Introduction
Older persons with both cognitive impairments and depressive symptoms are a vulnerable group at increased risk of functional dependence (Xiang and An, Reference Xiang and An2015), nursing home admission (Xiang and An, Reference Xiang and An2015), and adverse health outcomes (Mourao et al., Reference Mourao, Mansur, Malloy-Diniz, Castro Costa and Diniz2016). Reciprocal associations between depression and cognitive performance have consistently been shown over longer periods. That is, depression is associated with an increased risk of accelerated cognitive decline (Shin et al., Reference Shin2020; Byers and Yaffe, Reference Byers and Yaffe2011), and cognitive impairments are associated with a worse course of depression (Van den Kommer et al., Reference Van den Kommer, Comijs, Aartsen, Huisman, Deeg and Beekman2013). While most literature focused on long-term associations between depression and cognitive performance, depression and cognitive performance may also be associated in both directions from one day to the next. Getting more insight into the daily temporal associations between depression and cognitive performance may be valuable for the development of daily intervention strategies in order to maintain health, quality of life, functional outcomes and independence.
Day-to-day associations between depression and cognitive performance
Depressive symptoms (Rosmalen et al., Reference Rosmalen, Wenting, Roest, de Jonge and Bos2012) and cognitive performance (Nesselroade and Salthouse, Reference Nesselroade and Salthouse2004; Allaire and Marsiske, Reference Allaire and Marsiske2005) both show high day-to-day variability, and thus may be temporally associated with each other on a daily basis. Depression may induce next-day deficits in cognitive performance, for example through worse nutrition and poor physical activity during the day (Meeusen, Reference Meeusen2014), more alcohol consumption, or physiological alterations (e.g. decreased brain-derived neurotrophic factor-levels and inflammation [Kim et al., Reference Kim, Nunes, Oliveira, Young and Lafer2016]), while reduced cognitive performance may yield an emotional reaction resulting in next-day depressive symptoms.
Sleep
Sleep problems are common in late-life depression as well as cognitive impairments (Bao et al., Reference Bao2017; Hu et al., Reference Hu2017; Siddarth et al., Reference Siddarth2020), and sleep quality and quantity show high day-to-day variability (Knutson et al., Reference Knutson, Rathouz, Yan, Liu and Lauderdale2007; Dillon et al., Reference Dillon, Lichstein, Dautovich, Taylor, Riedel and Bush2015). It is therefore likely that sleep plays a role in the daily fluctuations of depression as well as cognitive performance.
It is well recognized that sleep plays a role in memory consolidation (Stickgold, Reference Stickgold2005; Diekelmann, Reference Diekelmann2014), and sleep deprivation has been found to be associated with reduced neurocognitive performance and alterations in brain activation (reviewed by Durmer and Dinges, Reference Durmer and Dinges2005), and reduced cerebral blood flow (Zhou et al., Reference Zhou2019). On the other hand, longer sleep duration has been associated with decreased physical activity levels (Stranges et al., Reference Stranges2008) and increased sleepiness (Horne, Reference Horne and Monk1991), which may in turn result in worse next-day cognitive performance. According to our knowledge, only two observational studies in real life specifically examined the impact of sleep on next-day cognitive performance. The first study found that both more and less than average sleep was associated with reduced next-day cognitive performance (Gamaldo et al., Reference Gamaldo, Allaire and Whitfield2010). The other study found that poor subjective (but not objective) sleep was associated with worse next-day cognitive performance in chronic pain patients, but better next-day cognitive performance in healthy persons (Curtis et al., Reference Curtis, Williams, McCoy and McCrae2018). The authors explained this latter finding by a state of hyperarousal due to sleep loss.
Depression may impact subsequent sleep problems through increased ruminative thinking (Takano and Tanno, Reference Takano and Tanno2011) and the associated autonomic arousal and emotional distress that associates with it (Harvey, Reference Harvey2002). A lack of sleep may result in next-day depressive symptoms by increased stress reactivity (Meerlo et al., Reference Meerlo, Sgoifo and Suchecki2008), or an increase in amygdala activity (Yoo et al., Reference Yoo, Gujar, Hu, Jolesz and Walker2007). Supportive of this, a bi-directional association between sleep and affect was found with a systematic review of daily diary studies: worse sleep predicted worse affect and vice versa (Konjarski et al., Reference Konjarski, Murray, Lee and Jackson2018). Longer sleep duration, on the other hand, may also result in next-day depressive symptoms, for example through increased inflammation (Dowd et al., Reference Dowd, Goldman and Weinstein2011), or decreased physical activity (Stranges et al., Reference Stranges2008).
