Over the past 50 years, breakfast has been touted as an essential part of the diet to prevent and/or treat obesity( Reference Leidy, Hoertel and Douglas 1 – Reference Spence 3 ). In fact, skipping breakfast has been associated with weight gain and other adverse health outcomes, including insulin resistance and an increased risk for developing type 2 diabetes( Reference Smith, Gall and McNaughton 4 – Reference Bi, Gan and Yang 9 ). Systematic reviews, including a meta-analysis, suggested that a positive association between skipping breakfast and being overweight or obese is globally observed regardless of cultural differences among countries( Reference Haug, Rasmussen and Samdal 10 – Reference Chatelan, Castetbon and Pasquier 13 ). On the other hand, some reported studies do not support a clear effect of regularly consuming or skipping breakfast on body mass and energy metabolism( Reference Brown, Bohan Brown and Allison 14 – Reference Betts, Chowdhury and Gonzalez 17 ).
Glycaemic control is a key component of effective diabetes management because postprandial glucose level and glucose fluctuations contribute to the activation of oxidative stress. The maintenance of near-normoglycaemia is critical to minimise the risk of developing micro- and macrovascular complications that are generally associated with diabetes( Reference Ceriello 18 – Reference Hu, Stampfer and Solomon 21 ), and postprandial hyperglycaemia is an independent risk factor for diabetes-associated complications and mortality( Reference Monnier, Colette and Owens 22 ). Lowering glycaemia excursion and glucose variability are now considered major treatment targets in diabetic patients( Reference Ceriello 18 , Reference Cavalot, Petrelli and Traversa 23 , Reference Siegelaar, Holleman and Hoekstra 24 ), because postprandial hyperglycaemia was strongly associated with future development of vascular complications even when glycaemic control is restored in patients with type 2 diabetes( Reference Cavalot, Petrelli and Traversa 23 , Reference Monnier, Lapinski and Colette 25 , Reference Ceriello, Colagiuri and Gerich 26 ).
From the perspective of the postprandial glucose excursions, it was reported that low-carbohydrate (carbohydrate < 45 %) breakfast should be recommended for impaired glucose regulation subjects( Reference Kang, Wang and Lifang 27 ) and high-carbohydrate (carbohydrate > 60 %) intake at lunch provided the most favourable postprandial glucose profile than even distribution for type 2 diabetes( Reference Pearce, Noakes and Keogh 28 ). The effects of breakfast skipping on postprandial glucose response have been investigated in healthy middle-aged individuals (lunch contains 48 % carbohydrate)( Reference Jovanovic, Leverton and Solanky 29 ) and type 2 diabetes (lunch contains 54 % carbohydrate)( Reference Jakubowicz, Wainstein and Ahren 30 ). These studies( Reference Jovanovic, Leverton and Solanky 29 , Reference Jakubowicz, Wainstein and Ahren 30 ) have shown that insulin and C-peptide peak were delayed 30 min after lunch on the breakfast skipping day compared with the three-meal day. Additionally, the plasma level of NEFA was significantly higher after lunch when breakfast was omitted. Therefore, these studies( Reference Jovanovic, Leverton and Solanky 29 , Reference Jakubowicz, Wainstein and Ahren 30 ) suggested that the postprandial hyperglycaemia after lunch was induced by hepatic insulin resistance and impaired insulin response. In our previous studies in healthy young individuals( Reference Kobayashi, Ogata and Omi 31 , Reference Ogata, Kayaba and Tanaka 32 ), energy intake has been matched across 24 h following breakfast omission by increasing intake at subsequent meals (lunch and dinner). The effects of breakfast skipping elevated the postprandial glycaemic response after lunch (62 % carbohydrate) and increased the overall 24-h average blood glucose( Reference Kobayashi, Ogata and Omi 31 ). Moreover, when breakfast skipping is done for six consecutive days, the postprandial glycaemic response only elevated after lunch (60 % carbohydrate) on the first day of the breakfast skipping, and average blood glucose within 24 h only increased when the individual had sedentary lifestyle( Reference Ogata, Kayaba and Tanaka 32 ). The concept of our studies( Reference Kobayashi, Ogata and Omi 31 , Reference Ogata, Kayaba and Tanaka 32 ) was to conduct experiments with the isoenergetic diets per d; in the breakfast skipping condition, the individual ate extra energy at lunch and dinner in order to compensate for breakfast (i.e. lunch/dinner plus breakfast contents 1/2). Therefore, it was difficult to determine whether the high glycaemic response after lunch and increased 24-h average blood glucose for the breakfast skipping condition were consequences of breakfast omission, that is, second-meal phenomenon, or the extra energy at lunch.
The purpose of the present study was to assess the postprandial glycaemic response and to measure insulin, C-peptide and NEFA levels when healthy young individuals had identical lunch and dinner, and the 24-h average blood glucose as a secondary outcome. Thus, the present study aimed to clarify the influence of breakfast skipping on dynamics of blood glucose and hormone in healthy young individuals.
Methods
Subjects
A total of nine male sedentary individuals participated in the present study; these individuals were recruited through a poster on the laboratory information board. Their age, height and body weight were 21·4 (SD 1·4) years, 175·1 (SD 5·4) cm and 73·3 (SD 16·2) kg, respectively. According to the WHO criteria( 33 ), one individual was overweight and one was obese. Individuals with food allergies, who are occasional or habitual breakfast skippers, who perform regular exercise (more than three times per week), who smoke, with chronic diseases (diabetes, hypertension and hyperlipidaemia) or who regularly use medications were excluded. The present study was conducted according to the guidelines laid down in the Declaration of Helsinki, and all procedures involving human subjects were approved by the Local Ethics Committee of Hiroshima University, Japan (reference no.: 29–55). The experimental procedures and possible risks were fully explained to the participants before they signed the written informed consent.
