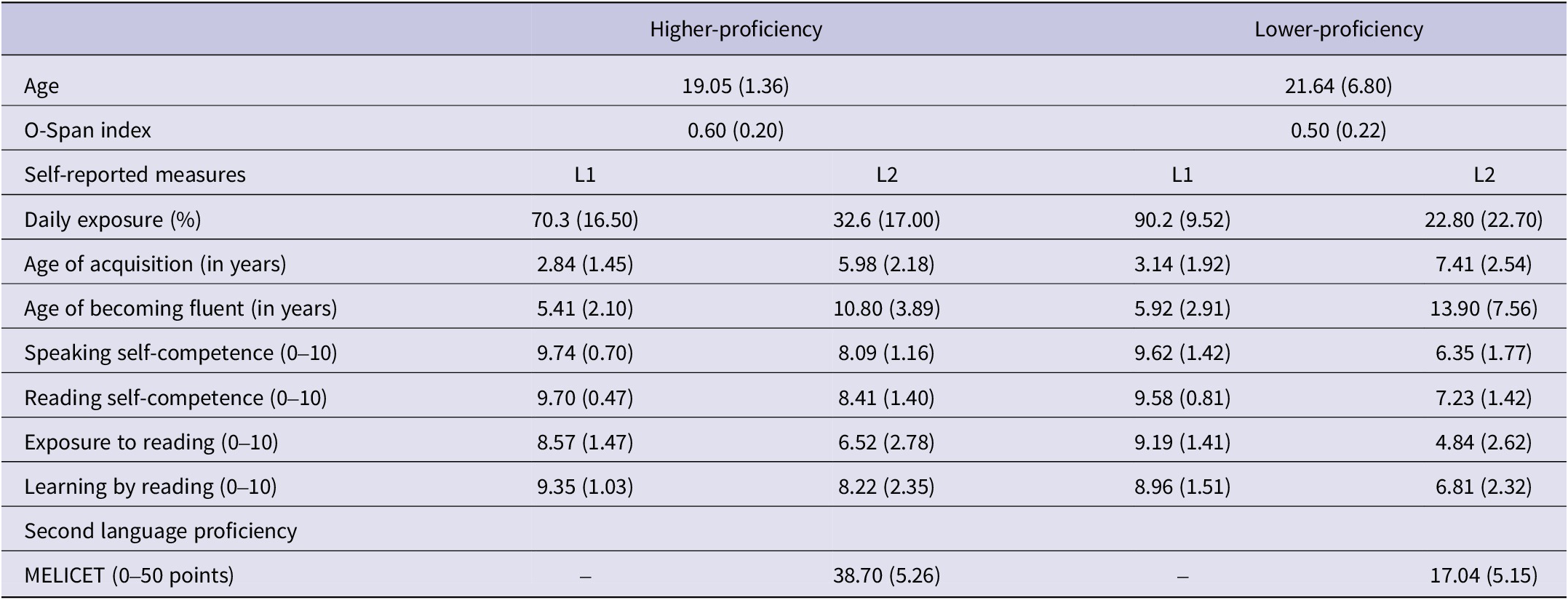
Note: Higher-proficiency group (n = 23) scored 30 or more in MELICET (M = 38.7, SE = 1.1) while lower-proficiency group (n = 24) scored 25 or less (M = 17.7, SE = 0.85) and significant differences between groups in this measure was found t(45) = 15.2, p < 0.01, d = 4.44. (*) Significant differences between groups (p < .05).
4.1.2. Materials and procedure
The experiment consisted of two sessions for which participants received course credit as compensation. We programmed and administered the tasks with the same experiment builder as in Experiment 1 (Anwyl-Irvine et al., Reference Anwyl-Irvine, Massonnié, Flitton, Kirkham and Evershed2020). The first session lasted 30 minutes and was administered remotely. Participants completed some questionnaires, the LEAP-Q (Marian et al., Reference Marian, Blumenfeld and Kaushanskaya2007) and an objective L2 proficiency measure (MELICET). Then participants came to the laboratory to complete the second session in person, which lasted 120 minutes. The procedure was similar to that of Experiment 1, with a learn-judge-remember task, a customized metacognitive questionnaire regarding the strategies used when studying the texts, and the standard Operational Digit Span task (O-Span) to assess that all participants ranged within normal standardized values and that groups did not differ in working memory (Oswald et al., Reference Oswald, McAbee, Redick and Hambrick2014).
4.2. Results
We report a 2 × 2 × 2 (language × text cohesion × proficiency group) mixed-factor ANOVAs for JOLs in the study phase and for accuracy in the learning assessment test. Language (Spanish–L1 vs. English–L2) and text cohesion (high vs. low) were within-subject factors, and the proficiency group (higher vs. lower) was a between-subject factor. As in Experiment 1, the alpha level was set to 0.05 and we corrected it by Bonferroni for multiple comparisons, in all analyses. All effect sizes are reported in terms of partial-eta-squared (η p2) for ANOVAs and Cohen’s d for t-tests. Table 5 shows partial means for JOLs and accuracy in the learning assessment test.
Table 5. Mean (and standard deviations) for JOLs scores (1–100 scale) and learning performance (proportion of correct responses) across language, cohesion, and proficiency group conditions.

Study phase (JOLs). Analysis on the JOLs showed a significant interaction between language and proficiency group, F(1, 45) = 23.48, p < .001, η p2 = .34. Post-hoc comparisons showed that participants in the higher-proficiency group did not differ in their JOLs between languages, t(45) = −0.77, p = 1.00 (L1: M = 62.0, SE = 3.08; L2: M = 61.5, SE = 3.39) whereas participants in the lower-proficiency group gave significantly higher JOLs for texts in L1 (M = 65.3, SE = 3.02) than for texts in L2 (M = 49.4, SE = 3.32), t(45) = 7.13, p < .001. Overall, this trend suggests that proficiency was modulating JOL values when studying in L2.
The interaction between language and cohesion was also significant, F(1, 45) = 66.4, p < .001, η p2 = .60. Post-hoc comparisons showed that JOLs for low-cohesion texts differed between languages, t(45) = 8.31, p < .001, while no significant difference between languages was found for JOLs in high-cohesion texts, t(45) = 0.17, p = 1.00. That is, participants gave higher JOLs in L1 for low-cohesion texts (M = 66.1, SE = 2.2) than in L2 (M = 50.1, SE = 2.63). This difference was not significant for high-cohesion texts, that received comparable JOLs in both languages (L1: M = 61.1, SE = 2.26; L2: M = 60.8, SE = 2.36). This suggests that the cohesion effect is significantly more salient in L2 and texts with low cohesion in L2 were the most difficult condition among all four.
The main effects of language, F(1, 45) = 26.41, p < .001, η p2 = .37, and cohesion, F(1, 45) = 8.47, p = .006, η p2 = .16 were significant. As expected, texts in L1 received higher JOLs (M = 63.6, SE = 2.16) than texts in L2 (M = 55.4, SE = 2.37). Similarly, texts with high cohesion received higher JOLs (M = 61.0, SE = 2.13) than texts with low cohesion (M = 58.1, SE = 2.22). The main effect of the group did not reach significance, F(1, 45) = 1.07, p = .31, η p2 = .02. Overall, the higher-proficiency group (M = 61.7, SE = 3.03) gave similar JOLs values to the lower-proficiency group (M = 57.3, SE = 2.97).
