Early economic evaluation is used to assess the potential cost-effectiveness of new health technologies in the very early stages of development, often even before the technology exists, to guide the target product profile and development process, suggest clinical evidence requirements, and identify potential risks to cost-effectiveness (Reference Annemans, Genesté and Jolain1). Such analyses usually use decision analytic modeling, which combines evidence from multiple sources, making best use of an often-limited evidence base and facilitating comprehensive sensitivity analysis to characterize uncertainty.
Developers of new technology can use the results of early economic evaluation in three ways (Reference Annemans, Genesté and Jolain1). First, by halting the development of technologies that are highly unlikely to be cost-effective at an earlier stage developers can reduce total investment and financial risk. Second, developers can identify and improve the key features of their technology (e.g., test accuracy) that drive the likelihood of it being cost-effective in the wider health system. As well as potentially changing features of the technology itself, early modeling can influence developers’ decisions about price, patient population, and position in the care pathway. For example, a technology that was originally designed with younger, independent patients in mind may in fact be likely to be cost-effective only for patients in care homes with significant care needs. This may require the usability of the technology to be reconsidered.
Finally, by specifying the critical evidence gaps surrounding the technology, early modeling can guide future evidence generation, resolving these uncertainties and smoothing the path for rapid uptake of cost-effective technology by health systems. This is particularly critical in the United Kingdom for diagnostics and devices, where guidance on cost-effectiveness published by the National Institute of Health and Care Excellence (NICE) Diagnostics Assessment Programme is nonmandatory. Tests must, therefore, demonstrate cost-effectiveness sufficient to encourage uptake by individual providers and local commissioners (2). For these reasons, the value of early decision modeling of diagnostics is increasingly being recognized (Reference Buisman, Rutten-van Mölken, Postmus, Luime, Uyl-de Groot and Redekop3;Reference Smith, Sutton and Shinkins4).
Early modeling may hold particular value for tests aimed at chronic diseases managed in primary care. General practitioners’ (GP) decision-making requirements for these conditions are complex, and test developers often have very limited insight into the current care pathways (Reference Turner, Van den Bruel, Jones, Plüddemann, Heneghan and Thompson5). Early modeling could be used to provide early insight into the care pathway and potential impact of a new test before the risks of development have been shouldered.
One such area is exacerbation management in patients with chronic obstructive pulmonary disease (COPD). COPD is an irreversible, progressive respiratory condition characterized by breathlessness and cough (Reference Jones, Gruffydd-Jones, Pinnock, Peffers, Lawrence and Scullion6). Although continuous management is required, medical intervention is most intense during acute exacerbations. An exacerbation may be the result of a bacterial infection, in which case antibiotics are an appropriate treatment, or may be caused by eosinophilic inflammation or a virus, in which case corticosteroids are likely to be more effective (Reference Burge and Wedzicha7). NICE guidelines recommend a steroid in primary care patients who have an increased breathlessness (to target eosinophilic inflammation), and an antibiotic in patients who have more purulent sputum (in case of bacterial infection) (8). However, these signs and symptoms have low diagnostic accuracy (Reference Papi, Bellettato, Braccioni, Romagnoli, Casolari and Caramori9), and in practice, patients are frequently prescribed both treatments simultaneously, as a precaution. To enable rapid treatment, many patients have a self-rescue pack containing both of these medications available at home. Hospitalization is required for severe exacerbations, which can be fatal (8).
Being able to accurately distinguish between exacerbation causes may have several advantages over current practice. Primarily, by better targeting treatment at those who are most likely to benefit, treatment effectiveness may improve. Steroids and antibiotics have different physiological effects, and there is some evidence that targeting corticosteroid treatment on the basis of blood eosinophil levels may reduce the high observed rates of treatment failure (Reference Bafadhel, McKenna, Terry, Mistry, Pancholi and Venge10). This could lead to reduced exacerbation duration, hospitalizations, and mortality, and improved health-related quality of life (HRQoL) (Reference Waljee, Rogers, Lin, Singal, Stein and Marks11). Reducing unnecessary treatment would also reduce the burden of adverse events, including hyperglycemia, which is estimated to occur in 28 percent of patients prescribed steroids, and diarrhea, which occurs as a result of approximately 5 percent of antibiotic prescriptions (Reference Vollenweider, Jarrett, Steurer-Stey, Garcia-Aymerich and Puhan12;Reference Karner and Cates13). Overall, this clinical rationale suggests that treatment with a targeted monotherapy will result in better patient outcomes than combined therapy, although there is only weak evidence to support this at present.