Based on our previous study( Reference Kobayashi, Ogata and Omi 31 ), power analysis revealed that a sample size of eight individuals is required to provide 80 % power to detect 5 % difference between two dietary conditions in the 24-h average blood glucose. Because the 24-h average glucose value was highly related with the postprandial glycaemic response( Reference Chen, Xu and Su 34 ), and there were no previous data about the difference of acute postprandial glycaemia which were available for the sample size calculation for our study design. To allow discontinuation or measurement errors during the meal intervention, nine individuals were recruited.
Study design
The present study was a randomised crossover design with the following two experimental conditions: with breakfast (i.e. three-meal condition) and without breakfast (i.e. breakfast skipping condition). The two trials were conducted 1 week apart.
At 1 d prior to each trial, the participants were instructed to refrain from rigorous physical activity and from consuming beverage containing energy, caffeine or alcohol. The energy intake was controlled by individually adjusting three meals prior to each trial, and the size of the standardised meal during each trial was individually adjusted (2579 (SD 569) kcal/d (10 791 (SD 2381) kJ/d), 15 % protein, 25 % fat and 60 % carbohydrates; Table 1), based on the estimated energy requirement assuming a physical activity factor at 1·50( 35 , Reference Hatamoto, Goya and Yamada 36 ). Note that the size of the standardised meal was provided for each individual combining the following: pre-packed food or canned food; rice as a staple diet; beef, fish, bean and curry as main dishes; miso soup, seaweed, bamboo shoot and Japanese radish as side dishes; cheese as a dairy product; and vegetable juice as a fruit.
Table 1. Energy intake and nutrients for three standardised meals on the experiment day
(Mean values and standard deviations)

* To convert energy in kcal/d to kJ/d, multiply by 4·184.
The participants came to the laboratory using a taxi to avoid the influence of physical activity on the experiment. During the experimental trials, the participants received breakfast at 08.30 hours (33·3 % of daily energy intake) or no breakfast (0 kcal (0 kJ)), lunch at 13.30 hours (33·3 % of daily energy intake) and dinner at 18.30 hours (33·3 % of daily energy intake); they were also instructed to sit on the chair except when going to the toilet, and they spent the whole time watching television or personal computers and reading books. When breakfast was skipped (i.e. breakfast skipping condition), the participants ate identical lunch and dinner as the three-meal condition; that is, the energy intake per d decreased to 2/3 compared with the three-meal condition (1717 (SD 381) kcal/d (7184 (SD 1594) kJ), 15 % protein, 25 % fat and 60 % carbohydrates).
Measurements
Continuous glucose monitoring
The glucose levels were continuously measured using a glucose monitor (iPro2; Medtronic MiniMed) connected to a glucose sensor (Enlite Glucose Sensor, Medtronic MiniMed). The mean absolute relative difference value of this device is 11 %( Reference Rodbard 37 ). The sensor was placed on each participant’s abdomen 1 d before the experiment. The participants were asked to record their blood glucose concentrations more than four times by a finger stick the day before the experiment for calibration purposes.
The blood glucose within 24 h was used from 08.00 hours at meal intervention day to 08.00 hours the next morning. Postprandial blood glucose response was calculated as an AUC of the blood glucose curve above average 15-min pre-meal levels within 4 h using the trapezoidal method. The glycaemic variability was evaluated using the flowing approaches:
(a) Mean amplitude of glycaemic excursion is defined as the average glycaemic excursions exceeding 1 sd ( Reference Service, Molnar and Rosevear 38 ).
Continuous overall net glycaemic action (CONGAn) is defined as the sd of all the differences between the current observation and the observation at n hours (1, 2 and 4 h before the observation)( Reference McDonnell, Donath and Vidmar 39 ). For instance, for n 1, the calculations would begin as follows: blood glucose at 09.00 hours minus blood glucose at 08.00 hours, blood glucose at 09.05 hours minus blood glucose at 08.05 hours, blood glucose at 09.10 hours minus blood glucose at 08.10 hours and so on until blood glucose 08.00 hours (the next day) minus blood glucose at 07.00 hours. Then, the sd of the summed differences between these data was calculated. CONGAn is similar to sd but assesses glucose variability within a predetermined time window.
(b) Mean indices of meal excursions is defined as glucose rise to peak (ΔG), time to peak (ΔT) and timeliness of recovery to baseline glycaemia (% baseline)( Reference Service and Nelson 40 ).
(c) Detrended fluctuation analysis (DFA), which accurately quantifies the temporal correlation property of time series data( Reference Peng, Havlin and Stanley 41 ), has been previously described in detail( Reference Ogata, Tokuyama and Nagasaka 42 ). In DFA, long-range correlation is characterised by the exponent α in the scaling (power-law) relation F(n) − n α , where F(n) is a square root of mean-square deviations around a polynomial trend averaged over segments with length n of integrated time series. In DFA, n represents the analysed window size in min, while F(n) is referred as the fluctuation function. In the present study, we used the glucose data measured every 5 min, and we calculated F(n) over the range 40 ≤ n ≤ 350 min (in the logarithmic scale, 1·602 ≤ log10 n ≤ 2·544) and estimated the crossover of its scaling behaviour using linear least squares fitting. Finally, the short-range scaling exponent (α 1) was shorter than the crossover point, and the long-range scaling exponent (α 2) was longer than the crossover point. Additionally, we calculated the mean values of log10 F(n) at each scale in every participant, which was denoted by log10 Fm(n). White noise (uncorrelated time series) is characterised by α = 0·5, while Brownian motion (integrated white noise) is characterised by α = 1·5. Similar to the relation between white noise and Brownian motion, the value of α after the integration is increased by 1. Therefore, a negatively correlated long-range fluctuation of increments is represented by 1 < α < 1·5 for the measured glucose time series, whereas a positively correlated increments is represented by 1·5 < α < 2·0; furthermore, α > 2·0 can be explained by the repeated integration.