Learning assessment test (accuracy). As for accuracy, the analysis showed a similar pattern. We found a significant interaction between language and proficiency group, F(1, 45) = 24.65, p < .001, η p2 = .35. Post-hoc comparisons showed that the higher-proficiency group had comparable accuracy in both languages, t(45) = −0.14, p = 1.00, so their learning was similar in L1 (M = 0.66, SE = 0.03) and L2 (M = 0.67, SE = 0.04). In contrast, accuracy for participants with lower proficiency did differ between languages, t(45) = 6.96, p < .001, and they achieved significantly better learning for texts in L1 (M = 0.63, SE = 0.03) than for texts in L2 (M = 0.35, SE = 0.04). It seems that proficiency plays a role when learning in L2 and lower-proficiency level might hinder learning.
The interaction between language and cohesion was marginally significant, F(1, 45) = 2.88, p = .096, η p2 = .06. Post hoc comparisons showed that accuracy for texts in L2 differed between cohesion condition, t(45) = 4.50, p < .001. Participants were more accurate in high-cohesion texts (M = 0.56, SE = 0.03) than in low-cohesion texts (M = 0.45, SE = 0.03). Nevertheless, no significant difference between cohesion conditions was found for texts in L1, t(45) = 2.36, p = .14 (high cohesion: M = 0.67, SE = 0.02; low cohesion: M = 0.61, SE = 0.03). Again, it seems that the cohesion effect is significantly more salient in L2 not only in JOLs but also in learning.
We found three significant main effects: language, F(1, 45) = 22.77, p < .001, η p2 = .34, with texts in L1 (M = 0.64, SE = 0.02) receiving higher scores in the learning assessment test than texts in L2 (M = 0.51, SE = 0.03); cohesion, F(1, 45) = 18.82, p < .001, η p2 = .30, with high-cohesion texts receiving higher scores (M = 0.62, SE = 0.02) than low-cohesion texts (M = 0.53, SE = 0.02); and proficiency group, F(1, 45) = 17.9, p < .001, η p2 = .29, with participants in the higher-proficiency group (M = 0.66, SE = 0.03) achieving overall better learning than participants in the lower-proficiency group (M = 0.49, SE = 0.03).
Language metamemory accuracy—resolution. We run a mixed-factor ANOVA and we found no significant main effect of Goodman–Kruskal Gamma correlations F(1, 44) = 2.20, p = .15, η p2 = .05, nor proficiency group, F(1, 44) = 0.00, p = .96, η p2 = .00. However, the interaction between both factors was significant, F(1, 44) = 4.93, p = .03, η p2 = .10. Post-hoc comparisons showed a marginal tendency for lower-proficiency group to have better resolution in L2 (M = 0.32, SD = 0.1) than in L1 (M = −0.00, SD = 0.09), t(44) = −2.62, p = 0.07, while no difference was found for the high-proficiency group (L1: M = 0.18, SD = 0.09; L2: M = 0.12, SD = 0.1, t(44) = 0.52, p = 1.00).
We also ran JOL and accuracy language index correlations with the two proficiency groups independently, and we observed that a significant positive correlation appeared for participants with higher proficiency (r = 0.42, p = .05) while participants with lower proficiency showed a significant negative correlation between JOL and accuracy index (r = −0.43, p = .04).
4.3. Additional analyses collapsing across both experiments
To further explore proficiency effects, we performed statistical analyses by collapsing across both experiments. Collapsing data from the two experiments allowed us to examine the effect of L2 proficiency as a continuous variable, increasing sample size and L2 variability. Note, however, that the two experiments differed in the form of presenting L1 and L2 (blocked or mixed) and not only in the proficiency level of our participants. Therefore, we included Experiment as a variable for these analyses. We used linear mixed-effect models, as implemented in the lme4 package (version 1.1–27.1; Bates et al., Reference Bates, Mächler, Bolker and Walker2014) in R using participants and items (texts) as crossed random effects. We selected JOLs and accuracy as the dependent variables of each model. We included as fixed effects language (L1, L2), cohesion condition (high, low), L2 proficiency (scores in MELICET), experiment (Exp1: language blocking, Exp 2: language mixing), and the interactions between them. We transformed the continuous variables (L2 proficiency) to normalize the distribution by scaling the scores. We selected sum contrast for language (L1 = −1; L2 = 1), cohesion (high = −1; low = 1) and experiment (Exp1 = –1; Exp2 = 1). We fitted the maximal model first (Barr et al., 2013), and in case of nonconvergence or singularities, we simplified it following recommendations outlined in Bates et al. (2021). We considered significant any fixed effect with a t-statistic higher than 2.
In JOLs, the results showed an interaction between language and cohesion. Low cohesion texts in L2 (M = 54.5; SE = 2.11) received significantly lower JOLs than low cohesion texts in L1 (M = 67.2; SE = 1.95), t(50.9)= 12.72, p < .0001, while no differences were found between languages for texts with high cohesion (L1: M = 66; SE = 1.95; L2: M = 62; SE = 2.10), t(48.8)= 4.03, p = .11. The interaction between language and L2 proficiency was also significant. The higher the L2 proficiency, the higher the JOL values stemming from higher JOLs in L2 (see Figure 1). In other words, the difference in JOLs between L1 and L2 was significant for people with low, t(71.2)= 25.86, p < .0001, and medium L2 proficiency, t(45.7)= 11.29, p < .0001, whereas people with higher L2 proficiency showed no differences between languages, t(73.5)= −3.28, p = .29.
