Modern approaches to nutrition and health research are continuing to evolve, with the past two decades witnessing continuous advances in technologies and a move towards larger multidisciplinary and multi-centred trials. These contemporary advances in research include systems biology approaches such as metabolomics, which provides a comprehensive assessment of metabolites in various biological samples. Metabolomics provides an insight into the metabolic changes within biological fluids, which are most closely related to metabolic end points or phenotypic features. The technique includes the use of NMR spectroscopy and/or MS coupled with GC or liquid chromatography. The applications of metabolomics in nutrition research are increasing annually and can be classified into two major areas: assessment of the broad metabolic response to dietary interventions and the discovery of novel metabolic markers of dietary intake( Reference Wittwer, Rubio-Aliaga and Hoeft 1 ). Metabolome-wide association studies are also emerging as a valuable approach in generating hypotheses related to novel disease biomarkers( 2 , Reference Nicholson, Holmes and Elliott 3 ).
Larger sample sizes, more diverse population groups and the availability of a wider range of expertise and analytical techniques are among the advantages of multi-centred studies. In addition, the ability to compare results across centres can provide a deeper understanding and allow the applicability of research findings across populations and geographical regions. If metabolomics is to be successfully applied in such multi-centred nutrition research, it is essential that the possibility of confounding influences on metabolic profiles, such as variation in the methods of sample collection and handling and different genetic, environmental, and ethnic or cultural backgrounds across centres, be explored. Diurnal variation, age, sex and recent diet have previously been reported to have an impact on the stability of urinary metabolic profiles in healthy individuals( Reference Walsh, Brennan and Malthouse 4 ).
By taking measures to standardise methodologies in metabolomic studies, it may be possible to minimise the unwanted effects of variation that could jeopardise the interpretation of metabolic data or the identification of relevant biomarkers( Reference Scalbert, Brennan and Fiehn 5 ). Factors related to regional differences between human populations are naturally more challenging to standardise compared with analytical techniques. Thus, it is important to investigate such influences to improve the interpretation and understanding of results in multi-centred research. The impact of geographical region on metabolomic samples has previously been assessed as part of the INTERMAP epidemiology study (an international population study on macronutrients and blood pressure)( Reference Dumas, Maibaum and Teague 6 – Reference Yap, Brown and Chan 9 ). The study showed distinct grouping of samples from China, Japan and two Western populations on the basis of principal component analysis (PCA) of their urinary metabolic profiles( Reference Holmes, Loo and Stamler 7 ). Specific combinations of biomarkers were detected between the three groups, related to genetic, dietary and gut microbial factors. The study also showed discrimination between north and south Chinese populations, but no differentiation between samples from the USA and the UK. These findings indicate that the urinary metabolic profiles across Western populations are similar; however, to our knowledge, regional differences in metabolic profiles across European Union populations are yet to be explored extensively. One study has reported differences in urinary profiles between British and Swedish subjects, thought to result from higher fish consumption by Swedish subjects reflected by elevated trimethylamine oxide excretion( Reference Lenz, Bright and Wilson 10 ).
The NUGENOB (Nutrient-Gene Interactions in Human Obesity) study (www.nugenob.org), a nutrigenomic investigation of obesity, included the metabolomic analysis of plasma samples from across eight European Union cities. The samples were used to test the validation and assessment of machine learning using various data modelling techniques( Reference Pers, Albrechtsen and Holst 11 ), but no assessment of regional differences in metabolomic profiles was conducted for that publication.
The present study was carried out on a subset of samples from a European Union Sixth Framework Programme-integrated project called LIPGENE (www.ucd.ie/lipgene/). The LIPGENE project used a multidisciplinary approach to examine how variation in dietary fat types interacted with common genetic variations to influence the development of the metabolic syndrome( Reference Phillips, Goumidi and Bertrais 12 ) and also included a metabolomic substudy on biofluid samples from seven laboratories across Europe. The aim of the present study was to assess the effects of geographical location on the metabolic profiles of individuals with the metabolic syndrome.
