In recent years, political scientists have shed new light on the political consequences of seemingly irrational decision making by various actors in the political realm. Research on political behaviour shows voters often use mental shortcuts—that is, heuristics—when thinking about public policy. These include elite partisan cues (see, for example, Pickup et al., Reference Pickup, Stecula and Linden2020), a disproportionate focus on short-term policy impacts (Jacobs and Matthews, Reference Jacobs and Matthews2012), opinion polls (Singh et al., Reference Singh, Roy, Fournier, Blais, Laslier and Van der Straeten2016) and ideology (Caruana et al., Reference Caruana, McGregor and Stephenson2015). And voters are not the only ones. Public servants and politicians exhibit similar “fast thinking” about policy, leading to predictable cognitive biases (Kahneman, Reference Kahneman2013). These patterns are consistent with classic concepts from behavioural science, including the Asian disease experiment (Sheffer and Loewen, Reference Sheffer and J. Loewen2019), anchoring (Bellé et al., Reference Bellé, Cantarelli and Belardinelli2018), escalation of commitment (Sheffer et al., Reference Sheffer, Loewen, Soroka, Walgrave and Sheafer2018), biased information processing (Doberstein, Reference Doberstein2017b) and framing effects (Walgrave et al., Reference Walgrave, Sevenans, Van Camp and Loewen2018). Much of this work uses the experimental method, which is an increasingly popular method in public administration research and practice (Doberstein, Reference Doberstein2017a).
As behavioural science has become more popular in political science, so has the applied discipline of behavioural insights (BI) gained prominence in government. The rapid growth of BI units within the public sector has been well documented in countries such as the United Kingdom (Halpern, Reference Halpern2015; John, Reference John2014), the United States (Congdon and Shankar, Reference Congdon and Shankar2015; DellaVigna and Linos, Reference DellaVigna and Linos2022) and Australia (Jones et al., Reference Jones, Head and Ferguson2021). Yet the situation in Canada remains underexplored, with little empirical evidence about the systematic use of BI across different policy contexts (though see Lindquist and Buttazzoni, Reference Lindquist and Buttazzoni2021, for an important exception).Footnote 1 This gap is surprising given Canada's leading role in BI and the emerging work on policy labs (Evans and Cheng, Reference Evans and Cheng2021). The country was an early adopter of the technique and has pioneered its application (Castelo et al., Reference Castelo, Hardy, House, Mazar, Tsai and Zhao2015; Robitaille et al., Reference Robitaille, House and Mazar2021). In this research note, we ask to what degree, in what policy areas and to what effect has BI been applied in Canada? To answer these questions, we provide an environmental scan of BI in Canada using a new, original dataset of all publicly facing BI projects run by the governments of Canada, Ontario and British Columbia since 2015. We also provide original data from interviews with staff in BI units across three jurisdictions. Our research note begins with a definition of BI, situated within the broader field of behavioural public policy. Next, we highlight how BI interventions can support the policy process and improve policy efficacy. Third, we present empirical data on the size and scope of the existing BI infrastructure in Canada, the number of BI projects by policy area, the efficacy of those projects, and staffing resources over time. We conclude by acknowledging the myriad pathways that BI can support the enhancement of citizen-oriented service delivery and by pointing to the ways in which political science as a discipline can engage with BI in discussions on policy tools, design and efficacy. In Canada, as in many advanced industrial democracies, many administrative processes do not work well for priority populations. We advocate for greater use of BI, especially as a way to reduce administrative burden and improve the lived experience of Canadians.
What Are Behavioural Insights?
Legislative actors advance policy goals, but public servants handle the specifics of policy design and implementation, using research and evidence (French, Reference French2018). Policy success depends on balancing competing considerations, such as financial inputs and policy goals, as well as citizen behaviour. As citizens access public services, they confront technical and cognitive factors that may produce suboptimal decisions (for themselves and/or the government). Citizens can also face cognitive overload (Ly and Soman, Reference Ly and Soman2013), which overwhelms their ability to effectively access public services (McFadden, Reference McFadden2006). For example, consider a municipality that institutes a small fine for violating a new bylaw. The city spends considerable effort designing the optimal fine amount. However, the online portal for paying the fine is clunky and unintuitive. A citizen who wants to make such a payment tries repeatedly, only to get frustrated and abandon the task. The citizen is no better off (having to engage in a more laborious trip to city hall to pay the fine) and the city is no better off (delaying payment and having to engage a more costly and inefficient mode of processing the payment).
