Rare diseases (RDs) are conditions affecting fewer than 1 in 2,000 people in the European Union, or less than 200,000 people in the United States (Reference Knoble, Nayroles, Cheng and Arnould1). RDs are often severe; few have curative therapies, whereby most treatments aim to alleviate symptoms, enhance quality of life or delay the health status deterioration, with the ultimate goal of controlling or modifying the disease trajectory (2). Thus, patient-reported outcome measures (PROMs) are increasingly adopted in health technology assessment (HTA) to estimate the benefits of treatments in terms of quality of life (1;3), especially when their responses can be converted into health state utility values (HSUVs). HSUVs represent individual preferences for a given health state measured on a scale from zero (“death”) to one (“full health”), which, if combined with time spent in that state, generate quality-adjusted life years (QALYs) (Reference Arnold, Girling, Stevens and Lilford4). QALYs are then incorporated into cost-utility models that inform reimbursement decisions in many HTA systems. Two groups of (direct and indirect) techniques exist to estimate HSUVs (Reference Arnold, Girling, Stevens and Lilford4), the latter using a particular type of PROMs called multi-attribute utility instruments (MAUIs). Their application to RDs may be challenging because of their low incidence and high heterogeneity. This paper discusses the pros and cons of each technique in relation to RDs (Table 1).
Table 1. Overview of the available techniques and implications for RDs
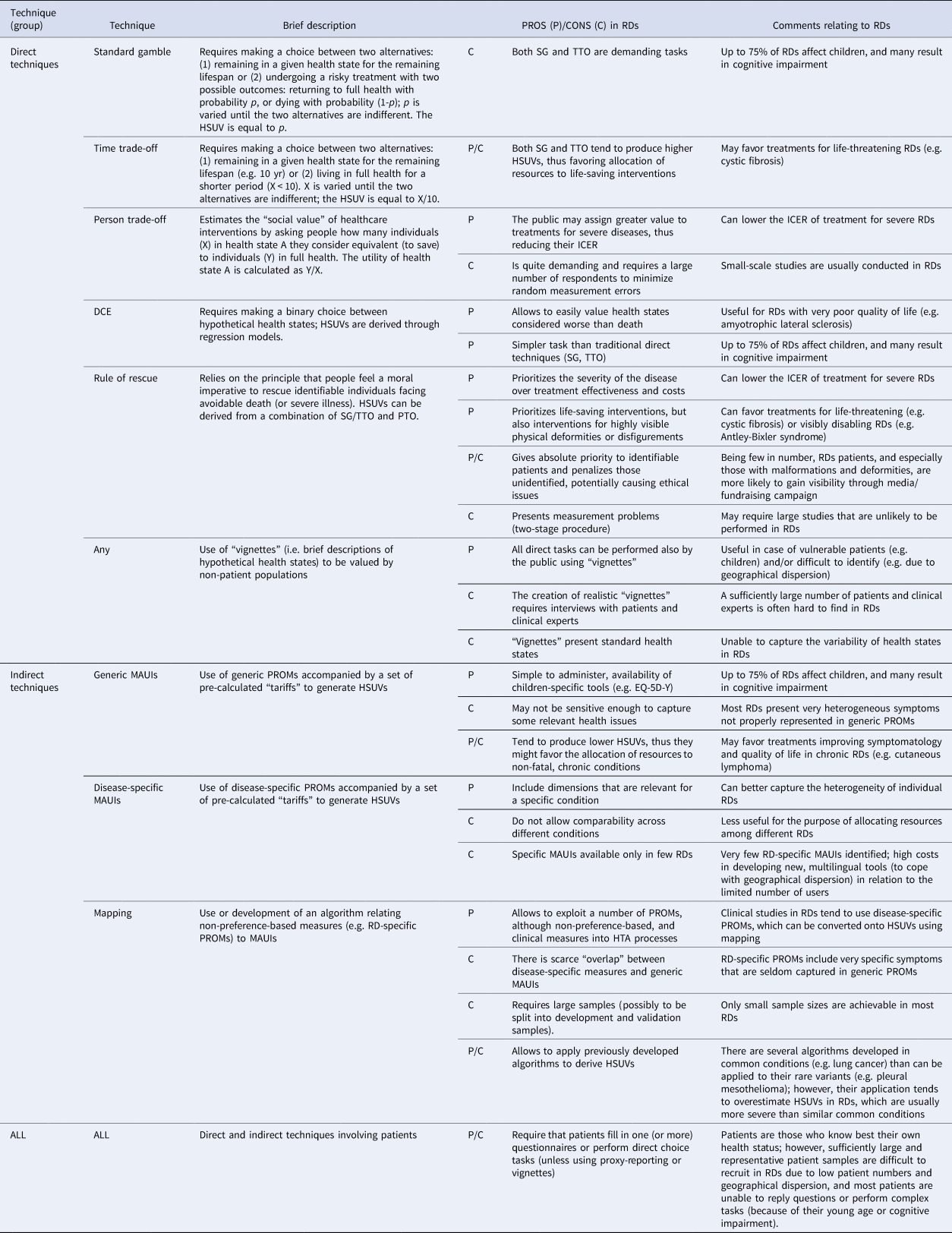
DCE, discrete choice experiment; EQ-5D-Y, EuroQol Five-Dimensional Questionnaire, Youth Version; HSUV, health state utility values; HTA, Health Technology Assessment; ICER, incremental cost-effectiveness ratio; MAUI, multi-attribute utility instrument; PROM, patient-reported outcome measure; PTO, person trade-off; RD, rare disease; SG, standard gamble; TTO, time trade-off.