Experimental methods
The LIPGENE cohort included a total of 486 subjects, all with the metabolic syndrome. Details of the recruitment process have been described previously( 13 ). In the present study, an analysis was carried out on the baseline samples of seven of the participating centres, representing a subset of the LIPGENE cohort, before the start of the dietary intervention. Subjects were recruited in Dublin, Ireland; Reading, UK; Maastricht, The Netherlands; Uppsala, Sweden; Oslo, Norway; Córdoba, Spain; and Marseille, France, based on the condition that they provided an additional urine sample for metabolomic analysis. The diagnosis of the metabolic syndrome required the fulfilment of three or more of the following criteria: fasting blood glucose concentrations of 5·5–7·0 mmol/l; serum TAG concentrations ≥ 1·5 mmol/l; serum HDL-cholesterol concentrations < 1·0 mmol/l in men and < 1·3 mmol/l in women; blood pressure ≥ 130/85 mmHg; a waist circumference >102 cm in men and >88 cm in women. Exclusion criteria were the presence of diabetes or other endocrine disorders, chronic inflammatory conditions, kidney or liver dysfunction, and Fe-deficiency anaemia (Hb levels < 120 g/l in men and < 110 g/l in women), use of prescribed hypolipidaemic or anti-inflammatory medications, consumption of fatty acid supplements, antioxidant vitamins, red rice yeast or greater than two servings of oily fish per week, and intense exercise training more than three times per week. The subjects arrived at the study centre following an overnight fast. The assessments of height, weight, waist and hip circumferences, nutrient intakes, blood pressure, and blood lipoprotein, fatty acid, glucose and insulin levels and metabolomic analyses of urine were performed by trained members of the LIPGENE consortium. The subjects were instructed to collect a sample of their midstream first-void urine on the mornings of their pre-intervention assessment. They were provided with insulated ice packs to store the samples immediately until the study investigator collected them. Metabolomic samples were centrifuged at 2500 g for 10 min at 4°C to remove any suspended solids from the urine. Detailed information on the LIPGENE project and standard operating procedures used in data collection can be obtained, with permission, at www.ucd.ie/lipgene/. The present study was conducted according to the guidelines laid down in the Declaration of Helsinki, and all procedures involving human subjects were approved by the ethics committees at each centre. Written informed consent was obtained from all the subjects.
Dietary intake
All the subjects completed 3 d weighed food diaries, including one weekend day and two weekdays( Reference Shaw, Tierney and McCarthy 14 ). Nutrient intakes were assessed using various dietary analysis programmes that incorporated foods specific to each country (Ireland: WISP version 3.0; UK: Food Base version 3.1; The Netherlands: Komeet and Orion; Sweden: MAT; Norway: Kostberegning system; Spain: Dietsource version 2.0; France: Nutrilog).
1H NMR spectroscopy
Urine samples were defrosted for 1 h at room temperature. Samples for NMR analyses were prepared by the addition of 200 μl phosphate buffer (0·2 m-KH2PO4 and 0·8 m-K2HPO4) to 500 μl urine. Following centrifugation at 8000 g for 5 min, an aliquot of 500 μl was removed and 10 μl sodium trimethylsilyl [2,2,3,3-2H4]propionate (TSP) and 50 μl 2H2O were added. Spectra were acquired on a 500 MHz DRX NMR spectrometer (BrukerBiospin) using the first increment of a NOSEY (nuclear Overhauser effect spectroscopy) pulse sequence at 25°C. Typically, spectra were acquired with 32K data points and 128 scans over a spectral width of 9 kHz. All 1H NMR urine spectra were referenced to TSP at 0·0 parts per million (ppm) and processed manually with AMIX with a line broadening of 0·2 Hz. The spectra were integrated into bins consisting of spectral regions of 0·04 ppm using AMIX. The water region (4·0–6·0 ppm) was excluded, and the data were normalised to the sum of spectral integrals.
Statistical analysis
The demographic characteristics, dietary intake data and plasma biochemistry results were analysed using SPSS for Windows (version 18; SPSS, Inc.). Differences between the three geographical regions (as determined by the metabolomic output) were assessed using ANOVA, controlling for sex and with appropriate post hoc tests. Differences were considered significant at P< 0·05.