Biochemical and hormonal blood analyses
Following an overnight fast, the participants visited the test site laboratory at 08.00 hours in the morning and rested before the first blood collection. A baseline blood sample was subsequently obtained. Blood samples (2·5 ml) were also collected at 0, 60 and 120 min after each meal sessions; blood was obtained at the same time even after skipping breakfast. Serum and plasma samples were obtained after a 15-min centrifugation at 4°C, and the samples were stored at −80°C until analysis. From the obtained blood samples, blood glucose, serum insulin, C-peptide and plasma NEFA levels were measured. All the analyses were conducted using LSI Medience according to the operation manual.
Physical activity
Physical activity was measured using a tri-axial accelerometer (JHA-75°c; Omron Healthcare)( Reference Ohkawara, Oshima and Hikihara 43 ). The participants wore the accelerometer on their waist during the intervention period. Physical activity was assessed using step counts.
Subjective appetite and feeling
The participants’ subjective appetite (hunger and fullness) and feelings (fatigue and concentration) were evaluated using visual analogue scales. These scales were completed pre-breakfast (08.30 hours) and post-breakfast (09.30 and 10.30 hours), pre-lunch (13.30 hours) and post-lunch (14.30 and 15.30 hours) and pre-dinner (18.30 hours) and post-dinner (19.30 and 20.30 hours). Hunger, fullness, exhaustion and concentration were rated on a 100-mm line preceded by the question ‘How hungry do you feel right now?’ and anchored by ‘not at all hungry’ on the left and ‘extremely hungry’ on the right. Fullness, fatigue and concentration were rated using a visual analogue scales with the question ‘How full/fatigue/concentration do you feel right now?’, with the anchors ‘no at all’ and ‘extremely’.
Statistical analysis
Data are presented as mean values and standard deviations. Differences in the 24-h average blood glucose, standard deviation, glycaemic variability (mean amplitude of glycaemic excursion, CONGAn and mean indices of meal excursions) and DFA indices (α 1, α 2 and Fm) and postprandial blood glucose response (lunch and dinner) between the three-meal and breakfast skipping conditions were analysed using the paired t test. The differences between the DFA scaling exponents (α 1 and α 2) and the uncorrelated ‘reference’ value of α = 1·5 were also evaluated using the paired t test. Physical activity level from 08.00 to 21.00 hours between the three-meal and breakfast skipping conditions was analysed using the paired t test. To evaluate the differences in blood glucose between the two-meal conditions, mean values in the morning (08.00−13.30 hours), afternoon (13.30−18.30 hours), evening (18.30−23.30 hours) and sleep (23.30−08.00 hours) were calculated for each participant, and repeated measures of two-way ANOVA followed by a post hoc Bonferroni test were used. To evaluate the differences in insulin, C-peptide, NEFA and visual analogue scales scores between the two-meal conditions and repeated measures of two-way ANOVA followed by a post hoc Bonferroni test were used. All statistical analyses were performed using SPSS version 22.0 (SPSS Japan Inc.). Differences were considered significant when the error probability was <0·05.
Results
Blood glucose
The level of HbA1c was 5·2 (SD 0·3) % (range 4·8−5·7 %), and all the participants were healthy. Note that only one individual had an HbA1c level of 5·7 %. However, of the eighteen samples at the fasting plasma glucose (i.e. two conditions per individual), two were found to have normal high glucose: one was 110 mg/dl (6·1 mmol/l) and the other was 114 mg/dl (6·3 mmol/l). (To convert glucose in mg/dl to mmol/l, multiply by 0·0555.)
Differences in the mean blood glucose between the two-meal conditions in the morning (08.00−13.30 hours) (109·3 (SD 25·4) mg/dl for the three-meal condition v. 104·9 (SD 16·5) mg/dl for the breakfast skipping condition, P = 0·536), evening (18.30−23.30 hours) (116·9 (SD 23·6) v. 119·4 (SD 18·9) mg/dl, P = 0·480) and during sleep (23.30−08.00 hours) (97·3 (SD 15·2) v. 102·9 (SD 31·3) mg/dl, P = 0·432) were not significant; however, there was significant difference in the blood glucose in the afternoon (13.30−18.30 hours) (109·7 (SD 23·6) v. 130·4 (SD 22·3) mg/dl, P < 0·05) (Fig. 1(a)).
