A third of all preschool-aged children living in low- and middle-income countries do not reach their cognitive developmental potential due to factors such as undernutrition and poverty(Reference McCoy, Peet and Ezzati1). Genetic and environmental factors influence cognitive development through the nature–nurture interaction(Reference Weaver2,Reference Petrill, Hewitt and Cherny3) . While genetic factors have a strong influence on brain development, environmental factors, particularly nutrition, also play a fundamental role(Reference Petrill, Hewitt and Cherny3,Reference Tucker-Drob, Briley and Harden4) . Early childhood is an important period when physiological and epigenetic changes can impact brain development(Reference Tucker-Drob, Briley and Harden4–Reference Black, Walker and Fernald6). The effect and intensity of both adverse and favourable influences during these developmental periods are dependent on the timing of their occurrence(Reference Engle, Fernald and Alderman5).
The Developmental Origins of Health and Disease hypothesis suggests that fetal growth has an impact on growth, neurodevelopment, health and disease vulnerability(Reference O’Donnell and Meaney7). The evidence is particularly strong for low birth weight and preterm children(Reference Upadhyay, Naik and Choudhary8). However, the association of birth weight with cognitive development extends beyond low birth weight since there is variability within the normal range of birth weight(Reference Andersen, Girma and Wells9). In studies among children born at term and with normal birth weight, birth weight was associated with different domains of cognitive development or intellectual quotient scores during childhood(Reference Huang, Martorell and Ren10–Reference Kumar, Kumar and Laxminarayan13) and early adolescence(Reference Richards, Hardy and Kuh12,Reference Veena, Krishnaveni and Wills14) . However, postnatal BMI shows inconsistent associations with children’s cognitive development, with some studies reporting inverse associations with perceptual reasoning, working memory and intellectual quotient scores(Reference Li, Yolton and Lanphear15), while others demonstrate no significant association across different cognitive domains or with intellectual quotient(Reference Veldwijk, Scholtens and Hornstra16,Reference Anderson, Kirkpatrick and Dolan17) . One possible explanation for this inconsistency is that BMI is not a good marker of body fat or fat-free mass (FFM)(Reference Wells18,Reference Freedman, Wang and Maynard19) .
Despite the distinct association of fat mass (FM) and FFM with children’s growth and health(Reference Wibaek, Vistisen and Girma20–Reference Abera, Tesfaye and Girma25), studies examining the relationship of FM and FFM with childhood cognitive function are scarce, particularly in low- and middle-income countries and among full-term children. Among very low birth weight preterm children, it has been shown that higher FFM, but not FM, accretion in early infancy was associated with better neurodevelopment at 1 year(Reference Ramel, Gray and Christiansen26). Another study among a similar population also revealed that FFM accretion in early infancy was associated with a higher full-scale intellectual quotient, whereas FM accretion was associated with poorer working memory at 4 years(Reference Scheurer, Zhang and Plummer27).
The Ethiopian infant anthropometry and body composition (iABC) cohort data showed wide variability of FM and FFM across the spectrum of birth weight(Reference Andersen, Girma and Wells9). From this cohort, we previously reported positive associations of FFM at birth with cognitive development at 2 years of age(Reference Abera, Tesfaye and Girma25) and developmental progression from 1 to 5 years assessed using the Denver-II Developmental Screening Test(Reference Abera, Tesfaye and Admassu24). Given the prolonged nature of brain development throughout childhood(Reference Stiles and Jernigan28), it is crucial to investigate the association of FM and FFM with cognitive function in later childhood. Therefore, we aimed to investigate the association of FM and FFM at birth and 0–5 years accretion with cognitive function at 10 years.
Methods
Study setting and participants
The study participants were recruited from Jimma Medical Center, Jimma, Ethiopia. Jimma town is located 350 km southwest of Addis Ababa, the capital city of Ethiopia. It is the largest town in southwestern Ethiopia and has a population of approximately 240 000(29). Jimma Medical Center, located in this town, serves as a referral hospital for a catchment area with about 15 million people(30).
This is a 10-year follow-up of the iABC birth cohort, initially established from December 2008 to October 2012. At enrolment, newborns and their mothers were recruited from Jimma Medical Center within 48 h after delivery. As described elsewhere(Reference Andersen, Girma and Wells9,Reference Andersen, Girma and Wells31) , the cohort included term newborns who resided in Jimma town (to ensure participation in follow-up visits), had a birth weight above 1500 g and had no congenital malformation. Mothers with their children were invited for visits, at birth, 1·5, 2·5, 3·5, 4·5 and 6 months and 4 and 5 years of child’s age. In the current follow-up visit, the children’s ages ranged from 7 to 12 years, henceforward referred to as the 10-year follow-up. Mothers/caregivers with their children were traced using their last registered phone number and address.
