1 Introduction
The Cohesion Policy is the most important investment policy of the European Union (EU); it aims at reducing the wide regional disparities existing in Europe,Footnote 2 by supporting economic growth and sustainable development of regions and cities, favoring job creation and business competitiveness, as well as improving quality of life of EU citizens, particularly in lagging behind regions (European Commission, 2016a ). The core of this policy is a coordinated mechanism of investment, primarily in the form of capital grants, disbursed by the EU to the Member States in addition to their national public spending.
This paper investigates the role of CBA, a methodology with a long tradition for the evaluation of public investment, in the context of the EU Cohesion Policy. At the European Commission (EC) level, CBA was first introduced in 1994 by the Directorate General for Regional Policy, with the release of the first CBA Guide. Since then, the Cohesion Policy has gradually promoted the practice of CBA, which has become today a mandatory requirement for applications of major projects whose total eligible cost exceed €50 million.Footnote 3 Five subsequent editions of the Guide (European Commission, 1994, 1997, 2002, 2008, 2014) have laid down the rules for project appraisal.
In order to apply for EU funds, Member States are required to present a financial and economic analysis of the investment project. A necessary requirement for obtaining the grant is that the project is not financially attractive for capital markets while economically efficient from the point of view of the society. In fact, a distinctive aspect and considerable advantage of the EC approach to CBA is that the estimation of both projects’ financial rate of return (FRR) and economic rate of return (ERR) is required in funding applications. While the former gives an indication of the project’s financial profitability and must be negative (or below the profitability level required by private investors), the latter shows whether the project is beneficial for the society and should therefore be higher than the social discount rate (SDR), as explained in Section 3.
This paper considers, project by project, the relation between ERR and FRR as a summary indicator of the role played by CBA in the appraisal process. In fact, such relation shows how extensively the use of shadow prices, the inclusion of externalities, and in general of nonmarket effects, readjusts the economic evaluation of the project compared to the financial one. In this perspective, the divergence for each project between ERR and FRR can be considered as a proxy indicator of the CBA role in taking into account market distortions and capturing the expected social benefits of the project, beyond its profitability from a simple financial point of view. Hence, we ask two simple questions in this paper: to what extent has CBA introduced corrections to the financial analysis, when performing an economic appraisal of major projects submitted to the EU during the last programming period? What are the main drivers of such corrections?
After presenting the development of the CBA practice over the last years in the context of the Cohesion Policy, we report some statistical evidence based on ex ante CBA of around 1000 projects appraised during the period 2007–2013, representing almost €180 billion of total investment cost. This appraisal is usually carried out by Members States, and the preparation of the CBA documentation is largely assisted by experienced consultants or by experts of JASPERS.Footnote 4 In the considered period, project applications were systematically appraised by the staff of the EC in some cases with the support of independent consultants. Our analysis focuses exclusively on data from Cohesion Policy grants applications; we do not have access to data on other projects for which Member States have decided not to apply for the EU grants. Moreover, we cannot control for optimism bias of the applicants or any issue of asymmetric information, neither this paper analyzes the subsequent history of the project, such as the EC decision on disbursing the grant or the implementation processes (e.g., some projects have been withdrawn, and some of them modified in terms of scope and/or timing). Our focus is limited to the ex ante appraisal at the time of the application, and particularly on CBA.
We find that, on average, the expected FRR is slightly negative (
$-2.9$
%), while the ERR is largely positive (16.2%); this suggests that, according to the applicants, proposed projects are expected to be beneficial for the society, although they are still not attractive for private investors. Moreover, by using simple econometric techniques, we find that ERRs and FRRs are positively correlated, but to a different extent in different sectors. By controlling for the size of investment, the time horizon of the CBA analysis, the location of the project, we find that projects in information and communication technology (ICT), roads and motorways and productive service show a higher ERR compared to the FRR. We discuss possible interpretations of these empirical findings, which are novel and not obvious. In fact, the relation between ERR and FRR can be weaker when (positive) externalities are very important (e.g., in some transport and environmental projects) and when shadow and market prices considerably diverge.
