Introduction
The global prevalence of depression has steadily increased from 1990 to 2019, 1 and approximately 1 in 5 adults in the US will experience major depressive disorder (MDD) at some point in their lifetime.Reference Hasin, Sarvet and Meyers 2 Depression was one of the top 10 causes of disability globally in 2019 and is associated with significant direct and indirect economic and societal burdens.Reference Greenberg, Fournier, Sisitsky, Pike and Kessler 3 , Reference Greenberg, Fournier and Sisitsky 4 The COVID-19 pandemic exacerbated many risk factors for depression across countries of disparate economic statusReference Panchal, Kamal, Cox and Garfield 5 , Reference Xiong, Lipsitz and Nasri 6; the prevalence of depressive symptoms in the US increased approximately threefold from 2017 to 2020,Reference Ettman, Abdalla, Cohen, Sampson, Vivier and Galea 7 along with increased associated economic burdenReference Cutler and Summers 8 and rates of suicidal ideation.Reference Czeisler, Lane and Petrosky 9 Therefore, it is important to understand the challenges currently facing mental health practices (eg, geographical and health inequities may limit access and quality of careReference Naslund, Aschbrenner and Araya 10) and the need to improve clinical management of depression.
Digital health technology is rapidly evolving the delivery of mental health care and holds promise for improving access to and quality of mental health care, thus improving the clinical management of depression.Reference Naslund, Aschbrenner and Araya10 Digital health technology includes categories such as digital therapeutics (eg, prescription or nonprescription digital therapeutics), digital interventions (eg, wellness apps), precision medicine (eg, digital biomarkers), and digital care (eg, telepsychiatry) (Figure 1).11 Digital therapeutics provide software-based treatments that have been approved or cleared by the US Food and Drug Administration (FDA) (“Software as a Medical Device,” or SaMD).Reference Patel and Butte12 Digital biomarkers hold promise for enabling scalable, time-sensitive, and cost-effective assessment of depressionReference Jacobson, Weingarden and Wilhelm13, Reference Jacobson, Weingarden and Wilhelm14 by providing real-time psychological, behavioral, and physiological data,Reference Marzano, Bardill and Fields15 but further validation is required. Telepsychiatry, the use of electronic information and telecommunication technologies to provide mental health services, has been adopted in direct patient care, telepsychiatry-enhanced referral, and collaborative or integrated care models.Reference Malhotra, Chakrabarti and Shah16 During the COVID-19 pandemic, telepsychiatry has transformed the mental health care landscape and may continue to help improve the clinical management of depression.Reference Duncan, Serafica, Williams, Kuron and Rogne17 This review aims to highlight the current gaps in depression management and consider how digital health technologies can help address challenges faced by patients living with depression and providers managing depression.
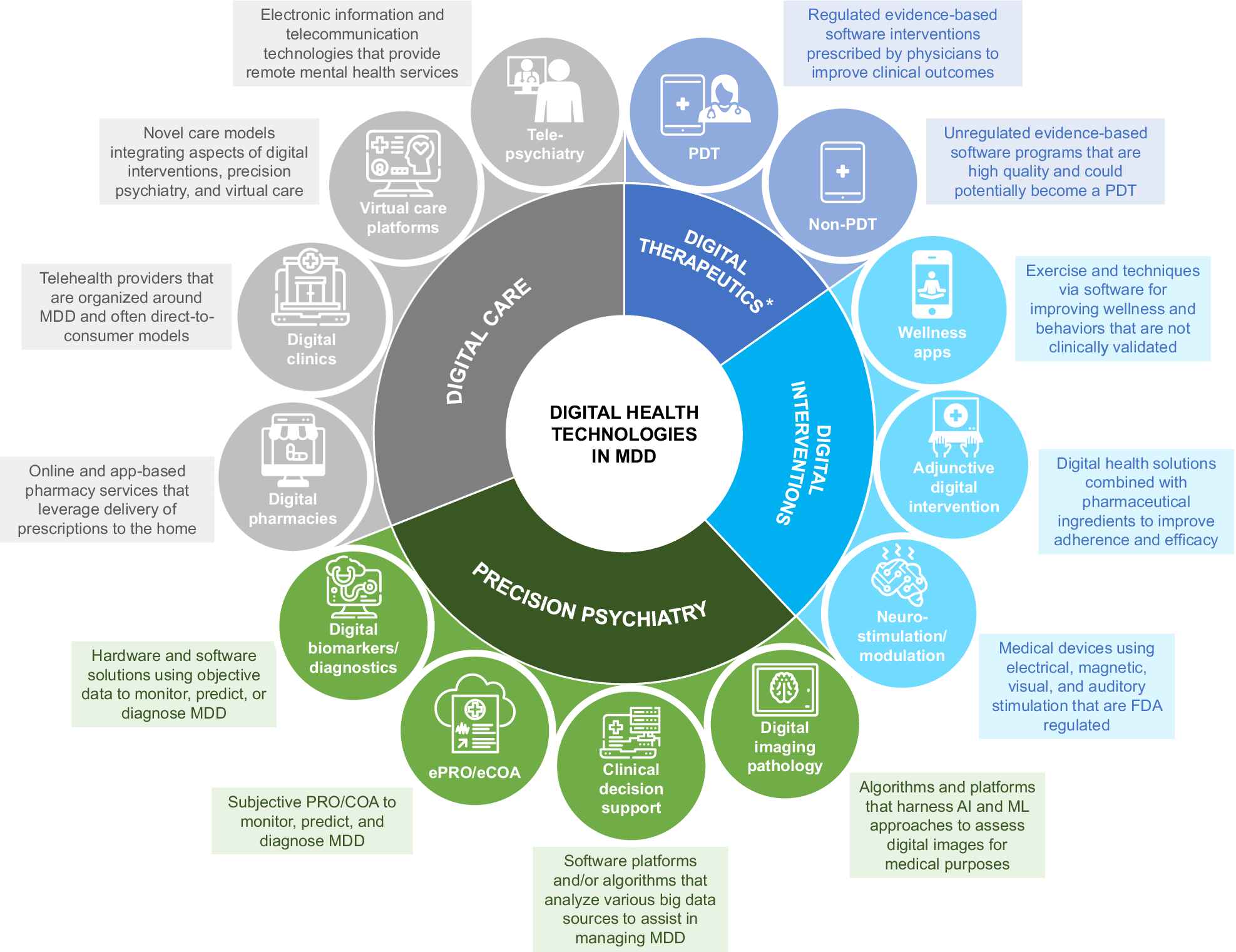
Figure 1. Different categories of digital health technologies. *Some apps are marketed as digital therapeutics under FDA enforcement discretion. Abbreviations: AI, artificial intelligence; eCOA, electronic clinical outcome assessment; ePRO, electronic patient-reported outcome; FDA, Food and Drug Administration; MDD, major depressive disorder; ML, machine learning; PDT, prescription digital therapeutics.