One innovative solution to this problem is behavioural public policy (BPP). BPP applies a behavioural lens to the policy process, with special focus on design, development, implementation and evaluation (Loewenstein and Chater, Reference Loewenstein and Chater2017; Oliver, Reference Oliver2017).Footnote 2 BPP's theoretical underpinnings are driven by the rationalist and behavioural sciences at the nexus of policy, economic and psychological research. To this, policy makers apply experimental and data-driven methodologies to make design recommendations, improve implementation and evaluate policy effectiveness. BPP focuses on perceived utility and incorporates behaviourally motivated techniques, including BI. These techniques often simplify services and improve outcomes for citizens and government (Chen et al., Reference Chen, Bendle and Soman2017; Ewert, Reference Ewert2020).Footnote 3 The term BI, as we apply it here, refers to the mechanism that underlies policy design and implementation.Footnote 4 BI may incorporate incentives (explicit inducements) and what Thaler and Sunstein (Reference Thaler and Sunstein2008: 6) call a “nudge” or “any aspect of the choice architecture that alters people's behavior in a predictable way without forbidding any options or significantly changing their economic incentives.”Footnote 5 Common behaviours include applying for public services/benefits, saving money, improving personal health, and adherence with laws or regulations (for example, paying taxes). BI can help encourage or discourage behaviours. BI can help promote pro-self and pro-social behaviours and encourage citizens to avoid “regret loss” from choices based on intuition and emotion rather than deliberation and reasoned analysis (Hagman et al., Reference Hagman, Andersson, Västfjäll and Tinghög2015). Above all, BI avoids rigid commitments that disengage citizens by removing their choices.
BI is unique because it integrates psychology, political and economic theory, and rigorous testing through randomized control trials. BI has its intellectual roots in behavioural research in Daniel Kahneman and Amos Tversky's work in the 1970s and borrows from their research on prospect theory (Reference Kahneman and Tversky1979) and mental shortcuts (Tversky and Kahneman, Reference Tversky and Kahneman1974). With the recognition that BI is not the solution to every policy problem (Richard Thaler (quoted in Nesterak Reference Nesterak2021) argues that “nudge is part of the solution to almost any problem but is not the solution to any problem”) and that policy makers must judiciously decide when to apply BI, the technique has recently become mainstream in public policy.Footnote 6 For example, McDavid and Henderson (Reference McDavid and Henderson2021) persuasively argue that the time has come for greater professionalization of BI, drawing insights from the field of evaluation to suggest a path forward. Mainstreaming BI is possible in part due to recent progress on ethics and ethical frameworks (for example, Delaney, Reference Delaney2018; Lepenies and Malecka, Reference Lepenies, Małecka, Poama and Lever2019; Lades and Delaney, Reference Lades and Delaney2022). These have allowed the field to more confidently advance practical discussions of how and when to apply BI (Ewert, Reference Ewert2020: 338).
As BI gains popularity, scholars are investigating its effectiveness, focusing on replication and publication bias. Some BI-style interventions have failed to replicate, even in controlled settings. Prominent examples include priming effects (Cesario, Reference Cesario2014) and inconsistencies in the ego depletion effect (for example, Carter et al., Reference Carter, Kofler, Forster and McCullough2015, Dang et al., Reference Dang, Barker, Baumert, Bentvelzen, Berkman and Buchholz2021). One challenge is the potential for heterogeneous effects across interventions, populations and field settings. Rather than generalize about its overall effectiveness, researchers are increasingly asking when and where BI can be effective (Bryan et al., Reference Bryan, Tipton and Yeager2021). A related concern involves systematic biases in the reporting of BI trials. Consistent with other fields (see, for example, Franco et al., Reference Franco, Malhotra and Simonovits2014; Esarey and Wu, Reference Esarey and Wu2016), meta-analysis shows evidence of publication bias in peer-reviewed behavioural science (Hummel and Maedche, Reference Hummel and Maedche2019). Using internal data from two large BI units in the United States, DellaVigna and Linos (Reference DellaVigna and Linos2022) show publication bias tends to exaggerate the effectiveness of BI interventions. In short, the evidence suggests that BI can be effective, that its effectiveness varies by context, and that academic publications involving BI may overstate the magnitude of these effects.