The rest of the paper is organized as follows: Section 2 presents the changing landscape of the Cohesion Policy over the last twenty years and the development of the CBA Guide since the release of its first edition in 1994 until the current one. Section 3 summarizes the most important feature of the CBA approach adopted and discusses some key parameters and important methodological issues. Section 4 presents a simple framework to empirically investigate the relation between ERR and FRR of projects evaluation, looking at data from a large sample of major projects appraised in the programming period 2007–2013. Section 5 presents the results of the empirical analysis while the concluding Section 6 discusses lessons learned from the analysis of ex ante CBA in the framework of Cohesion Policy, and challenges ahead for further research.
2 Background: the increasing importance of the CBA guide in response to the changing landscape of the EU Cohesion Policy
Since the European Economic Community was established in 1957 with the Treaty of Rome by six founder states,Footnote 5 and particularly after the first enlargement in 1973 where Denmark, Ireland and the United Kingdom obtained the EU membership, the regional policy has endured important transformations in terms of main objectives, financial tools available, allocated budget and so on (Goulet, Reference Goulet2008). In this evolving scenario, where the Cohesion Policy began to assume an increasingly high importance and the amount of allocated funds was rapidly growing, the practice of CBA started acquiring a stronger relevance and legal power which made it become the key tool to rule applications for funding of major investment projects by Member States. Table 1 presents an overview of the main objectives of the Cohesion Policy, allocated budget, country membership following the process of EU enlargement, different editions of the CBA Guides and their legal base, during five different programming periods.
Table 1 The evolution of the CBA guide in response of the changing landscape of the Cohesion Policy (1988–2020).

Before 1987, applications for Structural FundsFootnote 6 were left at the discretion of national governments and mostly considered as a sort of reimbursement for their contribution to the community budget (Sutcliffe, Reference Sutcliffe2000). However, after 1987 with the Single European Act, the Commission decided to start earmarking a five years budget for the Cohesion Policy, thus calling for a need to have common guidelines to evaluate and compare ex ante different project applications of Member States. In 1993 and 1994, the EC regulation governing Structural and Cohesion FundFootnote 7 started to require a CBA, as long with other types of information and the EC commissioned to a team of experts the first edition of its CBA Guide (European Commission, 1994); this was a brief document of 28 pages, without any legal status, just intended to bring some discipline in the applications of European Regional Development Fund (ERDF) which were very heterogeneous. In this respect, a study conducted by Florio (Reference Florio1997) on a sample of major projects co-financed during the period 1988–1993 shows the severe dispersion in some key parameters of CBA, also highlighting the fact that key items of CBA were available only for few applications.
The second version of the Guide (84 pages) (European Commission, 1997) presented minor methodological changes compared to the previous edition whilst the use of the Guide was also extended to the appraisal of Cohesion Fund (CF) applications. A third augmented edition of the Guide released in 2002 (European Commission, 2002) consisted of 135 pages. As Table 1 shows, the legal bases of this new guide laid on a new set of updated regulations for Structural Funds, CF and ISPAFootnote 8 applications, providing further and more specific indications on how to carry out the appraisal.
A major transformation of the Cohesion Policy took place in the period 2000–2006, following the biggest enlargement of EU. This enlargement, which saw ten new countries mainly from Eastern Europe obtaining the EU membership in 2004,Footnote 9 significantly amplified the disparities among regions as the EU’s population increased by 20% whilst its GDP only by 5% (European Commission, 2017). Figure 1 presents the geographical distribution of those regions whose GDP per head at Purchasing Power Parity (PPP) was inferior to the EU 27 average,Footnote 10 in the year of the enlargement. As the figure shows, lower income regions were mainly concentrated in New Member States, but also in Portugal, Greece and Southern Italy.
