Policy Significance Statement
Humanity is facing one of its greatest challenges in decades that requires governments to take complex decisions. The digitization of our society and the telecommunications sector has pushed the development of Big Data and artificial intelligence, offering unique opportunities to develop tools based on data. Much of these data are generated from the private sector and can be safely offered to governments for better-informed decisions and policymaking. For the case of mobility indicators, the evidence of this value has been demonstrated. However, there are still barriers in both sectors to scale these tools effectively and widely. We believe that the commitment to the complete digital transformation of the public sector, the creation of regulatory frameworks in accordance with the use of data for the public interest, and the incentives for companies to invest in these technologies and capacity building are key to their future development.
1. Introduction
The COVID-19 pandemic is the first event in decades that produces first-order negative impacts in public health and economies at global scale. Due to its high infection capability, human mobility and close social interactions are among the reasons for the rapid spread of the virus. In general, mobility is one of the main factors that causes the spread of infectious diseases (Wesolowski et al., Reference Wesolowski, Buckee, Engø-Monsen and Metcalf2016). In the case of SARS-CoV-2, initial studies highlighted the relation between human mobility and epidemic growth rate in China (Chinazzi et al., Reference Chinazzi, Davis, Ajelli, Gioannini, Litvinova, Merler, Pastore, Pionti, Mu, Rossi, Sun, Viboud, Xiong, Yu, Halloran, Longini and Vespignani2020; Kraemer et al., Reference Kraemer, Yang, Gutierrez, Wu, Klein, Pigott, du Plessis, Faria, Li, Hanage, Brownstein, Layan, Vespignani, Tian, Dye, Pybus and Scarpino2020). These studies also showed that mobility can be used to predict the spread and size of the epidemic and that mobility restrictions are effective especially in the early stages of the outbreak (Aleta et al., Reference Aleta, Martin-Corral, Pastore, Piontti, Ajelli, Litvinova, Chinazzi, Dean, Halloran, Longini, Merler, Pentland, Vespignani, Moro and Moreno2020; Pepe et al., Reference Pepe, Bajardi, Gauvin, Privitera, Lake, Cattuto and Tizzoni2020).
As a result of the epidemiological emergency, national and local governments started to implement policies of social distancing and lockdown in order to limit the contact rate and therefore reduce the spread of the virus. These policies are proved effective but deeply affected the mobility of societies and had an important impact in the economy. One of the first needs that governments had were tools to evaluate to which extent the lockdowns imposed were followed by the general population and what was its impact in the spread of the virus. The feedback given by such tools is key to evaluate when to lift or ease restrictions in order to control the spread of the virus.
The literature has extensively reported in the past the capabilities of using anonymized and aggregated mobile network data for charactering mobility and the spread of viruses. One of the first examples of that was implemented during the Haiti 2010 earthquake and subsequent cholera outbreak (Bengtsson et al., Reference Bengtsson, Lu, Thorson, Garfield and Von Schreeb2011). Nevertheless, the first time that such information was used to model the spread of an epidemic was done in Mexico during the H1N1 outbreak of 2011 (Frias-Martinez et al., Reference Frias-Martinez, Williamson and Frias-Martinez2011), which showed the potential of combining susceptible, infectious, or recovered (SIR) epidemiological modeling with cell phone mobility. The use of telecommunications company’s (telco) data to tackle epidemiological crisis and natural disasters was increasingly adopted, and it was finally during the 2013–2016 West Africa Ebola outbreak that reached public institutions (Wesolowski et al., Reference Wesolowski, Buckee, Bengtsson, Wetter, Lu and Tatem2014). From that point on, the use of cell phone traces for epidemiological emergencies has received a lot of attention (Wesolowski et al., Reference Wesolowski, Buckee, Engø-Monsen and Metcalf2016; Panigutti et al., Reference Panigutti, Tizzoni, Bajardi, Smoreda and Colizza2017). Such approaches have been taken to model the spread of Ebola (Wesolowski et al., Reference Wesolowski, Buckee, Bengtsson, Wetter, Lu and Tatem2014), Zika (Ajarethinam et al., Reference Rajarethinam, Ong, Lim, Tay, Bounliphone, Chong, Yap and Ng2019), cholera (Bengtsson et al., Reference Bengtsson, Gaudart, Lu, Moore, Wetter, Sallah and Piarroux2015), malaria (Wesolowski et al., Reference Wesolowski, Eagle, Tatem, Smith, Noor, Snow and Buckee2012; Ruktanonchai et al., Reference Ruktanonchai, DeLeenheer, Tatem, Alegana, Caughlin, zu Erbach-Schoenberg, Lourenço, Ruktanonchai and Smith2016), and dengue (Wesolowski et al., Reference Wesolowski, Qureshi, Boni, Sundsøy, Johansson, Rasheed, Engø-Monsen and Buckee2015), among others. In this context, when the SARS-CoV-2 outbreak started, the use of cell phone traces and, in general, of any digitally generated mobility traces, was rapidly adopted by the international community as a key tool to fight the pandemic. As an evidence of such interest, the research community announced several public calls to make aggregated and anonymized mobile phone data available (Buckee et al., Reference Buckee, Balsari, Chan, Crosas, Dominici, Gasser, Grad, Grenfell, Halloran, Kraemer, Lipsitch, Metcalf, Meyers, Perkins, Santillana, Scarpino, Viboud, Wesolowski and Schroeder2020; Oliver et al., Reference Oliver, Lepri, Sterly, Lambiotte, Deletaille, De Nadai, Letouzé, Ali Salah, Benjamins, Cattuto, Colizza, de Cordes, Fraiberger, Koebe, Lehmann, Murillo, Pentland, Pham, Pivetta, Saramäki, Scarpino, Tizzoni, Verhulst and Vinck2020)