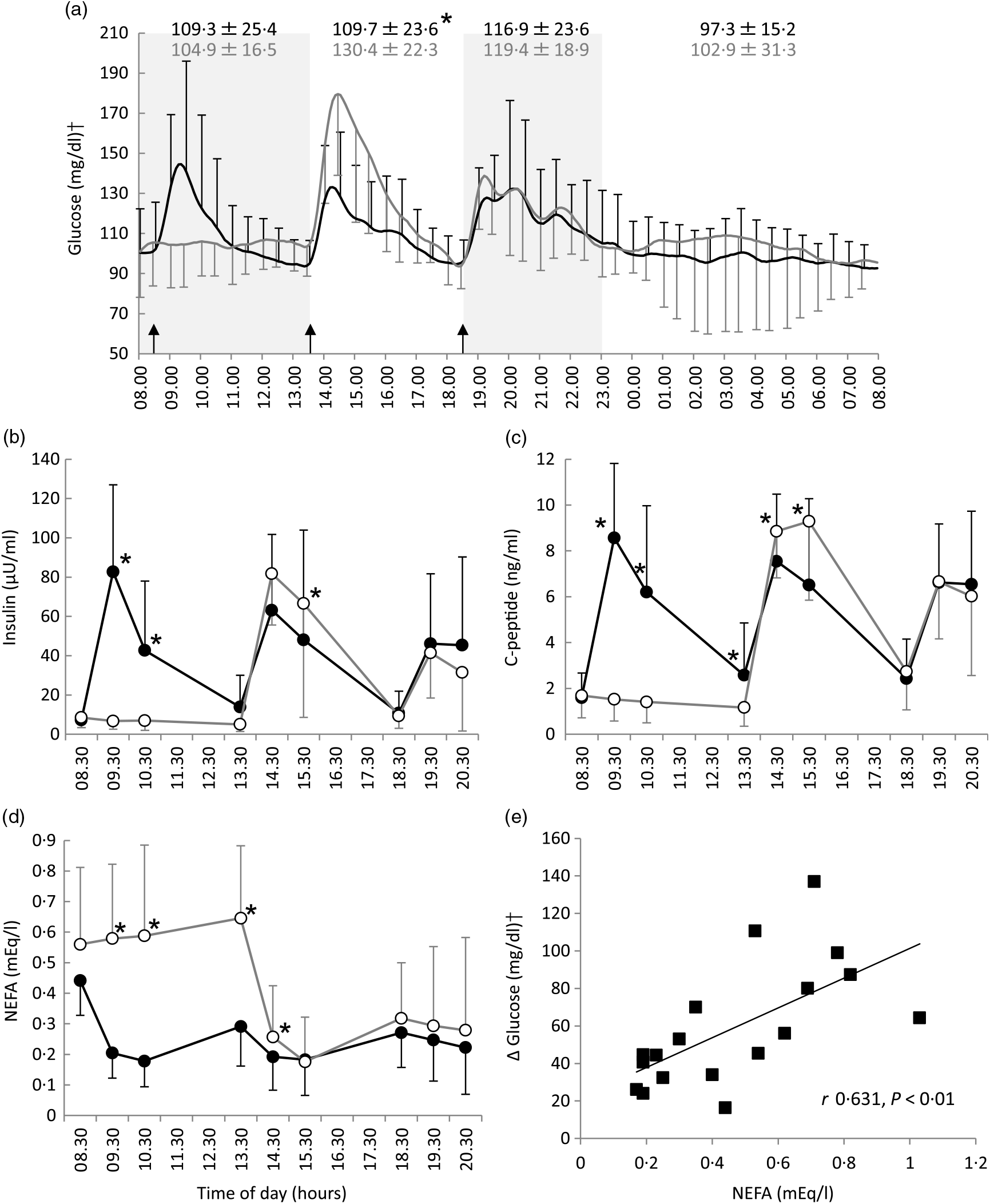
Fig. 1. All-day graphs for (a) glucose, (b) insulin, (c) C-peptide and (d) NEFA. Values are means, with standard deviations represented by vertical bars. (e) Relationship between pre-lunch NEFA and the maximal increase in blood glucose level after lunch. Mean values of blood glucose for all participants (n 9) were plotted every 5 min; +sd for three meals and –sd for breakfast skipping conditions were plotted every 30 min. Mean values of insulin, C-peptide and NEFA were plotted every 60 min; +sd for three meals and –sd for breakfast skipping conditions were also plotted every 60 min. * Significant difference between trials at the annotated time point (P < 0·05). •, Eating breakfast; ○, skipping breakfast. † To convert glucose in mg/dl to mmol/l, multiply by 0·0555.
After lunch, the postprandial blood glucose AUC in the breakfast skipping condition was significantly higher than that of the three-meal condition (3207 (SD 2476) v. 5762 (SD 2585) mg/dl × 4 h, P < 0·01), although there was no significant difference after dinner between these conditions (4092 (SD 3063) v. 4743 (SD 3636) mg/dl × 4 h, P = 0·537) (Table 2). Breakfast skipping did not significantly affect the 24-h average blood glucose values, CONGA1 and mean indices of meal excursions for dinner (P = 0·179, P = 0·145, P = 0·941, P = 0·800 and P = 0·079, respectively). Meanwhile, the 24-h sd (16·9 (SD 9·4) 21·6 (SD 10·6)), mean amplitude of glycaemic excursion (51·5 (SD 9·8) v. 66·5 (SD 10·2)), CONGA2 (1·27 (SD 0·81) v. 1·78 (SD 1·02)), CONGA4 (1·42 (SD 0·85) v. 2·11 (SD 1·22)) and mean indices of meal excursions for lunch (ΔG: 41·3 (SD 21·5) v. 81·1 (SD 30·4), −ΔG/ΔG: 85·9 (SD 11·6) v. 73·7 (SD 8·4)) were significantly different between the three-meal and breakfast skipping conditions (P < 0·05, Table 2).
Table 2. Indices of glycaemic variability for three-meal condition and skipping breakfast condition*
(Mean values and standard deviations)

MAGE, mean amplitude of glycaemic excursion; CONGAn, continuous overall net glycaemic action – the SD of all the differences, after the first n hours, between the current observation and the observation n hours (1, 2 and 4 h before the observation); MIME, meal indices of meal excursions; ∆G, postprandial glucose rise to peak; ∆T, time to peak; −∆G/∆G, baseline recovery 1 h after peak; DFA, detrended fluctuation analysis.
* To convert glucose in mg/dl to mmol/l, multiply by 0·0555.
† Postprandial blood glucose response within 4 h was calculated as an AUC of the blood glucose curve above average 15-min pre-meal levels during 4 h using the trapezoidal method.