Current challenges in the clinical management of depression
Gaps and challenges currently faced in the clinical management of depression include those related to disease awareness, screening and diagnosis, and treatment and monitoring (Figure 2).
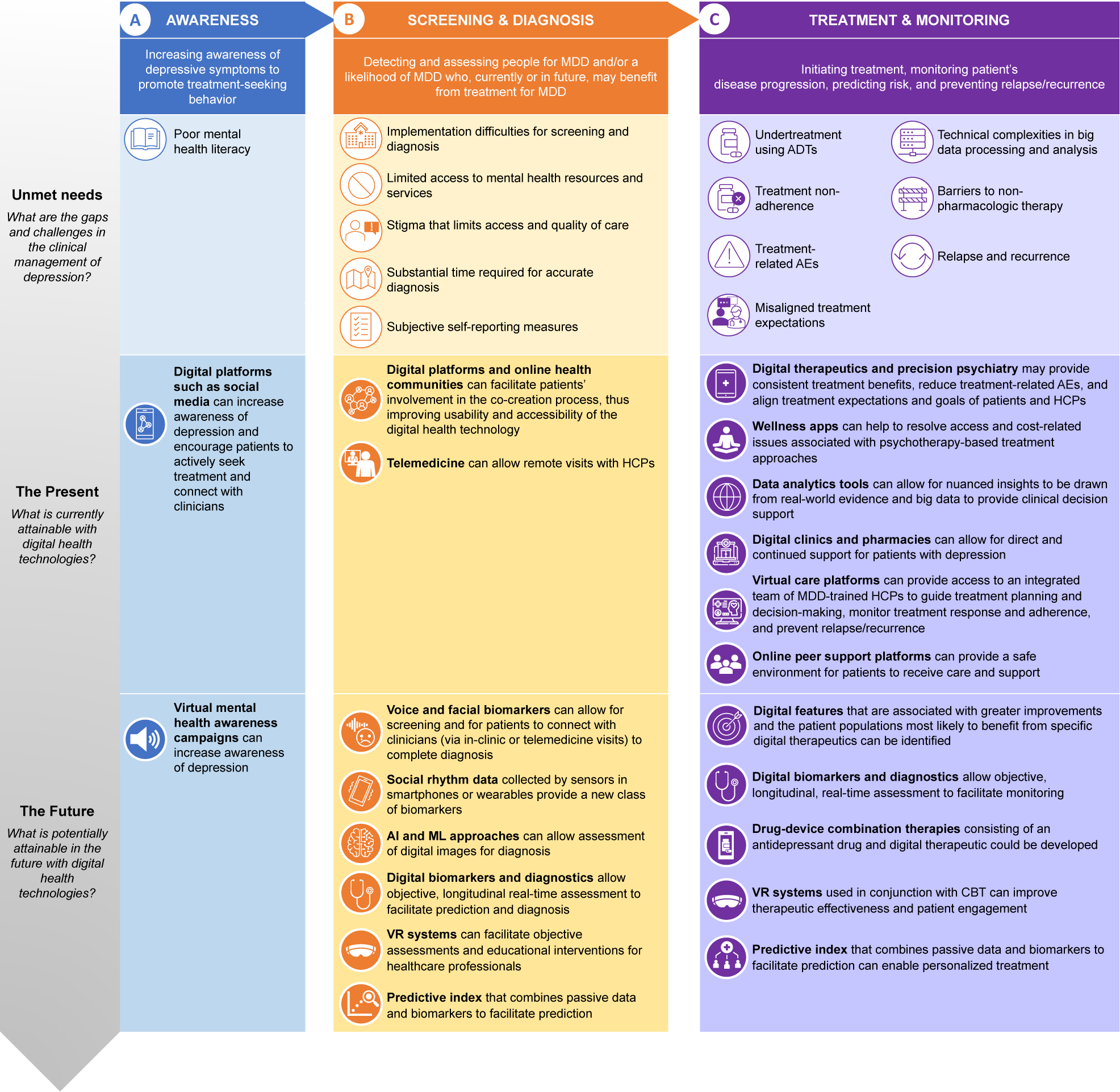
Figure 2. Major depressive disorder (MDD) patient journey—unmet needs in the clinical management of depression and the role of digital health technologies. Abbreviations: ADT, antidepressant therapy; AE, adverse event; AI, artificial intelligence; CBT, cognitive behavioural therapy; HCP, healthcare provider; MDD, major depressive disorder; ML, machine learning; VR, virtual reality.