At enrolment, 644 mother–newborn pairs were examined. Of these, 571 children met the inclusion criteria and were followed up. At the 10-year follow-up, 355 children attended, and 318 of them had Peabody Picture Vocabulary Test (PPVT) data.
Exposure variables
Body composition measurement
FM and FFM of newborns and infants at 1·5, 2·5, 3·5, 4·5 and 6 months were measured using air-displacement plethysmography (PEA POD, COSMED). PEA POD is an infant-sized air-displacement plethysmography that measures infant body composition using a two-component densitometry model(Reference Andersen, Girma and Wells9,Reference Wibaek, Vistisen and Girma20,Reference Andersen, Girma and Wells31) .
A child/adult version of air-displacement plethysmography (BOD POD, COSMED) was used to measure body composition starting from 4 years of child’s age(Reference Wibaek, Vistisen and Girma20). A two-point calibration process, with the empty chamber and using a calibration cylinder, was done every time the BOD POD was used. Before the measurement, children were asked to remove all clothes and put on a swim cap and tight-fitting underwear. Children were also informed about the measurement to ensure relaxation. Finally, children were sat on a paediatric chair inserted in the chamber, and FM and FFM were then measured in kg by trained research nurses.
Covariables
Head circumference at birth was measured in duplicate to the nearest 0·1 cm using a non-stretchable tape. Gestational age as per the Ballard score(Reference Ballard, Khoury and Wedig32), sex of the newborn and birth order were recorded at birth. In addition, maternal age and socio-economic characteristics were collected at birth. At 10 years follow-up, height was measured in duplicate using SECA 213 (Seca). Data on the child’s current school grade were collected from school records using a questionnaire. Breastfeeding status was assessed at 4·5 and 6 months of child’s age with the following categories: exclusive (no other foods given), almost exclusive (no other foods given except water), predominant (breast milk as primary food) and partial/no (breast milk, not the primary food/not breastfeeding).
Outcome variable
Cognitive function at age 10 years follow-up was assessed using the Peabody Picture Vocabulary Test Fourth Edition (PPVT IV). PPVT assesses receptive vocabulary, an important component of general intelligence that is predictive of academic success(Reference McKinlay, Goldstein and Naglieri33,Reference Eigsti and Volkmar34) . PPVT was translated into local Ethiopian languages (Amharic and Affan Oromo) and has been used in earlier cohort studies to assess the cognitive function of Ethiopian children(Reference Fink and Rockers35,Reference Leon and Singh36) . The test was developed for individuals aged from 2·5 to 90+ years. PPVT IV is composed of 228 items, divided into nineteen sets of twelve items each. Each PPVT IV item consists of two parts. The first part consists of stimulus words (for the examiner) and a corresponding page composed of four coloured pictures (for the examinee). The test requires the child to choose one of the four items (pictures) displayed on a test card illustrating the word spoken by the examiner. The test items are arranged from left to right in an increasing order of difficulty. The test procedure starts with age-appropriate test items/sets. Then the child is tested for items/sets arranged to the left of the start set until the child makes one or zero error (basal set) and the test continues to the right of the start set until the child makes eight or more errors (ceiling set).
Prior to data collection, research nurses were trained on how to administer PPVT, which was conducted in a private room. Ninety-seven participants were identified as having examination errors, where their testing was terminated before the ceiling set was established. Subsequently, we re-examined these children at their homes. Place of test administered was categorised as home or facility administered and controlled in all regression models.
Statistical analysis
Data were double-entered in EpiData version 4.4.2.0 and exported to Stata version 17 (StataCorp LLC) for further cleaning and analysis. Descriptive results were presented as mean and standard deviation (sd) for normally distributed continuous data and count (percent) for categorical variables. The wealth index was computed from self-reported ownership of twelve material assets: car, motorcycle, bicycle, electric stove, refrigerator, mobile phone, land, telephone, television, radio, access to electricity, source of drinking water and type of latrine. Principal component analysis was used to compute the wealth index, and the first component was grouped into wealth quintiles(37) and used in subsequent analyses. Grade-for-age was computed based on the United Nations Educational Scientific and Cultural Organization criteria(38). Height-for-age Z-score (HAZ) at 10 years was computed using the WHO Reference 2007 STATA macro package(39). To ensure comparability in the model estimates, FM and FFM measurements, as well as the PPVT score, were standardised.