In this paper, we present how Telefonica has supported decision-making processes by providing high-frequency and low-latency mobility insights for governments. We focus on some of the countries where Telefonica operates in Europe and Latin America: Argentina, Brazil, Colombia, Ecuador, Germany, and Spain. Other data-sharing initiatives were conducted in countries like Chile or the United Kingdom, but for the sake of length, we focus on the above-referred ones. The pro bono efforts and productive collaboration with governments along 2020 exemplify the strong commitment of Telefonica and the telco industry with the response to the pandemic and society’s development.
2. Argentina: Effective Collaboration Among Telefonica, the Academia, and the National Government
Telefonica Argentina was part of a public–private collaboration that started with a request for data and analysis by the national authorities at the beginning of the health crisis in March 2020. To respond to the government’s request, Telefonica signed a collaboration agreement with the National University of San Martín for the construction of an analytical hub through which the aid was materialized. Telefonica also signed agreements for the use of the tool with the National government and provincial agencies. The funding of the project was philanthropic until the end of 2020. The evolution of the pandemic in the last quarter of 2020 will determine the new scope of the project for 2021.
The initial requirements were related to the measurement of the citizen mobility throughout the Argentinian territory in order to (a) supervise the fulfillment of the isolation measures and (b) later monitor the reopenings. To this end, a mobility indicator called “Citizen Mobility Index” (IMC) was built, which indicates, from 0 to 1, a level of mobility in each locality of the country relative to the prepandemic mobility levels. The IMC index represents the relative level of mobility (number of journeys detected) of a certain population in relation to the average movement of a normal week. For example, an index of 0.5 means that the population moved 50% of what it used to move in an average week prior to the pandemic.
A forecasted index was also developed to help authorities assess if the mobility index is within the expected range. All the data used to build the index were anonymized and then aggregated by location.
It was a bespoke development, but many of the Big Data capabilities that provide commercial service to the operator were used. The delivery of the IMC is provided through a user-restricted website (https://www.pulsocovid.com). The data are updated daily with a latency of approximately 12 hr. The website contains a dashboard (Figures 1 and 2) with the evolution and geographic distributions of the indicator. The web tool was offered equally to all national agencies and then, at their advice, offered to all provincial authorities in the country.

Figure 1. Interactive map showing (a) mobility indexes in departments and towns (% Movilidad Localidad) and (b) forecasted mobility index (% Esperable).

Figure 2. Interactive dashboard showing historical mobility indexed along different towns and cities.
Currently, the tool is used by the provincial governments and the Ministry of the Internal Affairs as a source of information for daily decision-making (mobility control) and every 2 or 3 weeks when deciding on reopening or opening/lockdown policies.
Privacy by design was key. All the data used in the analysis complied with the following guidelines: (a) anonymization from the primary source of data, (b) aggregation, and (c) the opening by location must meet a minimum number of cases that prevent the indirect reidentification of individuals.
Regarding the communication plan, it was agreed to provide information reactively in most cases. However, and due to, among other aspects, official mentions made by government members, it had a great impact in the formal media. Thus, spokespersons of Telefonica and the National University of San Martín provided details on the methodology.
Telefonica highlights, as one of the main achievements, the rapid development of a customized tool that managed to meet privacy and utility requirements simultaneously. Multiple challenges also emerged. The main one was related to the cultural shift required to convince government stakeholders about the trust and privacy-preserving design of the tool. It required explanatory sessions about the use of the tool to national and provincial governments, since not all had the appropriate management teams to exploit the tool.
Telefonica was also challenged to protect its reputation. The company explained, whenever requested by the press and different opinion formers, how the tool complies with all the privacy policies. This project also highlighted the clear opportunities for codevelopment with the different actors in the public sector.