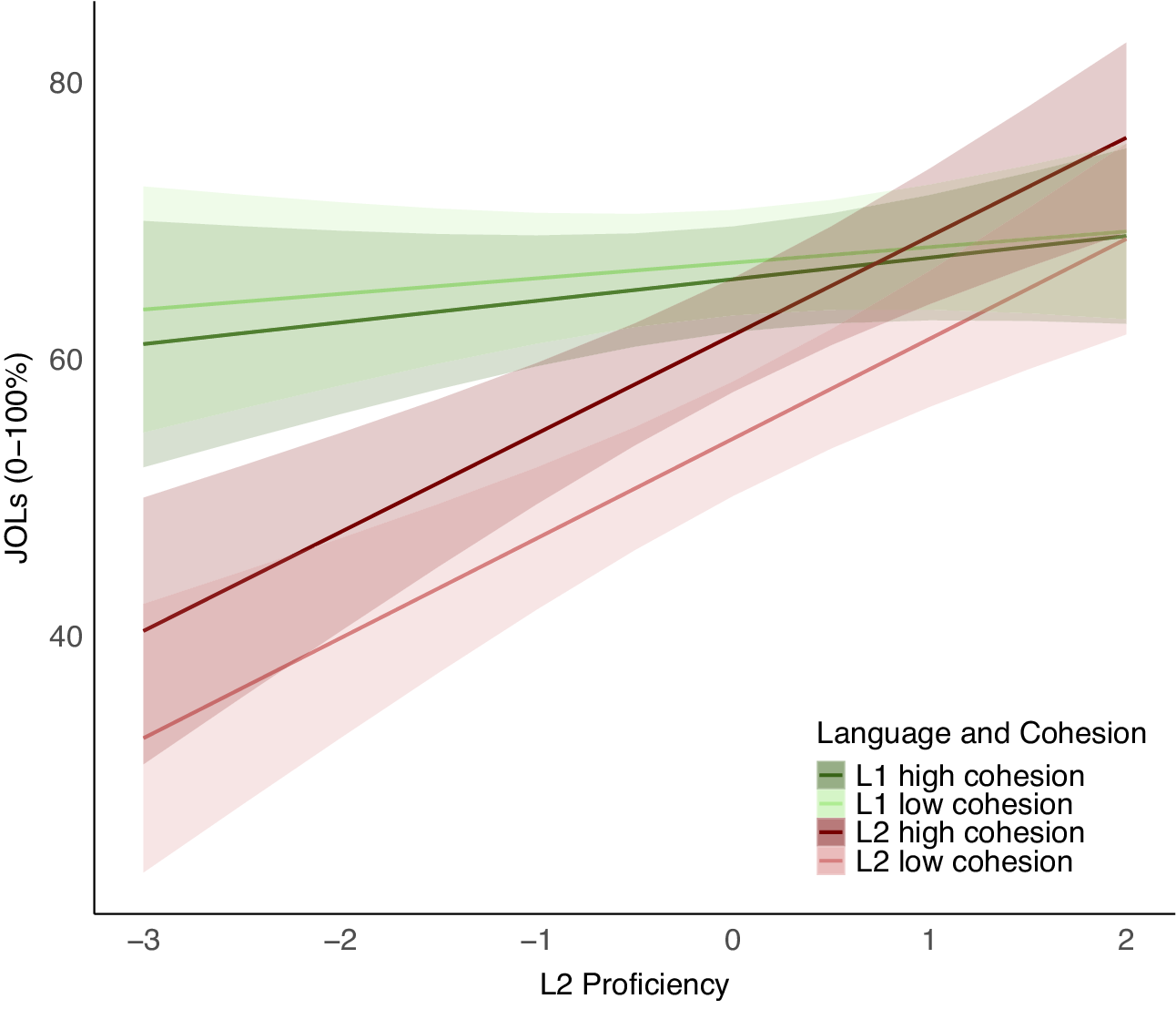
Figure 1. Reported JOLs along L2 proficiency divided by language and cohesion, with data collapsed across the two experiments. Note that higher L2 proficiency leads to higher JOL values in L2. There are significant differences between L1 and L2 for participants with low and medium L2 proficiency, but no language differences for those with high L2 proficiency.
The triple interaction between language, cohesion, and experiment was also significant (see Figure 2). In Experiment 1, where language was blocked, the difference between languages was significant in both cohesion conditions (high L1: M = 68.7; SE = 2.25, high L2: M = −62.7; SE = 2.47, t(69)= 5.99, p = .03; low L1: M = 65.9; SE = 2.26; low L2 M = 60.2; SE = 2.47, t(69.2)= 5.76, p = .03). However, in Experiment 2, where language was mixed in the study phase, the difference in JOLs between languages (L1 vs. L2) was only significant for low cohesion texts, where L1 received significantly higher JOLs values (M = 68.5; SE = 2.71) than L2 (M = 48.9; SE = 2.75), t(100)= 19.67, p < .0001, with no significant differences in high cohesion texts (L1: M = 63.4; SE = 2.71; L2: M = 61.3; SE = 3.39, t(53.6)= 2.08, p = .58). Hence, analysis of the collapsed JOLs data did not change the pattern of results observed when the analyses were performed separately for each experiment.
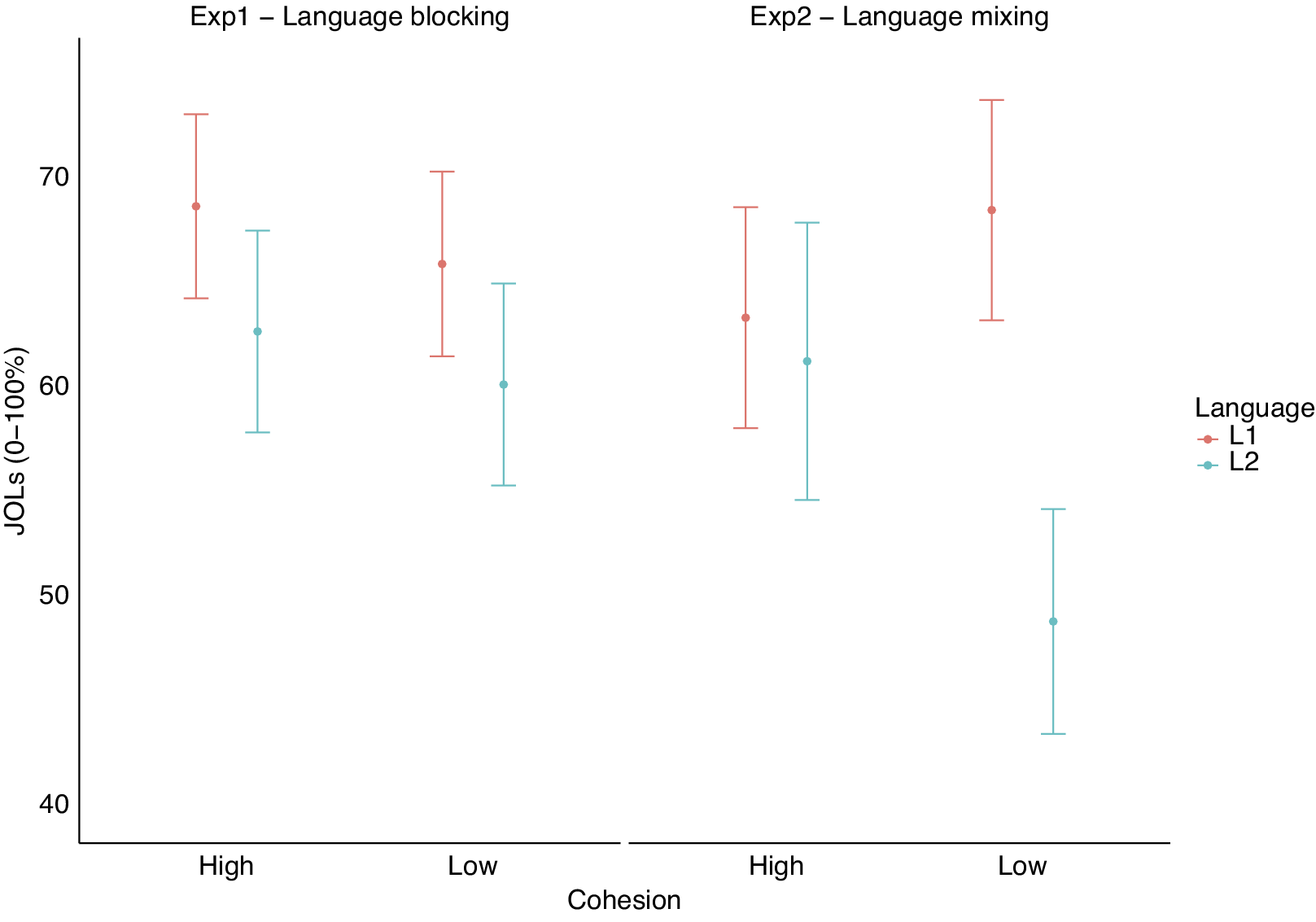
Figure 2. Reported JOLs divided by language, cohesion, and L2 proficiency by experiment. Note: Significant triple interaction between language, cohesion, and experiment. In Experiment 1, L1 texts received significantly higher JOLs than L2 texts, regardless of cohesion conditions. In Experiment 2, this difference only appeared for low-cohesion texts, with no significant language differences for high-cohesion texts.