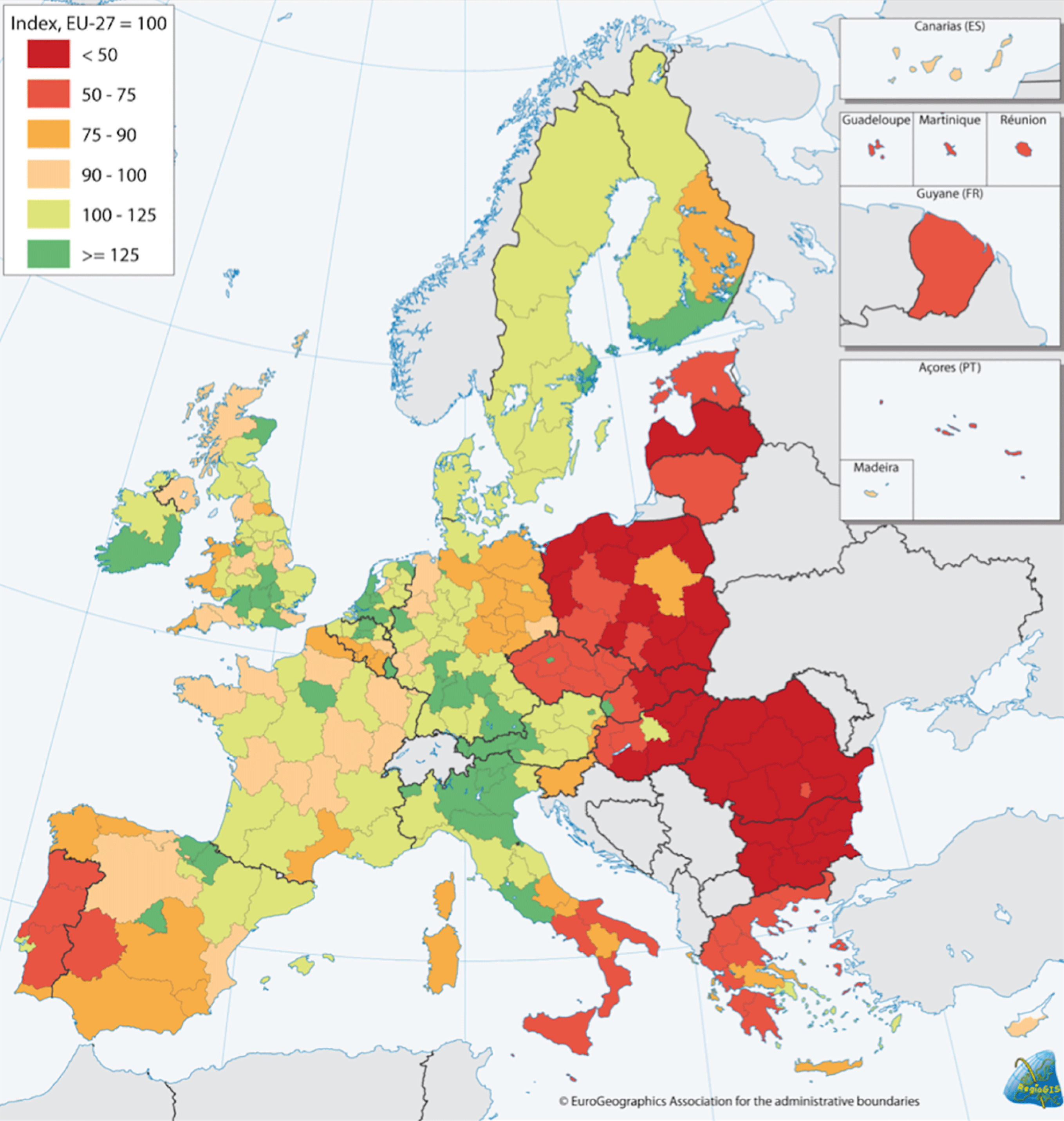
Figure 1 GDP per head at PPP during the year of the biggest enlargement (2004). Source: European Union (2007a , p. 8).