3. Brazil: The Telco Industry Joining Forces to Fight Against the Pandemic
Following the WHO statement recognizing COVID-19 as a global pandemic, Vivo (Telefonica operating business in Brazil) along with the main telco companies in the country decided to start a proactive collaboration. Vivo was prepared to develop the project in a short time, thanks to the sustained investment in their Big Data and Artificial Intelligence (AI) capabilities, over the last 5 years.
The task force was formed by the telco industry (Vivo, Claro, Tim, and Oi) and ABR Telecom: Brazilian Telco Association, a neutral agency that acted as a mediator in the data exchange process. Cloud and data visualization providers that focused on the visual representation of the insights also joined. The collaboration was designed as a philanthropic contribution, starting in April 2020 until December 2020. The evolution of the pandemic in Brazil will determine how these efforts will be kept during 2021.
Framing the collaboration was a complex process. There were technical challenges to integrate data from different companies in order to create uniform and robust mobility indicators. Operational challenges like the short timing for delivery or regulatory issues also raised. The dynamic of the collaboration articulated around a task force with representatives from all the stakeholders as well as intensive communication with public authorities. Internally, much of the efforts were devoted to create highly accurate processes for daily validations before dashboard updates. The government also required day-to-day collaboration for better decision-making in policies to combat the pandemic. Transparency about information sharing was also important for the success of the project.
Two use cases were prioritized: (a) Monitoring social distancing by providing a social isolation indicator. This is a key metric that was used extensively by local governments in Brazil as authorities implemented a soft lockdown. The algorithm to build the indicator of social isolation by municipality and state is based on the calculation of the volume of mobile lines per antenna. The index summarizes the volume of phone lines that remain in the same place throughout the day compared with the night hours of the previous day, which creates a quantitative estimate of the volume of people who stay at home (or close to).
As the data are aggregated by antenna and subsequently at the municipality and state level, the anonymity and security of the information is guaranteed. The index was the most reliable way to obtain a daily reference on how population followed the advice. The methodology behind this indicator is to use the volume of connections to cell sites, comparing the nighttime period to the mobility during the day (Figure 3).

Figure 3. Dashboard representation of the social isolation rate.
(b) Mobility trends. Insights are represented in a dashboard that allows the user to have a square view of 4 km², which mapped users’ behavior in the previous period (baseline) compared to the current day (Figure 4).

Figure 4. Dashboard for the mobility trend analysis.
Both indicators were proposed by telco operators since, in addition to addressing all Ley General de Protección de Datos (LGPD) (information security) issues, they met the needs for planning actions to combat the pandemic.
Based on the insights, it was possible to offer intelligence and inputs to take the best actions against the pandemic, such as opening commercial activities, anticipating holidays, blocking strategic routes, extending the vehicle rotation, identifying and preventing areas with a high number of people, and management and prevention in points of interest (hospitals and airports).
Both use cases were implemented as a bespoke development, but it was possible to advantage on some existing variables and models created for other internal use cases. The insights are updated daily, from the data generated by the mobile network the day before. The insights were made available through a website that includes a dashboard with time series of metrics and heatmaps. The access is private and restricted to governments that requested permission. It was governments’ decision when to provide the insights to the general public, as in the case of the Sao Paulo Government (see Figure 5).

Figure 5. Indicators published by the Government of Sao Paulo (https://www.saopaulo.sp.gov.br/coronavirus/isolamento).
The legal and institutional affairs teams carefully reviewed ethics and regulatory aspects, which includes ensuring privacy of the data. Privacy protection was guaranteed by the anonymization and subsequent aggregation (at the level of municipality and state) of the data.
The consortium also devoted much attention to the creation of a communication plan to the public. This was important to raise awareness on how the telco industry contributes to combat the pandemic but also to build trust on how data were handled. The collaboration was communicated widely through the open media. Initially, there were some specific concerns about privacy policy, but after explanations about transparency and that the project is GDPR compliance, it was very well received by the community.
In summary, this an example of the great commitment of the telco industry with the Brazilian society. There were significant challenges like the development of use cases together with the other three telcos, respecting data privacy and creating homogenous criteria for data analysis. In addition, implementing the project with a short timing and regularly disseminating outcomes was also challenging.
It is noteworthy the excellent collaboration among telcos and the neutral role of the ABR Telecom association. A previous and recurring definition of some neutral and specialized entities in different areas could speed up the data sharing for future projects. Vivo is highly confident in the development of more initiatives that positively impact our society and, at the same time, create value for the reputation of the company. In addition, as an additional learning, the transparency in the actions carried out and closer ties between public and private sectors will help to prepare for future pandemics.