For the learning assessment test, the results showed a significant interaction between language and L2 proficiency. Again, as shown in Figure 3, the difference in accuracy between L1 and L2 was significant for participants with low, (L1: M = 0.49; SE = 0.29; L2: M = −0.99; SE = 0.28, t(Inf)= 1.48, p < .0001), and medium L2 proficiency, (L1: M = 0.80; SE = 0.17; L2: M = 0.21; SE = 0.17, t(Inf)= 0.60, p = .01), whereas people with higher L2 proficiency showed no differences between languages (L1: M = 1.11; SE = 0.29; L2: M = 1.41; SE = 0.23, t(Inf)= −0.29, p = .31). See supplementary materials for a summary of the JOLs mixed-effect model and the accuracy mixed-effect model.
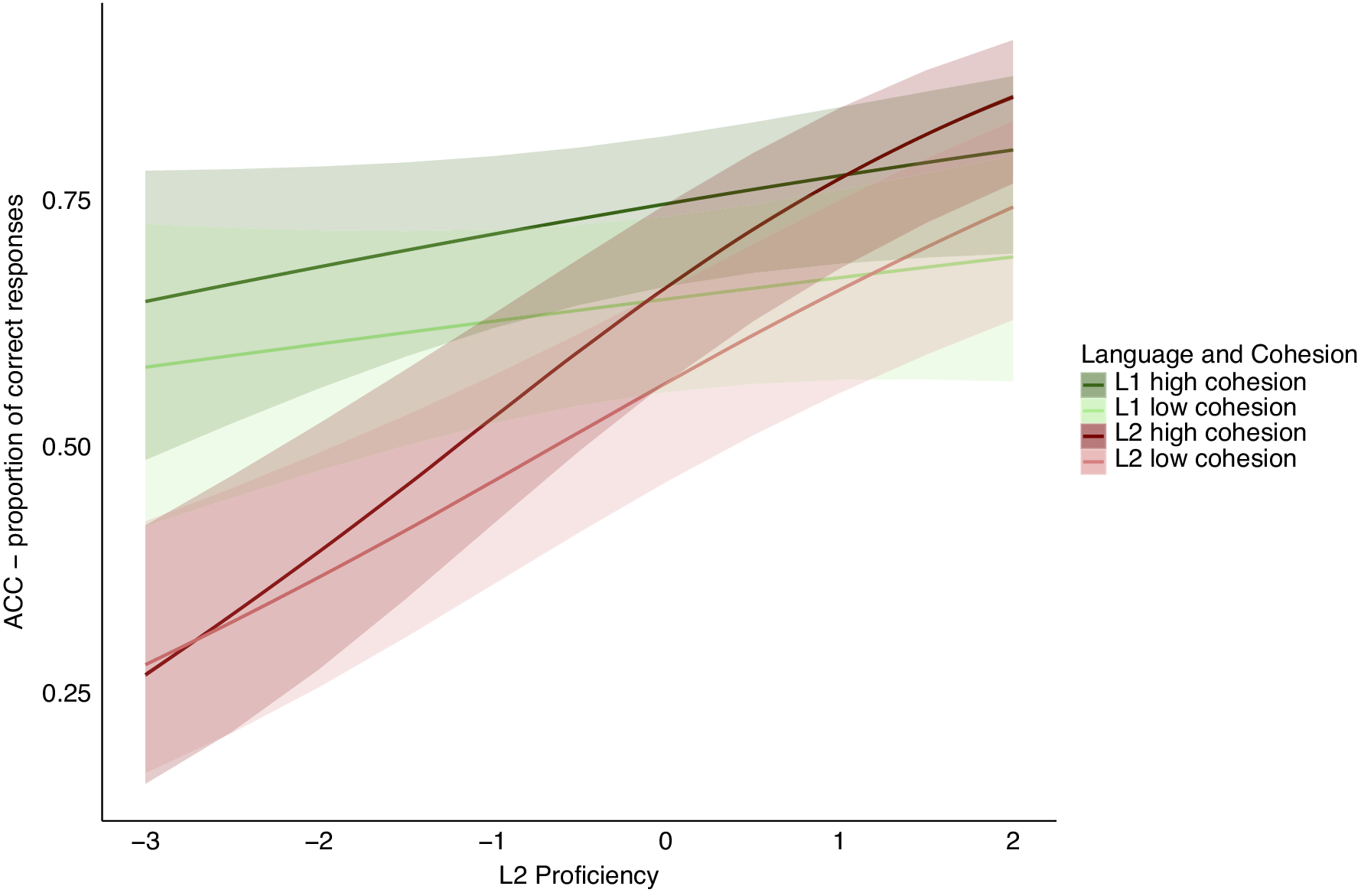
Figure 3. The proportion of correct responses along L2 proficiency divided by language and cohesion, with data, collapsed across the two experiments. Note that significant interaction between language and L2 proficiency in the learning assessment test. The accuracy difference between L1 and L2 was significant for participants with low and medium L2 proficiency, while no differences were observed for those with high L2 proficiency.
Customized metacognitive self-report questionnaire. As in Experiment 1, we also explored whether the strategies differed between proficiency groups (see Table 2S in Supplementary Materials for partial means in the questionnaire). We run repeated measures ANOVAs for each of the items in the questionnaire with language as a within-subject factor and proficiency group as a between-subject factor.
We found a significant interaction between the elaboration strategy and proficiency group, F(1, 45) = 4.37, p = .04, η p2 = .09. However, post-hoc analysis showed no significant difference in any of the comparisons (all p > .05).
We also found a significant difference in the use of metacognitive self-regulation between languages F(1, 45) = 4.12, p = .05, η p2 = .08, with such strategy being more prevalent in L2 (M = 4.11, SD = 0.27) than in L1 (M = 3.73, SD = 0.25) regardless of the proficiency group.
Regarding the use of effort regulation, we found a marginal significant main effect of proficiency group, F(1, 45) = 3.85, p = .06, η p2 = .08, that was mediated by a significant interaction, F(1, 45) = 11.41, p = .002, η p2 = .20. Post-hoc comparison showed a significant difference between proficiency groups in the use of effort regulation in L2 (high-proficiency group: M = 2.43, SE = 0.39; low-proficiency group: M = 4.13, SE = 0.39), t(45) = −3.07, p = .02.