Awareness
Poor mental health literacy, or the lack of knowledge and understanding of mental health disorders like depression, may prevent patients from recognizing depression symptoms early and seeking appropriate treatment.Reference Latha, Meena, Pravitha, Dasgupta and Chaturvedi 18 Poor awareness of depression may also contribute to the stigma associated with the disorder that, in turn, diminishes treatment seeking and adherence to mental health services.Reference Salerno 19 In a study involving more than 50 000 individuals across 21 countries, the vast majority of those affected by depression did not seek treatment due to stigma or lack of knowledge of the disorder.Reference Thornicroft, Chatterji and Evans-Lacko 20 For adolescents, many reported moderate to high levels of stigma associated with depression and low levels of mental health literacy, which contributed to their early termination of treatment.Reference Salerno 19 Men tend to experience higher levels of stigma regarding help-seeking and having depression than women,Reference Staiger, Stiawa and Mueller-Stierlin 21 which may result in higher rates of suicide.Reference Stiawa, Müller-Stierlin and Staiger 22
Screening and diagnosis
Over the last two decades, the US Preventive Services Task Force and Agency for Healthcare Research and Quality guidelines have recommended routine screening for depression in healthcare settings to address the under-recognition of depression.Reference Trivedi, Jha and Kahalnik 23 However, the rates of depression screening remain low (1.4% of all primary care visits in the US in 2012), 24 and there have been few specific guidelines to support health system implementation of universal depression screening in primary care.Reference Last, Buttenheim and Futterer 25 Barriers to depression screening and the lack of adequate follow-up in patients who screened positive for depression include lack of resources for treatment referrals, limited access to mental health services, and unequal geographical distribution of specialists.Reference Colligan, Cross-Barnet, Lloyd and McNeely 26 , Reference Andrilla, Patterson, Garberson, Coulthard and Larson 27 Furthermore, health equity challenges and stigma associated with depression, including racialized framing on the part of the healthcare provider (HCP), may limit access and quality of care.Reference Knaak, Mantler and Szeto 28 , Reference Bailey, Mokonogho and Kumar 29 LGBTQ (lesbian, gay, bisexual, transgender, and queer) individuals may be at a higher risk for suicidal behavior and discrimination in healthcare settings due to stigma compared with heterosexual individuals.Reference Meyer 30 , Reference Kaniuka, Pugh and Jordan 31
Even with screening, diagnosis may be hampered by several challenges. Substantial time is required for an accurate diagnosis of depression, which is often observed alongside other physical and psychiatric comorbiditiesReference Lam, McIntosh and Wang 32 that may obscure a diagnosis of depression. Additionally, the diagnostic workup involves self-reported measures that may be subjected to biases and may be challenging to implement in a clinical setting (eg, integration into clinic workflow and electronic health records [EHRs] and interpretation of self-reported outcomes by HCPs) and in certain populations (eg, children, individuals with organic or neurological deficits, individuals with developmental or communication disorders, and individuals with low mental health literacy).Reference Richter, Fishbain, Richter-Levin and Okon-Singer 33 , Reference Finlay and Lyons 34
Treatment and monitoring
Patients with depression may be undertreated with antidepressant therapies (ADTs), as observed in a longitudinal study involving 789 primary care patients with depressive symptoms, in which 41% were undertreated (ie, did not receive ADTs even though an ADT was indicated).Reference Davidson, Romaniuk and Chondros 35 , Reference Keyloun, Hansen, Hepp, Gillard, Thase and Devine 36 Patients may discontinue chronic treatment due to lack of rapid and consistent treatment benefits,Reference Pompili, Venturini and Palermo 37 treatment-related adverse events (AEs),Reference Pompili, Venturini and Palermo 37 -Reference Ferguson 40 and expectations of treatment that are misaligned with the treatment goals of HCPs.Reference Winter and Barber 41 , Reference Mercier, Auger-Aubin and Lebeau 42 Nonadherence to ADTs is associated with increased severity of depressive symptoms, risk of relapse (ie, early return of symptoms within the expected duration of a current episode) and recurrence (ie, a new major depressive episode following a full-episode remission), and healthcare resource utilization, as well as significantly higher medical costs, inpatient admissions, and visits to the emergency department.Reference Lewis, Marston and Duffy 43 , Reference Ta, Sullivan, Tung, Oliveri, Gillard and Devine 44
Both the American Psychiatric Association and the American Psychological Association practice guidelines recommend that HCPs consider appropriate treatments (including both pharmacologic and nonpharmacologic therapy options) for preventing relapse in patients with depression who have achieved remission. 45 , 46 Nonpharmacologic therapy refers to health interventions that are not based on medication (eg, psychotherapy, physical exercises, and transcranial magnetic therapy).Reference Gartlehner, Wagner and Matyas 47 In a large sample of primary care patients, the majority of patients with depression (51%-58%) reported strong preferences for nonpharmacologic therapies,Reference Dorow, Löbner, Pabst, Stein and Riedel-Heller 48 but only about 20% of patients initiated such treatment,Reference Waitzfelder, Stewart and Coleman 49 and about 40% to 60% of those who initiated nonpharmacologic therapy did not complete the treatment,Reference Lazaratou, Anagnostopoulos, Vlassopoulos, Tzavara and Zelios 50 suggesting that there are substantial barriers both to initiating and to adhering to nonpharmacologic therapy in the real-world practice. 51 These barriers may be structural (eg, transportation problems, time constraints, and cost) or emotional (eg, social stigma and discomfort talking about problems with a therapist).Reference Mohr, Ho and Duffecy 52 There is also a lack of sufficient evidence for their effectiveness and cost-effectiveness in primary care settings. 53
There is an increasing need for more personalized approaches to monitor and treat depression, but this is associated with several challenges.Reference Deif and Salama 54 It is difficult to integrate patient information in a clinically relevant manner due to technical complexities in processing and analyzing data generated from multidimensional data sets and/or using artificial intelligence (AI) modeling.Reference Deif and Salama 54 In addition, there are various ethical concerns, including privacy and security issues and risks of social discrimination.Reference Deif and Salama 54 The APA has published an app evaluation model that assesses access and background, privacy and safety, clinical foundation, usability, and therapeutic goal of a given app to attempt to address these concerns based on individual patients’ needs. 55
Rapid growth of digital health technologies
Digital health technologies have emerged over the past decade for the management of various diseases. In 2013, a mobile medical app (WellDoc’s BlueStar Rx) was cleared by the FDA as a prescription digital therapeutic (PDT) to support the management of type 2 diabetes by analyzing patient data, formulating personalized guidance, and summarizing data analytics to the healthcare team for clinical decision support.Reference Fleming, Petrie, Bergenstal, Holl, Peters and Heinemann 56 In 2017, the FDA cleared a PDT (Pear Therapeutic’s reSET) that delivers a computerized version of cognitive behavioral therapy (CBT) and serves as an adjunct to clinician-supervised outpatient therapy programs in patients with substance use disorder. 57 Since then, the FDA has cleared more mobile medical apps, including those that help diagnose autism spectrum disorder: (a) Cognoa’s Canvas Dx is a software that uses a machine learning (ML) algorithm to receive input from parents or caregivers, video analysts, and HCPs to help clinicians evaluate a patient’s risk of the disorder, and (b) EarliTec’s EarliPoint Evaluation is a device that uses AI to detect a patient’s moment-by-moment looking behavior, serving as an objective measurement tool that helps clinicians diagnose and assess the disorder. 58 , 59 Recently, the FDA also issued marketing authorization for a virtual reality (VR)-based app, EaseVRx, as an adjunctive treatment for chronic low back pain.Reference Rubin 60 Both the Digital Therapeutics Alliance 61 and the Digital Medicine Society 62 offer educational resources dedicated to the applications of digital health technologies.