4. Colombia: Mobile Network Operator Data to Boost the Research on the Pandemic
The Ministry of Science, Technology and Innovation of Colombia launched an innovation call named “MinCienciatón,” seeking to find technological answers to the COVID-19 pandemic. Telefonica Movistar Colombia was interested in providing data to one of the shortlisted projects. It was a proposal for systemic models to simulate the dynamics of the pandemic in five cities. A multidisciplinary team of researchers leads the project “COVID-19 Epidemiological Intelligence System (SISCOVID)” from the University of Los Andes, National Consulting Center, and University of Ibague, in alliance with researchers from the University of Liverpool, Northwestern University, Santa Fe Foundation, and Telefonica Movistar Colombia. The project aims to study the dynamics of COVID-19 through mathematical and computational simulation models supported by mobility data (including mobile network operator [MNO] data) and surveys, to provide evidence for decision-making in the cities of Barranquilla, Bogotá, Cali, Cartagena, and Medellín. The collaboration is aligned with the company´s “Big Data for Social Good” initiative that advocates the use and share of data for sustainable development.
Following the request of the leading research group, Movistar’s shared 3 weeks of data for this purpose, which included origin–destination matrices, within cities (neighborhood/commune level), grouped by age ranges, and timetables for two of the five cities: Barranquilla and Cartagena.
Two deliveries were made: the first was on July 17, when all the stricter quarantine orders were in place; and the second on September 15, 15 days after the end of the strict quarantine, to compare the behavioral change.
The final deliverable was a database with an origin–destination matrix within each city, and within each of the boroughs and neighborhoods, by time slot, indicating the volume of commuters both outbound and inbound from home. It also offers the possibility to classify by age and gender. This allowed the research group to carry out contrasts with the mobility data patterns before the pandemic, and to estimate the speed of spread of the infection.
Regarding privacy issues, Movistar has guidelines and principles regarding the use of data in Big Data projects, which were applied to review the information delivered. All data handled to the researchers were anonymized and aggregated at origin. The analysts of Telefonica classified each anonymized mobile phone journey into time intervals (morning, afternoon, and night), considering the time at origin and destination. The research group defined the time limits of the three intervals. For each of the time bands, the location in which the user remained connected the longest was determined. Then, the matrices were created considering the points with the longest connection time of each strip. In addition, for each cell phone line, it was assigned an age band attribute. Then, origin destination matrices were constructed with two levels of aggregation: time of the day and age band.
In all cases, the data delivered are volumes of people who moved from one place to another with a minimum threshold of individuals per group. This, along the absence of personal information, guarantees that no reidentification of individuals is possible, in compliance with the country’s legal regulations.
The result of the research shows a great progress in two mathematical simulation models: compartmental susceptible exposed infected and resistant (SEIR), and agent-based model. Those allowed simulating scenarios with different interventions in cities and predicting how different indicators would behave in each city (Figure 6), infections by SARS-COV-2, number of hospitalized, or number of deaths, among others. The project information is available at https://siscovid.com. It has been used by the different cities to analyze the impact of the decisions taken to counter the effects of COVID-19 in 2020. This information will also be key in determining when outbreaks of the virus could emerge in the future.

Figure 6. Dashboard with relevant indicators, as part of the SISCOVID website.
We shall highlight the value of private sector and academia working together for a greater contribution to society. This project reinforces our belief that MNO data can a make a real difference in solving society’s challenges.
5. Ecuador: Fast Response to the Information Request Issued by the National Government
As a response to the global health crisis caused by COVID-19, the National Emergency Operations Committee created a situational room made up of several public institutions such as the Ministry of Telecommunications and the Information Society (MINTEL), and many other ministries and public agents. In this context, MINTEL raised the objective of developing a technological tool that allows making decisions to contain the health emergency based on evidence and data analytics, through the use and exploitation of information from digital and technological tools.
The MINTEL coordinates the development of a technological ecosystem whose primary source of information is the telcos operating in Ecuador. The information provided by these companies about population’s mobility levels is essential for the development of early warnings in the context of the health emergency, as well as a solid basis for the evidence-based decision-making process.
This collaboration is within the framework of the State of Exception established by the National Government, which lasted from March 16 to September 13, 2020. Thus, the telcos are required to deliver free of charge daily mobility indicators to MINTEL. The scope and use cases of mobility indicators are defined only by the national government. Therefore, information on use cases and the decision-making process is limited.
Telefonica Ecuador and the National Government had previous collaboration experience related to managing and processing information on mobility and analysis of migratory flows. This fact facilitated the information to be delivered in a timely manner. The deliverables were aggregated datasets and reports with mobility indicators at different geographic aggregation levels. The metric used to better understand the trends in mobility and the impact of mobility restrictions was the percentage of mobility reduction among municipalities. This was crucial in the assessment and effectiveness of mobility restriction measures in Ecuador. The development of such indicator was internal, but it was clearly defined, so it was easy to understand for authorities. Thus, they were aware of its importance for policymaking in the context of the pandemic.