The main effect of mental demand was also significant, F(1, 45) = 49.1, p < .001, η p2 = .52, and was mediated by a significant interaction with proficiency group F(1, 45) = 10.5, p = .002, η p2 = .19. Participants in the lower group reported higher mental demand in L2 (M = 5.63, SE = 0.24) than in L1 (M = 3.38, SE = 0.3), t(45) = −7.33, p < .001. Such difference was only marginally significant for the higher-proficiency group (L1: M = 3.91, SE = 0.32; L2: M = 4.74, SE = 0.25, t(45) = −2.63, p = .07).
We found a significant main effect of performance, F(1, 45) = 7.05, p = .003, η p2 = .18, with participants reporting better self-perceived performance for texts in L1 (M = 5.02, SE = 0.17) than in L2 (M = 4.47, SE = 0.19), regardless of their proficiency level.
Similarly, we found a significant main effect of effort, F(1, 45) = 4.47, p = .04, η p2 = .09, with participants reporting having made higher effort for texts in L2 (M = 4.95, SE = 0.19) than in L1 (M = 4.55, SE = 0.21), regardless of their proficiency level.
4.4. Discussion
In Experiment 2 we wanted to explore whether the effects encountered in the first experiment varied as a function of L2 proficiency level. Overall, we observed a similar pattern of results in both studies wherein we replicated the effects of language and cohesion in both JOLs and memory. Specifically, individuals were assigned lower JOLs when studying texts in L2 (as opposed to texts in L1) and for low-cohesion texts (in contrast to high-cohesion texts). However, different patterns emerged between the higher- and lower-proficiency groups in both the monitoring measure (JOLs) during the study phase and in the subsequent learning assessment test.
First, the cohesion effect interacted with the language effect so that JOLs for texts with high cohesion did not differ between languages, whereas JOLs for texts with low cohesion were significantly higher in L1. The observed interaction suggests that high-cohesion texts may create a perception of learnability regardless of the language in which they are presented. However, the significant difference in JOLs between languages for low-cohesion texts indicates that the impact of cohesion on perceived learning difficulty might vary considerably between L1 and L2 and people found the L2 low-cohesion texts as the most difficult condition among all. For learning outcomes, this interaction revealed that people achieved similar learning within cohesion conditions for texts in L1, but high-cohesion texts were favored, compared to low-cohesion texts, in L2. Results from previous studies seem to contradict this pattern. For example, Jung (Reference Jung2018) investigated whether cognitive task complexity affects L2 reading comprehension and found that task complexity did not affect reading comprehension scores, although participants perceived the complex tasks significantly more demanding. Nevertheless, bilingual participants in Jung’s (Reference Jung2018) study reported staying in English-speaking countries, for at least 6 months. In our experiment, even people in the high-proficiency group were moderate in English–L2 and reported significantly less frequent exposure and use of English–L2 compared to Spanish–L1. This makes the results of the two studies difficult to compare.
More interestingly, the language effect in JOLs was modulated by the proficiency level. The differences encountered in JOLs between languages were only evident for the lower-proficiency group, who predicted greater difficulties in L2 compared to L1, as opposed to the higher-proficiency group, who predicted similar performance in both languages. This was exactly what the learning assessment test revealed. Participants with higher proficiency learned information from texts in L1 and L2 equally. However, participants with lower proficiency levels showed an L2 cost for learning. It seems that the proficiency level plays a crucial role in L2 self-regulated learning. These results go in line with previous research that had already reported that less proficient L2-English speakers needed longer time for reading, particularly when encountering sentences that conflicted with the previously established expectations in the text. This indicates a decreased efficacy in the high-level cognitive processes involved in L2 processing (Pérez et al., Reference Pérez, Schmidt and Tsimpli2023). On the contrary, in their study, higher-proficient participants showed better text comprehension, and better ability to generate predictive inferences. Thus, they concluded that linguistic proficiency makes a difference in high-ordered processes such as inferential evaluation, revision, and text comprehension (Pérez et al., Reference Pérez, Schmidt and Tsimpli2023). It seems that when studying in L2, lower-proficiency learners might encounter greater challenges, especially when the to-be-study material is ambiguous or incongruent.
Note that when merging data from both experiments, we found a three-way interaction involving language, proficiency, and type of study (language blocking vs. language mixing) in JOLs. The language difference in low cohesion texts was significant only for low-proficient participants in the language mixing condition (Experiment 2). Presenting texts with mixed languages likely increased the prominence of language as a cue for participants with lower L2 proficiency due to the need for language control from switching.
In sum, it seems that participants could correctly monitor their learning regardless of their proficiency level. Participants were able to detect the difficult parts of the material and adjust their judgments accordingly. Moreover, the learning assessment test was consistent with their predictions. This is true even when they are not highly proficient, as shown by the accuracy resolution. The interaction between the Goodman–Kruskal correlations and the proficiency group showed that the lower proficiency group was more accurate in L2 texts than in L1, suggesting that their performance in L2 was consistent with what they had previously predicted (JOLs) and reported in the questionnaire (higher mental demand L2 condition).
Apparently, participants could devote sufficient cognitive resources so as to unfold metacognitive strategies and correctly monitor their learning. Results from the qualitative questionnaire suggested that participants engaged different learning strategies depending on the language (L1 vs. L2) and their L2 proficiency level. For example, metacognitive self-regulation was more frequently used in L2 than in L1 in both proficiency groups. More interestingly, lower-proficiency participants used effort regulation more frequently in L2. This may suggest that people had enough cognitive resources available and could use them to select efficient learning strategies even when studying in L2. Studies have highlighted the flexibility of cognitive processes, indicating that individuals can flexibly allocate cognitive resources depending on task demands and situational factors (Broekkamp & Van Hout-Wolters, Reference Broekkamp and Van Hout-Wolters2007; Panadero et al., Reference Panadero, Fraile, Fernández Ruiz, Castilla-Estévez and Ruiz2019). This adaptability allows individuals to optimize their learning strategies, even in challenging L2 learning contexts. Nevertheless, although participants could devote sufficient cognitive resources so as to unfold metacognitive strategies and correctly monitor their learning, L2 proficiency seems to play a critical role in learning outcomes, as lower proficient participants showed an L2 learning cost despite unfolding monitoring processes correctly.
5. General discussion
The goal of the present study was to explore the consequences of studying texts in L2 on the cognitive and metacognitive processes involved in successful learning, and whether they varied as a function of L2 proficiency. In two experiments, university students were asked to study L1 and L2 texts that differed in difficulty (high and low cohesion). After comprehensively reading each text, they were asked to judge their learning and to answer some questions regarding what they had just studied. Results indicated that studying texts in L2 did not compromise the monitoring of learning since participants—regardless of their proficiency level—were able to use language and cohesion as cues to indicate the difficulty of the texts and judge their learning accordingly. Moreover, data from the learning assessment test validated the pattern observed in the study phase with the JOLs.