Direct Techniques
The most common techniques for measuring HSUVs directly include standard gamble (SG) and time trade-off (TTO). SG involves trading a sub-optimal health state A with the risk of immediate death (1-p), and the HSUV is represented by p (i.e. the probability of returning to “full health”). TTO trades duration of life against quality of life, and the HSUV is the ratio between time in full health (X) and time (e.g. 10 yr) in state A (Reference Arnold, Girling, Stevens and Lilford4). The person trade-off (PTO) is similar to TTO but focuses on persons instead of time as the trade-off unit. It requires indicating how many patients (X) in state A and (Y) in “full health,” respectively, are considered equal to saving one life year (Reference Nord5). The HSUV of A is calculated as Y/X (Reference McKie and Richardson6), which is a “social” value as opposed to the “individual” one obtained from SG/TTO (Reference Nord5). The “rule of rescue” relies on the moral imperative people feel to rescue identifiable individuals facing an imminent risk of avoidable death, irrespective of cost-effectiveness considerations (6;7). It can provide HSUVs but raises measurement challenges, since it requires a two-stage procedure combining SG or TTO (evaluating individual utility) and PTO (evaluating social utility), and ethical issues, since prioritizing interventions based on “identifiability” is not morally justifiable, and contradicts with the impersonal logic underlying cost-utility analysis (Reference McKie and Richardson6). Lastly, discrete choice experiments (DCEs) ask respondents to choose between hypothetical health states and derive HSUVs through regression techniques (Reference Bansback, Brazier, Tsuchiya and Anis8).
The measurement of HSUVs using direct techniques is conducted either with patients (or caregivers as proxy respondents), who value their own health state, or members of the public, who value hypothetical health states represented in “vignettes.” The use of “vignettes” is advantageous in RDs, since they can be designed to incorporate relevant health issues and limit the use of patient-level data. The risk is that the health states presented may not fully capture the experience of individual cases because of an extremely varied symptomatology. Moreover, the creation of realistic vignettes requires an extensive qualitative work (e.g. in-depth interviews and focus groups) involving patients that may be difficult to identify and recruit, and a sufficient amount of clinical expertise and literature that may be lacking in RDs. Lastly, there may be differences among valuation methods leading to inconsistent HSUV results (1;9–11).
SG and TTO may be challenging or unfeasible to administer in several RDs that affect children (around 75 percent) or are associated with cognitive and communication impairments, unless parent or caregiver proxy reporting is used (3;11). The PTO is usually performed by the public, who may assign a greater value to treatments for people with serious conditions, including RDs (Reference Silva and Sousa7). However, the task requires large samples of participants to minimize measurement errors (Reference Nord5), while small-scale studies are usually conducted in RDs.
In the recent literature (7;12), the “rule of rescue” approach has been encouraged to value health states in RDs. This approach, by giving priority to identifiable people may favor RDs since patients are few in number, often children, or presenting visible deformities or disfigurements. Social media also play a role in increasing their recognizability and visibility in society compared to common conditions. Moreover, the estimated budget impact of rescue treatments for RD patients is perceived as negligible by society. In RDs, the “rule of rescue” has been discussed in relation to severe traumatic brain injury, where the decision to perform decompressive craniotomy is often taken irrespective of the patient's subsequent quality of life, procedural costs, or trade-offs in using these resources to improve health in the wider community (Reference Honeybul, Gillett, Ho and Lind13).