Data were anonymized, aggregated, and extrapolated. The information provided to authorities did not required further anonymization. The indicator computed the daily variation of movements generated and attracted to each municipality compared to a prepandemic average.

where TM is the total of movements in municipality i, and mgi and mai are the total of movements generated and attracted by municipality i.

where TMi,d is the total of movements in municipality i in day d, and
$ \overline{TM_{\iota }} $
is the average total of movements in municipality i prior to the pandemics.
Telefonica leveraged synergies in the development of Big Data and mobility studies in the context of forced displacements, tourism, and transport analysis for both public bodies (Ministry of Tourism and National Transit Agency) and multilateral organizations (World Bank and Inter-American Development Bank). Therefore, it was not necessary to develop the deliverables from scratch. Prior to the development of the government order, the Big Data team at Telefonica Ecuador had already initiated predictive analysis internally that would allow it to identify, if necessary, the rate of spread, the speed of spread, and the direction of spread (Figure 7). Then, there was some previous familiarity with the requirements.

Figure 7. Dashboard with relevant metrics about mobility and the spread of COVID-19 in Ecuador.
Due to the experience in regulatory and technical issues in the treatment of sensitive mobility information generated by Telefonica Ecuador, this process did not imply major setbacks for the development and delivery of information. However, it was observed that the interinstitutional coordination by MINTEL presented difficulties due to the number of actors involved and the guidelines for the delivery of information.
Due to the sensitive information handled, the regulatory vice president of Telefonica Ecuador channeled and moderated the requirements issued by the National Government. In this way, the information requests were at all times aligned with the legal framework in force in Ecuador. In this sense, the information provided by Telefonica Ecuador ensured privacy by providing only aggregated and anonymized data. Consequently, the National Government, having consolidated information from the rest of public institutions and companies, was the only stakeholder entitled to communicate outcomes externally.
One of the most important challenges resulted from the number of actors involved in the collaboration. In addition, the lack of guidelines and standards for the information to be delivered by all the data providers was a significant barrier toward creating homogeneous quality standards. The collaboration did not imply an obstacle to the reputation and privacy policies implemented by Telefonica Ecuador. The company was well prepared to manage the risk, due to the technical and legal experience in the execution of Big Data projects with mobile network data. However, decision-making by the end user could be improved by involving Telefonica more actively, given that it has more experience in handling such insights.
Finally, this project did help to create public awareness on the importance of the mobility information available, thanks to the investment in Big Data and AI. In addition, it demonstrates the usefulness of this information to face crises such as the health emergency caused by COVID-19. This, in turn, implies that national authorities place more emphasis on the use of tools that allow evidence-based decision-making, which will promote the application of more effective policies that benefit the society.
6. Germany. Synergies with Existing Commercial Partnerships Boosted the Response to the Pandemic
As the COVID-19 pandemic started to evolve in Germany in early 2020, and infection rates developed in a way near exponential growth, the German government introduced several lockdown measures (e.g., closure of nonrelevant businesses and travel restrictions) in order to get the spread of the infection under control.
Shortly afterward, Telefonica Germany was approached by two companies in the market of mobility behavior analytics (Teralytics and Senozon). They both had a request from the Robert Koch Institute (RKI), which is the leading authority for public health in Germany, for the delivery of analytic reports on the effect of the introduced lockdown restrictions. While Teralytics was already a long-standing partner of Telefonica Germany, the partnership with Senozon had to be formalized. The collaboration with Senozon was launched originally only with a temporary scope, to support the COVID-19-related analyses, but is likely to evolve into a permanent one now.
The requirements of the RKI were an analysis, which should show how the movement of people in different regions of Germany has changed after the introduction of the lockdown measures, in form of, for example, a heatmap that displays where people have significantly reduced travel compared to the time before the lockdown. In addition, the report should show where people were traveling to and from, before and after the lockdown, again on a county base (Figure 8).

Figure 8. Visual representation of mobility indicators and origin–destination matrices (source: Teralytics).
In order to create the reports, Telefonica Germany provided anonymized data of network events to both partners, which created the analysis based on these data. Our data protection, legal, and regulatory experts, of course, performed a review of the particular use case. In both cases, the data necessary for the reports were provided free of charge.
The platform of Telefonica Germany which is used in anonymization process called “data anonymization platform” (DAP)Footnote 1 is existing already for several years and serves as base for similar commercial business partnerships. The process during which data are anonymized and processed in a way that no deanonymization and no conclusion on individual customer are possible anymore is approved by the German ministry for data protection (Federal Commissioner for Data Protection and Freedom of Information [BfDI]). The concept of patented DAP is based on technical and organizational separation of the individual parts of the anonymization process, which performs a segmentation, transformation, and aggregation, which leads to anonymity. The so-called zones are operated by different service providers, whereby no “zone operator” is able to identify a single person. This is ensured through the use of different techniques like K-anonymity, differential privacy, complex cryptography procedures, key management, and other procedures, which we combined in a smart way. The DAP was developed with the German BfDI and is certified by TÜV Saarland.