The DFA plots for the three-meal condition (Fig. 2(a)) and for the skipping breakfast condition (Fig. 2(b)) are shown in Fig. 2. The two-line regression placed a crossover point for the grouped data at approximately 130 min, and the individual crossover points fell in a range from 110·0 to 197·5 min on the three-meal condition and from 82·5 to 152·5 min on the skipping breakfast condition. In the short-range regimen (<130 min for these conditions), the scaling exponent (α 1) for the three-meal condition was 2·95 (SD 0·19), and for the skipping breakfast condition, it was 3·02 (SD 0·12). These α 1 scaling exponents were larger than the ‘uncorrelated reference value’ of α = 1·5 (P < 0·001), meaning that the glucose fluctuation short-range regimen was positively correlated; that is, the net effects of glucose flux/reflux persisted within these shorter time scales. In the long-range regimen, the scaling exponent (α 2) for the three-meal condition was 1·57 (SD 0·18) and that for the skipping breakfast condition was 1·84 (SD 0·44). Moreover, the scaling exponent for the skipping breakfast condition was significantly different from 1·50 (P < 0·05), but the scaling exponent for the three-meal condition was not significantly different from 1·50 (P = 0·717). Between the two-meal conditions, there was no significant difference in Fm (Table 2).
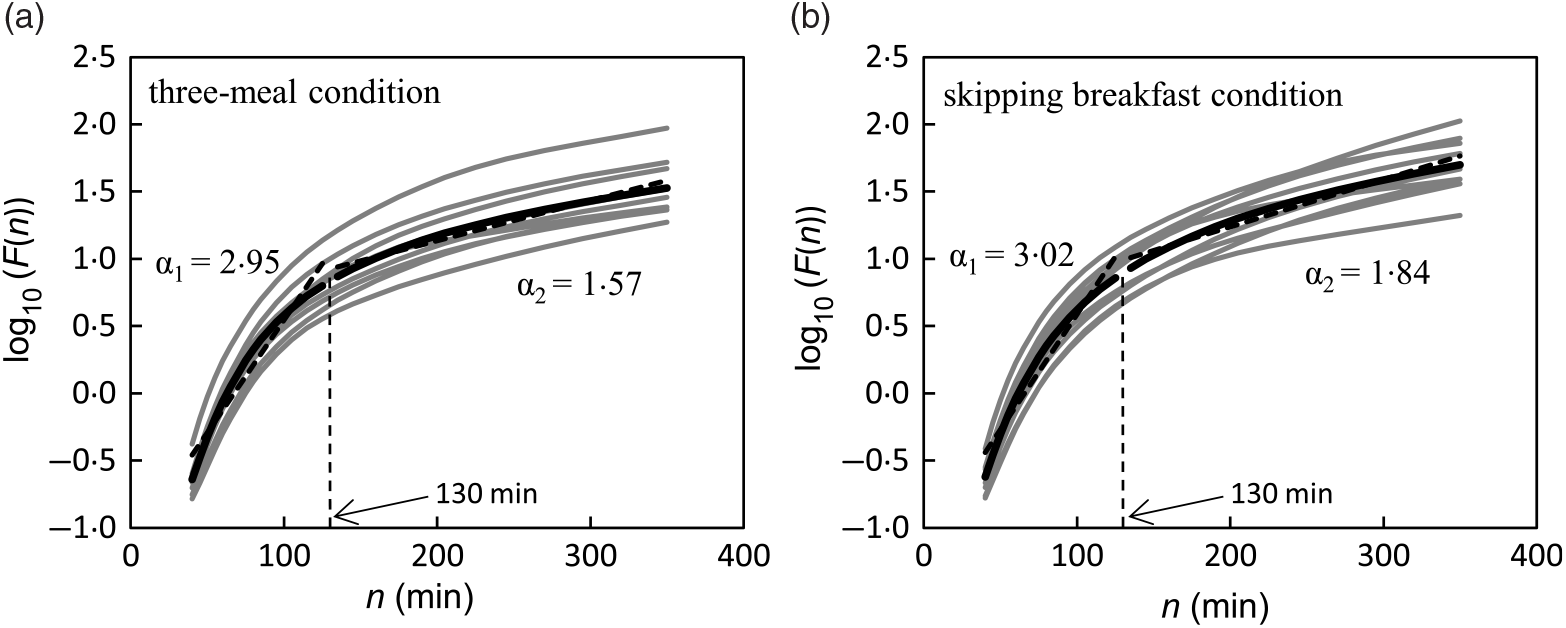
Fig. 2. Trial fluctuation functions F(n) for three-meal (a) and breakfast skipping conditions (b) (n 9). The black lines show the data for the mean value of each condition, and the grey lines show the data of each participant.
Biochemical and hormonal markers
Compared with the three-meal condition, insulin and C-peptide levels in the morning were significantly lower, and insulin and C-peptide levels after lunch were significantly higher on the breakfast skipping condition: insulin at 09.30 hours (82·7 (SD 44·3) v. 6·7 (SD 4·2) μU/ml, P < 0·001), insulin at 10.30 hours (42·7 (SD 35·3) v. 6·9 (SD 5·0) μU/ml, P < 0·01), insulin at 15.30 hours (48·1 (SD 55·8) v. 66·5 (SD 58·0) μU/ml, P < 0·05), C-peptide at 09.30 hours (8·6 (SD 3·2) v. 1·5 (SD 0·9) ng/ml, P < 0·001), C-peptide at 10.30 hours (6·2 (SD 3·8) v. 1·4 (SD 0·9) ng/ml, P < 0·01), C-peptide at 13.30 hours (2·6 (SD 2·3) v. 1·2 (SD 0·8) ng/ml, P < 0·05), C-peptide at 14.30 hours (7·5 (SD 2·9) v. 8·9 (SD 2·0) ng/ml, P < 0·005) and C-peptide at 15.30 hours (6·5 (SD 3·8) v. 9·3 (SD 3·4) ng/ml, P < 0·001). The NEFA level in the morning and after lunch in the breakfast skipping condition was significantly higher than that of the three-meal condition: at 09.30 hours (0·20 (SD 0·08) v. 0·58 (SD 0·24) mEq/l, P < 0·001), at 10.30 hours (0·18 (SD 0·08) v. 0·59 (SD 0·30) mEq/l, P < 0·01), at 13.30 hours (0·29 (SD 0·13) v. 0·65 (SD 0·24) mEq/l, P < 0·001) and at 14.30 hours (0·19 (SD 0·11) v. 0·26 (SD 0·17) mEq/l, P < 0·05). However, these results were not observed after dinner (Fig. 1(b)–(d)).