During the COVID-19 pandemic, there was a rapid growth in access to and capabilities of digital health technologies worldwide.Reference Omboni, Padwal and Alessa 63 A 2021 global survey involving 293 HCPs showed that various subsegments of digital health saw considerable growth (up to 36%) from 2020 to 2021, 64 with telehealth and telemedicine emerging as key digital approaches to disease management.Reference Omboni, Padwal and Alessa 63 The majority of telehealth-related literature published during the COVID-19 pandemic (92.3%) reported provision of telehealth services for conditions not related to COVID-19,Reference Doraiswamy, Abraham, Mamtani and Cheema 65 with the most common being integrated clinical care (49.7%), including a combination of triage, diagnosis, treatment, follow-up, and rehabilitation services.Reference Doraiswamy, Abraham, Mamtani and Cheema 65 A 2020 survey of more than 3000 patients treated with telepsychiatry during the COVID-19 pandemicReference Guinart, Marcy, Hauser, Dwyer and Kane 66 and a separate survey of more than 100 mental health cliniciansReference Gentry, Puspitasari and McKean 67 during the COVID-19 pandemic revealed that both patients and clinicians were supportive of telepsychiatry, were satisfied with the quality of care, and would consider using this modality in the future.
The use of digital technologies for depression, including telehealth and telemedicine, also increased during the pandemic; patients with depression were three times more likely to use telehealth than those without.Reference Andersen, Rowland, Gloster and McElfish 68 , Reference Arafat, Zaman and Hawlader 69 The rapid growth in digital health technologies globally presents opportunities toward bridging the current gaps in the clinical management of depression. Besides therapeutic interventions, digital technologies may support clinical management of depression by: (a) capturing longitudinal, temporally dense, and multimodal mental health data for use in diagnosis and monitoring; (b) analyzing data using ML paradigms to generate personalized and clinically actionable insights and predictions; and (c) supporting integrated care by facilitating connections to clinical care, peer support, personalized resources, emergency care, and even novel therapies.Reference Torous, Bucci and Bell 70 Several studies have demonstrated the potential of using digital biomarkers (based on sensor data from wearable devices or mobile apps) for the prediction and detection of depression.Reference Opoku Asare, Terhorst, Vega, Peltonen, Lagerspetz and Ferreira 71 -Reference Rykov, Thach, Bojic, Christopoulos and Car 73 There are also opportunities to develop online and mobile apps for the prevention of depression.Reference Sander, Paganini and Terhorst 74 However, it should be noted that unlike conditions such as diabetes, where significant adoption of digital technologies has been observed, depression is more complex due to the unique challenges associated with the clinical management of the disorder (eg, poor mental health literacy, stigma, and the lack of objective tools for assessment and diagnosis). Further studies would be required to refine digital technologies for real-world implementation in depression management, which includes encouraging continued engagement with digital health technology beyond first-time use. It is worthwhile to examine lessons learned from other disease areas where high rates of engagement of digital technologies have been achieved.
The present: What is currently attainable with digital health technologies
Digital health technologies for depression gained broad public attention after the release of the SuperBetter app,Reference Roepke, Jaffee, Riffle, McGonigal, Broome and Maxwell 75 MoodHacker app,Reference Birney, Gunn, Russell and Ary 76 and SPARX,Reference Merry, Stasiak, Shepherd, Frampton, Fleming and Lucassen 77 which are self-management tools to reduce depressive symptoms supported by randomized controlled trial data.
In a randomized controlled trial, participants using SuperBetter (n = 190) experienced a greater reduction in depressive symptoms than those placed on a waiting list (n = 93), as assessed by the Center for Epidemiological Studies Depression questionnaire (CES-D) over the course of 6 weeks.Reference Roepke, Jaffee, Riffle, McGonigal, Broome and Maxwell 75 No significant difference in depressive symptoms was observed between a version of SuperBetter using CBT and positive psychotherapy (PPT) strategies and a general version of the app focused on self-esteem and acceptance.Reference Roepke, Jaffee, Riffle, McGonigal, Broome and Maxwell 75 This study was limited by a high rate of attrition and may not be generalizable due to recruitment from a self-help website, which may have captured a particularly motivated and/or hopeful segment of patients with depression.Reference Roepke, Jaffee, Riffle, McGonigal, Broome and Maxwell 75
The MoodHacker app was assessed vs access to relevant depression websites in a randomized controlled trial of 300 employed adults with mild to moderate depression.Reference Birney, Gunn, Russell and Ary 76 Participants using MoodHacker self-reported significant effects on symptoms of depression at the 6-week follow-up when compared with participants in the nontreatment arm.Reference Birney, Gunn, Russell and Ary 76 However, a general attenuation of effects was observed at the 10-week follow-up.Reference Birney, Gunn, Russell and Ary 76 Limitations of this study included the use of a convenience sample of interested individuals, the use of self-report surveys, and the use of compensation for completing assessments, which may reduce generalizability of results.Reference Birney, Gunn, Russell and Ary 76
The CBT-based intervention SPARX was assessed for noninferiority to treatment as usual in adolescents aged 12 to 19 years who were seeking help for depressive symptoms at primary care sites in a randomized controlled trial (n = 94 and n = 93, respectively).Reference Merry, Stasiak, Shepherd, Frampton, Fleming and Lucassen 77 SPARX was noninferior to treatment as usual following intervention and at the 3-month follow-up, as assessed by the observer-rated children’s depression rating scale-revised.Reference Merry, Stasiak, Shepherd, Frampton, Fleming and Lucassen 77 The study was limited by the clinical service setting, wherein data on treatment adherence were limited, as well as by the heterogeneity of “treatment as usual,” which primarily comprimised face-to-face counseling and varied based on primary care site.Reference Merry, Stasiak, Shepherd, Frampton, Fleming and Lucassen 77
Despite limitations, these types of digital health technology are rapidly evolving the delivery of care for depression and hold promise for improving the clinical management of depression. There are several categories of digital health technologies for depression (Figure 2), and each of these categories may play a role in addressing the gaps and challenges in the clinical management of depression.