The 1H NMR metabolomic data were examined with multivariate data analysis using SIMCA-P+ (version 10.0; Umetrics). The urine data were analysed following the application of Pareto scaling. PCA, an unsupervised pattern recognition technique and hierarchical cluster analysis were applied to each dataset. This initial step was used to explore the data for outlying samples and data trends. Any trends in the data such as differences between the groups were further explored using partial least-squares discriminant analysis (PLS-DA). The quality of each model was judged by its R 2 value (goodness-of-fit parameter, the fraction of total variation represented by the model) and Q 2 value (the predictive ability parameter, the fraction of total variation predicted by the model). Additionally, permutation testing was performed on each PLS-DA model (with twenty permutations) to assess the validity of the model. Permutation testing was performed in SIMCA-P+.
PCA and PLS-DA were also applied to the plasma fatty acid data. The NMR and fatty acid data were combined and analysed by block scaling the NMR data (Pareto scaling for NMR data and no scaling for the fatty acid data).
Results
For the present metabolomic substudy, 219 (108 males; 111 females) volunteers from the European Union Framework Programme 6 LIPGENE study, aged 34–70 years, provided samples. The demographic characteristics of all the subjects are given in Table 1. There were no significant differences in age, BMI, systolic blood pressure or homeostasis model assessment of insulin resistance (HOMA-IR) index between the males and females. Diastolic blood pressure (males: 87 mmHg; females: 83 mmHg) and waist:hip ratio (males: 1·02 cm; females: 0·91 cm) were significantly different between the males and females (P= 0·002 and P⩽0·001, respectively).
Table 1 Demographic characteristics of male and female subjects from the LIPGENE cohort that provided metabolomic samples† (Mean values and standard deviations)
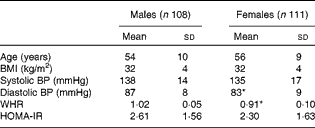
BP, blood pressure; WHR, waist:hip ratio; HOMA-IR, homeostasis model assessment of insulin resistance.
* Mean value was significantly different from that of male subjects (P< 0·05).
† Differences between the groups were assessed using independent t tests.
Multivariate data analysis of urinary 1H NMR metabolomic samples and plasma fatty acids
The initial PCA score plot of the urinary 1H NMR metabolomic samples is shown in Fig. 1, indicating a tendency for centres to group in particular regions of the score plot. The samples were subsequently analysed using a hierarchical cluster analysis, and closer inspection revealed that the clustering of centres correlated with their geographical region (Supplementary data, available online). Cluster 1 was predominantly composed of samples from Uppsala and Oslo, cluster 2 was predominantly composed of samples from Córdoba and Inserm, and cluster 3 was a mixture of the remaining centres, with Dublin being the main contributing centre. The following three European regions were identified: northwest (NW: Dublin, Reading and Maastricht); northeast (NE: Uppsala and Oslo); southwest (SW: Córdoba and Inserm). Plasma fatty acid concentrations (Supplementary data, available online) were also subjected to PCA (data not shown), and a trend similar to that observed for the metabolomic urine data was observed. A stable PLS-DA two-component model (R 2 X: 0·32, R 2 Y: 0·63, Q 2: 0·60, Q 2 intercept for permutation testing: 0·0, − 0·285) of the three geographical regions was constructed for the plasma fatty acid data (Fig. 2(a)). A similar trend was observed for the PLS-DA model (R 2 X: 0·33, R 2 Y: 0·53, Q 2: 0·39, three-component model) of the urine data (Fig. 2(b)), and the key metabolites responsible for the separation are listed in Table 2 as defined by the Variable Importance in Projection scores of the PLS-DA model. The validation of the model was performed using permutation testing, and this resulted in a Q 2 intercept of (0·0, − 0·127). The combined dataset (plasma fatty acids and urine) also demonstrated a clear separation between the three regions (Fig. 3), following PLS-DA (R 2 X: 0·30, R 2 Y: 0·58, Q 2: 0·45, three-component model). Validation using permutation testing resulted in a Q 2 intercept of (0·0, − 0·26). The NW group was characterised by higher levels of urinary hippurate and N-methylnicotinate. The NE group was characterised by higher levels of urinary creatine and citrate and plasma EPA. The SW group was characterised by higher levels of urinary trimethylamine oxide and lower levels of plasma EPA.