Additionally, as many patients have a self-rescue pack, there is the potential for patients to be able to self-test and self-treat, reducing the need for consultations with their GP. Finally, testing could reduce inappropriate antibiotic prescribing, a major cause of antimicrobial resistance (AMR) (14).
One proposed tool being developed is a point-of-care test, Rightstart® (Mologic, Bedford, UK) that uses a panel of biomarkers to distinguish between eosinophilic and bacterial exacerbations, and is designed to be used by patients or their carers in the home. The Rightstart test would be used, in conjunction with advice from the patient's GP, to determine the optimal treatment at exacerbation onset.
The test will consist of disposable test cassettes and a compact opto-electronic reader capable of quantifying and interpreting the test results. The test cassette comprises a multiplex test strip allowing simultaneous measurement of up to five inflammatory biomarkers. The prototype blood test requires a fingerprick sample (similar to diabetic blood glucose testing) where the site of blood collection is cleaned with a topical germicide, and the skin pierced with a sterile lancet. After a droplet has formed, the blood is captured in a capillary tube (automatically filling by surface tension) and inserted into the test device. The assay process then starts, and the result is displayed in the reader window after 10 minutes. As the test is still in development, characteristics such as shelf life of reagents are still to be ascertained.
The primary aim of this economic evaluation was to develop an early decision model to evaluate the potential cost-effectiveness, in terms of costs per quality-adjusted life-years (QALYs), of testing using the Rightstart test, to guide treatment stratification for management of COPD exacerbations in the United Kingdom primary care setting compared with current practice from the perspective of the NHS. Secondary aims were to identify the likely determinants of cost-effectiveness to inform future research and development, and to quantify the potential impact on antibiotic prescribing, including assessing the effect of applying a published estimate of AMR costs to antibiotic prescribing (Reference Oppong, Smith, Little, Verheij, Butler and Goossens15).
Methods
To assess the existing evidence in COPD exacerbation point-of-care testing and guided treatment, we conducted a rapid review of the literature, searching Medline, Embase, and the Cochrane library using a search strategy developed to identify papers in the following areas: (i) Existing economic evaluations of COPD; (ii) Guidelines relating to the clinical care pathway in England; (iii) COPD epidemiology, including disease severity and progression; (iv) Costs and health state utility values for stable COPD exacerbations; (v) Existing tests for exacerbation treatment stratification, and studies of treatment effectiveness with and without stratification testing.
Commentaries, conference abstracts, non-English language papers, and papers published before 1996 were excluded. The results of the literature review were used to design the model and systematically identify model inputs.
A model concept was developed iteratively based on previous models and care pathway evidence identified in the rapid review, with input from the test developer (Mologic) and three GPs (G.H., C.C.B., and H.A.) who provided informal expert opinion through discussion. The model concept went through three iterations of development and review with the GPs and developer before being finalized, to ensure face validity.
Model Structure
The model was built using Excel 2013 (Microsoft, USA). To improve transparency and reproducibility, the base case model has been made available online (16).
Parameter values were taken from the literature, predominantly from Jordan et al. (Reference Jordan, Majothi, Heneghan, Blissett, Riley and Sitch17); full parameter values and sources are presented in Table 1. Conflicting or unavailable evidence was supplemented with expert opinion from the GPs, which was elicited informally through discussion.