Situating BI in the Public Policy Literature and Process
The traditional policy cycle includes agenda setting, policy formulation, decision making, implementation and evaluation (Howlett et al., Reference Howlett, Ramesh and Perl2020). Classically, the cycle envisions senior policy makers and their staff at the centre of a top-down policy process (Wegrich and Jann, Reference Jann, Wegrich, Fischer, Miller and Sidney2006). However, the rise of behaviourally informed policy implementation brings a renewed emphasis on citizens as end-users of public services (Pal et al., Reference Pal, Auld and Mallett2021: 235–36). Brock similarly notes the pivot to the “deliverology” model of public service that is meant to transcend “the traditional obstacles to change in the public sector that [include] bureaucratic lethargy, lack of expertise, intransigence and risk aversion” (Reference Brock2021: 230). In one sense, this behavioural turn expands on previous studies of implementation that focus on citizen experiences, such as “street- level” bureaucracy (Brodkin, Reference Brodkin2012; Lipsky, Reference Lipsky2010), “bottom-up” implementation (Sabatier, Reference Sabatier1986; Thomann et al., Reference Thomann, van Engen and Tummers2018) and “backward mapping” (Elmore, Reference Elmore1979). But even here, the perspective is usually that of a policy maker or public manager, rather than the citizen. In short, there is a gap between prominent theories of public policy (which focus on decision making) and questions of citizen engagement (which focus on service-level interactions with government). Underlying this gap is the 40-year decline in institutional trust and confidence in government.Footnote 7
With the rapid expansion of the state in the postwar era, citizens are presented with a great number of areas and ways in which government can provide services. But this wealth of choice has come with a problem: undersubscription and unequal access to public services. Low uptake is particularly concerning because it disproportionately impacts the least resourced populations, such as seniors, immigrants, refugees, Indigenous peoples, and youth. Compounding this complexity is the digital divide and the transition to digital government (Longo, Reference Longo2017), particularly in the pandemic and post-pandemic environment. Research by the Citizens First initiative suggests a growing challenge for service delivery in Canada. Their 2020 report uses polling data to show that overall satisfaction with public services is at a two-decade low (Citizen First, 2021).
In this context, BI has proven a timely resource for policy makers. As Howlett notes, “conceptual work on procedural policy instruments [has tended to lag] behind administrative practice” (Howlett, Reference Howlett2013: 119). BI is already firmly present in Canadian public policy making. As a diagnostic tool, BI helps policy makers focus on policy goals, anticipate delivery gaps and increase service uptake. One of the most effective ways to increase uptake is to reduce administrative burden (Herd and Moynihan, Reference Herd and Moynihan2019; Moynihan et al., Reference Moynihan, Herd and Harvey2015). Burdens arise when an individual experiences program or service enrolment or engagement as onerous (Burden et al., Reference Burden, Canon, Mayer and Moynihan2012). Burdens result from costs imposed on the user, such as the hassle of figuring out eligibility requirements (learning costs), potential stigma in accessing public services (psychological costs) and the time/effort required to fill out documentation (compliance costs) (Herd and Moynihan, Reference Herd and Moynihan2019). In this way, BI can be considered a type of procedural policy instrument capable of reducing burdens and narrowing this divide. What appears on the surface as an operational challenge (improving policy uptake) is really an interconnected chain of policy design and service delivery choices that influence how citizens experience government.
Policy makers are increasingly optimistic about the potential for BI to improve public policy and service delivery. In a recent survey of 719 Canadian public servants, Doberstein and Charbonneau (Reference Doberstein and Charbonneau2020) find BI teams (and innovation labs more generally) are now seen as the single most important source of public sector innovation. However, it would be naive to suggest that more innovative service delivery will remedy all problems of citizen engagement. Failing to engage with citizens can be viewed as the product of choices by politicians—the result of purposefully underinvesting in the welfare state. It is therefore no accident when the most underserved are those with the fewest resources to access the system (Banting, Reference Banting, Banting, Hoberg and Simeon1997, Reference Banting2010). In this way, policy design and implementation are deeply political.