Overall, participants judged texts in L2 as more difficult than texts in L1. This language effect in JOLs is consistent with the results of our previous studies involving single words and lists in L1 and L2. Thus, Reyes et al. (Reference Reyes, Morales and Bajo2023) also reported that participants were sensitive to linguistic features of the to-be-studied material and predicted better learning in L1 than in L2 when they studied concrete vs. abstract words and lists of words grouped into semantic categories vs. lists of unrelated words. Hence, our new results extend these findings with a more complex set of materials and two different proficiency groups.
Similarly, high-cohesion texts were judged as easier to learn than low-cohesion texts. The effect of cohesion goes in line with previous studies on monolingual text comprehension and learning assessment (Carroll & Korukina, Reference Carroll and Korukina1999; Lefèvre & Lories, Reference Lefèvre and Lories2004; Rawson & Dunlosky, Reference Rawson and Dunlosky2002). But the most remarkable pattern here is that even though participants judged texts in L2 as more difficult to learn, this difficulty did not preclude the use of monitoring processes that allowed them to detect difficult material (low-cohesion texts) and to accurately judge it as more challenging to learn. Hence, monitoring processes were not impaired as a consequence of L2 context.
In Experiment 2, the main effects in JOLs were modulated by the interaction between factors. Thus, cohesion interacted with language such that there was no difference in L1 and L2 for high-cohesion texts, yet for low-cohesion texts, the L2 condition received significantly lower JOLs. Results after collapsing across experiments validated this interaction. This suggests that participants found low-cohesion texts in L2 as the most difficult condition among all four. This pattern did not support our initial hypothesis that, under the L2 condition monitoring and regulation processes might be compromised. In fact, the contrast between high and low cohesion in L1 was less pronounced than that observed in L2. Although the result was unexpected, it is conceivable that the presence of a salient cue such as language (L1 vs. L2) could diminish the salience of text cue (cohesion) as a cue, which, in turn, may reduce the perceived learning difficulties (JOLs) within the easier L1 condition. In this line, Magreehan et al. (Reference Magreehan, Serra, Schwartz and Narciss2016) reported data indicating a reduction of the font type effect on JOLs when other cues were available and thus, we might have expected an attenuation of the cohesion effect in our study when L1-L2 language cues were evident.
Note that a difference between Experiment 1 and 2 was that language was blocked in Experiment 1, whereas it was semirandom in Experiment 2. In fact, the triple interaction between language, proficiency, and type of study (language blocking vs. language mixing) was significant when merging both experiments. The difference between languages in low cohesion texts was only significant when participants studied in a language mixing condition (Experiment 2). Mixing languages in text presentation may have heightened the salience of language as a cue due to the need for language control derived from the switching, at the expense of within-text cues like cohesion. More cognitive resources were devoted to the task because of the necessary language control. Consequently, the cohesion cue was more prominent under the more demanding language condition (L2), which made the interaction between the two factors (cohesion x language) appear. Interestingly, the subsequent learning test also showed a tendency for the cohesion effect to be modulated by language. Thus, the learning outcome was significantly worse in low-cohesion texts than in high-cohesion texts, but only in L2. Hence, it is also possible that the semirandom mix of the languages across the texts may have increased the overall need for regulation, and participants may have engaged in control processes leading them to learn and perceive low-cohesion texts equally easily than high cohesion in L1. In contrast, when learning was performed in the more demanding L2 condition, the engagement of control processes may have not reduced the learning difficulty of the texts which were in turn also perceived as more difficult. Because these explanations are ad hoc, they should be more directly tested. Further research should directly address the consequences of language blocking and mixing in JOLs and memory performance. Overall, regarding our main question of whether L2 texts compromised metacognitive monitoring, results seem to suggest otherwise.
Furthermore, some nuances were found in Experiment 2, between proficiency groups. Interestingly, participants with a higher proficiency level judged L1 and L2 texts equally easy to learn and indeed did not show any sign of L2 cost in the learning assessment test. The lower-proficiency group, though, considered L2 texts as more difficult during the study and actually showed a disadvantage in L2 learning. This pattern of results remains consistent when considering both experiments together. The most remarkable pattern is that language did not impede monitoring under text cohesion manipulations. Low and medium-proficiency participants can still metacognitively monitor the difficulty of the texts, although they cannot compensate for such difficulty. Low and medium proficiency may impact learning, although participants seem to still use metacognitive processes to detect difficulties and regulate their learning. The poorer learning outcomes in L2 for low and medium-proficiency relative to high-proficiency participants go in line with previous research that reported a recall cost in L2 when participants were tested with essay-type questions, presumably due to a lack of writing skills (Vander Beken et al., Reference Vander Beken, Woumans and Brysbaert2018). We intended to overcome this issue by including open-ended questions that did not require much elaboration, which might have prevented the production deficit. Furthermore, our rubric accepted answers if they included the keywords, regardless of grammatical, syntactic, or orthographic errors. One might claim that both proficiency groups differed in their reading and writing skills, that the lower-proficiency group is composed of unskilled or inexperienced readers in general. However, proficiency groups did not differ in their accuracy for texts in L1, which proves that the differences in the learning assessment test cannot be explained by participants in the higher-proficiency group being better comprehenders. Hence, several factors could explain our lower-proficiency group recall cost in L2: impaired encoding, difficulty in integrating the information, or simply retrieving it from memory.
Note, however, that none of the experiments showed any evidence for compensation. Thus, participants in Experiment 1 did not compensate for the difficulty detected in the study phase to achieve comparable learning in L2 (in contrast to L1) and in low-cohesion (in contrast to high-cohesion) texts. Similarly, in Experiment 2, lower-proficiency individuals did not show compensation effects despite they also detected the difficulty of the low-cohesion texts. The only condition where learning for low-cohesion and high-cohesion texts was comparable was when higher-proficiency individuals studied in L1. However, the effect of cohesion was also absent in JOLs for these individuals, suggesting that for higher-proficiency individuals, the lack of coherence effects in learning outcomes was not due to compensation but to the fact that low- and high-cohesion texts produced similar levels of difficulty for them. As mentioned, this might be due to the greater engagement of control processes when L1 and L2 are presented in a mixed format which may induce better learning in L1. Koriat et al. (Reference Koriat, Ma’ayan and Nussinson2006) proposed that the relationship between monitoring and control processes arises from the fact that metacognitive judgments are based on feedback from the outcome of control operations. Monitoring does not occur prior to the controlled action, but rather, it takes place afterward. According to this hypothesis, the difficulty of an item is monitored ad hoc: learners allocate the appropriate resources to an item based on its demands, and they recognize that a specific item will be challenging to remember when they realize that it requires a relatively higher level of effort to commit to memory. Thus, although the initial assessment of a situation provides valuable information for executing control actions, the feedback obtained from these actions can subsequently be used as a foundation for monitoring. This monitoring process, in turn, can guide future control operations, creating a cyclical relationship between monitoring and control. In other words, subjective experience informs the initiation and self-regulation of control operation that may in turn change subjective experience.