The main part of the daily interactions necessary to create and maintain the reports for the RKI took place between the RKI and Teralytics and Senozon. Telefonica Germany ensured the constant flow of data to the partners along the support to enable the partners. As we provide the mobile network data to our analytical partners on a near real-time basis, Teralytics and Senozon deliver updated dashboards next day within the morning.
The partners created dashboards for the RKI, which showed the changes in the movement behavior of people in Germany as configurable heatmaps and OD matrices on county level and calculation of, for example, path lengths and time of travel per ZIP-code area (Figure 9). To use standard OD matrices on a district level for the RKI was a decision of the RKI, because the group of scientists at the RKI are already working with this geographical granularity. Within the public information on a daily basis in newspapers, the amount of journeys per district was taken as a very easy to understand KPI for everyone and suggested by Teralytics. The reports are also shared with Telefonica. In some cases, the reports are even publicly available on the Internet or TV (as a screenshot). The largest German newspaper (“Bild Zeitung”) used the Teralytics report to create a so-called “Corona Radar” on their website, where everybody could check how the lockdown measures were impacting the travel behavior in his specific region.

Figure 9. Examples from the Senozon dashboard. Left: general movement metrics (like path length). Right: average travel distance by ZIP code.
Based on the dashboards, the German government could judge whether the movement restrictions introduced as part of the overall lockdown were effectively limiting people’s travel behavior. The collaboration (and the reports) is still in place; however, since the lockdown has ended in the meantime, the current relevance has dropped. German researchers are also using our daily data to feed different models that describe how the pandemic in Germany develops and why. This research is a collaborative effort of members of the Computational Epidemiology Project Group at the RKI in Berlin and the Research on Complex Systems Group at the Institute for Theoretical Biology and IRI Life Sciences at Humboldt University of Berlin. See also https://www.covid-19-mobility.org.
Telefonica Germany announced the support of the RKI with anonymized data in press release earlier this year. While the overall reactions to this announcement were very positive and Telefonica was perceived as a contributor to the joint efforts to fight the pandemic, some critical voices questioned whether the anonymization process was indeed set-up in a way that deanonymization of data was totally prevented. Telefonica already expected this fact. Therefore, we informed the BfDI formally about our support for the RKI before we started because of the expected high public awareness. Looking back, it was exactly the right way. Our COVID-19 support for Germany finds the way into the most important news program of the day, the “Tagesschau” at prime time and many other top newspapers.
The usage of this kind of analysis as one tool in the effort necessary to control the pandemic development in Germany showed the value that analysis based on data from mobile networks can have. In fact, we experienced an increased demand for this kind of analytic reports through our partners shortly after the use cases were made public.
7. Spain: The National Statistics Office Pioneering the Use of Telco Data to Fight the Pandemic
Months before the start of the crisis, the Spanish National Statistics Office (INE) put out to tender a public procurement of telco data, which was attended by the three main operators in the country (Telefonica, Vodafone, and Orange). INE, for the first time in its history, defined a framework to use mobility data as a source on the statistical production of the Population and Housing Census. The first proposed project was the measurement of mobility from different angles, such as the daily commuting of the Spanish population. The INE has carried out similar studies based on census information, which do not consider the dynamic nature of daily and seasonal mobility. Thus, when the crisis broke out in early March 2020, the INE was already obtaining mobility indicators based on telco data generated by 80% of the country’s mobile lines. To adapt the indicators to the context of the pandemic, a subproject of the previous one was created, in which the indicators were customized to quantify the degree of mobility or confinement with respect to the situation prior to the pandemic.
The collaboration model is based on the leadership of the INE, who required each operator to supply origin–destination matrices for daily mobility. These are aggregated and anonymous data that quantify the volume of journeys between geographical areas (cells) defined by the INE. It is important to note that each operator anonymized the data at source and excluded any type of sociodemographic variable, preventing reidentification and ensuring the privacy of users. In the case of Telefonica, we applied the same privacy-protection procedures as for the rest of commercial projects. For example, the minimum amount of individuals reported for any origin–destination combination is 15, in compliance with the national regulatory guidelines.
The agency also provided the operators with methodological recommendations for the matrices to be generated with a common criteria. The INE integrated the datasets and created the mobility indicators from the OD matrices (provided by the three operators). A dedicated taskforce was created made up of members of the three operators and the INE, running daily meetings and actively exchanging information. The statistical office did the integration of each operator’s datasets into a single dataset, and subsequently produced the output indicators. All the external communication was also led by the INE (https://www.ine.es/covid/covid_movilidad.htm), which minimized the concerns of the Spanish public opinion about privacy and the use of telco data. Transparency in communicating the project was key. The project was showcased in the media on national TV channels and newspapers. The INE openly offers reports, visualizations, and output indicators. During the state of alarm (between March and May 2020), the operators provided the data free of charge daily. The set of indicators are generated at least until the end of 2020. For the INE, it was a highly complex project, since, in addition to the strong pressure to generate high-frequency indicators, it was the first time they worked with telco data.