Taking into account the literature on administrative burden, policy makers must be careful not to use BI solely as a solution to “middle-class” problems—such as saving for retirement, buying energy-conserving appliances, and so on (Benartzi et al., Reference Benartzi, Beshears, Milkman, Sunstein, Thaler, Shankar, Tucker-Ray, Congdon and Galing2017). Policy makers who apply BI have demonstrated its potential to yield high returns at low cost—but, in many cases, these successes are concentrated among those who are already capable of effectively interacting with government. If BI works well for the middle class but does not reduce administrative burden among those with fewer resources, it will not realize its potential to advance democratic, pro-social outcomes. It is equally important that policy makers understand the potential for heterogeneous results, such as differences by race, gender and income. BI should not be applied without consideration for resource disparities, lest the public view it simply as “policymaking by other means” (Herd and Moynihan, Reference Herd and Moynihan2019).
For these reasons, an ethical lens is crucial when using BI, especially when selecting targeting behaviours and considering downstream impacts. For example, a Canadian government experiment aimed to increase women's enrolment in the military using Facebook ads with different appeals (Privy Council Office, 2017). But MacDonald and Paterson (Reference MacDonald and Paterson2021) argue that the evidence is mixed about whether increasing women's participation in the Armed Forces will increase equity. They cite research on the Royal Canadian Mounted Police (Bastarache, Reference Bastarache2020) to point out that when women enter traditionally male-dominant professions, it may accompany increased reports of sexual assault and harassment. We do not suggest it is inherently unethical to nudge women into the Armed Forces; however, we believe an ethical lens is required both in the scoping process and in post hoc analysis.
Data, Methods and Analysis
Our analysis is a four-pronged environmental scan. An environmental scan is a method to gather information and identify opportunities and risks for future decisions. The method involves various data sources, including both published and unpublished information (Porterfield et al., Reference Porterfield, Hinnant, Kane, Horne, McAleer and Roussel2012). Environmental scans are not exhaustive but rather give a general picture of a field (Wheatley and Hervieux, Reference Wheatley and Hervieux2020). Our environmental scan considers (1) the number and location of existing BI units in government, (2) BI projects from public-facing reports spanning 2015 (the first published results from a publicly reported BI project by government in Canada) to 2022 by policy area, (3) BI projects by methodology and (4) BI projects by rate of success. While we are relying on self-reported government data, and recognize the associated concerns around governments only presenting “successes,” we observe that governments are increasingly following an “open science” model of BI. For example, the government of British Columbia has preregistered several field trials, which limits the degree to which public reporting may represent (only) positive outcomes. Preregistration is an encouraging trend given the concerns over replication and publication bias described earlier in this research note.
Finally, we supplement our environmental scan with interview data conducted with BI personnel across five BI units in three jurisdictions. Semistructured interviews took place remotely between July 2020 and July 2021. The thematic foci of these conversations included project design, responses to project successes and failures, and methodological approaches, as well as partnerships across government units. While we do not present a full thematic analysis of the interview data, we do use the observations that were noted across multiple offices to complement our empirical findings. Since Canada's BI field is comparably small and there are only three stand-alone units at present (though several mini units within government departments), we report interview comments anonymously without geographic or jurisdictional identification.
BI units in Canada
The nudge movement was an important catalyst in the development and institutionalization of BI in Canada. The template for BI teams is largely found in the 2010 British initiative under David Cameron's Cabinet Office. One of the authors of Nudge (2008), Richard Thaler, served on its advisory board, with social and political scientist David Halpern serving as its chief executive. In 2015, Barack Obama established the Social and Behavioral Sciences Team in the White House Office of Science and Technology Policy. This followed the appointment of Nudge co-author Cass Sunstein as administrator of the Office of Information and Regulatory Affairs in 2009.
Canada's BI experience reflects policy learning from these international efforts. In 2017, the federal Impact and Innovation Unit (IIU), previously known as the Innovation Hub, was founded as part of the federal government's Innovation Agenda. The IIU is embedded within the Privy Council Office. The interdisciplinary team consists of approximately 15 individuals with a variety of educational backgrounds, including education and neuroscience. The stated goal of the IIU is to reduce barriers to innovation within government and to “leverage the benefits of impact measurement to support evidence-based decision-making” (Impact and Innovation Unit, 2021). Examples of completed projects include an effort to increase charitable giving (2017) and help low-income families access the Canada Learning Bond for post-secondary education (2018). The IIU mostly runs projects through partnerships with other government departments such as the Canada Revenue Agency and Employment and Social Development Canada. Typically, these partnerships result in randomized controlled trials (RCTs) to test potential solutions and improve policy outcomes.