NEFA levels pre-lunch time (13.30 hours) were positively correlated with the rise in blood glucose after lunch, that is, the maximum glucose after lunch minus the average 15-min pre-lunch glucose levels (r 0·631, P < 0·01, Fig. 1(e)).
Physical activity
The participants’ step counts in the three-meal and breakfast skipping conditions were 547 (SD 266) and 534 (SD 258) counts, respectively (P = 0·890).
Rating of appetites and feelings
Ratings of hunger (Fig. 3(a)), fullness (Fig. 3(b)), fatigue (Fig. 3(c)) and concentration (Fig. 3(d)) are shown in Fig. 3. Rating scores for hunger in breakfast skipping condition were significantly higher than those in the three-meal condition (09.30 and 10.30 hours). Additionally, rating scores for fullness in the three-meal condition were significantly higher than those in the breakfast skipping condition (09.30 and 10.30 hours). These subjective appetites showed to be slightly different at pre-lunch (13.30 hours). On the contrary, rating scores for fatigue and concentration showed a slight difference between the two conditions (fatigue, 14.30 hours; concentration, 08.30 and 09.30 hours).
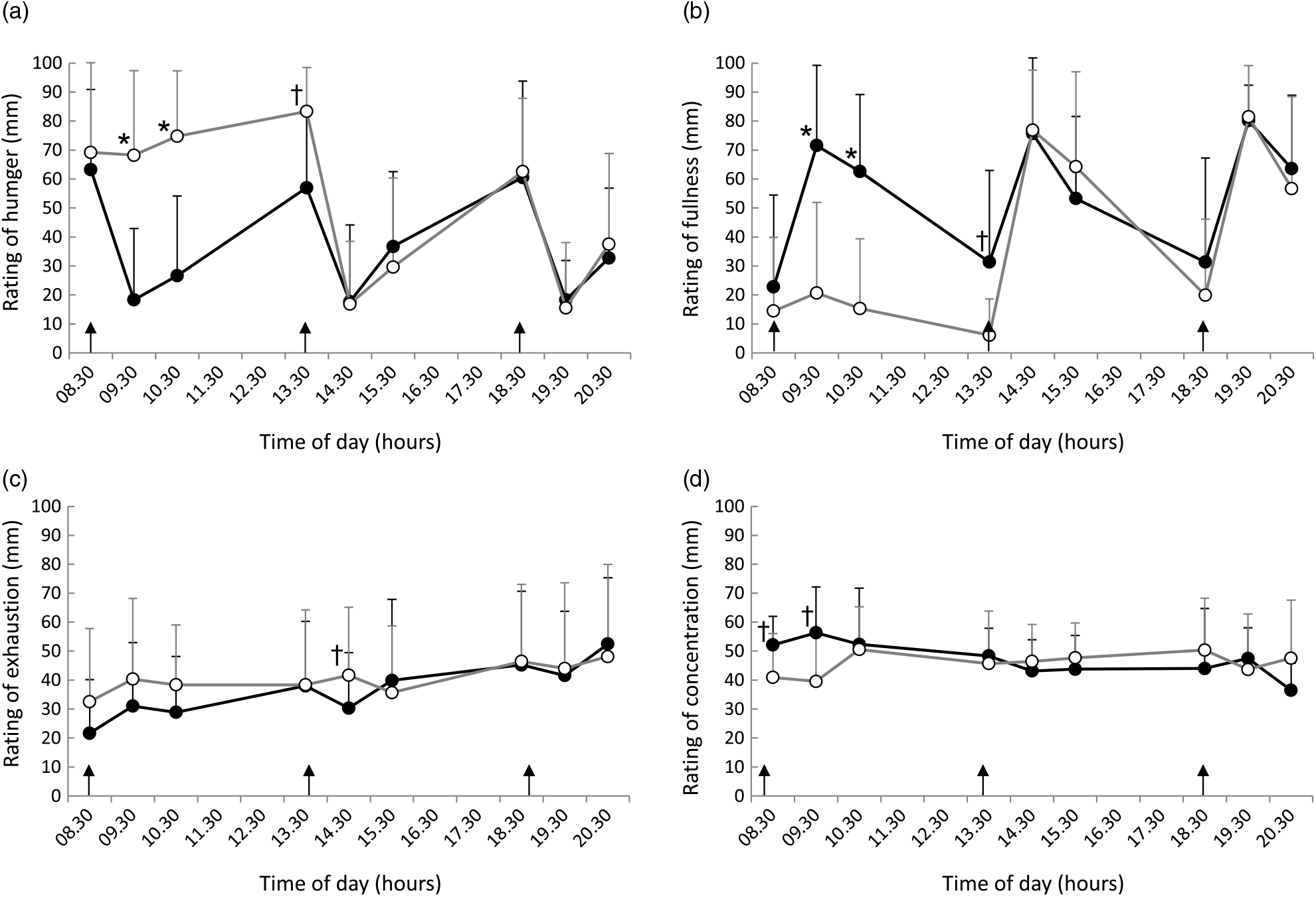
Fig. 3. Ratings of (a) hunger, (b) fullness, (c) exhaustion and (d) concentration throughout the day. Data are plotted every 60 min. Values are means, with standard deviations represented by vertical bars. * Significant difference between trials at the annotated time point (P < 0·05). † P < 0·1. •, Eating breakfast; ○, skipping breakfast.