Another key aspect was Telefonica’s experience (through its LUCA data unit) in working with mobility data. This facilitated rapid technical developments, but also ensured the effective application of strict ethical, legal, and privacy requirements, in compliance with the existing regulation.
Undoubtedly, the biggest challenge Telefonica faced in this project was the short time and latency to provide data updates. The effort was worth it as this type of projects help public administrations to become aware of the impact on decision-making based on Big Data, especially in a context of national emergency. However, we consider that there is still a long way to go for administrations to effectively incorporate data from the private sector into management in an agile manner.
Telefonica Spain has also shared anonymized and aggregated mobility data, together with 14 other European telecommunication providers, with the European Commission to develop insights and tools to support European recommendations to the European Center for Disease Control (ECDC) and the eHealth Network (comprised of member states health policymakers). More information on this subject can be found in the paper published by the European Commission for the same special collection (EU Science Hub, 2020).
8. Conclusions
The rapid spread of SARS-CoV-2 worldwide motivated the implementation of reduced mobility policies to mitigate the pandemic. As a result, governments and public institutions needed data-driven insights to evaluate and measure the impact of the decisions implemented. In this context, Telefonica took the initiative to start collaborations with governments and other institutions in the countries where it operates to deploy such tools. The pandemic impacted differently in the countries discussed in the article, so the response of the different national governments was also different. Each Telefonica Operating Business decided locally the best approach to support the government’s response, but, at the end, many similarities emerged among those approaches. The main parameters that defined each of the country initiatives are summarized in Table 1.
Table 1. Summary of the data-sharing initiatives developed by Telefonica across its footprint to combat the COVID-19 pandemic
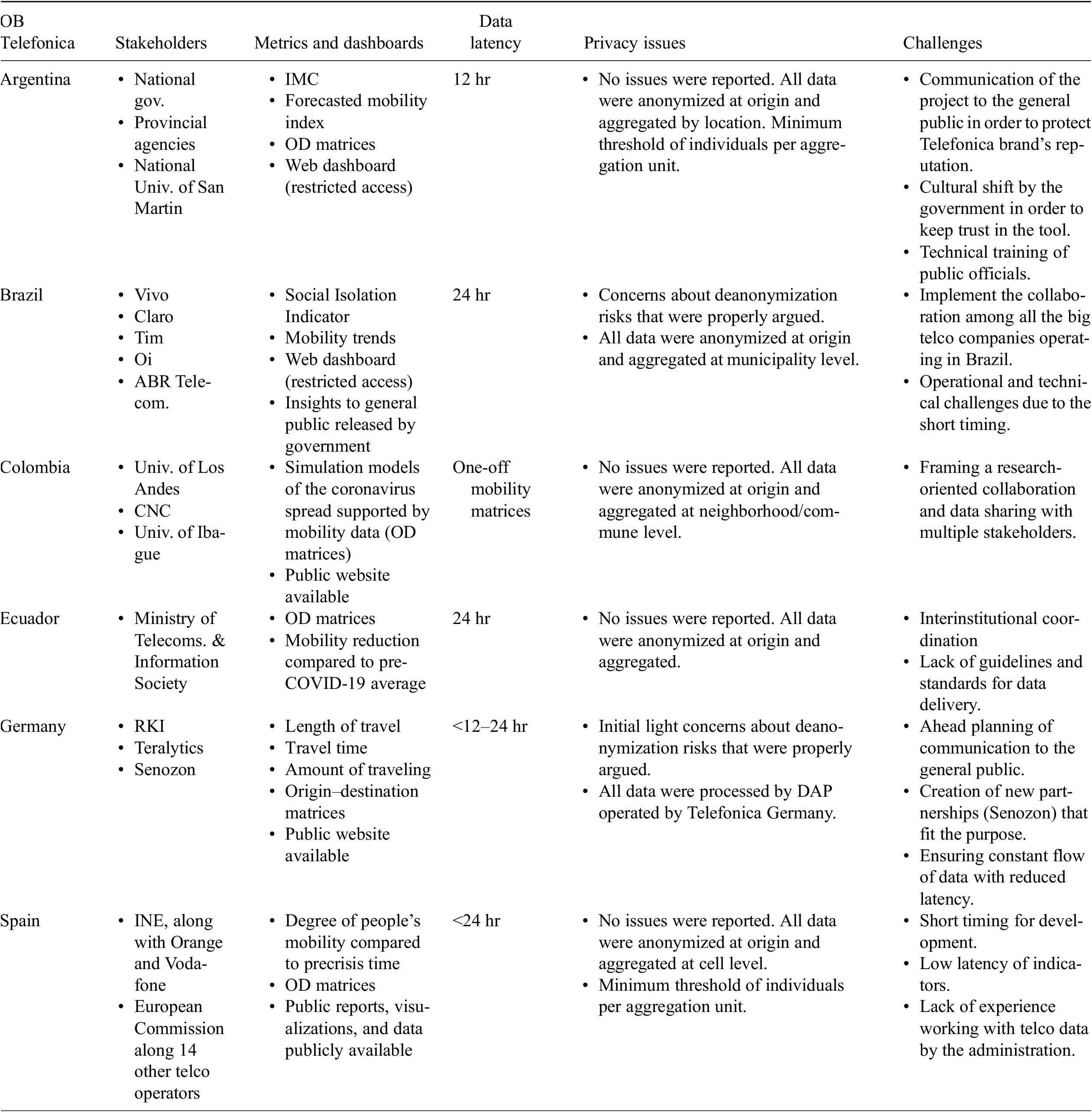
Abbreviations: CNC, National Consulting Center; DAP, data anonymization platform; IMC, Citizen Mobility Index; INE, National Statistical Office; OB, operating business; RKI, Robert Koch Institute.