Several provinces have their own BI teams (full listing in the supplementary material). Designs vary by province. Ontario's BI unit was developed in 2015 within the Treasury Board Secretariat. Its policy successes include an increase in the uptake of the human papillomavirus (HPV) vaccine, increasing cervical cancer screening rates for women, and enhanced engagement with provincial and municipal online services. Using RCTs, the BI unit reported a 143 per cent increase in organ and tissue donor registrations, an 82 per cent increase in correct recycling of organics, and increased use of the province's online portal to complete administrative tasks such as renewing driver's licence plate stickers (Government of Ontario, 2018). Much like the federal unit, the Ontario team works with other sectors of government. Correspondingly, its focus is jurisdictionally tailored to problems that fall under the province's purview. It also has partnerships with academia—specifically, the Behavioural Economics in Action at Rotman and the Southern Ontario Behavioural Decision Research Group, which support RCT design and analysis.
British Columbia's Behavioural Insights Group (BIG) started in 2016 and is situated within the Public Service Agency. Similar to the federal and Ontario BI teams, the British Columbia team partners with other government ministries to design and test policy solutions. In its first four years, the unit designed 19 RCTs and 35 behavioural “lenses,” which offer advice to client ministries about how BI might improve a policy, program or service. Starting in 2017, Alberta's CoLab engaged in BI work but was disbanded under the United Conservative government in 2020. Nova Scotia is in the process of expanding its BI capacity and has already reported notable success with an organ donation campaign in 2019. Several other provinces support behavioural labs, which may support community partnerships or industry, and their focus is not necessarily on BI alone (Evans and Cheng, Reference Evans and Cheng2021). However, they often feature BI approaches and may work in tandem with BI units. And while no municipality has a stand-alone BI team at present, several larger cities have ongoing funding for projects, such as CityStudio (Vancouver) or Civic Innovation (Toronto) that focus on enhancement of citizen engagement and improvements to service delivery. Outside of government, there is a Canadian office of the Behavioural Insights Team, which is a social purpose company that emerged from the original BI unit in the UK government. Established in 2019, the office follows a consultancy model, working with government actors and not-for-profit organizations to support BI projects.
BI projects
We next address our data collected from public reports published by the three active BI units in Canada (the federal IIU and the BI units in Ontario and British Columbia). Our dataset includes a total of 59 projects across 13 policy areas (see supplementary material for more information on coding). Our data is supplemented by email correspondence and interviews with individuals in five BI units (federally and provincially) to address discrepancies or data limitations in the reports and to gain insight into project efficacy and rollout. Where appropriate, we supplement the data with observations from our interview data. Given the small number of projects, the analysis is necessarily limited. However, we are able to provide top-line information in policy coverage, methodological design, and goal-meeting or project efficacy.
Figure 1 illustrates the number of projects by policy area (full dataset available in supplementary materials). A clear majority of projects (N = 30) were initiated in the areas of health (N = 17) and government operations (N = 13; for example, administrative fines, documentation renewals), though we do see a variety of projects across social and administrative policy areas. Health projects range from the Ontario BI unit's “Supporting Ontario's COVID-19 Response” to helping physicians improve prescribing behaviour to reduce the prescription of opioids. A total of 21 per cent of these projects were initiated through the federal IIU, while the remaining 79 per cent come from provincial offices (68 per cent from Ontario and 11 per cent from British Columbia). Interview participants R1 and R2 both noted the suitability of administrative operations to BI trials because they often entail fewer ethical concerns (compared with projects involving social policy) and because they lend well to experimentation using digital touchpoints (that is, sending notices, online platform-based delivery). During the early days of the COVID-19 pandemic, these factors facilitated the continuation of BI work.

Figure 1 Number of Projects by Policy Area.
We also evaluate the form of these projects, specifically the methodological approaches taken. The majority of publicly released BI projects are field studies (N = 32)—that is, interventions or initiatives that apply BI principles in real-world settings. Another 22 projects focused on user-centred design; four focused on using survey experiments to test BI assumptions. All of these projects are evidence-based responses to policy challenges, with RCTs as the dominant methodological design (49 per cent) and the remainder using survey research or qualitative designs such as focus group analysis and post hoc reviews by experts in the field.Footnote 8 Interviews with R2 and R3 yielded a preference for a multi- or mixed-method approach to validate findings with supplementary stakeholder interviews, or to engage priority communities with requests for feedback. Respondents demonstrated a responsiveness to broad critiques of BI as being too quantitatively focused, and they accentuated the importance of consultation with program beneficiaries to reduce the risk of losing the “human” aspect of their goal.