The single-subject approach in order to take into account individual differences
Most previous studies on daily associations between depression, sleep and cognitive performance used multilevel analyses to evaluate daily associations within persons. As such, these studies still focused on group-averages and thus between-person associations. Such group-aggregated results can only be generalized to individual persons, under very strict conditions. That is, the average, the variance, the covariance and lagged covariance between variables should be exactly the same for all individuals, and no changes over time in these statistical characteristics should be present (assumption of ergodicity: Nesselroade, Reference Nesselroade, Collins and Horns1991; Hamaker et al., Reference Hamaker, Dolan and Molenaar2005; Molenaar and Campbell, Reference Molenaar and Campbell2009). It is very likely that the condition of ergodicity is not met for sleep, depression and cognitive performance. Consequently, findings from studies focusing on group-averages on these associations will not be applicable to individual persons. Single-subject studies analyze each individual separately, and are thus able to explore the temporal association of variables over time within an individual. Consequently, single-subject studies yield results that apply to the individual, which is essential in the presence of large inter-individual differences. Furthermore, they can account for the time-varying nature of sleep, depression and cognitive performance, by virtue of the multitude of repeated assessments.
Objective of the present study
The objective of the present study was to explore the temporal dynamic association of depressive symptoms, cognitive performance and sleep using a single-subject design in older persons with both depression and cognitive impairments. We hypothesized that the presence, nature (i.e. is it a positive or a negative association?), strength and direction (i.e. does X precede Y or does Y precede X?) of the associations differ between persons.
Methods
Design and participants
This study, the “idiographic study on cognition, affect and sleep in the elderly” (i-CASE) comprised an idiographic single-subject study design in older persons with depressive symptoms and cognitive impairments. Each participant completed 63 assessments of depression, sleep, and cognitive functioning using a diary and actigraphy. Following the idiographic single-subject design, time-series of each participant were analyzed separately.
Between January 2016 and January 2019, participants were recruited from the University Center Psychiatry and the Memory Clinic of the University Medical Center Groningen, and a mental health care institute in Groningen (Lentis). Patients were eligible if they 1) were aged ≥ 60 years, 2) had depressive symptoms defined as a major depressive disorder according to Diagnostic Statistical Manual (DSM) criteria or a 15-item Geriatric Depression Scale (GDS) score ≥ 4, 3) had cognitive impairment defined as a diagnosis of dementia or mild cognitive impairment (MCI) according to the multidisciplinary team of the Memory Clinic, a Mini Mental State Examination (MMSE) score < 25, or a Montreal Cognitive Assessment (MOCA) score < 26. Patients were excluded if they had a somatic disorder influencing short-term survival, had moderate to severe dementia according to a clinical dementia rating scale ≥ 2, had a bipolar, psychotic or substance use disorder during the past 2 years, were not able to participate due to for instance functional or language impairments, or if the treating specialist deemed the patient mentally incompetent to give consent for study participation.
The study protocol was approved by the institutional review board at the University Medical Center Groningen, and written informed consent was obtained from each study participant (METc 2013/019)
Procedures
At baseline, a trained research assistant administered several questionnaires and interviews, which are described in the Supplemental file. After this, the study period of 63 consecutive days started. During the 63-day study period, participants filled out an electronic diary on a study laptop every evening within 1 hour before going to bed. The diary included self-report questionnaires and a computerized cognitive task battery. Participants were instructed to fill-out the questions and perform the cognitive task in a quiet place with minimal distraction. Additionally, participants wore a MotionWatch8 (CamnTech) during the whole study period of 63 days in order to estimate sleep.
Variables in the time series analyses
Depressive symptoms, cognitive performance and sleep were the endogenous variables that were assessed on a daily basis and used in the time series analysis. Endogenous variables are variables that are both determinant and outcome at the same time in the time series analysis.