Association between body composition and cognitive function
Individual growth measurements at consecutive time intervals might be correlated. Thus, conditional growth modelling was used to assess the association of postnatal FM and FFM accretion over selected time periods from 0 to 5 years of age with cognitive function at 10 years of age. However, conditional growth modelling requires participants to have complete data at all time points, which reduces the sample size due to the exclusion of individuals with missing observations at different time points(Reference Tu, Tilling and Sterne40). Thus, three analytical steps were carried out in this study. In the first step, we predicted FM and FFM data using linear spline mixed effect modelling using R statistical software version 4.2.2 (R Foundation for Statistical Computing). Linear spline mixed effect modelling assumes the child to have a linear pattern of growth within a set of pre-defined knot points and different rates of growth across sets of knot points(Reference Howe, Tilling and Matijasevich41). We determined knot points based on a previous study from this cohort(Reference Wibaek, Vistisen and Girma20) and the Akaike and Bayesian information criterion. Finally, knot points at 3, 6, 48 and 60 months were selected. Children having at least three measurements were included in linear spline mixed effect modelling (one at birth, one measurement from 0 to 6 months and one measurement from 4 to 5 years of age).
In the second step, using the estimated FM and FFM data, we computed conditional FM and FFM accretion from 0 to 3 months, 3 to 6 months, 6 months to 4 years and 4 to 5 years. Conditional growth modelling produces statistically independent conditional estimates, which represent the difference between the actual growth and expected growth over a specific period, based on the prior FM and FFM z-scores(Reference Tu, Tilling and Sterne40). These estimates will be referred to as accretions. Positive values indicate that the child grew faster than expected, while negative values indicate that the child grew slower than expected based on standardised previous measurements.
In the third step, multiple linear regression analyses were used to assess the association of FM and FFM accretion at 0–5 years with cognitive function at 10 years in separate models. Model 1 included age at the 10-year follow-up, sex and place of test. Model 2 was additionally adjusted for child characteristics at birth (head circumference, gestational age, birth order), HAZ and academic grade at 10 years follow-up. Model 3 was further adjusted for maternal characteristics: wealth index, maternal age and maternal education. Covariables were selected based on related literature(Reference Abera, Tesfaye and Admassu24,Reference Abera, Tesfaye and Girma25,Reference Krishnaveni, Veena and Srinivasan42) . Separate models were fitted for FM and FFM accretions. Since accretions computed from conditional growth modelling are uncorrelated(Reference Tu, Tilling and Sterne40), they were included simultaneously in a regression model. As an example, the FM accretion model was specified as f(cognitive function) = FM0–3months + FM3–6months + FM6–48months + FM48–60months + sex + …… + covariableN.
Similar models were built to assess the association of birth FM and FFM with cognitive function. For example, model for FFM at birth was specified as f(cognitive function) = FFMat birth + sex + …… + covariableN. Model assumptions were checked: normal distribution of residuals was visually examined using pnorm and qnorm plots; homoscedasticity was visually checked by plotting residuals against fitted values. Multicollinearity between exposure variables was assessed using variance inflation factor.
As a sensitivity analysis, we also assessed the association between conditional FM and FFM accretion with PPVT score using observed FM and FFM measurements. In addition, since data of school type had a large number of missing, we ran sensitivity analyses and adjusted school type (private v. government) in models 2 and 3. Since we had only limited breastfeeding data, it was excluded from the main analysis. Instead, we conducted sensitivity analyses to account for breastfeeding status at 4·5–6 months of the child’s age.
Results
Participant characteristics
A total of 644 children were enrolled at birth, of whom seventy-three were excluded: ten because they were preterm and sixty-three because they lived outside Jimma town. Among 571 children recruited to the iABC cohort, 318 had PPVT score data at 10 years (Fig. 1). These children were not different (all P> 0·05) from those who did not have PPVT data with respect to sex, birth characteristics (gestational age, length, weight, FM, FFM), wealth status, maternal height and maternal education. However, those who had PPVT data at 10 years were more likely to be firstborns and have younger mothers (online Supplementary Table 1). The mean (sd) age of children at the 10-year follow-up was 9·8 (1·0) years and ranged from 7 to 12 years. The mean (sd) HAZ score at 10 years was −0·7 (0·9). The mean (sd) maternal age at the time of birth was 24·8 (4·7) years. At the child’s birth, 214 (61·1 %) of mothers reported having attained primary education, and 66 (18·9 %) had attained secondary education (Table 1). The mean (sd) PPVT score was 184 points (40).

Figure 1. Flow diagram of the study participants. LSMEM, linear spline mixed effect modelling; PPVT, Peabody Picture Vocabulary Test.