Project efficacy is measured through a very simple metric: whether the BI project yielded a significant result on its stated goal. Importantly, the scale of BI results is moderate. Some projects hope to increase uptake by a modest 3 to 5 per cent; however, given the scale of these interventions, this can translate into tens—or even hundreds—of thousands of citizens. In every interview, practitioners noted the challenges of articulating the significance of what appeared to be modest effects when demands on government resources are so large. However, three respondents also observed that government partners would quickly embrace these findings when they viewed them as sustainable ways to enhance participation at a low cost to the budget unit. Many projects that we tracked are still ongoing and therefore may have only reported preliminary data. However, of those that are registered as complete, 80 per cent were successful at attaining their goal. These outcomes ranged from reducing delinquency on tax collection payments by 35 per cent in British Columbia to increasing applications for the Ontario Electricity Support program for low-income Ontarians.
One might reasonably ask how confident we can be that the BI interventions were the cause of these impacts. We suggest confidence depends on research design. When the project involves an RCT, randomization to treatment and control ensures an unbiased estimate of the impact (for example, intent-to-treat effect, average treatment effect). When we restrict our analysis to only those completed BI projects involving an RCT (N = 25), we find a similar efficacy as the full sample: 80 per cent of BI RCTs in Canada were successful at attaining their goal.
Finally, we use Canada's Government Electronic Directory Services to estimate changes in the number of BI personnel over time. We collect cross-sectional directory data across seven timepoints, spanning 2016 to 2022 (see supplementary material for more information). For each timepoint, we identified employees who either worked in units containing the phrase “behaviour” or those who worked at the IIU. We then manually reviewed each case, excluding observations where the employee did not appear to work in BI. In Figure 2, we tally the number of personnel by year. We find that the number of BI staff has increased tenfold over six years, with an average annual increase of approximately 48 per cent. In 2016, six federal employees worked in BI. By 2022, there were 63.Footnote 9 Further, our data allow us to map the spread of BI across departments. For example, in 2016, BI staff worked in just two departments: the Canada Revenue Agency (N = 1) and Privy Council Office (N = 5). By 2022, we find BI staff in 10 departments, including the Public Health Agency of Canada (N = 16), Immigration, Refugees and Citizenship (N = 5), Treasury Board Secretariat (N = 3) and Environment and Climate Change (N = 2). Growth has been especially strong in the Public Health Agency. In 2019, prior to the COVID-19 pandemic, the agency reported just one BI employee. By 2022, the BI team had grown to 16.
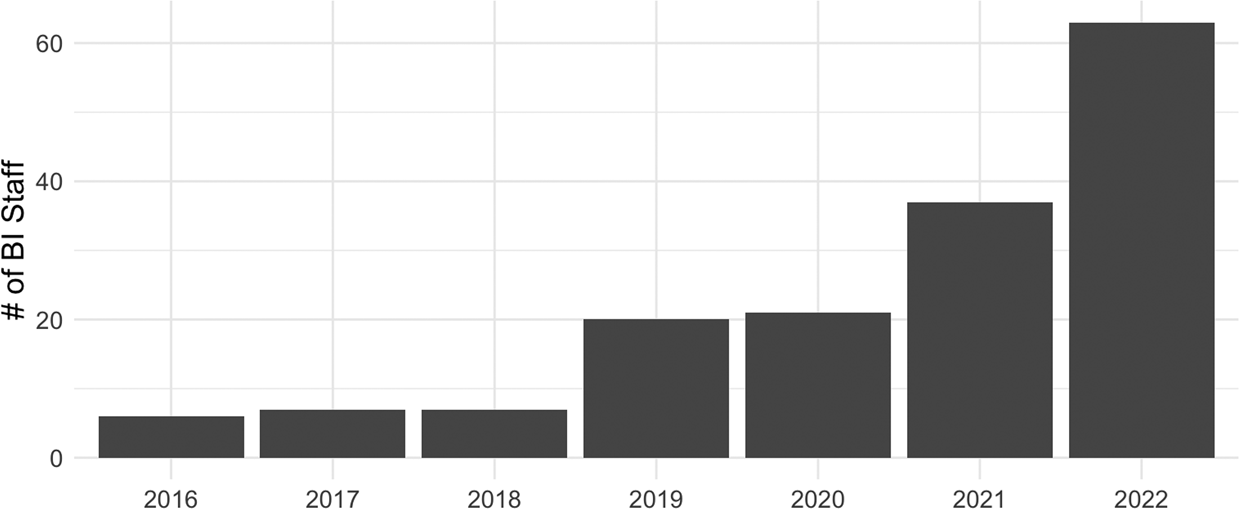
Figure 2 Growth of BI staff in the Government of Canada, 2016–2022.