We relate the success of these initiatives to several shared factors.
-
• Speed. Telefonica has been a pioneer in the digitization of its networks and in the business exploitation of Big Data and AI. In all cases, the operators had been investing steadily in these technologies for several years, which facilitated a quick response due to the synergies with existing technical and human capacities. Perhaps the biggest technological challenge was the shortening to one day, or even less, of the data-generation latencies.
-
• Privacy by design. Safeguarding data privacy is a fundamental requirement with which Telefonica is very familiar. The teams were especially careful that the information that was shared outside the company was secure, respecting the privacy of users and current legislation. We also believe that the fact of working in a regulated sector has given special credibility and confidence to the population regarding the management of private data.
-
• Commitment of Telefonica and the telco industry with the society. The telco industry and Telefonica have a strong commitment to the societies where they operate; for this reason, when the crisis broke out, there was no hesitation in making a proactive and philanthropic offer to the authorities, for the sake of a rapid response to the crisis.
We also have identified areas to work on and improve toward maximizing the impact.
-
• Potential of telco data. To date, restrictions on mobility are one of the main levers for governments to contain the epidemic. Undoubtedly, we have found that population mobility insights have been the element most in demand as the most effective way to monitor lockdowns. However, there are other use cases for which telco data can be very valuable and have not been exploited, for example, the prediction of areas of expansion of the virus, the appearance of outbreaks, or the monitoring of economic recovery (according to mobility trends). Some institutions (mostly from the academia) have shown interest in carrying out this exploration, but from a research perspective.
-
• Public–private partnerships. Collaboration between the public and private sectors on data is especially complex and, in each country, followed a different approach. Interestingly, in all cases, the end users were government stakeholders, but each country articulated the delivery of data with completely different partnership structures. Once the tools were delivered, from Telefonica, we would have liked to have had more feedback from the public sector on how they have been used in decision-making during the pandemic as well as their impact. In any case, we believe that the collaboration has increased the awareness of public stakeholders about the potentiality of telco data. New horizons have been opened for the standardization of this data source by national statistical offices, ministries, and other public bodies.
-
• Business model sustainability. Due to the urgency and unforeseen crisis context, all efforts have been made in a philanthropic way. Without a financial sustainability plan (that addresses the costs and investments required), it would be difficult to sustain these services in the medium and long term. It is essential that governments and companies codesign fair business models that guarantee the immediate and sustained availability of these tools in anticipation of future crises or prolongation of the current one.
Funding Statement
None.
Competing Interests
All authors are employees of Telefonica, and their contributions are provided from that perspective. All authors declare no competing interests in relation to the submitted article.
Author Contributions
Conceptualization, P.A.d.A.; Writing-original draft, P.A.d.A., E.F.M., A.S., D.G.K., W.R., M.C.D., C.C., J.J.M., J.M.P., M.S., T.T., E.D., and C.d.C.; Methodology, A.S., D.G.K., M.C.D., J.J.M., M.S., and E.D.
Data Availability Statement
The data and insights mentioned in this article are partially available in accordance with the decision of the national and local governments. Please refer to the following official websites for further information: Argentina (https://www.pulsocovid.com); Brazil (https://analytics.fiquebemfiqueemcasa.com.br/bigdata/home); Colombia (https://siscovid.com); Germany (https://www.covid-19-mobility.org); and Spain (https://www.ine.es/covid/covid_movilidad.htm).
Acknowledgment
None.
Comments
No Comments have been published for this article.