Table 1. Socio-demographic characteristics of children attending the 10-year follow-up* (Numbers and percentages; mean values and standard deviations)
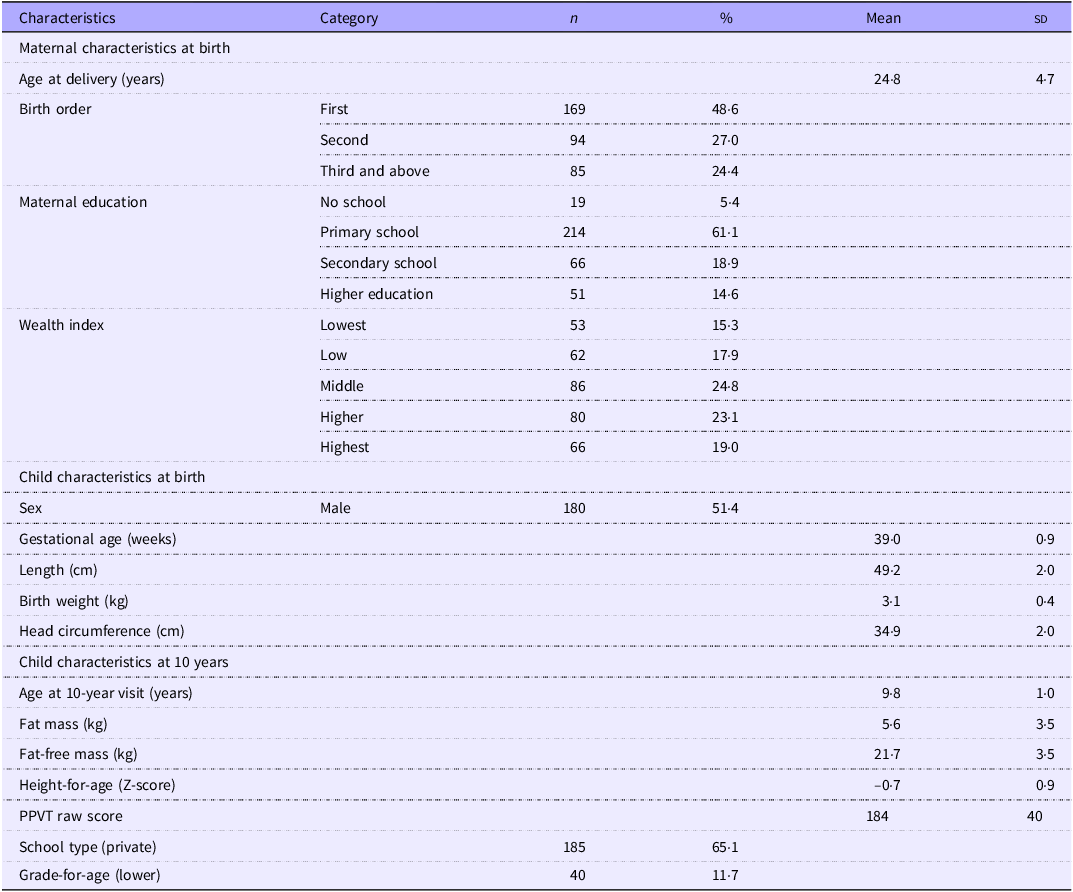
PPVT, Peabody Picture Vocabulary Test.
* Values are expressed as mean (sd) for continuous variables and as n (%) for categorical variables.
Among children who attended the 10-year follow-up, males had higher mean FFM at birth than females (2·9 v. 2·8, P< 0·001). Similarly, FFM was different between males and females up to 6 months (P< 0·001). However, FM was not different between males and females from birth to 5 years (P> 0·05) (Table 2).
Table 2. Fat mass and fat-free mass by sex and age (n 318) (Mean values and 95 % confidence intervals)
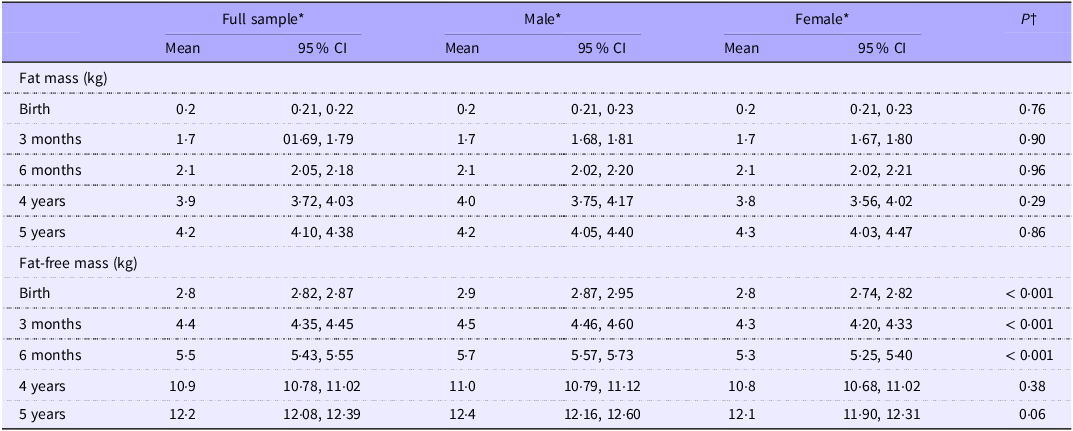
* Data are mean (95 % CI).