Table 1. Parameter Values and Sources
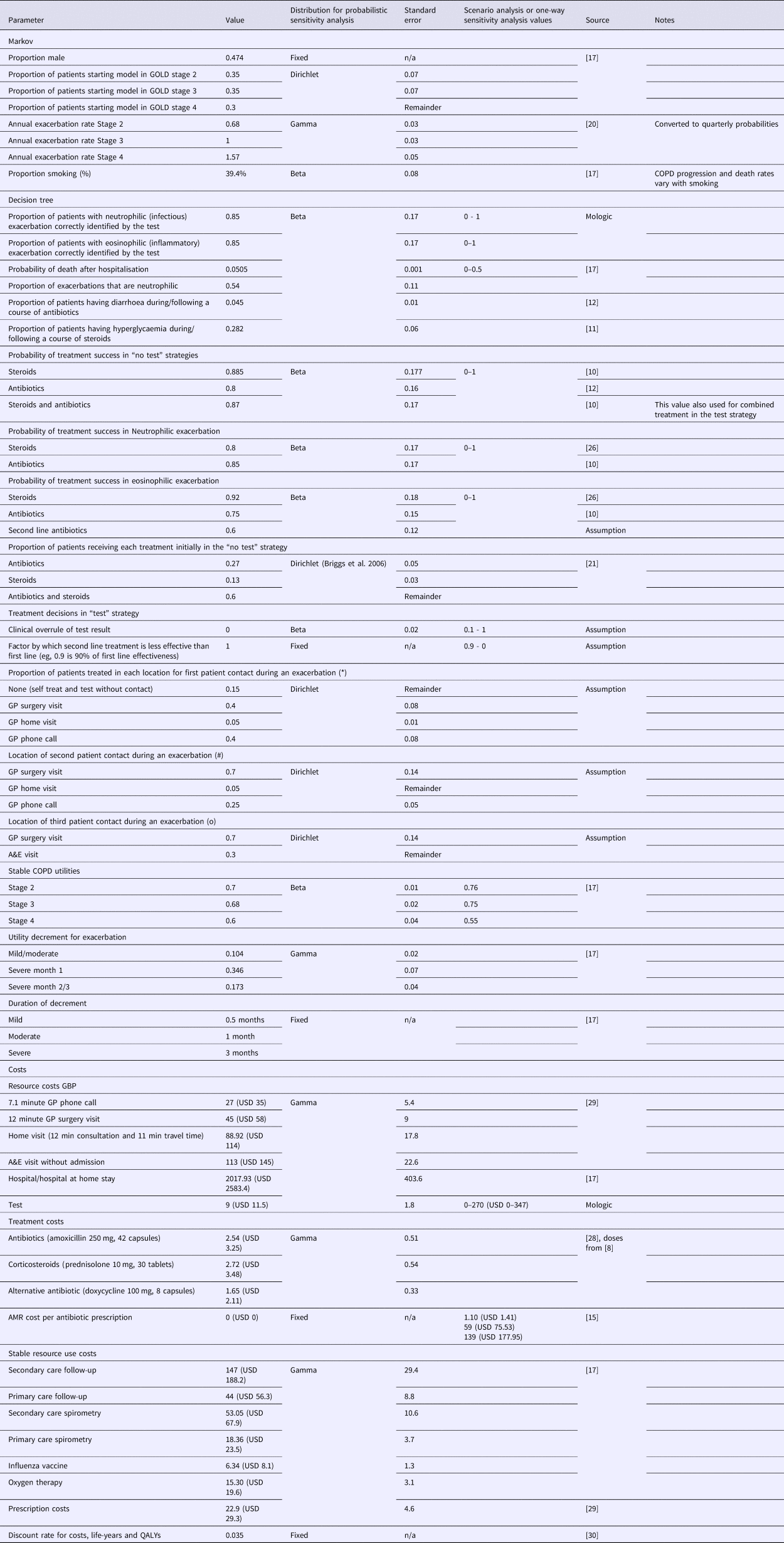
Note: *, #, and o symbols indicate the location of first, second, and third patient contact during an exacerbation as demonstrated in the decision tree model in Supplementary Figure 1. GBP is converted to USD at 1:1.28 rate of 29 October 2018.