Discussion
In the present study, we conducted an experiment to clarify the influence of breakfast skipping on the dynamics of blood glucose and hormone responses in healthy young individuals. All the meal including the breakfast consisted of a normal Japanese standardised meal (15 % of energy from protein, 25 % from fat and 60 % from carbohydrate). As a result, the omission of breakfast resulted in a significantly higher glycaemic response only after lunch as compared with the glycaemic response after an identical lunch when breakfast was consumed. Interestingly, despite the difference in the total energy intake (breakfast skipping condition was 33 % decreased), the 24-h average blood glucose was similar between the two-meal conditions. Furthermore, plasma NEFA level was significantly higher after lunch when breakfast was omitted, and the level of NEFA positively correlated with the postprandial glycaemic response.
The result of the hyperglycaemic response after lunch showed the ‘second-meal phenomenon’ in the present study, and it is consistent with the previous studies( Reference Jovanovic, Leverton and Solanky 29 , Reference Bonuccelli, Muscelli and Gastaldelli 44 , Reference Wajngot, Grill and Efendić 45 ). The second-meal phenomenon has been observed consistently in healthy people( Reference Jovanovic, Leverton and Solanky 29 , Reference Bonuccelli, Muscelli and Gastaldelli 44 , Reference Wajngot, Grill and Efendić 45 ), but its presence is controversial in people with diabetes mellitus( Reference Ceriello 18 , Reference Cavalot, Petrelli and Traversa 23 , Reference Siegelaar, Holleman and Hoekstra 24 , Reference Carey, Halliday and Snaar 46 – Reference Nuttall, Mooradian and Gannon 48 ). The second-meal phenomenon can influence the postprandial glycaemic response at the next meal: meal skipping( Reference Jovanovic, Leverton and Solanky 29 , Reference Jakubowicz, Wainstein and Ahren 30 , Reference Jovanovic, Gerrard and Taylor 47 , Reference Lee, Tura and Mari 49 , Reference Chowdhury, Richardson and Tsintzas 50 ), fermentable dietary fibre consumption( Reference Nilsson, Ostman and Preston 51 – Reference Brighenti, Benini and Del Rio 54 ), fibre-enriched bread consumption( Reference Lunde, Hjellset and Holmboe-Ottesen 55 ), low glycaemic-index meal consumption( Reference Jenkins, Wolever and Nineham 56 – Reference Liljeberg and Björck 63 ), high-protein meal consumption( Reference Chen, Jovanovic and Taylor 64 – Reference Park, Heden and Liu 66 ) or high-fat meal consumption( Reference Ando, Nakae and Usui 67 ). Moreover, the extent of postprandial rise in plasma glucose depends not only on the quantity and nature of food ingested but also on the metabolic state, that is, increased muscle glycogen storage( Reference Jovanovic, Leverton and Solanky 29 , Reference Carey, Halliday and Snaar 46 , Reference Jovanovic, Gerrard and Taylor 47 , Reference Chen, Jovanovic and Taylor 64 , Reference Wolever, Bentum-Williams and Jenkins 68 ), decreased hepatic insulin clearance( Reference Wajngot, Grill and Efendić 45 ), slowed gastric emptying( Reference Arai, Mizuno and Sakuma 61 , Reference Granfeldt, Wu and Björck 62 ), increased incretion hormone secretion and stronger glucose potentiation and suppression of endogenous glucose production( Reference Liljeberg and Björck 63 ), immediately prior to eating.
We investigated the possible role of NEFA level, because suppression of NEFA after the first meal has been suggested as an important mechanism for the second-meal phenomenon. Due to omission of breakfast, plasma NEFA level was significantly higher in the morning, that is, acute elevation of plasma NEFA induced hepatic insulin resistance and increased hepatic glucose production in healthy individuals( Reference Boden 69 , Reference Ferrannini, Barrett and Bevilacqua 70 ). The NEFA level just before lunch correlated positively with the post-lunch glucose increment, a finding that is consistent with the previous reports showing similar results in both diabetic and nondiabetic people( Reference Jovanovic, Leverton and Solanky 29 , Reference Carey, Halliday and Snaar 46 , Reference Jovanovic, Gerrard and Taylor 47 , Reference Lee, Tura and Mari 49 , Reference Chen, Jovanovic and Taylor 64 , Reference Wolever, Bentum-Williams and Jenkins 68 ). Therefore, skipping breakfast triggers hepatic insulin resistance and increased hepatic glucose production; consequently, glucose level elevated the postprandial glycaemic response after lunch. Wolever et al.( Reference Wolever, Bentum-Williams and Jenkins 68 ) showed that the acute role of NEFA on insulin action has been demonstrated in a study where the different compositions of the first meals resulted in different patterns of NEFA responses prior to the second meal. The lesser rebound of plasma NEFA resulting in lower concentrations before lunch was also closely related to the improved glycaemic responses after lunch in nondiabetic subjects. Jovanovic et al.( Reference Jovanovic, Leverton and Solanky 29 ) showed a negative relationship between the increment in muscle glycogen signal and pre-lunch NEFA level and hypothesised that suppressed NEFA level after the first meal improves insulin action, which would facilitate postprandial muscle glycogen storage. The authors also showed that the NEFA levels before lunch correlated positively with the post-lunch increase in blood glucose( Reference Jovanovic, Leverton and Solanky 29 ). The present study is different from the present study based on the following points: (1) identical meals were provided, not according to the individual’s body weight, (2) glucose levels were measured only up to 4 h after lunch and (3) the authors did not mention the average blood glucose levels within 24 h. By contrast, Jakubowicz et al.( Reference Jakubowicz, Wainstein and Ahren 30 ) showed that breakfast skipping in patients with type 2 diabetes induced higher glucose excursions after lunch and dinner, and plasma NEFA levels were significantly increased throughout the day. Thus, skipping breakfast increases postprandial hyperglycaemia after lunch and dinner in association with impaired insulin response, despite eating the same amount of energy for lunch and dinner( Reference Jakubowicz, Wainstein and Ahren 30 ). The effect of second-meal phenomenon was not only restricted to the subsequent meal but also extended to dinner in patients with type 2 diabetes; that is, it had a much longer duration than previously anticipated. In fact, omission of breakfast indeed worsened the postprandial glucose and impaired insulin secretion, as reflected by both delayed insulin peak and decreased insulin response at lunch and dinner( Reference Jakubowicz, Wainstein and Ahren 30 ). Furthermore, the increased postprandial hyperglycaemia after lunch and dinner was not caused by the meal energy in patients with type 2 diabetes.