† Independent sample t test.
Association of fat mass and fat-free mass at birth with cognitive function at 10 years
FFM at birth was associated with a higher PPVT score at 10 years (Fig. 2 and online Supplementary Table 2). A 1 sd (1 sd = 0·3 kg) higher FFM at birth was associated with a 0·14 sd (95 % CI 0·01, 0·28) or 5·6 points higher PPVT at 10 years. Across all models, FM at birth was not associated with PPVT score at 10 years, and the coefficients were close to zero (Fig. 2 and online Supplementary Table 2).
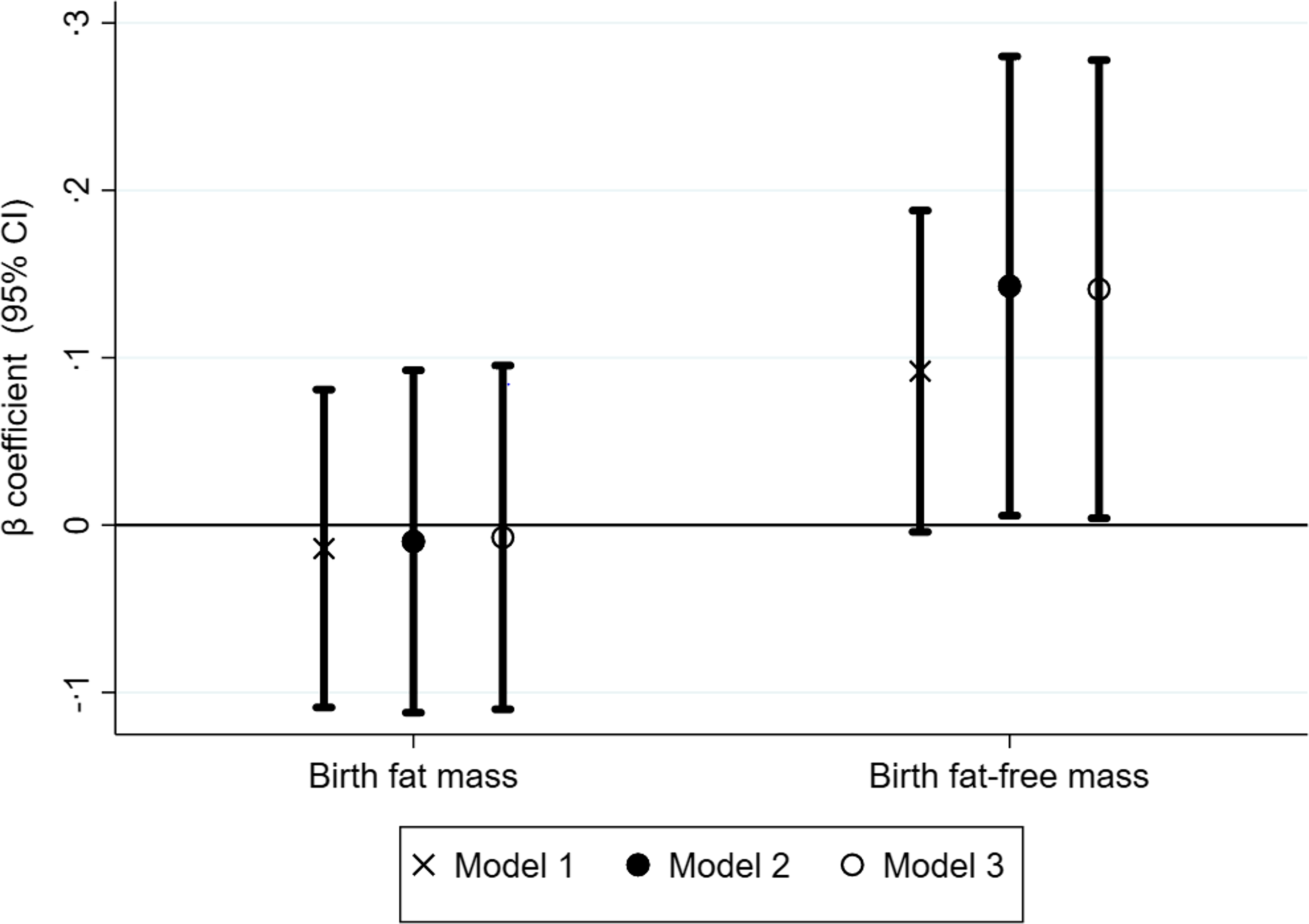
Figure 2. Association of birth fat-free mass and fat mass with cognitive function at 10 years. The Y-axis shows β coefficients from linear regression models with 95 % confidence intervals. Model 1 was adjusted for age, sex and place of test. Model 2 was further adjusted for head circumference at birth, birth order, gestational age, height-for-age Z-score at 10 years and academic grade at 10 years. Model 3 was further adjusted for maternal educational status, maternal age at childbirth and wealth index.