For depressive symptoms the 8-item Patient Health Questionnaire (PHQ-8, Kroenke et al., Reference Kroenke, Strine, Spitzer, Williams, Berry and Mokdad2009) was used. The PHQ-8 evaluates current presence and severity of eight out of the nine DSM-IV depressive symptoms (without suicidal ideation). For the present study, the item on sleep was also not included in order to reduce overlap with our measure for sleep. Each of the seven remaining items was rated on a 7-point Likert scale from (1 not present to 7 most severe). An average score was calculated by dividing the total score by the number of items, resulting in a possible score from 1 (not present) to 7 (most severe). For one participant (Participant 8), the PHQ-score was log-transformed to make it normally distributed.
Sleep was defined as the total time during the night-time sleep-period that was actually spent in sleep (total night-time sleep time [TST]) as derived from the MotionWatch8. TST was calculated by adding up each 30-second epoch during the night-time sleep-period that was defined as sleep.
Because working memory was previously found to co-vary with mood within persons (Riediger et al., Reference Riediger, Wrzus, Schmiedek, Wagner and Lindenberger2011; Brose et al., Reference Brose, Schmiedek, Lövdén and Lindenberger2012; Brose et al., Reference Brose, Lövdén and Schmiedek2014), we focused on working memory for the present analysis. Working memory was assessed every evening with the One Back Task (ONB) as part of the Cogstate Brief Battery (Hammers et al., Reference Hammers2012). For the present analyses, the reaction time for correct responses was the primary outcome of the ONB. These reaction times were transformed using a base 10 logarithmic transformation in order to make them normally distributed, and the inverse was taken so higher scores represent better scores.
Data preparation
A “time” variable was created, indicating the unit of the time series analysis (or assessment day, thus ranging from 1 till the number of the last day). Each unit in the time series consisted of sleep during the night and depression and cognitive performance during the following evening (see Figure 1). Missing values were imputed for each participant separately with the R-package Amelia. The number of missing values that were imputed for each person can be found in Supplemental Table 1. For some participants the length of the time series deviated from 64, because it was logistically more achievable to extend the study period or to stop earlier. We standardized the three endogenous variables for each person separately (so one unit in the variable was equivalent to one person-specific SD).

Figure 1. The timing of assessment of sleep, depression and cognition illustrated for 2 days within the 63-day study period1.
1Because the study period started with the evening assessment, ‘time’ = 1 consisted only of data for depression and cognitive performance, but not for sleep. The first sleep assessment was therefore represented at ‘time’ = 2. ‘Time’ = 64 therefore represented the last sleep assessment, and no data for depression and cognitive performance. As a result of this data organization, a contemporaneous association of sleep with depression and/or cognitive performance would indicate an effect of sleep last night on depression and/or cognitive performance the subsequent evening. A lagged effect of sleep on depression and cognitive performance would indicate an effect of sleep the night before last night on depression and cognitive performance in the evening. The missing value for sleep at ‘time = 1’, for depression and cognitive performance at ‘time’ = 64, and any other missing values were imputed for each participant separately with the R-package Amelia.
Power
For time-series analysis, power is derived from the number of repeated measures instead of the number of participants, as the analyses are done for each individual separately. It is difficult to make power analyses for time series analyses, because effect sizes as commonly used in conventional group-based study designs do not apply for the analyses of effects over time in single persons. This, because the direction of causality and the number of lagged influences in the system under investigation is usually unknown and bidirectional and feedback effects can be present as well (Brandt and Williams, Reference Brandt and Williams2007). Furthermore, the regression coefficients of a VAR cannot be interpreted individually, as they are part of a system of variables that are dynamically related to each other. As a result, “effect sizes” as commonly used in conventional study designs do not apply. Simulation studies have shown that time-series analysis can already be done with 30 repeated measures, although more observations yield more power and more reliable results (Lütkepohl, Reference Lütkepohl2005). Often, a minimum number of 50 is used (Box et al., Reference Box, Jenkins and Reinsel1994). Although there is some progress about power calculations for n-of-1 experimental designs (Percha et al., Reference Percha, Baskerville, Johnson, Dudley and Zimmerman2019), simulation studies must still be done to acquire more information about power analysis for n-of-1 observational studies using VAR in the current field.
Statistics
The temporal dynamic associations between sleep, depression and cognitive performance were evaluated using vector autoregressive modeling (VAR) analyses. VAR analysis separates time-lagged effects from contemporaneous effects. Therefore, the model allows to make inferences about the temporal order of effects. Bi-directional and feedback effects can also be investigated because variables can be treated as determinant and outcome at the same time (i.e. endogenous variables). In the present study, the VAR model consisted of a system of three endogenous variables representing our three primary outcomes for sleep, depression and cognitive performance. Each of the endogenous variables was regressed on its own lagged values and the lagged values of the other endogenous variables, as well as on the contemporaneous and lagged exogenous variables.