Lastly, DCEs may be promising in RDs, especially in those associated with very poor quality of life (e.g. amyotrophic lateral sclerosis, ALS), since health states can be valued as “worse than death” without altering the task, as is required with lead-time TTO. Moreover, DCEs are cognitively simpler than traditional direct techniques, since they require expressing a preference between state A and state B, without trading against risk of death or duration of life (Reference Bansback, Brazier, Tsuchiya and Anis8); thus, they are less affected by measurement errors when administered to vulnerable RD patients.
Indirect Techniques (MAUIs)
HSUVs can be estimated indirectly by using MAUIs, which are PROMs based on individual preferences, typically obtained in country-level surveys where members of the public value a sample of health states by using direct techniques or DCEs (8;14), and subsequently aggregated as mean scores. Therefore, MAUIs are provided with a value set of “tariffs” for every combination of the instruments' domains/levels. The most popular generic MAUIs are the EuroQol 5-dimension (EQ-5D), the Health Utility Index (HUI), and the Short Form 6 Dimension (SF-6D) (Reference Brazier, Ara, Rowen and Chevrou-Severac14). Disease-specific MAUIs also exist, which are useful to provide HSUVs in conditions where generic ones are not appropriate, sensitive or responsive, or to compare HSUVs across different studies on a specific condition. However, these tools do not allow cross-disease comparisons and their role in HTA is often limited to providing additional supporting evidence of treatment benefits (beyond the cost-utility model) (Reference Rowen, Brazier, Ara and Azzabi Zouraq15). In studies where MAUIs have not been used, “mapping” is an accepted alternative to generate HSUVs through the development and use of a model or algorithm that uses data from other measures of health outcomes (Reference Longworth and Rowen16), such as non-preference-based PROMs.
Indirect methods avoid asking patients the complex task of trading health states with different risks of death (SG) or years of remaining life (TTO). Such trade-offs need to be done only once by involving the public in the valuation exercise. The resulting “tariffs” are used to derive HSUVs by administering the corresponding MAUIs to patients. However, the difficulties encountered in the collection of PROMs in RDs also apply to MAUIs (Reference Slade, Isa, Kyte, Pankhurst, Kerecuk and Ferguson3). First, the low prevalence of each RD results in small and heterogeneous samples affecting data collection and statistical analyses (1;3). Second, even though MAUIs are much easier to respond to compared to SG/TTO, they remain challenging for children and may need to rely on parent proxy reporting. Some simplified self-reported MAUIs, such as the EQ-5D-Y (Youth), are available for children and may facilitate the estimation of HSUVs in pediatric RDs. Third, the administration of MAUIs to RD patients may be challenging due to their geographical dispersion, which generally requires multi-site studies with related logistic and financial issues.
Fourth, generic MAUIs may not be sensitive enough to capture relevant health issues in RDs, particularly in the more heterogeneous conditions (Reference Knoble, Nayroles, Cheng and Arnould1). In a recent survey, most RD patients reported that EQ-5D-5L did not capture important issues affecting their daily life, such as fatigue, relationship/social life, and co-morbidities (Reference Efthymiadou, Mossman and Kanavos17). A systematic review of HSUVs in Duchenne Muscular Dystrophy (DMD) identified the use of EQ-5D or HUI3 in all studies deriving HSUVs, but that they did not capture relevant quality of life dimensions such as hope, fear, fatigue, social participation, and dignity (Reference Szabo, Audhya, Malone, Feeny and Gooch18). However, the level of sensitivity of MAUIs may vary according to the specific instrument adopted and individual RDs. For example, HUI3 compared to EQ-5D has a greater coverage of domains relevant for DMD patients such as ambulation and dexterity (Reference Szabo, Audhya, Malone, Feeny and Gooch18).