Fig. 1 Principal component (PC) analysis score plot of urinary metabolomic profiles from all the seven participating centres of the LIPGENE study. Each centre is represented by its first letter and a colour: Dublin, Ireland (D; ); Reading, UK (R; ■); Maastricht, The Netherlands (M;
); Uppsala, Sweden (U;
); Oslo, Norway (O;
); Córdoba, Spain (C;
); Inserm, France (I;
). PC1 and PC2 explain 16·4 and 10·4 %, respectively, of the variance in data.

Fig. 2 Partial least-squares discriminant analysis score plot of the three geographical groups in Europe: (a) plasma fatty acids and (b) urine. Each group is represented by the following symbols: northwest, ; southwest,
; northeast, ■. (a) R
2
X: 0·32, R
2
Y: 0·63, Q
2: 0·60; (b) R
2
X: 0·33, R
2
Y: 0·53, Q
2: 0·39.
Table 2 Metabolomic differences in urinary biomarkers across the three geographical groups*
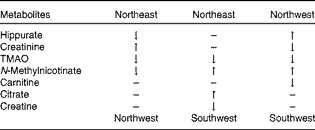
TMAO, trimethylamine oxide.
* Differences observed when the top group was compared with the bottom group.

Fig. 3 Partial least-squares discriminant analysis score plot of combined urine and plasma fatty acid data for the three geographical groups in Europe. Each group is represented by the following symbols: northwest, ; southwest,
; northeast, ■. (a) R
2
X: 0·30, R
2
Y: 0·58, Q
2: 0·45.
Demographics and clinical biochemistry for the three geographical groups
Table 3 summarises data for age, BMI, blood pressure and plasma biochemistry (mean ± sd) for the three geographical regions. One-factor ANOVA showed no significant differences in age, systolic blood pressure, waist:hip ratio, HOMA-IR index and plasma concentrations of insulin, TAG, total cholesterol, LDL-cholesterol and HDL-cholesterol between the three groups. BMI was 6 % lower in the NW group and 9 % lower in the NE group compared with the SW group, but that in the NW and NE groups was not significantly different. Diastolic blood pressure was 3 % lower in the NW group and 7 % lower in the SW group compared with the NE group, but that in the NW and SW groups was not significantly different. Blood glucose levels were 8 % lower in the NW group and 3 % lower in the NE group compared with the SW group, but those in the NW and NE groups were not significantly different. TAG-rich lipoprotein levels were 36 % higher in the NW group compared with the SW group, and neither of these groups was significantly different from the NE group. TAG-rich lipoprotein–cholesterol levels were 42 % higher in the NW group and 32 % higher in the NE group compared with the SW group, but those in the NW and NE groups were not significantly different.
Table 3 Age, anthropometry, blood pressure and plasma biochemistry data for the three geographical groups§ (Mean values and standard deviations)
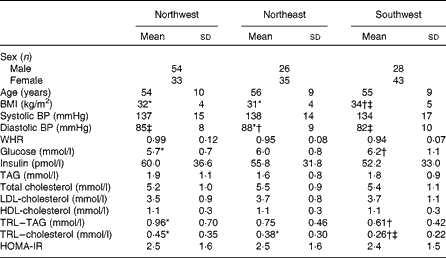
BP, blood pressure; WHR, waist:hip ratio; TRL, TAG-rich lipoprotein; HOMA-IR, homeostasis model of assessment of insulin resistance.
* Mean value was significantly different from that of the southwest group (P< 0·05).
† Mean value was significantly different from that of the northwest group (P< 0·05).
‡ Mean value was significantly different from that of the northeast group (P< 0·05).