We chose to include one lag in the model, which is equivalent to one day. This, because we expected the values of sleep, depression and cognitive performance of the previous day to be most relevant for their current values for most persons. This resulted in the following equations:

The parameters α1, β1, and γ1 were estimated for depression. For sleep the parameters α2, β2 and γ2 were estimated, and for cognition the parameters α3, β3 and γ3 were estimated. The residuals (ε1 t , ε2 t and ε3 t ) should be serially uncorrelated but can be contemporaneously correlated. The correlation between the residuals can be interpreted as the contemporaneous effects between the endogenous variables and are denoted c1, c2 and c3 in the equations (Brandt and Williams, Reference Brandt and Williams2007). The abovementioned equations result in the following model that was estimated for each participant (Figure 2):

Figure 2. Estimated model for each participant1.
1The 3 arrows denoted with c1, c2 and c3 are the contemporaneous associations. Note that for the contemporaneous association of Depressiont with Cognitiont the direction of the association is unclear, because they are assessed right after each other. This association is therefore represented with a double-headed arrow. However, note that for the contemporaneous of Sleept with Depressiont and for Sleept with Cognitiont there may be a direction, because this is the association of sleep last night with depression and cognition in the evening. Therefore this association is represented with a one-headed arrow.
The vertical arrows denote the autoregressive effects α1, β2 and γ3.
The diagonal arrows denote the cross-lagged effects β1, γ1, α2, γ2, α3, and β3.
In the present study, the r-package AutoVAR (Emerencia et al., Reference Emerencia, van der Krieke, Bos, de Jonge, Petkov and Aiello2016; van der Krieke et al., Reference Van der Krieke2015) was used to perform the analyses. See Supplemental file for a description of AutoVAR.
Because some studies suggest that both more and less sleep may have negative effects (Gamaldo et al., Reference Gamaldo, Allaire and Whitfield2010), we repeated the analyses using a variable for TST that was transformed in such a way that 1 unit increase represented a deviation (up or down) of 1 standard deviation (SD) from the person-specific average TST. In these analyses, the transformed TST variable was included as exogenous variable only (i.e. as an independent, but not as a dependent variable). This, because this variable was highly skewed and could not be normalized through transformation. Two exogenous variables for transformed TST were included in these models: at time = t and at time = t–1.
The coefficients of the VAR equations cannot be interpreted individually because they are part of a dynamic system in which all variables are interrelated. That is, the net effect of a predictor may be different from its effect on a particular lag due to autoregressive effects and feedback loops. Therefore, we additionally used the techniques of Granger Causality and Cumulative Orthogonalized Impulse Response function (COIRF) analysis (Granger, Reference Granger1969; Lütkepohl, Reference Lütkepohl2005; Brandt and Williams, Reference Brandt and Williams2007). The Granger Causality technique evaluates whether current values of Y are (significantly) predicted by previous values of X beyond previous values of Y only. We performed COIRF to evaluate the dynamic effect size of those associations between endogenous variables that showed a significant Granger Causality or contemporaneous association. COIRF evaluates the net strength of the associations, by visualizing the cumulative effect of a shock (or 1 SD increase) in one variable on the other variables over a certain period of time (in our case 10 days). It incorporates potential effects of different lags, autoregressive effects and feedback loops, and takes into account both contemporaneous and lagged associations. It assumes a specific ordering of the contemporaneous association. For the associations of sleep with depression and cognition, the ordering of the contemporaneous association was always from sleep to depression/cognition because this was inherent to the design (see Figure 1). Therefore, we examined only this order for the association of sleep with depression and cognition. For the association between depression and cognition the order of the contemporaneous association was unknown (because they were assessed right after one other in the evening diary, see Figure 1), so we examined both orders. COIRF analyses were performed using the irf function of the vars package in R.
In order to estimate whether the presence and nature of the associations differed between persons, we compared the r-coefficients of the contemporaneous associations, and the VAR estimates between persons using Fisher Z’s formula: Z = (z1 – z2)/(square root of [(1/N1 – 3) + (1/N2 – 3)], where z = .5[ln(1+r) – ln(1–r)], and N is the length of the time series (t) (Fisher, Reference Fisher1925). All pairs of associations with z < −2.58 or z > 2.58 were deemed statistically significant (which is analogous to p < .01).