The lack of evidence for compensation agrees with previous studies indicating that participants do not fully compensate for item difficulty effects through self-regulation. Although one would expect that if learners detect difficulties in the learning materials, they would compensate for this by allocating more time or by selecting a different strategy to better learn this information, there is strong evidence that neither self-paced study (how people allocate their study time), item selection for re-study, or the use of strategies (e.g., distributed practice, retrieval practice) completely compensate for difficulty (Cull & Zechmeister, Reference Cull and Zechmeister1994; Koriat, Reference Koriat2008; Koriat et al., Reference Koriat, Ma’ayan and Nussinson2006; Koriat & Ma’ayan, Reference Koriat and Ma’ayan2005; Le Ny et al., Reference Le Ny, Denhiere and Le Taillanter1972; Mazzoni et al., Reference Mazzoni, Cornoldi and Marchitelli1990; Mazzoni & Cornoldi, Reference Mazzoni and Cornoldi1993; Nelson & Leonesio, Reference Nelson and Leonesio1988; Pelegrina et al., Reference Pelegrina, Bajo and Justicia2000; see Tekin, Reference Tekin2022 for a review).
The fact that the cohesion effects in learning were evident in most conditions of Experiments 1 and 2 does not discard the possibility that learning is influenced by the language of study. First, medium-low-proficiency participants showed poorer levels of learning in their L2 than in their L1. Second, results from the qualitative questionnaire suggested that participants engaged in different learning strategies when studying in L1 and L2. This might support the idea that they confront L2 learning with different learning strategies. Overall, participants relied on deep-level strategies more in L1 than in L2. However, metacognitive self-regulation was more frequently used in L2 than in L1 in both proficiency groups in Experiment 2. This may suggest that participants had enough cognitive resources available and could use them to select efficient learning strategies even when studying in L2. On the other hand, the selection of some strategies might require extra study time, which was not possible under the time constraint of our experiment. Thus, participants might have used deep-level strategies more frequently in L1 because they might have needed longer study time allocation to use them in L2 learning (Stoff & Eagle, Reference Stoff and Eagle1971). Nevertheless, the qualitative language difference in the use of metacognitive strategies is an aspect to further explore in the future.
In sum, self-regulated learning is a complex process that appears not to be hindered by L2 processing. So far, we have explored the monitoring process and the short-term consequences in memory and learning derived from studying in L2. As self-regulated learning unfolds, monitoring and control processes arise to evaluate and adjust one’s attention, understanding, and behavior. Participants estimate task difficulty, make learning judgments, and allocate resources accordingly, adjusting the pace of learning or regulating strategies use, for instance (Panadero, Reference Panadero2017). Apparently, our sample monitored the to-be-studied material correctly. However, medium-low participants were not able to compensate for the self-perceived difficulty in achieving successful learning in L2, as evidenced by the accuracy of the learning assessment test. This suggests that while L2 learners can monitor effectively, their control processes might be compromised, particularly among those with lower proficiency. Apart from being cognitively demanding, reading and learning in L2 involves a range of factors intertwined with the learner’s L2 proficiency, such as the knowledge of morphosyntax and vocabulary, especially academic vocabulary (Silva & Otwinowska, Reference Silva and Otwinowska2019). Thus, our results raise a note of caution for L2 learning in academic settings. Proficiency level seems to introduce some nuances in the learning processes, which may impact the resources available for metacognitive processing while performing school-related activities. Future research should identify the direct and indirect effects of language proficiency and self-regulated learning strategies on academic outcomes in bilingual education.
In sum, self-regulated learning is a complex process that appears not to be hindered by L2 processing. So far, we have explored the monitoring process and the short-term consequences in memory and learning derived from studying in L2. As self-regulated learning unfolds, monitoring and control processes arise so as to evaluate and adjust one’s attention, understanding, and behavior. Participants estimate task difficulty, make learning judgments, and allocate resources in accordance, adjusting the pace of learning or regulating strategies use, for instance (Panadero, Reference Panadero2017). Apparently, our sample monitored the to-be-studied material correctly. However, medium-low participants were not able to compensate for the self-perceived difficulty in achieving successful learning in L2 as proven by the accuracy of the learning assessment test. Thus, our results raise a note of caution for L2 learning in academic settings. Proficiency level seem to introduce some nuances in the learning processes which may impact the resources available for metacognitive processing while performing school-related activities. Future research should identify the direct and indirect effects of language proficiency and self-regulated learning strategies on academic outcomes in bilingual education.
Moreover, there are other crucial factors that might also play a role in the self-regulation of learning (Lonka et al., Reference Lonka, Olkinuora and Mäkinen2004). Text difficulty and language proficiency might not be the only aspects that guide self-regulated learning: executive functions, motivation, self-efficacy, and other task or situational demands can also influence the learning process. A framework that incorporates all these metacognitive and motivational components of self-regulation into account would be more suitable to explain the effects of learning in L2 on monitoring and retention. Overall, our study underscores the importance of considering factors such as L2 proficiency that affect self-regulated learning. It also highlights the need to accommodate these complex dynamics between monitoring and control strategies to enhance academic success in bilingual settings.
Supplementary material
To view supplementary material for this article, please visit http://doi.org/10.1017/S1366728924000683.
Data availability statement
The data that support the findings of this study are openly available in OSF at https://osf.io/dw4y7/?view_only=4eeb04437db14d69b2269a8d19392df5
Acknowledgments
This research was supported by the doctoral research grant FPU18/01675 to Marta Reyes; by grants from the Spanish Ministry of Economy and Competitiveness (PPID2021-127728-NB); to M. Teresa Bajo and Julia Morales, and by the Research Fund of the Universidad Loyola Andalucía to Julia Morales. This work is part of the doctoral thesis of the first author and it has been supervised by the other two coauthors in equal terms. The funding bodies had no role in study design, data collection, and analysis, the decision to publish, or the preparation of the manuscript. We wish to acknowledge Simon Verley for his assistance in script programming. Funding for open access charge: Universidad de Granada / CBUA.