Association of fat mass and fat-free mass accretion from 0 to 5 years with cognitive function at 10 years
Higher FFM accretion from 0 to 3 months was positively associated with PPVT scores (β = 0·5, 95 % CI 0·08, 0·93), whereas FFM accretion from 3 to 6 months was negatively associated with PPVT scores (β = −0·48, 95 % CI −0·90, −0·07). For instance, a 1 sd higher (1 sd = 0·43 kg) FFM 0–3 months was associated with a 0·50 sd (20 points) higher PPVT score, whereas 1 sd higher (1 sd = 0·53 kg) FFM 3–6 months was associated with −0·48 sd (19·2 points) lower PPVT score. After 6 months, all effect sizes were close to zero, and the association was non-significant (Fig. 3(a) and online Supplementary Table 3). FM accretion from 0 to 5 years was not associated with cognitive function at 10 years of age (Fig. 3(b) and online Supplementary Table 3).
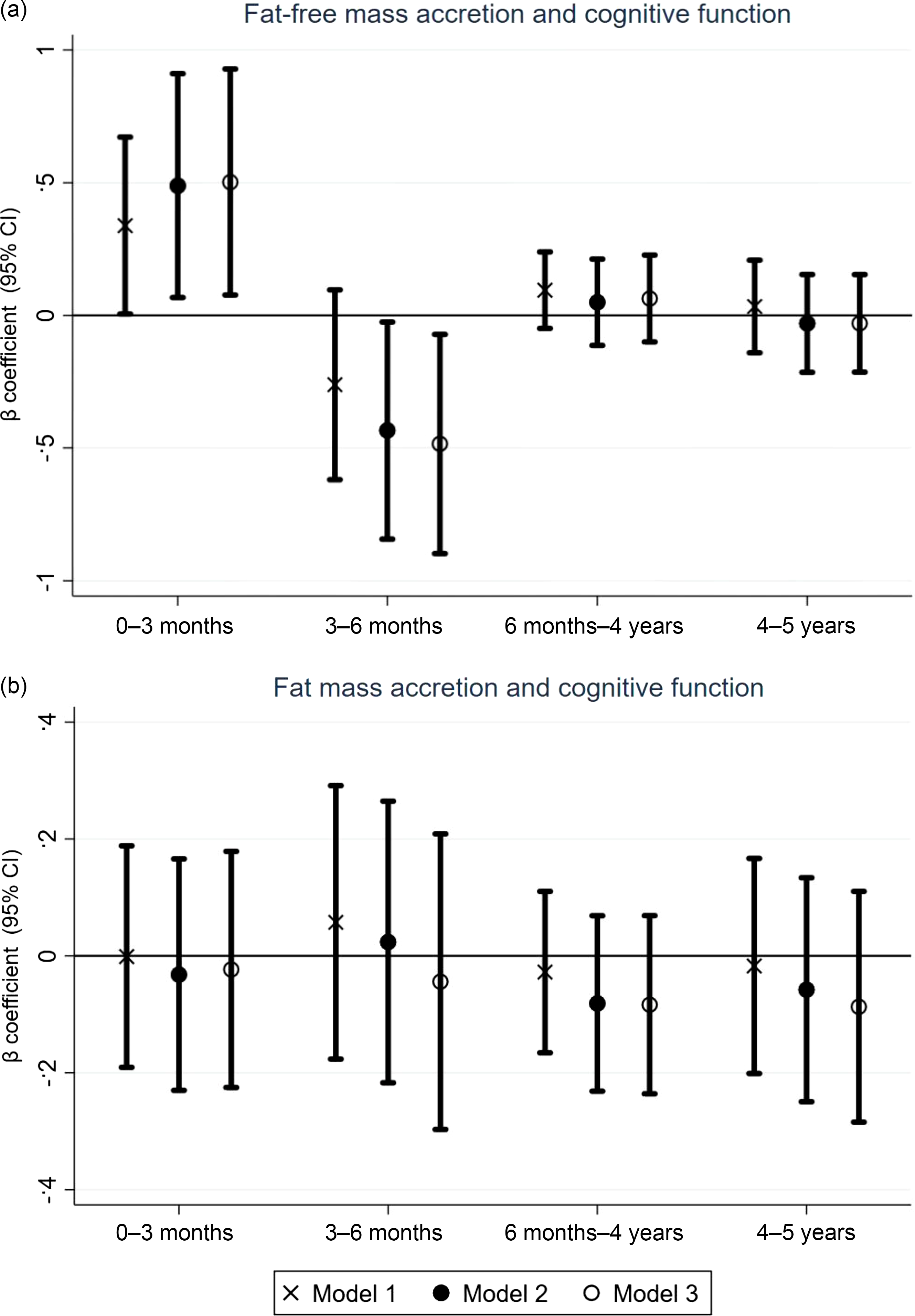
Figure 3. (a) Association of fat-free mass accretion from 0 to 5 years with cognitive function at 10 years. The Y-axis shows β coefficients from linear regression models with 95 % confidence intervals. Model 1 was adjusted for age, sex and place of test. Model 2 was further adjusted for head circumference at birth, birth order, gestational age, height-for-age Z-score (HAZ) at 10 years and academic grade at 10 years. Model 3 was further adjusted for maternal educational status, maternal age at childbirth and wealth index. (b) Association of fat mass accretion from 0–5 years with cognitive function at 10 years. The Y-axis shows β coefficients from linear regression models with 95 % confidence intervals. Model 1 was adjusted for age, sex and place of test. Model 2 was further adjusted for head circumference at birth, birth order, gestational age, HAZ at 10 years and academic grade at 10 years. Model 3 was further adjusted for maternal educational status, maternal age at childbirth and wealth index.
In the sensitivity analysis, the estimates did not change markedly with the models using the observed data and in school type adjusted models (online Supplementary Tables 4 and 5). The sensitivity analysis adjusting for breastfeeding status at 4·5–6 months of child’s age yielded comparable effect sizes, though the level of significance changed (online Supplementary Table 6). We also ran another sensitivity analysis excluding the measurements taken at 10 years (age and height at 10 years), and the P-value pattern remained similar, with only some changes in effect size.
Discussion