Because multiple tests were done, we used a more conservative alpha of .01 for indicating statistical significance.
Results
Sample
Twelve older persons participated in the i-CASE study. Two participants (participant 1 and 3) were excluded because their depressive symptoms were already in remission at the start of the study, one participant (Participant 6) refused to do the cognitive test battery, and for one participant (Participant 12) the multidisciplinary team of the Memory Clinic could not confirm MCI. Therefore, eight participants were included in the present analysis: four women and four men, aged 61–83 years, with varying levels of education, Geriatric Depression Scale scores ranged between 5 and 12, and Pittsburgh Sleep Quality Index scores ranged between 5 and 18. During the whole study period, participants slept on average 6.1 to 8.9 hours per night, scored between 1.3 and 5.0 on a scale from 1 to 7 on depressive symptoms, and it took them on average 618 to 912 ms for a correct response on the one-back task (see Supplemental Table 1).
Results of the VAR analyses: Granger causality and contemporaneous associations
The results of the VAR analyses accompanied with the Granger causality tests demonstrated differences in presence, nature (i.e. is it a positive or negative association), and direction (i.e. does X precede Y or does Y precede X?) of the associations between participants, which we describe below (see Figure 3A to 3F and 4A to 4D for lagged associations, and Table 1 for contemporaneous associations).

Figure 3. (A to F) Estimates and 99% CI of the lagged effects between sleep, depression and working memory resulting from the VAR analyses. The asterisks indicate a significant Granger causality effect (p < .01). For Participant 8 the depression score was logtransformed to achieve normality. Missing values were imputed with Amelia.
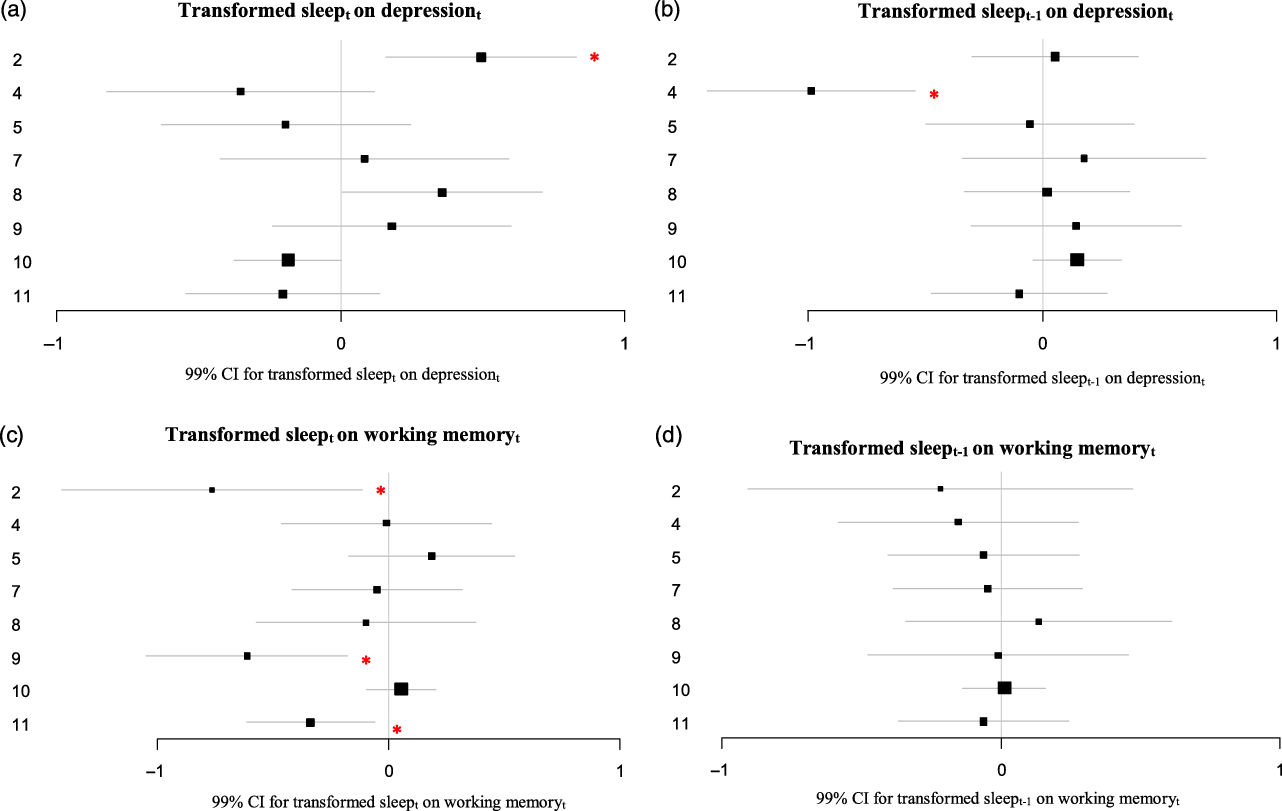
Figure 4. (A–D) Estimates and 99% CI of the lagged effects of more or less than average sleep on depression and working memory resulting from the VAR analyses.