Currently available technologies aimed at addressing challenges in awareness and prevention
The use of social media platforms can help increase awareness of depression and encourage patients to actively seek treatment and connect with physicians.Reference Latha, Meena, Pravitha, Dasgupta and Chaturvedi 18 Directly increasing patient and caregiver awareness of risk factors for depression can help promote proactive pursuit of treatment or incentivize visits to the clinic.Reference Eigenhuis, Waumans and Muntingh 78 There are also efforts to use a multimodal framework to combine heterogeneous sets of features obtained from social media data, including inferences of demographic information (eg, age, sex, and race), that can be crucial for stratifying our understanding of population-level epidemiology of mental health disorders like depression and help develop demographic-aware interventions.Reference Yazdavar, Mahdavinejad and Bajaj 79 , Reference Cacheda, Fernandez, Novoa and Carneiro 80
Currently available technologies aimed at addressing challenges in screening and diagnosis
Digital health technologies may help address barriers to the implementation of depression screening and provide objective assessment to facilitate diagnosis. Telemedicine is already being implemented successfully to allow remote visits with HCPs, thus helping to overcome challenges associated with diagnosis (eg, limited access to mental health resources/services and stigma associated with seeking diagnosis and treatment).Reference Barnett and Huskamp 81
Currently available technologies aimed at addressing challenges in treatment and monitoring
Digital technologies may also help guide treatment planning and medical decision-making and monitor or promote treatment adherence in patients with depression. Mobile apps such as MINDSTRONG are being evaluated for preemptive and early intervention of brain health care to improve clinical outcomes and reduce hospital visits.Reference Mazurek Melnyk, Hoying and Tan 82 VitalSignReference Xiong, Lipsitz and Nasri 6 software facilitates treatment selection, implementation, and revision for patients with depression.Reference Trivedi, Jha and Kahalnik 23 The software can be augmented by other models of care (eg, mental health navigation and behavioral activation teletherapy) and offers the potential to add-on research and quality improvement projects.Reference Trivedi, Jha and Kahalnik 23 Several studies have demonstrated the effectiveness of MoodGYM (a CBT-based intervention for depression that has been available since 2001 and used by over three-quarters of a million people) 83 in reducing depressive symptoms, but in some of these studies, adherence rates were low (10%-27%).Reference Christensen, Griffiths and Korten 84 , Reference Twomey and O’Reilly 85 Free online access to this intervention can potentially benefit underserved patient populations.Reference Twomey and O’Reilly 85
Although mobile apps targeting treatment adherence have been understudied, several recent examples have shown promise: AiCure uses a HIPAA-compliant and scalable AI platform to monitor treatment adherence 86 , Reference Bain, Shafner and Walling 87; Mango Health and Health Prize are incentive-based apps to improve access and adherence to prescribed treatments 88 , 89; Medisafe is an app that provides reminders and alerts for users to take their prescriptions, thus improving treatment adherence 83 , Reference McGuiness, Michaeli, Wang and Wade 90; and Innerworld is a social VR platform that can help patients with depression set and reach mental health goals and promote treatment adherence. 91 Some app-based digital therapeutics for depression use positive psychology approaches to promote app engagement, which may affect treatment adherence.Reference Marshall, Dunstan and Bartik 92
Digital therapeutics have been approved by the FDA for the treatment of depression. One example is Otsuka Pharmaceutical’s Abilify MyCite that combines pharmacologic therapy with a wearable sensor and a mobile app that help monitor treatment and enhance collaboration between patients and their providers. 93 Other digital therapeutics for the treatment of depression are available under FDA enforcement discretion and were intended as adjunctive digital interventions to reduce depressive symptoms: Deprexis (developed by Orexo and GAIA) is a software platform that uses CBT-based techniques and AI to personalize treatment and help patients with depression learn how to overcome negative thoughts and behavior patternsReference Twomey, O’Reilly, Bültmann and Meyer 94; SparkRx (developed by Limbix) is a mobile app that uses CBT-based techniques to treat adolescent depression 95; and Feel program (developed by Feel Therapeutics) 96 , 97 uses an emotion sensor (a wristband) combined with a mobile app to obtain real-time objective data of changes in emotional state and provide personalized interventions (eg, CBT program and virtual support from board-certified health coaches). The use of digital therapeutics may help provide consistent treatment benefits, reduce treatment-related AEs, and align the treatment expectations and goals of patients and HCPs; however, this may be a work in progress for some of the existing digital tools, including both PDT and non-PDT. 95 -Reference Keefe, Cañadas, Farlow and Etkin 102
Digital health technologies may help address privacy and security issues, as well as the structural or emotional barriers (including stigma) associated with personalized approaches to treating depression.Reference Trivedi, Jha and Kahalnik 23 For VitalSign,Reference Xiong, Lipsitz and Nasri 6 data are stored securely, and access to the data is closely monitored to ensure the confidentiality of these records.Reference Trivedi, Jha and Kahalnik 23 In addition, the remote patient assessment feature allows patients to complete the assessment at home using their own electronic devices; this potentially facilitates access to important and timely health data for resource-poor clinics that care for underserved and minority populations.Reference Trivedi, Jha and Kahalnik 23 The Health Rhythms app uses AI to tailor interventions and enable personalized treatment, 103 whilst the Feel program provides real-time support and evidence-based interventions, CBT, and remote data-driven personalized coaching. 96 , 97
Telemedicine or care coordination platforms can help provide remote care to rural areas or hard-to-reach communities, thereby enhancing patient-provider collaboration.Reference Falconer, Kho and Docherty 104 Services such as Valera Health, 105 BetterHelp, 106 Talkspace, 107 Silvercloud Health, 108 Ginger, 109 and Lyra HealthReference Agrawal and Prabakaran 110 provide access to integrated teams of healthcare practitioners, specialists, and therapists trained in managing MDD. In nonclinical settings, online peer support groups and other resources 111 - 113 can provide a safe environment for patients with depression to receive care and support. While there is a paucity of high-quality studies examining the effectiveness of online peer support groups, they remain promising for depression management.