We examined the relationship between birth and early childhood FM and FFM accretion with cognitive function at 10 years of age using a prospective birth cohort with accurate FM and FFM measurements. Birth FFM, but not FM, showed a significant positive association with cognitive function at 10 years. Higher FFM accretion from 0 to 3 months had a positive association with cognitive function, whereas FFM accretion from 3 to 6 months showed a negative association. For growth periods after 6 months, the effect sizes became negligible, and the association was not significant. FM accretion from 0 to 5 years showed no significant association with cognitive function at 10 years.
This finding of an association between birth FFM and cognitive function is consistent with our previous study from this cohort(Reference Abera, Tesfaye and Admassu24,Reference Abera, Tesfaye and Girma25) . Notably, FFM at birth was associated with higher global and language development at 2 years of age(Reference Abera, Tesfaye and Girma25), as well as with favourable global developmental progression from 1 to 5 years of age(Reference Abera, Tesfaye and Admassu24). Similarly, FFM at birth showed a positive association with cognitive outcomes among term-born Indian children(Reference Krishnaveni, Veena and Srinivasan42). The enduring positive association between FFM at birth and cognitive function observed at 10 years highlights the long-term and continued impact of fetal FFM on cognitive development and function throughout childhood. However, we cannot rule out that the small effect size seen in this association could be due to residual confounding. Fetal brain development encompasses processes of neural cell production, migration and differentiation, which are predominantly protein-dependent processes and protein is the building block of FFM(Reference Stiles and Jernigan28). This might explain the close-to-zero effect size and non-significant association between FM at birth and cognitive function in this study. The lack of association of FM at birth is similar to our previous findings at 2 years(Reference Abera, Tesfaye and Girma25) and developmental progression from 1 to 5 years of this cohort(Reference Abera, Tesfaye and Admassu24).
As evidenced by other studies, having a larger brain at birth is also associated with late childhood cognitive function(Reference Veena, Krishnaveni and Wills14,Reference Heinonen, Räikkönen and Pesonen43) . In the present study, the association between birth FFM and cognitive function persisted after head circumference was adjusted. This suggests that there might be different pathways to the association between FFM and cognitive function beyond the association of higher FFM with higher fetal brain size.