The asterisks indicate a significant VAR effect (p < .01). For Participant 8 the depression score was logtransformed to achieve normality. For participant 10, the variables for depression and working memory were logtransformed to achieve normality. Missing values were imputed with Amelia.

Note. VAR = vector autoregressive modeling.
1 The correlation coefficients indicate the correlation between the residuals of the VAR models.
2 Missing values were imputed by the r package Amelia
*p < .01; **p < .001
Effects on depressive symptoms
For three out of eight participants, significant effects on depressive symptoms were found. More sleep last night preceded more depressive symptoms in the following evening in one participant (Participant 10; r = .39; p < .001; see Table 1, results of the contemporaneous associations). This effect in participant 10 was significantly different from that in participants 2 and 11 (Fisher Z: −4.04 and 2.70, see Supplement 2). For none of the participants sleep the night before last night significantly granger caused depressive symptoms in the evening (Figure 3A). Better working memory performance in the evening granger caused less depressive symptoms the following evening in Participant 7 (so indicating significant Granger Causality: B = −0.45; p(Granger causality) = .007). In contrast, better working memory performance in the evening granger caused more depressive symptoms the following evening in Participant 4 (B = 0.35; p(Granger causality) = .009; Figure 3B). Fisher Z test supported the difference in presence and nature of this association in participants 4 and 7: the association in these participants differed significantly from each other, but also from most other participants (see Supplement 2).
Effects on total night-time sleep duration
None of the participants had significant granger causality on total night-time sleep duration (see Figure 3C and D).
Effects on working memory performance
Two out of eight participants had significant effects on working memory performance. For Participant 8, more hours of sleep last night (r = −0.35; p = .005; see Table 1) as well as the night before last night (B = −0.30; p(Granger causality) = .008; see Figure 3E) preceded worse working memory performance. This effect in participant 8 was significantly different from that in participants 4 and 11 (Fisher Z: 3.61 and −3.81, see Supplement 2). For Participant 11, more depressive symptoms yesterday evening granger caused worse working memory performance the following evening (B = −0.25; p(Granger causality) = .009; Figure 3F). This effect in participant 11 was significantly different from that in participant 2 (Fisher Z: 4.30, see Supplement 2).
Effects of more or less than average sleep
The transformed sleep variable could not be entered as an endogenous variable in the VAR models, because it was highly skewed and could not be normalized through transformation. Therefore, two variables representing more or less than average sleep at t and at t–1 respectively were entered as exogenous variables in the VAR model. For two participants more or less than average sleep preceded depressive symptoms. For Participant 2, more or less hours of sleep than average preceded more depressive symptoms the following evening (B = 0.49; p < .001, Figure 4A). This effect in participant 2 was significantly different from that in most other participants (see Supplement 2). For Participant 4, more or less hours of sleep than average preceded less depressive symptoms the evening of the day thereafter (so, with an extra day in-between; B = −0.99; p < .001; Figure 4B). For three participants more or less than average sleep preceded worse working memory performance (Participants 2, 9 and 11; B = −0.76; p = .005, B = −0.61; p < .001 and B = −0.34; p = .002 respectively; Figure 4C). This effect in participants 2 and 9 indeed were significantly different from that in the 4 participants without an effect, but in participant 11 the effect differed significantly from that in participants 2 and 5 only (see Supplement 2). For none of the participants more or less than average sleep preceded worse working memory performance the evening of the day thereafter (so, with an extra day in between; see Figure 4D).