The future: What is potentially attainable with digital health technologies
Technologies under investigation aimed at addressing challenges in screening and diagnosis
Digital biomarkers hold promise in enabling objective detection of depression. Ellipsis Health is an ML-based mobile app that detects depression using speech analysis of smartphone audio, which can be easily recorded and transmitted, potentially allowing accessible screening and monitoring of depression.Reference Lin, Nazreen and Rutowski 114 Kintsugi is a passive vocal biomarker instrument that uses short clips of free-form speech for depression screening. 115 , 116 Kintsugi’s technology can be integrated into enterprise call centers, telehealth platforms, and remote patient monitoring apps, potentially expanding access as well as standardizing the quality of screening in primary care settings. A multi-site fully remote e-clinical validation study that enrolled participants with MDD to evaluate the sensitivity and specificity of Kintsugi’s technology’s prediction has recently been completed. 115 Winterlight Labs has developed a tablet-based assessment that also uses speech analysis as a digital biomarker that can help identify depression and be used for long-term remote monitoring of behavioral health and treatment response. 117 There is an ongoing trial that uses this technology to examine changes in speech features over time in patients with psychiatric disorders (including depression). 118 Sonde Health is a voice-based technology that can be used as a digital biomarker for depression, with a sensitivity and specificity of 0.60 and 0.70, respectively.Reference Sverdlov, Curcic and Hannesdottir 119 , 120 This smartphone technology offers an accessible, objective, private, noninvasive, and cost-effective way to evaluate mental fitness. 120 Users have demonstrated high levels of engagement; the app keeps patients engaged when they are not seeing their HCP and allows the patient and their HCP to monitor measurements between appointments.Reference Sverdlov, Curcic and Hannesdottir 119 , 120 The app may also help HCPs evaluate treatment response. 120
Digital technology may facilitate a more personalized, convenient, and cost-effective approach to assessment.Reference McIntyre, Lipsitz and Rodrigues 121 A recent study has shown that a mobile app-based version of the established Cognition Kit Digit Symbol Substitution Test (DSST) correlated with the paper-and-pencil version of the test in patients with MDD.Reference McIntyre, Lipsitz and Rodrigues 121 Furthermore, these patients reported that the Cognition Kit DSST app was user friendly, easy to navigate, and preferable over the paper-and-pencil version of the test.Reference McIntyre, Lipsitz and Rodrigues 121 Further studies are required in healthy individuals to validate the promising findings reported by patients with MDD.Reference McIntyre, Lipsitz and Rodrigues 121 VitalSignReference Xiong, Lipsitz and Nasri 6 is an EHR-integrated web-based iPad app designed for use in clinics that facilitates screening and diagnosis in patients with depression using the two-item version of the Patient Health Questionnaire (PHQ-2).Reference Trivedi, Jha and Kahalnik 23 Patients screening positive are then prompted to complete a full PHQ-9 to aid HCPs in diagnosis and treatment planning. Follow-up assessments of symptoms, side effects, and adherence are recorded in an easy-to-search format that HCPs may use alongside clinical information recorded in the EHR.Reference Trivedi, Jha and Kahalnik 23 The software has been optimized for integration and interoperability and frequently undergoes upgrades to improve efficiency, accuracy, and quality of care based on feedback from HCPs.Reference Trivedi, Jha and Kahalnik 23 Screening efforts are focused on identifying patients with depression, and there are no additional screening assessments for those who screen negative on the PHQ-2; thus, patients with current suicidality who do not have significant depressive symptoms may be missed.Reference Trivedi, Jha and Kahalnik 23
Virtual reality environments can efficiently evoke and quantitatively score cognitive and emotional status and behavior in a reproducible, objective, standardized, dynamic, accurate, and culturally or contextually correct manner by using standardized and age-appropriate cognitive and emotional challenges in a computer-generated environment.Reference Bell, Nicholas, Alvarez-Jimenez, Thompson and Valmaggia 122 VR environment-based educational interventions can help mental HCPs update their skills and knowledge in a simulated environment, which in turn may improve the quality of health care and patients’ satisfaction.Reference Kyaw, Saxena and Posadzki 123
Technologies under investigation aimed at addressing challenges in treatment and monitoring
Whilst there exists a wealth of mobile apps aimed at the treatment of depression, most are not backed by robust scientific evidence, and there are few ongoing studies evaluating the use of mobile apps as interventions for MDD, highlighting a need for further clinically oriented and systematic validation and testing of such mobile apps.Reference Eis, Solà-Morales and Duarte-Díaz 124
CT-152 is a PDT developed by Otsuka Pharmaceutical and Click Therapeutics with the aim to be classified by the US FDA as a SaMD. 100 , 125 This mobile app delivers a digital cognitive-emotional training intervention (Emotional Face Memory Task) for the treatment of MDD; a pilot trial in 51 participants with MDD showed a greater reduction in the severity of depressive symptoms in patients who received the PDT compared with those who did not, although further studies are needed to elucidate its precise mechanism of action.Reference Iacoviello, Wu and Alvarez 126 , Reference Iacoviello, Murrough and Hoch 127 Pathway is another example of a mobile app for MDD management (tracking symptoms, medications, side effects, and treatment progress) that is under development. Based on a pilot feasibility randomized controlled trial, 18-week use of the Pathway app showed significant improvements in patient engagement and patient-provider communications compared with the usual care control group.Reference McCue, Blair and Fehnert 128