The starting population comprised patients aged 60 years with COPD at GOLD stages 2 to 4, who have experienced exacerbations previously and have a self-rescue pack available at home. GOLD stages are internationally recognized measures of COPD severity, ranging from stages 1 (least severe) to 4 (most severe) (Reference Rabe, Hurd, Anzueto, Barnes, Buist and Calverley18). Stage 2 is the least severe stage where patients’ symptoms are likely to warrant prescription of self-rescue packs (Reference Antonelli-Incalzi, Imperiale, Bellia, Catalano, Scichilone and Pistelli19). The self-rescue pack contains antibiotics and oral corticosteroids (see Supplementary Table 1 for assumed dose). The self-rescue packs of patients in the test strategy also contain the single-use Rightstart test strips, with the test device made available at home. A patient having an exacerbation will take the test and then treat with the monotherapy indicated by the test result. They may entirely self-manage, or self-treat after consulting their GP by means of telephone, in person, or during a home visit.
The model combines a decision tree of exacerbation testing, treatment and short-term outcomes (Supplementary Figure 1), with a Markov model of lifetime COPD disease progression (Supplementary Figure 2), adapted from a model of exacerbation management (Reference Jordan, Majothi, Heneghan, Blissett, Riley and Sitch17).
The decision tree modeled exacerbation testing and treatment decisions and short-term outcomes. Patients in the no test strategy were classified by treatment choice (steroids, antibiotics, both), taken from a national study of prescribing choices in primary care (Reference Boggon, Hubbard, Smeeth, Gulliford, Cassell and Eaton20). Using the clinical evidence directly, rather than artificially simulating patient groups with different exacerbation etiologies, was used to minimize the number of assumptions needed, given the absence of evidence available on how many patients are treated correctly or incorrectly. This also has the consequence that the clinical effectiveness benefits of monotherapy compared with combined therapy are small, and there are several patient characteristics where treating with monotherapy is less effective than combined, for example, in treating neutrophilic exacerbations (Table 1).
If the first-line treatment was not effective, patients were assumed to be treated with the alternative (steroids if the first treatment was antibiotics, and vice versa). Further treatment failure, or failure following dual therapy, was treated with alternative antibiotics. If this treatment failed the patient was hospitalized, after which they could either die, fully recover, or partially recover, in which case they moved to a stable state at the next most severe GOLD stage (Supplementary Figure 1).
By contrast, patients in the test strategy were simulated according to exacerbation etiology, and could be treated correctly or incorrectly accordingly; the probability of correct treatment was determined by the test diagnostic accuracy. These simulated patient groups were derived from the evidence used in the no-test strategy, with assumptions around underlying etiology applied. Treating a patient with the correct monotherapy was assumed to have a higher probability of the treatment being effective than treating them inappropriately (Reference Bafadhel, Davies, Calverley, Aaron, Brightling and Pavord21). The outcomes following treatment success or failure were the same as for patients in the no-test strategy. In sensitivity analyses, we also allowed for the possibility that the patient or their GP could ignore the test result and use dual therapy instead (clinical overrule).
Patients who recovered after first or second treatments were classified as having a mild exacerbation. These patients were assumed to always fully recover. Patients who required more treatment were classified as having a moderate exacerbation, while hospitalization defined a severe exacerbation. This classification was used as current exacerbation severity definitions are predominantly based on resource use and duration (Reference Jordan, Majothi, Heneghan, Blissett, Riley and Sitch17;Reference Jones and Higenbottam22). We assumed that patients with moderate or severe exacerbations could either recover fully (i.e., return to their original GOLD stage at the end of the exacerbation) or partially (i.e., progress to the next GOLD stage), while only patients with a severe exacerbation could die as a result. Exacerbation severity was assumed to be independent of the patient's GOLD stage.
The Markov model included eight health states to capture the progression of COPD over time. They included: three stable COPD states (defined as GOLD stage 2, 3, and 4), each linked to an exacerbation state and two dead states (exacerbation death and all-cause mortality). The model used a 3-month cycle to approximately match the maximum length of exacerbation development and recovery (Reference Calverley, Anderson, Celli, Ferguson, Jenkins and Jones23). We assumed only one exacerbation was possible per cycle. The probability of developing an exacerbation varies with GOLD stage (Reference Antonelli-Incalzi, Imperiale, Bellia, Catalano, Scichilone and Pistelli19). At the end of an exacerbation, patients either recover or die of the exacerbation. If they recovered they could return to their current stage stable state (full recovery), or to the next stage up (partial recovery). The time horizon was 40 years, to account for events over the whole life course. Exacerbation outcomes were determined by the decision tree.