Using RD-specific MAUIs helps overcome the issue of poor sensitivity with generic ones, but only few instruments are available (e.g. ALS Utility Index, Short Bowel Syndrome-Quality of Life scale (Reference Rowen, Brazier, Ara and Azzabi Zouraq15)), and the rarity of each condition can make the cost of new instruments development unsustainable (Reference Slade, Isa, Kyte, Pankhurst, Kerecuk and Ferguson3). Lastly, “mapping” allows to exploit disease-specific, non-preference-based PROMs, which are preferred in clinical studies on RDs (Reference Pearson, Rothwell, Olaye and Knight11), but presents several pitfalls in RDs, such as lack of sufficiently large samples to develop and test algorithms, limited “overlap” between RD-specific and generic PROMs, or poor applicability of algorithms developed in similar non-RDs (Reference Meregaglia, Whittal, Nicod and Drummond19).
Implications for HTA
The impact of using different techniques to estimate HSUVs for HTA was assessed in a wide range of conditions, including some RDs (Reference Arnold, Girling, Stevens and Lilford4). Overall, direct methods tend to produce consistently higher HSUVs than indirect methods. In ALS, the HSUVs derived from SG were significantly higher than those from EQ-5D for all severity levels (Reference Green, Kiebert, Murphy, Mitchell, O'Brien and Burrell20). Similarly, in systemic sclerosis, the agreement between SF-6D and TTO/SG was poor, with SF-6D providing lower values than direct techniques (Reference Khanna, Furst, Kee Wong, Tsevat, Clements and Park21). In esophageal cancer, TTO values were higher or lower than EQ-5D depending on tumor stage (Reference Wildi, Cox, Clark, Turner, Hawes and Hoffman22). Since the utility of death is fixed at zero (Reference Arnold, Girling, Stevens and Lilford4), using direct techniques in RDs might favor new treatments for life-threatening diseases, including those with onset in early childhood (30 percent of children with RDs do survive to age 5 (Reference Slade, Isa, Kyte, Pankhurst, Kerecuk and Ferguson3)), rare infectious diseases (e.g. tuberculosis), or rare cancers (e.g. pleural mesothelioma). Conversely, the use of MAUIs, giving more space for utility gain, may favor treatments targeting symptoms relief and quality of life improvement in chronic RDs (e.g. cutaneous lymphoma). Lastly, the “rule of rescue” approach and PTO may advantage treatments for RDs in general, if people assign greater value to health gains in rare and severe conditions (Reference Hughes, Tunnage and Yeo12).
Conclusions
The estimation of HSUVs is crucial in RDs, given the growing use of PROMs to record quality of life gains from new treatments. However, there is no agreement on the most appropriate technique, and each may present pros and cons for individual RDs. Overall, the rarity of each condition allows the identification of only a few representative patients which affects the precision of the aggregate HSUVs resulting from the administration of MAUIs, or the evaluation of the individuals' own health status in direct measurement tasks. In very heterogeneous RDs, different techniques can be used for patient subgroups to address their specific characteristics and increase the sample size (Reference Ara, Brazier and Young23). Moreover, there is a dearth of disease-specific MAUIs that could replace generic ones when these are not sensitive enough. The large number of RDs, the low prevalence for each, and patients' geographical dispersion discourages the investment of resources in developing new multilingual tools (Reference Slade, Isa, Kyte, Pankhurst, Kerecuk and Ferguson3), as well as performing ad hoc evaluation studies because of logistical issues and long timelines for recruitment and data collection. In most RDs affecting children (Reference Slade, Isa, Kyte, Pankhurst, Kerecuk and Ferguson3), the use of children-specific MAUIs is encouraged. For its simplicity, the visual analogue scale (VAS) may be a further option, although it is a choice-less task and therefore less preferred than other direct techniques (Reference Brazier and Rowen9). Moreover, studies should take a family perspective to incorporate the HSUVs of parents (11;24). The use of less conventional approaches such as “vignettes,” PTO, “rule of rescue,” and DCEs requires further evidence on their usefulness in RDs and acceptability in HTA, given that some agencies already have special processes for the assessment of treatments for RDs (e.g. higher cost-effectiveness thresholds, reflecting the value of treating severe illnesses where no other treatment exists) (11;25). Overall, the establishment of a set of recommendations is required to inform the estimation of HSUVs across different RDs, and to address the HTA implications of using alternative techniques.
Acknowledgments
This research was funded by the European Commission's Horizon 2020 research and innovation program and was undertaken under the auspices of IMPACT-HTA (Grant number 779312). The results presented here reflect the authors' views and not the views of the European Commission. The European Commission is not liable for any use of the information communicated. The authors are grateful to Dr Karen Facey and Dr Amanda Whittal for providing useful comments on this manuscript.