Note: Data collected from the Government Electronic Directory Services.
Going Forward
Behavioural science is changing public policy, and, with it, political science. This research note provides four empirical contributions about BI in Canada. First, we observe that governments are actively investing in BI as a policy-making tool.Footnote 10 Governments invest in BI to increase program success and more efficiently use financial resources. Second, governments are expanding the scope of BI, moving beyond central agencies to line departments. The number of BI practitioners across government has increased dramatically—by our estimates, a tenfold increase in just six years. Third, our data show that, in general, BI initiatives are working. A critic might observe that governments are only likely to advertise successful projects, leaving failed projects firmly within the private reports of civil servants. However, our data show that governments have acknowledged several instances where BI projects have come up short. In part, this is because the methodological approach to BI embraces failure as a part of design and evaluation. Finally, as demonstrated in our reporting and data analysis, Canadian governments are using BI across policy domains, with a clear emphasis on improving government operations and service delivery, health, and social welfare.
From a comparative perspective, our findings dovetail with recent research on the application and methodology of BI across contexts. Like others (for example, Hummel and Maedche, Reference Hummel and Maedche2019: 52), we find that BI projects in Canada are most common in the domain of health. Similarly, we find a growing acceptance for non-experimental BI work in Canada, which parallels recent research from Australia (for example, Ball and Head, Reference Ball and Head2021: 114–16). Nonetheless, we recognize several limitations of our Canada-focused environmental scan. First, limited data availability mean we cannot report on some projects. For example, we find few instances where Canadian governments discuss using BI to improve tax collection. Yet media reports (Beeby, Reference Beeby2018) and our own data on the number of BI personnel suggest the Canada Revenue Agency is actively using BI to improve tax collection. Additionally, interview data that we have collected indicates numerous projects in progress across multiple policy domains that are not yet ready for disclosure. We argue that even without the full universe of BI data, our study includes many policy initiatives that reflect a particular approach to policy making—one that should be recognized in political science. Additionally, no policy scholar has a complete list of government projects to evaluate, owing to the same data considerations we face here (for example, access to information, privacy, secrecy). A second limitation involves how to define a BI project. As we have noted above, distinguishing between what the government might formally call a BI project and instances where BI tools or approaches have been used informally means that we may have undercounted our observations of BI in government.Footnote 11
In closing, we reflect on Michael Atkinson's 2013 presidential address to the Canadian Political Science Association. Atkinson (Reference Atkinson2013) urged the discipline to embrace its roots in social and behavioural science. He framed this as an empirical and normative calling: to improve governance and, by extension, the lives of Canadians. In many ways, the discipline has taken up the charge. So too have some parts of government. In this note, we reflect on BI as a potentially powerful tool that can help public policy practitioners and scholars live up to Atkinson's ideal and strengthen citizen–government engagement in Canada. This can include external evaluation of BI policy design and implementation on its own merits but could also extend to collaboration between BI practitioners and political scientists, who bring a rich skillset around research questions and methodological design, while simultaneously taking a critical lens to governments’ policy design choices.
This research note highlights the fertile ground that exists to explore a robust research agenda on BI or with BI. Two streams clearly emerge: (1) evaluating the BI projects conducted by government and (2) using BI either with or independent of government as a tool to generate insights around program delivery. We make no claim that BI is the only or best tool to resolve any policy problem. We merely suggest it is an underused tool for resolving policy challenges in novel and cost-effective ways. We hope political scientists will take up this call.
Supplementary Material
To view supplementary material for this article, please visit https://doi.org/10.1017/S0008423923000100