Utilities
Each GOLD stage was assigned a utility value from reported EQ-5D-5L scores (Reference Jordan, Majothi, Heneghan, Blissett, Riley and Sitch17) valued using the U.K. crosswalk time trade-off tariff (Reference van Hout, Janssen, Feng, Kohlmann, Busschbach and Golicki24). The impact of exacerbation was accounted for with a utility decrement which varied according to the severity and duration of the exacerbation, which lowered the HRQoL during the exacerbation cycle.
The state utility for all exacerbating patients for a particular GOLD stage in each cycle is the stable GOLD stage utility minus the expected value of the decrement for an exacerbation given the proportion of patients with each exacerbation type, which is determined in the decision tree.
Because exacerbations can develop rapidly, we assumed that patients immediately reached their maximum decrement, so a patient with a severe exacerbation has a severe utility value from the start of the exacerbation.
Transition Probabilities
Proportions of GOLD stages across the primary care patient population, exacerbation rates and mortality associated with each GOLD stage, treatment decisions for patients in the absence of the test, type and frequency of adverse events, and outcomes (i.e., death, fully recover, partially recover) from hospitalization were identified from the literature (Table 1). Disease progression without an exacerbation used the same methods as reported by Jordan and colleagues (Reference Jordan, Majothi, Heneghan, Blissett, Riley and Sitch17), taking a weighted average of progression rates for smokers and nonsmokers, according to the proportion of smokers in the target population, and allowing for variations in progression rates with age (Reference Atsou, Chouaid and Hejblum25).
The rapid review identified only one study that measured treatment outcomes following testing, which investigated steroid use following a different eosinophil test in a secondary care population (Reference Bafadhel, McKenna, Terry, Mistry, Pancholi and Venge10). As a result, plausible values for treatment outcomes were adapted from this source and from Cochrane reviews on treatment efficacy in untested patients (Reference Walters, Tan, White, Gibson, Wood-Baker and Walters26). An initial estimate of diagnostic accuracy was provided by the manufacturer. As the test is still in development, actual diagnostic accuracy was unavailable.
Resource Use
The model took an NHS health system perspective and, therefore, included only health system costs. An initial estimate for the cost of the test (GBP 9 (USD 11.5) per test strip, with the device supplied free of charge) was provided by the manufacturer. Resource use was taken from a previously published model (Reference Jordan, Majothi, Heneghan, Blissett, Riley and Sitch17) and expert opinion. Costs were obtained from the previous model (Reference Jordan, Majothi, Heneghan, Blissett, Riley and Sitch17), NHS Reference Costs (27), the British National Formulary (28), and PSSRU Unit Costs (Reference Curtis29), and where necessary were inflated to 2015 values using the Hospital and Community Health Services inflation index. Adverse events (hyperglycemia from steroids and diarrhea from antibiotics) were included as the cost of one additional appointment for treatment of the adverse event. Routine pharmacotherapy calculations are reported in Supplementary Table 1. Costs and QALYs were discounted at 3.5 percent (30).
Model Assumptions
The base case model contained several assumptions. Assumptions that were relaxed in the sensitivity analysis are marked (*): (i) The probability of a treatment being effective is fixed, and does not change based on whether treatment is first-line or not.* (ii) The test result was always used to determine treatment and was never ignored or overruled.* (iii) AMR was not accounted for as no estimates of the health care cost or QALY impact were available.* (iv) Due to the nature of a Markov model, the probability of progressing to a more severe GOLD stage was based on the current exacerbation and current GOLD stage, not any previous exacerbation or stage history in preceding cycles. (v) Patients could progress up a GOLD stage, but not down, to reflect that COPD is a progressive, irreversible condition. (vi) Patients could only progress up one GOLD stage per cycle. (vii) Patient movement through the Markov model occurred at the end of the cycle, with no half-cycle correction.* (viii) Exacerbation management and outcomes are independent of GOLD stage and patient characteristics. (ix) Only patients who were admitted to hospital could die of their exacerbation. (x) The costs and utilities of exacerbation states in the Markov model were calculated as expected values from the decision tree based on the proportion of mild, moderate, and severe exacerbations. (xi) The sensitivity and specificity of the test were assumed to be independent.