Results of the cumulative orthogonalized impulse response function analysis (COIRF)
The COIRF analyses showed differences in nature and direction of the associations between depression and working memory over a period of 10 days while incorporating potential effects of different lags, autoregressive effects and feedback loops (see Supplemental Table 2, and Supplemental Figure 1). To illustrate, for participant 4, a 1 SD shock (increase) in working memory resulted in a 0.51 or 0.31 SD increase in depression over a 10-day period (depending on which order for the assumed contemporaneous association was chosen), while for participant 7, it resulted in a 0.59 or 0.58 SD decrease in depression (although the associations were not statistically significant, see Supplemental Table 2). So, the nature of the association differed between these two participants. Furthermore, in participant 11 the direction of the association differed from that of Participants 4 and 7 (i.e. a 1 SD shock in depression was associated with a 0.35 or 0.26 SD decrease in working memory over the next 10 days [so from depression to working memory vs. the other way around]). Furthermore, a shock in sleep did not significantly predict changes in working memory in participant 8 nor depression in participant 10 (see Supplemental Table 2, and Supplemental Figure 1). We were not able to evaluate the impact of a shock in the transformed sleep variable (representing the effect of a 1 SD increase or decrease in sleep) on depression or working memory because this sleep variable could not be normalized through transformation, and therefore not entered as an endogenous variable in the VAR model and thus also not in the COIRF model.
Discussion
Summary of findings
This series of single-subject studies in eight older persons with depressive symptoms and cognitive impairments found that the presence, nature, and direction of the daily temporal association between depressive symptoms, working memory and sleep differed between persons. This suggests that different persons may need different types of interventions in order to alleviate depressive symptoms.
Interpretation of findings and clinical implications
Depressive symptoms and cognitive performance
For one of the eight participants more depressive symptoms on a given day preceded worse working memory performance the next day. For this participant, one SD increase in depressive symptoms on a given day was associated with about 0.3 SD decrease in working memory over a period of 10 days (see Supplemental Table 2, participant 11). For persons showing such an association, effective depression treatment may perhaps enhance cognitive performance. For one other participant the direction of the association was reversed: worse working memory on a given day preceded more depressive symptoms the next day. Being aware of the cognitive deficits may have resulted in an increase in depressive symptoms for this person. Although the cumulative effect of decreased working memory on depressive symptoms over a 10-day period did not reach statistical significance for this person, a psychological intervention to increase the adjustment to the cognitive impairments may perhaps be helpful for persons who do show such an association (e.g. Regan and Varanelli, Reference Regan and Varanelli2013).
Associations of sleep with depressive symptoms and cognitive performance
In two out of eight participants changes in sleep preceded changes in depressive symptoms, but the nature of this relation differed between the two participants. Longer sleep duration preceded depressive symptoms the following day in one participant, although the cumulative effect of longer sleep duration on depressive symptoms over a period of 10 days was statistically not significant. In another participant shorter or longer sleep duration than average for this person preceded depressive symptoms. Unfortunately, we were not able to evaluate the cumulative effect of shorter or longer sleep duration on depressive symptoms over a period of 10 days, because this variable was highly skewed (and could not be entered as an endogenous variable). A previous nomothetic study also found longer or shorter sleep duration than average to be associated with worse depressive symptoms (Van Mill et al., Reference Van Mill, Vogelzangs, van Someren, Hoogendijk and Penninx2014).
For none of the participants, changes in depression were associated with changes in sleep the following night, which is in contrast to a review that reported bi-directional effects between affect and sleep (Konjarski et al., Reference Konjarski, Murray, Lee and Jackson2018). This may be explained by the fact that some symptoms of depression may result in more sleep (e.g. loss of energy/fatigue), while other symptoms may result in less sleep (e.g. ruminative thinking).
Longer sleep duration was associated with worse next-day working memory performance in one out of eight participants. However, the cumulative effect of longer sleep duration on working memory over a 10-day period was not significant for this participant. In three other participants, shorter and longer sleep duration than average was associated with worse next-day working memory performance. This suggests that too short and too long sleep duration may both impact cognitive performance in the same person.