FFM accretion from 0 to 3 months was positively associated with cognitive function at 10 years, whereas FFM accretion from 3 to 6 months was negatively associated. The effect sizes were relatively large in these periods, suggesting a potentially important role of FFM accretion in cognitive function during this timeframe. Infant growth within the first 3 months of life can include rapid growth after birth(Reference Davies, Platts and Pritchard44). During this period, much of FFM accretion may contribute to brain growth. In a previous study of this cohort, it was found that nearly 55 % of infants were born with higher FFM, followed by a distinct FFM growth trajectory with a quadratic shape during the first 6 months(Reference Andersen, Wibaek and Kaestel45), which might indicate a catch-down pattern. This might explain the varying pattern of association seen in this study during early infancy. Currently, we have no explanation for the negative association of higher FFM from 3 to 6 months and cognitive function, and further research is needed to fully elucidate the underlying mechanisms.
We found no association between FFM accretion and cognitive function beyond infancy. Nutrition and environmental stimulation are essential and complementary, each playing a distinct yet interconnected role in shaping the developing brain(Reference Walker, Powell and Grantham-McGregor46). Postnatally, the brain undergoes a period of plasticity, where experiences also play an essential role in shaping its neural organisation, brain development and function. Greenough(Reference Greenough, Black and Wallace47) termed this process experience-dependent brain development. This might be the reason for the absence of an association and close-to-zero effect sizes, especially after 6 months.
The present study has the strength of being a birth cohort that followed children from birth to 10 years of age. Air-displacement plethysmography was used to measure FM and FFM, which is an accurate method of measuring body composition(Reference Ellis, Yao and Shypailo48). We used conditional growth modelling, which allowed us to include all the accretion periods together in one model separately for FM and FFM and enabled us to control the effect of tissue accretion at different time points. There are, however, some limitations to this study, which should also be taken into account when interpreting this result. Ninety-seven children were re-tested in their homes due to examination errors. Differences in places of testing might result in systematic differences in test scores between those who were examined at home and those who were examined at a facility; however, we adjusted for place of test in all analyses. There was a loss to follow-up at the 10-year visit. However, those lost to follow-ups and those included in the analysis were similar in terms of sex, length at birth, gestational age, birth weight, birth FM, birth FFM, wealth status, maternal height and maternal education, although children included in this analysis were more likely to be firstborns and had younger mothers. Due to air-displacement plethysmography validation and children’s inability to sit still inside BODPOD, we did not measure FM and FFM from 6 months to 4 years. These missed periods might have given additional insights regarding associations between early childhood growth and cognitive function. We also did not have comprehensive data on infant feeding, which might be an important variable to be considered in this analysis. Furthermore, the evidence from this cohort is representative of children born at health facilities but might not reflect the general population characteristics, as the participants were only newborns delivered at the institution.
In conclusion, FFM rather than FM at birth showed a significant association with cognitive function at 10 years. The observed association highlights that optimal maternal and fetal nutrition during pregnancy contributes to establishing a strong foundation for cognitive function, paving the way for continued cognitive function throughout late childhood, which will also impact children’s academic achievement and future success. FFM accretion during infancy had a distinct and potentially more pronounced effect size compared with other developmental stages. The results of this study underscore the need for further investigations to understand the mechanism of these associations seen during infancy.
Acknowledgements
We extend our sincere gratitude to the research staff for their unwavering dedication. We are also deeply grateful to the study participants for their time and valuable contributions. Special thanks go to Gregers S Andersen for his invaluable work in establishing the cohort. We also acknowledge Melkamu Berhane, who was leading the iABC cohort, and Dorothea Nitsch’s unreserved support during manuscript preparation.
GSK Africa Non-Communicable Disease Open Lab (project no. 8658) funded the study. The funders had no role in the study design, data collection, analysis and decision to publish or preparation of the manuscript.
The authors’ responsibilities were as follows: H. F., J. C. K. W., S. F., T. G., R. W., D. Y., M. F. O., M. A. and R. A. designed the study; R. A., B. Z. and B. S. M. supervised the data collection; H. F., J. C. K. W., S. F., R. W., M. F. O., M. A. and R. A. participated in methodology; R. A. analysed the data and interpreted the findings. R. A. wrote the first draft. B. K., B. S. M., D. Y., T. G., B. A., S. F., H. F., J. C. K., A. A. M., M. F. O., R. W. and M. A. commented on the manuscript, contributed for manuscript revisions, read the final manuscript and approved it for submission.
The authors declare no conflicts of interest.
This study was conducted according to the guidelines laid down in the Declaration of Helsinki, and ethical clearance was obtained from the Jimma University Ethical Review Board of the College of Public Health and Medical Sciences (reference IHRPHD/333/18) and the London School of Hygiene and Tropical Medicine (reference 15076). Written informed consent was obtained from all mothers or caregivers.
Supplementary material
For supplementary material/s referred to in this article, please visit https://doi.org/10.1017/S0007114524002605