Efforts to develop treatment strategies for MDD and other psychiatric indications by combining pharmacologic therapies (eg, ADTs) with digital therapeutics (eg, mobile medical apps, which are medical devices approved/cleared by the FDA or conformed with the Medical Device Regulation (MDR [EU]) 2017/745Reference Keutzer and Simonsson 129) are ongoing,Reference Sverdlov, van Dam, Hannesdottir and Thornton-Wells 130 , Reference Bulaj 131 and there are opportunities to create integrated digital health ecosystems for the treatment of MDD.Reference Spadaro, Martin-Key and Bahn 132 Behavior theories such as the health belief model, the behavioral intervention technology model, and the just-in-time adaptive intervention (JITAI) model can help developers evaluate the feasibility of digital mental health services. For example, JITAI is an intervention design aiming to provide the right type/amount of support, at the right time, by adapting to an individual’s changing internal and contextual state and impact on patient-reported outcomes (PROs).Reference Nahum-Shani, Smith and Spring 133 , Reference McIntyre, Ismail and Watling 134 The availability of increasingly powerful mobile and sensing technologies underpins the use of JITAIs to support health behavior, in which the state of the individual can change rapidly, unexpectedly, and outside of standard treatment settings.Reference Nahum-Shani, Smith and Spring 133 However, the lack of publications investigating whether JITAI mechanisms lead to an increase in the effectiveness of the mobile apps highlights the need for further research, especially in real-world applications. There is a need to address the major gaps that exist between the growing technological capabilities for delivering JITAIs and research on the development and evaluation of these interventions.Reference Nahum-Shani, Smith and Spring 133
Digital technologies may soon facilitate long-term monitoring that in turn may help prevent the relapse and recurrence of depression. Remote measurement technologies can allow tracking of current depression status and identification of behavioral markers related to depression in a continuous and effortless manner for long-term passive monitoring.Reference Meyerhoff, Liu and Kording 135 Ecological momentary assessment can enable the collection and integration of multimodal data passively, continuously, and objectively and may be useful in assessing how depressive symptoms, including suicidal thoughts and behaviors, unfold in real-world contexts.Reference McIntyre, Lee and Rong 136 -Reference Kivelä, van der Does, Riese and Antypa 138 It is important that these technologies are validated for their sensitivity and specificity in detecting depressive symptoms. Multivariable prognostic models may aid in better prediction of relapse and remission of depression at the individual patient level, which in turn can support precision medicine and allow resources to be better targeted toward relapse prevention; such risk prediction tools are increasingly recommended by policymakers as they can be successfully incorporated into information technology systems in real-world practice.Reference Moriarty, Castleton and Gilbody 139 Digital phenotyping offers a powerful approach for forecasting risk for suicidal ideation over time.Reference Brown, Taylor and Bryan 140
The use of VR systems in conjunction with CBT programs addressing depression holds special promise for improving therapeutic effectiveness and patient engagement. A recent scoping review revealed that the use of CBT in a VR environment or the use of VR therapy in addition to CBT was an effective adjunct to the treatment of depression.Reference Baghaei, Chitale, Hlasnik, Stemmet, Liang and Porter 141 A review of 23 research studies showed that VR intervention was more effective in symptom management than the standard of care for depression.Reference Ioannou, Papastavrou, Avraamides and Charalambous 142 Viewing positive scenes in a VR environment has been shown to significantly decrease self-reported anhedonia, depression, and negative affect in patients with clinically significant depression.Reference Chen, Barnes-Horowitz, Treanor, Sun, Young and Craske 143 Whilst VR has been used for more than 3 decades in research laboratories and university clinics to facilitate CBT and other therapeutic approaches in behavioral medicine, its use in clinical care has yet to become commonplace. As VR systems now become more accessible and affordable, there is potential for large-scale implementation and a paradigm shift in the development and dissemination of VR in clinical practice.Reference Lindner 144
Limitations of digital health technologies in MDD and challenges to real-world implementation
Several challenges still need to be addressed for large-scale implementation of digital health technologies for the clinical management of depression. Whilst the application of big data to health care holds great promise, there are some hurdles to overcome. Firstly, symptoms related to depression, low mood, and tiredness may hinder the patient’s engagement with digital technologies.Reference Borghouts, Eikey and Mark 145 Patients’ mistrust in data sharing due to privacy concerns and confidentiality breaches may reduce their acceptability of digital health technologies.Reference Wies, Landers and Ienca 146
Existing studies on monitoring users’ well-being/depressive states via smartphones rely on correlation analysis to understand the association of well-being/depressive states with different aspects of context modalities and users’ interactions with smartphones. A key challenge is to uncover the causal links between the depressive states and the smartphone interactions and context modalities. Understanding the causal effects between them would enable ubiquitous computing researchers as well as doctors to build more effective behavioral interventions.Reference Mehrotra, Hendley and Musolesi 147 In addition to limitations on generalizability and possible selection bias, user engagement challengesReference Koh, Tng and Hartanto 148 and considerable dropout rates have presented challenges in clinical trials of smartphone apps.Reference Torous, Lipschitz, Ng and Firth 149