Sensitivity Analyses
Probabilistic sensitivity analysis (PSA) was carried out over 1,000 iterations; distributions for parameters are described in Table 1. A cost-effectiveness acceptability curve (CEAC) was drawn to demonstrate the probability of the new test being cost effective at a willingness-to-pay per QALY threshold between GBP 0 (USD 0) and GBP 50,000 (USD 64,000).
One-way and two-way sensitivity analyses were carried out to examine the determinants of cost-effectiveness. The values used in one-way sensitivity analyses are reported in Table 1. Several scenario analyses were also run to evaluate structural uncertainty. These included modeling the external costs of AMR to future patients by applying an additional cost to each antibiotic prescription, according to published estimated values (Reference Oppong, Smith, Little, Verheij, Butler and Goossens15). The relationship between exacerbation outcome and disease progression was also eliminated by setting the probability of full recovery to 1. The assumption that the effectiveness of antibiotics and steroids is constant, regardless of whether the treatment is first or second line, was relaxed by reducing the effectiveness of second-line treatments by a percentage of first-line effectiveness, from 90 percent down to 0 percent. Finally, the effect of testing itself was examined by varying the treatments given to the no test group initially.
Results
In the base case analysis, the test strategy dominated usual care (Table 2). The cost-effectiveness plane (Supplementary Figure 3) shows that the range of incremental cost-QALY pairs occupies a narrow North–West to South–East axis, reflecting the strong correlation between worsening health outcomes and increasing costs. This is due to exacerbation severity being partially defined by resource use. The CEAC (Supplementary Figure 3) shows that the probability of testing being cost-effective was above 50 percent at all thresholds above zero, although it never exceeded 70 percent.
Table 2. Results of Base Case Model and Deterministic Sensitivity Analysis
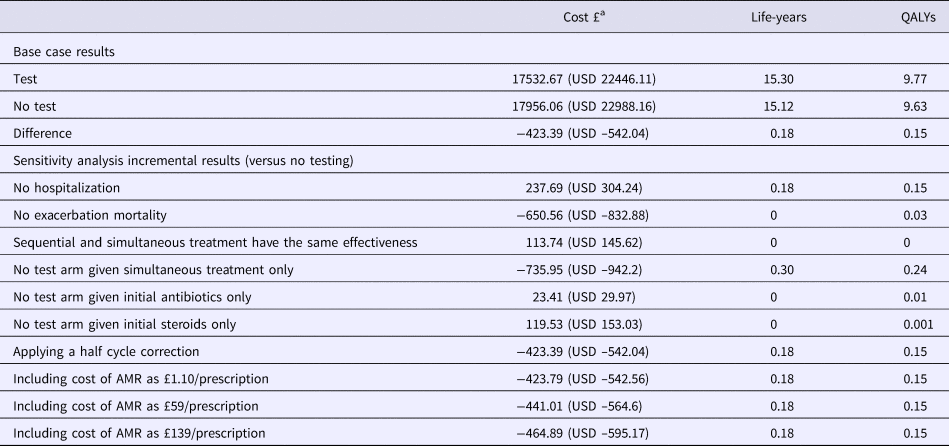
QALY, quality-adjusted life year; AMR, antimicrobial resistance.
a GBP is converted to USD at 1:1.28 rate of 29 October 2018.
One-way sensitivity analysis suggested that testing would be cost-effective at the NICE threshold of GBP 20,000/QALY (USD 25,600/QALY) gained unless the test cost was greater than GBP 260 (USD 333). Other sensitivity analyses revealed that the results were not affected by varying diagnostic accuracy between 0.5 and 1. This results from the assumption that test-guided monotherapy is more clinically effective than dual therapy in the majority of cases, which is one of the underlying rationales for testing (the others being reducing costs and unnecessary antibiotic prescribing), although this assumption was applied conservatively. Similar incremental costs and effects were obtained when modeling scenarios in which all no-test patients received either only steroids or only antibiotics compared with usual care.