For persons in whom longer sleep duration has adverse effects sleep restriction therapies may perhaps be beneficial (Lancee et al., Reference Lancee, Maric and Kamphuis2019), while persons for whom longer or shorter sleep than usual has adverse effects may benefit from combined strategies to improve sleep as well as restrict sleep duration within a certain limit (e.g. Gebara et al., Reference Gebara2018; Gee et al., Reference Gee, Orchard, Clarke, Joy, Clarke and Reynolds2019; Lancee et al., Reference Lancee, Maric and Kamphuis2019).
Implications for clinical care
This study showed that different persons may benefit from different interventions or advices. Implementing ambulatory diary and sensor assessments in clinical care settings may therefore help formulate patient-specific interventions or advices. However, it remains unclear to what extent it is feasible to equip a large number of patients with ambulatory sensors. Currently, technologies are increasingly being developed to enable this. Clinical care applications that implement single-subject analyses in clinical care settings to help in the diagnostic process are being developed (Fernandez et al., Reference Fernandez, Fisher and Chi2017; Fisher et al., Reference Fisher2019; Bos et al., Reference Bos2019, conference abstract). In order to support this, tools to automatically preprocess and analyze data are being developed. Examples of this are the development of AutoVar to automatically perform VAR analysis (Emerencia et al., Reference Emerencia, van der Krieke, Bos, de Jonge, Petkov and Aiello2016; van der Krieke et al., Reference Van der Krieke2015), the development of an r-package that automatically preprocesses actiwatch data (ACTman: Kunkels et al., Reference Kunkels, Knapen, Zuidersma, Wichers, Riese and Emerencia2020), and the development of a platform that integrates data from sensors and diaries (Physiqual; Blaauw et al., Reference Blaauw2016). Furthermore, implementing single-subject research in clinical care will also be more feasible when it is rewarding for patients to participate. Personalized feedback encourages shared decision making and patient empowerment, and appears particularly motivating to increase compliance in large samples (Kramer et al., Reference Kramer2014; Van der Krieke et al., Reference Van der Krieke2017; Van Roekel et al., Reference Van Roekel, Vrijen, Heininga, Masselink, Bos and Oldehinkel2017). So, in the near future diary and sensor assessments may be implemented in clinical care settings.
Methodological considerations
The present study has several strengths. First, the intensive longitudinal assessment for each participant made it possible to evaluate temporal associations at the level of the individual patient. Second, although the sample consisted of older persons with cognitive impairments, adherence was relatively high, suggesting that this patient group is capable of performing daily assessments. This study has also some methodological challenges. First, as inherent to the single-subject study design, the results are not generalizable to other patients. However, generalizability was not an objective of the present study as the aim focused on finding patterns in individual persons. Second, like in nomothetic studies, the found associations in the present study are not necessarily causal, but may be explained by other unmeasured (fluctuating) variables. Third, the associations found by the present study do not say something about long-term effects, such as the course of depressive episodes. Instead of this, information about daily associations may be helpful for defining personalized daily intervention strategies, which may also have long-term effects (e.g. Van Roekel et al., Reference Van Roekel, Vrijen, Heininga, Masselink, Bos and Oldehinkel2017). Fourth, it remains unclear to what extent unreliability of each instrument may have influenced the current findings. Psychometric studies on single individuals should therefore be topic of future single-subject research. Fifth, we had to transform depression data for participant 8, while for the other participants untransformed data was used. This may have affected the coefficients for this participant, and limited comparison to the coefficients of the other participants. However, we chose not to transform data of all participants, because the assumptions were met with the untransformed data, so transforming is not advised (Feng et al., Reference Feng2014).
Conclusion
The presence, nature and direction of the associations between sleep, depressive symptoms and cognitive performance differ between persons. Getting more insight into these daily temporal associations for a specific patient may guide the treating specialist in formulating an intervention strategy, which may help maintain the patients’ health, quality of life, functional outcomes and independence.
Conflict of interest
None.
Source of Funding
This work was supported by Stichting MIND (project code 2013 6740) and Alzheimer Nederland (project code WE 09-2014-01).
Description of authors’ roles
MZ, RCOV and AL drafting first version of manuscript and substantially contributed to the design and conception of the work, all authors: critically revised the manuscript, read and approved the final version of the manuscript.
Acknowledgements
We would like to thank all the participants of this study for their participation. We would like to thank Elisabeth H (Elske) Bos for her kind advice with the analyses.
Supplementary material
To view supplementary material for this article, please visit https://doi.org/10.1017/S1041610221000065