There are also high costs associated with implementation, training, and long-term maintenance.Reference Agrawal and Prabakaran 110 , Reference Marwaha, Landman, Brat, Dunn and Gordon 150 Digital health technologies are not equally available to all social groups due to economic, geographical, social, or cultural inequalities.Reference Wies, Landers and Ienca 146 This divide may hinder access for patient populations who could benefit most from these technologies.Reference Saeed and Masters 151 Mental health apps that saw a surge in downloads during the COVID-19 pandemic (eg, Headspace, Calm, Talkspace, Teladoc’s BetterHelp, and Noom) have since experienced declining downloads. 152 Whilst the rapid growth of digital health technologies during the pandemic was fuelled by the relaxation of regulations and increased insurance reimbursements, it remains unclear how much of this rapid expansion will be sustained and integrated across various countries given that many of the regulatory changes were temporary.Reference Kinoshita, Cortright and Crawford 153 The pathways to market for digital health products are evolving, and there is a lack of structured processes around evaluation and commissioning of such products.Reference Williams, Stott, Bromwich, Oblak, Espie and Rose 154 Many behavioral health apps are either unevaluated in clinical trials or claim evidential support by using an evidence-based foundation, such as CBT. Furthermore, expert-reviewed systematic frameworks for evaluating such apps have found inconsistent and/or contradictory results.Reference Carlo, Hosseini Ghomi, Renn and Areán 155 The FDA Software Precertification (Pre-Cert) Pilot Program findings reported that the speed of innovation may necessitate new statutory authority to foster innovation while continuing to provide reasonable assurance of safety and efficacy. 156
Perspectives and needs of patients and caregivers
There is value in identifying the digital features that are associated with greater improvements and the patient populations most likely to benefit from specific mobile app interventions.Reference Serrano-Ripoll, Zamanillo-Campos, Fiol-DeRoque, Castro and Ricci-Cabello 157 To improve usability and accessibility of digital health technologies, it would be beneficial to collaborate with patients throughout the entire process: from idea creation and design, to testing, and finally implementation.Reference Henni, Maurud, Fuglerud and Moen 158 The value of cocreation has been well studied in other sectors, but there are few examples of effective cocreation within the digital mental health landscape. The availability of digital health platforms and online health communities may facilitate cocreation by encouraging transparent communication with patients, improving health literacy (in patients and healthcare organizations), providing informational and social support to patients, and empowering patient self-management.Reference Rezaei Aghdam, Watson, Cliff and Miah 159
Payors’ perspectives
In the US, the Centers for Medicare and Medicaid Services has recently approved a new category of digital health services—Remote Therapeutic Monitoring—to complement the existing suite of Remote Physiological Monitoring codes covered under Medicare. 160 Whilst this was an important milestone, how payors in general would cover costs associated with digital health services could greatly impact the uptake of digital health technologies in mental health care.Reference Hayes, Dawson and McCoy 161 In the EU, reimbursement processes for digital health technologies are beginning to take shape; Germany, Belgium, and France have standardized reimbursement processes in place or soon to be launched, while other EU countries have expressed interest in implementing standardized reimbursement processes for digital health technologies. 162
Conclusions
Digital health technology has facilitated the clinical management of depression and will likely have future growth with continued expansion and integration in patient care. Ongoing efforts to optimize and validate the use of digital health technology should provide a personalized medicine approach in detecting, treating, and monitoring depression.
Acknowledgments
The authors thank Liting Hang, PhD, and Brilee Coleman, PhD, of MediTech Media, Ltd, Atlanta, GA, USA, for providing writing/editorial support, which was funded by Sage Therapeutics, Inc., and Biogen Inc.
Author contribution
Conceptualization: R.S.M., G.B., S.T.T., G.M., D.S., A.C., G.S., M.G., R.J.; Methodology: R.S.M., G.B., S.T.T., G.M., A.C., M.G., R.J.; Project administration: G.M.; Resources: G.M., G.S.; Supervision: G.M., R.J.; Visualization: G.B., S.T.T., G.M.; Writing—original draft: R.S.M., W.G., G.B., S.T.T., G.M., D.S., A.C., G.S., M.G., R.J.; Writing—review and editing: R.S.M., W.G., G.B., S.T.T., G.M., D.S., A.C., G.S., M.G., R.J.
Financial support
This work was supported by Sage Therapeutics, Inc., and Biogen Inc.
Disclosure
R.S.M. has received research grant support from CIHR/GACD/National Natural Science Foundation of China (NSFC); speaker/consultation fees from Lundbeck, Janssen, Alkermes, Neumora Therapeutics, Boehringer Ingelheim, Sage, Biogen, Mitsubishi Tanabe, Purdue, Pfizer, Otsuka, Takeda, Neurocrine, Sunovion, Bausch Health, Axsome, Novo Nordisk, Kris, Sanofi, Eisai, Intra-Cellular, NewBridge Pharmaceuticals, AbbVie, and Atai Life Sciences. R.S.M. is the CEO of Braxia Scientific Corp. G.B. has received research support from the ALSAM Foundation and is a coinventor on US patents 9 569 562 and 9 747 423 “Disease Therapy Game Technology”; these patents are related to digital health technologies and are owned by the University of Utah. G.B. is the founder and owner of OMNI Self-care, LLC, a company creating digital content to promote disease self-management. S.T.T. and W.G. do not have any conflicts to report. R.J. has received research support from AbbVie/Allergan, Lilly, Lundbeck, Otsuka, Pfizer, Shire, and Takeda; speaker/consultation fees from AbbVie/Allergan, Acadia, Adamas, Alfasigma, Akkermes, Axsome, Biogen, Boehringer Ingelheim, Cingulate Therapeutics, Corium, Eisai, Evidera, Impel, Intra-Cellular Therapies, Ironshore Pharmaceuticals, Janssen, Lilly, Lundbeck, Merk, Neos Therapeutic, Neurocrine Biosciences, Osmotica, Otsuka, Pamlab, Pfizer, Sage Therapeutics, Shire, Sunovion, Supernus, Takeda, Teva, Transcend Therapeutics, and Tris Pharmaceuticals; and has served on advisory boards for Adamas, Alkermes, Corium, Eisai, Janssen, Lilly, Lundbeck, Merck, Neos Therapeutics, Neurocrine Biosciences, Otsuka, Pamlab, Sage Therapeutics, Shire, Sunovion, Supernus, Takeda, Teva, and Usona. G.M. and D.S. are former or current employees of Biogen Inc. and may hold stock. A.C., G.S., and M.G. are employees of Sage Therapeutics, Inc., and may hold stock and/or stock options.