Cost-effectiveness was, therefore, primarily dependent on the effectiveness of treating each type of exacerbation (inflammatory or infectious) with each treatment (Table 2). If dual therapy, or indeed either monotherapy, results in better health outcomes than using test-guided treatment in the majority of cases, the test ceases to be effective, and, therefore, cost-effective. Diagnostic accuracy does not become important until the clinical utility of test-guided treatment itself has been determined.
Assuming clinical utility is established, whether the patient and clinician implement the test-guided treatment is the other key determinant of cost-effectiveness. In some cases, such as elderly patients with co-morbidities, the test result may be ignored in favor of dual therapy, as a precaution. When this happens testing becomes less cost-effective because the benefits of testing are lost while its costs are still incurred.
Similarly the benefits of testing are largest when hospitalization rates are affected. If treatment is assumed to have no effect on hospitalizations (or if the cost of hospitalizations is reduced to GBP 0 [USD 0]), while retaining the effect on mortality, the intervention is no longer cost saving and instead costs GBP 1626/QALY (USD 1,616/QALY). Conversely, assuming no effect on mortality increased the cost saving to GBP 642 (USD 822), while reducing the lifetime QALY gain from testing to 0.03.
Applying AMR costs to antibiotic prescribing increased the cost savings from testing slightly, to between GBP 424 (USD 543) and GBP 465 (USD 595) per person, depending on which estimates of the cost of drug resistance were used (European, American or Global) (Reference Oppong, Smith, Little, Verheij, Butler and Goossens15). Testing reduced the mean number of antibiotic prescriptions per exacerbation from 0.9 to 0.6.
Discussion
Our results suggest that, if appropriately targeted monotherapy with either antibiotics or oral steroids for exacerbations can be demonstrated to be as effective as antibiotics and oral steroids together (dual therapy), point-of-care testing has the potential to provide health gains and cost savings in this setting. The lack of evidence on the effectiveness of test-guided treatment makes it difficult to assess how feasible these gains are. However, the clinical effectiveness of monotherapy versus dual therapy used in the model are based on conservative assumptions, to avoid overstating the likely benefits. Overall, this analysis has demonstrated the potential value of testing, identified the mechanism by which that value could be achieved, and indicated the key additional evidence required.
This study applies early decision analytic modeling to a treatment stratification test for acute exacerbations of COPD managed in primary care. This model could be applied to alternative diagnostic technologies in this setting, and may be relevant to other health systems internationally, although this will be dependent on the care pathways being similar. To facilitate this, we have made the code for the base case model available (16).
There is likely to be regional variation in the care pathway that is not fully captured in the model. In particular, we focused on GP practice, but respiratory nurses also play a key role in patient care. To address this, we plan to assess care pathway variation using a national survey, and to refine and validate the model in light of this.
In a condition where antibiotic use is widespread and steroid treatments can confer harm through adverse events, we have shown that point-of-care testing could reduce treatment overuse. In particular, in the base case analysis testing reduced antibiotic prescribing by a third. To account for the effect of this on AMR, we applied estimates based on lost productivity, as health service-specific estimates are not available (Reference Oppong, Smith, Little, Verheij, Butler and Goossens15). This goes beyond the health system perspective used in this analysis, and may also underestimate total relevant costs (Reference Oppong, Smith, Little, Verheij, Butler and Goossens15). The value of avoiding AMR, to the COPD patient considered here, to future patients, and for the wider health system, has not been established (Reference Smith and Coast31), but developing more comprehensive estimates of these costs and health outcomes is essential to incorporate the long-term harms of AMR into economic evaluation.
The model was subject to several structural assumptions that may affect the results. First, the clinical definitions of an exacerbation were partly defined by resource use (for example, a severe exacerbation is partly defined by the patient being hospitalized), with the result that increased costs are strongly correlated with poorer outcomes (Reference Jones and Higenbottam22). Further research on the relationship between GOLD stage, exacerbation severity and quality of life would clarify this.
We also used GOLD stages as a proxy for quality of life assessment and as a measure of disease progression, as most clinical and all economic evidence we identified was based on this measure. Clinically, GOLD stages have now been superseded by GOLD grades (Reference Vogelmeier, Criner, Martinez, Anzueto, Barnes and Bourbeau32), which are designed to better reflect patient experiences. Further research is required on the costs, utilities and outcomes of patients at different GOLD grades.