The 24-h average blood glucose was not different between the two-meal conditions, with the following possible mechanisms: (1) because insulin and C-peptide levels for the three-meal condition were significantly higher compared with that of breakfast skipping condition, (2) because second-meal phenomenon was induced in the breakfast skipping condition and (3) because humans have glucose homeostasis. In the present study, the healthy individuals ate breakfast that has even distribution (33 % of total energy and 60 % carbohydrate); consequently, the postprandial glycaemic response after lunch was significantly increased in the breakfast skipping condition, which is consistent with the previous studies( Reference Jovanovic, Leverton and Solanky 29 , Reference Bonuccelli, Muscelli and Gastaldelli 44 ). Previous study that considered the amount of carbohydrate ingested at breakfast, although it is not a breakfast omission trial, reported the following: for type 2 diabetes, it was reported that big breakfast (33 % of total energy and 37–48 % carbohydrate) improved glycaemic control than small breakfast (12·5 % of total energy and 60–70 % carbohydrate)( Reference Rabinovitz, Boaz and Ganz 71 ), and high-carbohydrate (carbohydrate > 60 %) intake at lunch provided the most favourable postprandial glucose profile than even distribution( Reference Pearce, Noakes and Keogh 28 ). For impaired glucose regulation subjects, it was reported that low-carbohydrate (carbohydrate < 45 %) breakfast improved glycaemic control( Reference Kang, Wang and Lifang 27 ).
Our previous studies( Reference Kobayashi, Ogata and Omi 31 , Reference Ogata, Kayaba and Tanaka 32 ), which used a metabolic chamber, were conducted with the same energy intake (as to our present study) per d to measure the energy expenditure; in the breakfast skipping condition, the individual ate extra energy at lunch and dinner in order to compensate for breakfast. Consequently, the overall 24-h average blood glucose and glycaemic response increased when the breakfast was omitted and stayed under the indirect calorimetry( Reference Kobayashi, Ogata and Omi 31 , Reference Ogata, Kayaba and Tanaka 32 ). In the present study, breakfast skipping resulted in hyperglycaemic response after lunch even if participants consumed the same amount of energy. However, despite the difference in the total energy intake, the 24-h average blood glucose was similar between the two-meal conditions. Thus, it was suggested that reducing the number of meal (i.e. at least breakfast skipping) has a negative effect on glucose metabolism even in healthy young individuals. Taken together, the result of the hyperglycaemic response after lunch was due to the breakfast skipping, and the increased 24-h average blood glucose was also due to the second-meal phenomenon. The present study showed that there was no significant difference in the fasting blood glucose level the day after the meal intervention day between the two conditions. The postprandial hyperglycaemia is a more sensitive early symptom of diabetes than fasting blood glucose( Reference Monnier, Colette and Boniface 72 ). Moreover, postprandial hyperglycaemia is a risk factor that is different from fasting hyperglycaemia, and it increases the risk of mortality( 73 – Reference Nakagami 76 ). The results of the present study, therefore, suggest that breakfast skipping increases the risk of lifestyle-related diseases in healthy individuals.
The dietary behaviours, such as skipping breakfast, what to eat for breakfast, how much to eat during breakfast and the macronutrient composition of breakfast, receive an increasing attention worldwide because of their significant physical, mental and cognitive effects during childhood and adolescence( Reference Nyaradi, Li and Foster 77 , Reference Burrows, Goldman and Pursey 78 ). In the present study, fatigue was slightly higher in the afternoon in the skipping breakfast condition than that in the three-meal condition. This finding suggested that a sudden increase in blood glucose level may have an influence on mentality. With regard to the rating of concentration, it was slightly lower in the skipping condition in the morning than that in the three-meal condition. This finding suggested that the omission of breakfast caused a decrease in concentration. Therefore, breakfast is important in improving not only physical performance but also mental performance.
The limitations of the present study were as follows: the sample size was small and the age of the subjects was limited.
In conclusion, a single incident of breakfast skipping increases postprandial hyperglycaemia after lunch in association with impaired insulin response in healthy young individuals. The present study shows that skipping breakfast influences glucose regulation in healthy young individuals. From the viewpoint of glucose regulation, it was suggested that it is important to eat breakfast even in healthy young individuals. Future studies should consider the chronic effects of breakfast skipping on glucose regulation and macronutrient composition of breakfast effects on glucose regulation.
Acknowledgements
We thank all the study investigators and staff and individuals who participated in the present study, especially Dr Kumpei Tokuyama.
The present study was supported by a Grand-in-Aid for Japan Society for the Promotion of Science (JSPS) Research Fellows (no. 17K179110A to H. O. and no. 18K17949 to Y. H.).
The authors’ contributions are as follows: H. O., Y. H., E. Y. and H. T. designed the experiments. H. O. conducted the experiments, performed the data analysis and wrote the manuscript. Y. H., Y. G., E. T. and E. Y. conducted the experiments. Y. H., E. Y., K. Kiyono, Y. U., K. Kawanaka and N. O. edited the manuscript. All authors read and approved the final version of the manuscript.
The authors declare that there are no conflicts of interest.