§ Differences between the groups were assessed using ANOVA, controlling for sex with Scheffe's post hoc tests for variables showing significance.
Nutrient intake for the three geographical groups
Nutrient intake data (mean ± sd) for the three geographical regions are summarised in Table 4. Only those subjects who completed the food records adequately were included in the present analysis (n 213). Data on fatty acid intake were available only for 161 subjects. When controlling for sex, ANOVA showed no significant differences in protein intake across the three groups. However, statistical analysis showed that the NW group had a significantly (P= 0·009) higher energy intake compared with both the NE and SW groups. The SW group had lower intakes of carbohydrate (P≤ 0·001) and PUFA (P= 0·001), but higher intakes of total fat and MUFA (both P≤ 0·001) compared with both the NW and NE groups. Saturated fat intake was similar in both the NW and SW groups (12 % of total energy), and although statistically significant, it was just 1 % higher in the NE group (13 % of total energy). Long-chain n-3 fatty acid, long-chain n-6 fatty acid and fibre intakes were all significantly (all P≤ 0·001) lower in the NW and SW groups compared with the NE group. There were no significant differences in these nutrient intakes between the NW and SW groups.
Table 4 Nutrient intakes for the three geographical groups§ (Mean values and standard deviations)
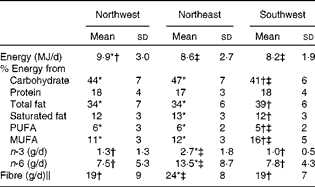
* Mean value was significantly different from that of the southwest group (P< 0·05).
† Mean value was significantly different from that of the northeast group (P< 0·05).
‡ Mean value was significantly different from that of the northwest group (P< 0·05).
§ Differences between the groups were assessed using ANOVA, controlling for sex with Scheffe's post hoc tests for variables showing significance.
∥ Calculated using the method of the Association of Official Analytical Chemists.
Discussion
With the progression of nutrition research, metabolomics will undoubtedly continue to be employed in large multi-centred studies. To date, urine has been a commonly used biofluid in metabolomic studies and is suitable for large cohorts because it is non-invasive and easy to collect. The present study indicates that geographical location had an impact on the urinary 1H NMR metabolic profiles across a European population, with differentiation between groups from the NW, NE and SW regions. The use of metabolomics to identify potentially important metabolites related to a disease or an intervention may become easier when sources of sample variation can be identified and controlled for in future analyses. Otherwise, a strong variation can dominate the differentiation between samples, causing minor yet important signals to be missed.
In the present study, initial PCA of the urinary 1H NMR metabolomic data showed that samples from each of the seven centres grouped closely together in the score plot. The fact that centres from similar geographical regions were located in proximity to each other indicated that the differences were not just centre specific and that geographical location in Europe influenced urinary metabolic profile characteristics. Holmes et al. ( Reference Holmes, Loo and Stamler 7 ) indicated that geographical influences were greater than sex effects in the differentiation between groups in the INTERMAP study. It is possible that genetic and environmental factors such as diet played a role in driving the differentiation between the three groups. In addition, the plasma fatty acid data showed similar group differentiation, which is indicative of dietary influences since they are closely associated with dietary patterns such as fish or olive oil intake.
The characteristics of the three geographical groups were relatively homogeneous, and the five criteria used to define the metabolic syndrome did not appear to be consistently more or less severe in any single group. Although the SW group had a significantly higher mean BMI compared with the NW and NE groups (34 (sd 5) kg/m2 compared with 32 (sd 4) and 31 (sd 4) kg/m2, respectively), each group fell into the same classification category (obese). In addition, small but significant differences were observed in diastolic blood pressure between the three groups, but the classification categories were again the same (pre-hypertensive and increased metabolic risks, respectively). Fasting glucose levels were significantly higher in the SW group (6·2 mmol/l) compared with the NW group (5·7 mmol/l), and neither of these groups were significantly different from the NE group. However, the HOMA-IR index was consistent across each group (NW: 2·5, NE: 2·5 and SW: 2·4), suggesting again that there was commonality in metabolic health across the three geographical regions.