Additionally, the sensitivity and specificity for identifying eosinophilic exacerbations were assumed to be independent in PSA, although in practice most tests have some correlation between the two (Reference Novielli, Cooper, Abrams and Sutton33).
Finally, the structure of the model may introduce bias in favor of monotherapy rather than dual therapy. Each monotherapy was assumed to have fixed treatment effectiveness, regardless of whether it was first or second line, which means that multiple sequential monotherapies have higher overall effectiveness than that of dual therapy. As we assumed no patients in the base case test strategy received dual therapy (perfect implementation), this biases the base case result in favor of testing (Reference Novielli, Cooper and Sutton34). It is notable that the original no-test strategy was dominated by strategies where all patients received initial monotherapy (either steroids or antibiotics). Testing was also not cost-effective compared with this monotherapy strategy (Table 1). This assumption was explored in sensitivity analysis by reducing the effectiveness of the second-line treatment and by varying implementation probability.
There is some limited evidence that sequential treatment may be more effective by improving first-line treatment effectiveness (Reference Bafadhel, McKenna, Terry, Mistry, Pancholi and Venge10), but we have only been able to speculate on the exact relationship, particularly pertaining to antibiotic treatment. Further research on the clinical outcomes of test-guided treatment is essential to determine test cost-effectiveness.
Using early modeling in this setting has provided useful insights to guide future technology development in COPD, but the evaluation is limited by lack of evidence on treatment effects. To some extent this is inevitable, as part of the role of early modeling is to guide future evidence generation. However, the typical research and development of a new diagnostic rarely generates evidence around the care pathway and longer term outcomes of testing, which were the main inputs driving cost-effectiveness. Diagnostic evaluation typically prioritizes analytical and clinical validity, rather than clinical utility (Reference Parkinson, McCormack, Keating, Gutman, Hamilton and Mansfield35), in part due to the resources required for database and registry studies or large long-term trials. Decision modeling offers a solution to some of these barriers, but is reliant on good clinical evidence on treatment effects. This raises the question of how to generate sufficient evidence to build comprehensive and valid models for the evaluation of diagnostics. In the case of this decision problem specifically, future work is necessary to evaluate and validate some of the assumptions on the care pathway and clinical effectiveness parameters.
In conclusion, this model has evaluated the potential cost-effectiveness of test-guided treatment for COPD patients managed in primary care who are experiencing an exacerbation. The test in question is still in development, but the model results apply to any test that stratifies patients at this point in their care. The key finding is that testing may be highly cost-effective by both improving health outcomes and reducing treatment overuse. However, it should be noted that the benefits of subsequent treatments (whether guided by the test or clinical judgement) are still unknown, and this is likely to be the ultimate determinant of cost-effectiveness.
Supplementary Material
The supplementary material for this article can be found at https://doi.org/10.1017/S0266462318003707
Supplementary Table 1: https://doi.org/10.1017/S0266462318003707
Supplementary Figure 1: https://doi.org/10.1017/S0266462318003707
Supplementary Figure 2: https://doi.org/10.1017/S0266462318003707
Supplementary Figure 3: https://doi.org/10.1017/S0266462318003707
Author ORCIDs
Lucy Abel, 0000-0001-5857-1269.
Acknowledgements
The authors acknowledge Gita Parekh and Paul Davis at Mologic for providing information on the design, intended use, and price of the test. Thanks also to Chris Brightling for providing advice on the secondary care pathway, and Raymond Oppong for providing assistance in the use of antimicrobial resistance cost estimates.
Funding/Support
This study was funded by the Innovate UK Small Business Research Initiative (SBRI) (reference: 63624-463199). This project was supported by the NIHR Community Health Care MedTech and In Vitro Diagnostic Co-operative and the NIHR Oxford Biomedical Research Centre. The views expressed are those of the authors and not necessarily those of the NHS, the NIHR, or the Department of Health.
Conflicts of Interest
Authors Abel, Yang and Turner report grants from Innovate UK and from National Institute of Health Research during the conduct of the study. Authors Dakin and Hayward report grants from Innovate UK during the conduct of the study. Authors Roberts, Ashdown, and Van den Bruel, have nothing to disclose.