Appendix 1. Example of a high-cohesion and a low-cohesion English version
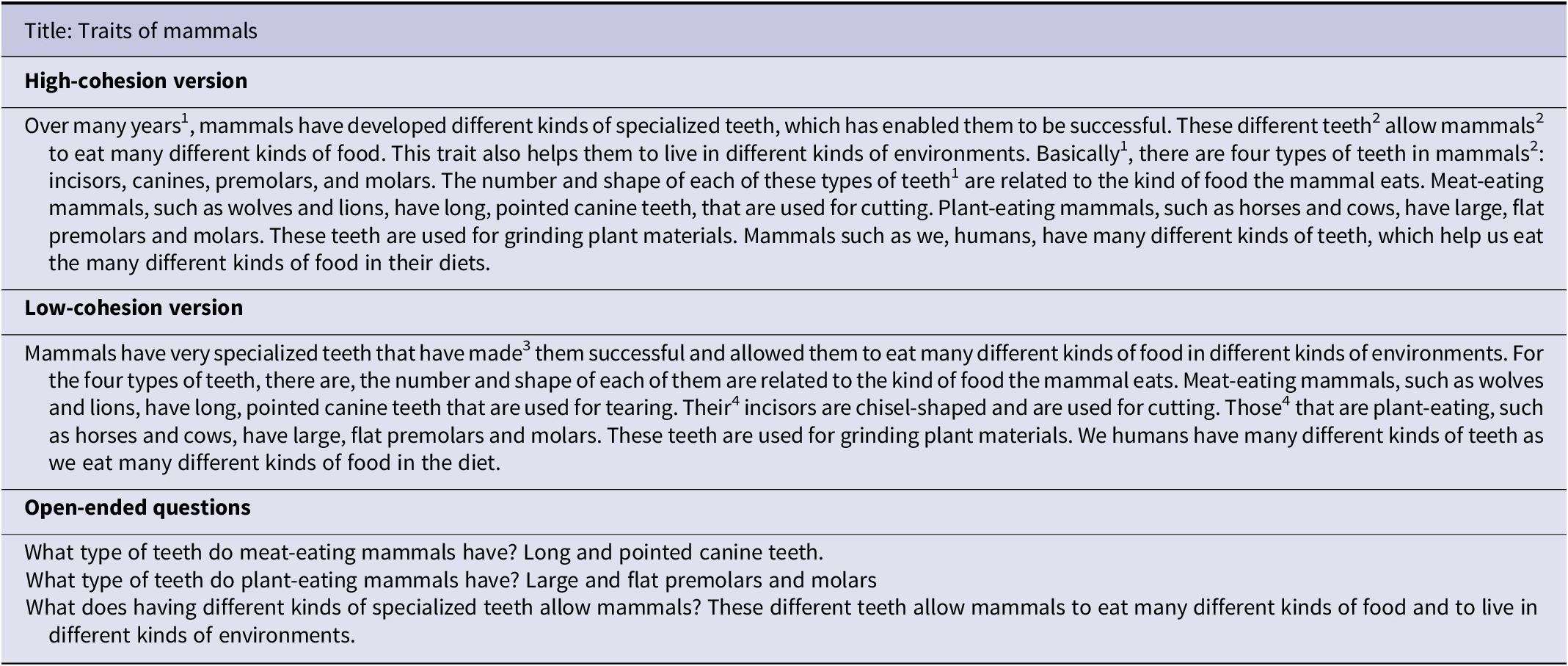
Note: 1 = noun repetition, 2 = use of connectors, 3 = use of passive voice, 4 = pronoun replacement to create ambiguity.
Appendix 2. Texts and references they were extracted from
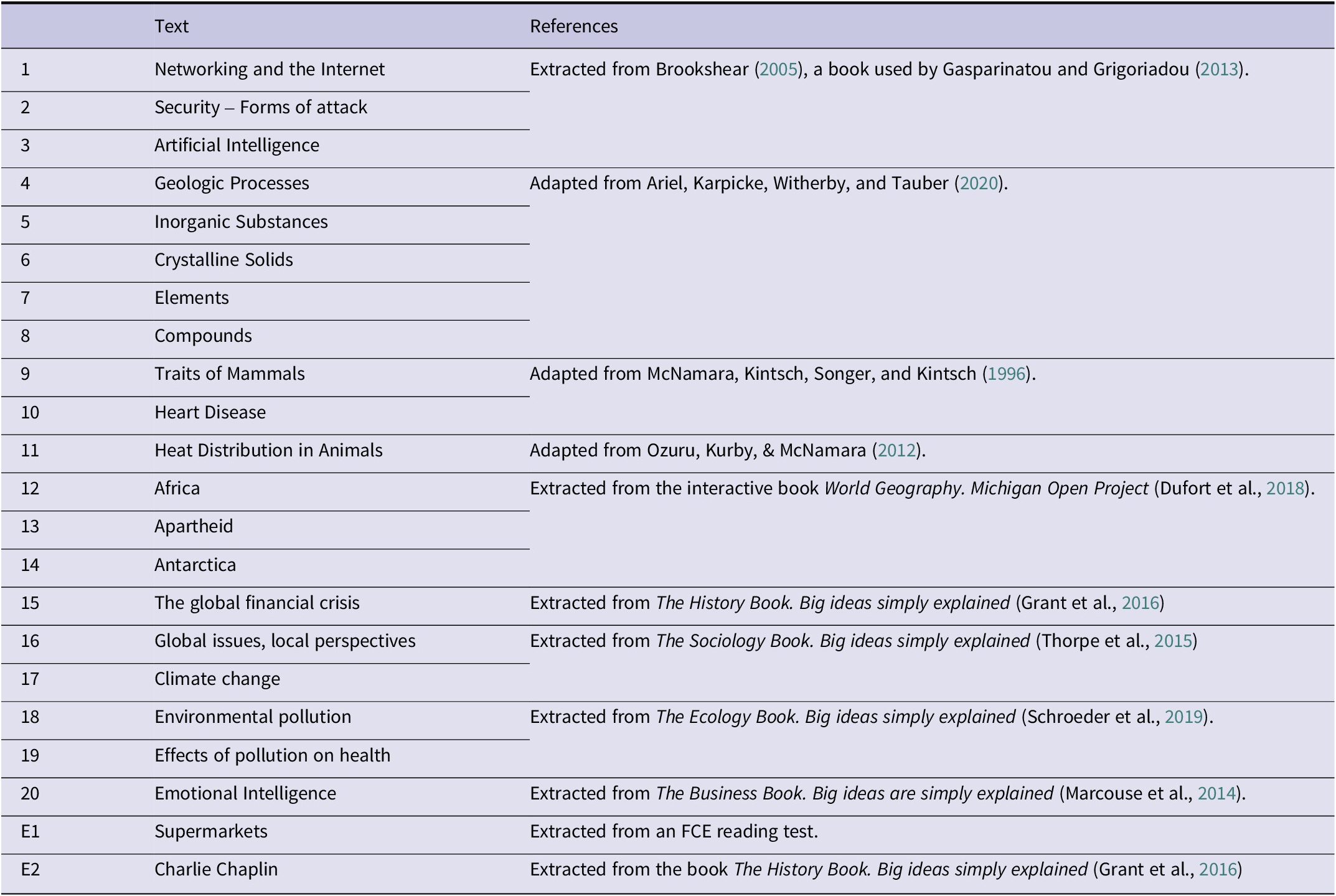
Appendix 3. English version of the customized metacognitive questionnaire. Note that the learning strategy each item refers to appears in brackets
-
1. I memorized and repeated keywords to remind me of important concepts in the texts. (Rehearsal)
-
2. I tried to understand the material in the texts by making connections between the concepts. (Elaboration)
-
3. When studying the information in the texts, I found myself questioning things to decide if I find them convincing. (Critical thinking)
-
4. When studying the materials in the texts, I often missed important points because I was thinking of other things. (R) (Metacognitive self-regulation)
-
5. When the content was difficult, I gave up or only paid attention to the easy parts. (R) (Effort regulation)
-
6. How mentally demanding was the task? (Mental demand)
-
7. How successful were you in accomplishing what you were asked to do? (Performance)
-
8. How hard did you have to work to accomplish your level of performance? (Effort)