Differences in dietary intakes provide a more plausible explanation for the variation in fatty acid profiles, and the metabolites that differentiated the three groups in the urinary 1H NMR score plot have previously been reported to be related to dietary intake( Reference Wittwer, Rubio-Aliaga and Hoeft 1 , Reference Walsh, Brennan and Malthouse 4 , Reference Dragsted 15 ). The plasma fatty acid data were consistent with the dietary data, indicating that the NE group had significantly higher intakes of n-3 and n-6 fatty acids compared with both the NW and SW groups. Plasma linoleic acid (18 : 2n-6), EPA and DHA (22 : 6n-3) levels were significantly higher in the NE group than in the other two groups. In addition, it is likely that the metabolomic data reflect other dietary components that were not included in the dietary intake data, such as dietary polyphenols.
The NW group had higher levels of urinary hippurate and N-methylnicotinate. Urinary hippurate is formed by the conjugation of glycine and benzoate in the liver, arising from the degradation of plant phenols in the large intestine. It has previously been reported to be associated with higher intakes of polyphenol-rich foods( Reference Manach, Williamson and Morand 16 – Reference Walsh, Brennan and Pujos-Guillot 18 ). N-Methynicotinate has been reported to be associated with discrimination between sources of dietary protein, which also supports the concept that urinary metabolomic data can be influenced by diet( Reference Holmes, Loo and Stamler 7 ). The NE group had higher levels of urinary creatine and citrate. Creatine excretion has been reported to be associated with high intakes of meat and fish( Reference Stella, Beckwith-Hall and Cloarec 19 , Reference Wyss and Kaddurah-Daouk 20 ), and citrate has been shown to have several origins within energy metabolism. The SW group had higher levels of urinary trimethylamine oxide, which has been reported to be associated with higher intakes of meat and fish( Reference Dragsted 15 , Reference Stella, Beckwith-Hall and Cloarec 19 , Reference Svensson, Akesson and Nilsson 21 ). These findings indicate that differences in dietary intake patterns may have contributed to the differentiation between the urinary metabolic profiles of the three geographical regions.
It is likely that dietary intake alone was not responsible for the differentiation of the urinary metabolomic samples and that other environmental and genetic factors played a role. An association between genetic variation and geographical location in Europe has previously been shown in the Population Reference Sample Project( Reference Novembre, Johnson and Bryc 22 ). The study performed PCA on SNP data obtained from 3192 Europeans and demonstrated that individuals from the same geographical location clustered together, with major populations being distinguishable from each other. In another study, it has been estimated that stable genetic and environmental factors contribute to 47 % of variation in urinary metabolic profiles( Reference Nicholson, Rantalainen and Maher 23 ).
In a nutritional intervention or in the assessment of any diet and health relationship, it is important to minimise the variation in baseline data for a better detection of any factors related to the field of investigation. In the present study, we have shown that geographical location may have a strong influence on urinary 1H NMR metabolomic samples. To understand the nature of these regional characteristics, such factors can be considered in future multi-centre metabolomic studies, thereby improving data interpretation.
Supplementary material
To view supplementary material for this article, please visit http://dx.doi.org/10.1017/S0007114513002602
Acknowledgements
The authors sincerely thank the wider LIPGENE group of investigators and the volunteers for their commitment and patience during the study.
The present study was funded by the European Commission Framework Programme 6 as part of the LIPGENE project (FOOD-CT-2003-505944).
The authors' contributions were as follows: M. C. W. was responsible for data collection, data analysis and manuscript writing; G. A. M. L. performed the metabolomic analysis; H. M. R. was the coordinator of the LIPGENE human dietary intervention study; J. F. F. performed the fatty acid analysis; C. A. D., W. H. M. S., J. A. L., U. R., J. L.-M., C. D. and B. K.-W. organised the collection of LIPGENE data; L. B. performed the metabolomic analysis and was responsible for the conception and design of the experiment and manuscript writing; M. J. G. was involved in the study design and manuscript editing. All authors contributed to the revision and final approval of the paper.
The authors had no conflicts of interest.