During the following period 2007–2013, relevant for the analysis of project applications undertaken in this paper, two other Eastern countries, Bulgaria and Romania, joined the community. The EU allocated €347 billion for Cohesion Policy, with the highest concentration of funds earmarked for lagging regions (Dijkstra, Reference Dijkstra2014; European Commission, 2017). In terms of investment composition, there was also a shift of priorities in less developed regions from infrastructure development toward business competitiveness and innovation goals (Dijkstra, Reference Dijkstra2014). Moreover, compared to the previous period, a larger number of actors at a different level were involved in the selection process such as different managing authorities and EC services. This emphasized the necessity to have clearer guidance and common rules to select the best projects and facilitate learning mechanisms among players (European Commission, 2008). To allow for timely preparation of projects, the Working Document n.4 was published in 2006 by the EC, leading toward higher levels of consistency and rigor in conducting CBA (European Commission, 2006). This document also introduced an updated and more effective mechanism to calculate the base of the EU grant, as explained in the next section, and was followed by the fourth edition of the Guide (European Commission, 2008). The methodological development of the new Guide was favored by the experience gained through the use and application of the previous editions especially after the period of enlargement (European Commission, 2008). Although it was still considered as a set of suggestions, for the first time the EC had to check whether different applications were coherent with this guidelines, for example, in terms of working hypotheses and methods used for the calculation of performance indicators (European Commission, 2008).
Finally, the latest and current version of the Guide was released in 2014 and consists of 358 pages. The Guide offers comprehensive guidance for performing CBA and presents specific recommendations and case studies for five main sectors (transport, environment, energy, broadband, and Research & Development (R&D)) according to the priorities of the period 2014–2020. Today, CBA is mandatory to apply for co-funding (ERDF and CF) and the 2014 Guide, is backed by the EU legislation. Thanks to the Implementing Commission Regulation 207/2015 (European Union, 2015) setting out in a legally binding manner the main principles of conducting the CBA, the 2014 Guide can be now considered the reference point for all managing authorities and those involved in the project appraisal (European Commission, 2014).
3 The economic and financial analysis of projects: key methodological issues
In this section, we briefly summarize the main methodological CBA approach adopted by the EU, which is consistent across the five editions of the guide. In particular, we refer to the more recent CBA Guides (there are no significant differences between the 2008 and 2014 editions). A key and distinctive aspect of such approach is that the application for funding requires both a financial and an economic analysis of projects within a consistent accounting system. Here we first introduce both types of analysis and then we focus on the relation existing between the two.
3.1 Financial analysis
The main purpose of the financial analysis is to assess whether the project is sustainable and/or viable from a financial point of view. In this respect, the financial net present value (FNPV) gives an indication of the ability of the project’s net revenues to repay the initial investment, regardless of the sources of financing; it is calculated as a difference between the expected investments and operating costs less the expected revenues, all values discounted and net of potential avoided costs and occurred benefits in a counterfactual scenario (otherwise called “without the project” scenario).Footnote 11 The EC Guide adopts the Discounted Cash Flow method which consists in discounting project’ costs and revenues, usually expressed in real terms, with a reference Financial Discount Rate (FDR), for a time horizon which varies depending on the sector. This represents the opportunity cost of the capitalFootnote 12 and is calculated looking at possible returns of alternative financial investments. The average financial rate of a mix of securities, suggested by the Guide as a reference point for the financial analysis of EU projects in the period 2007–2013 which is relevant for this analysis, was 5% in real termsFootnote 13 (European Commission, 2008).
The estimation of the FRR defined as the discount rate that produces a FNPV equal to zero is also required to project applicants. The calculation of both the FNPV and the FRR is based on observable market prices. A project in need of financial support and therefore eligible to receive a EU grant, should present a negative FNPV meaning that it is not profitable from a financial point of view as it is not able to generate sufficient revenues given the reference FDR.Footnote 14 At the same time, the FRR which is a scale-invariant pure number, should assume a lower value compared to the FDR (European Commission, 2008, 2014).
Before introducing the economic analysis, we discuss the pro-rata application of the discounted net revenues (Implementing Reg. 207/2015), formerly known as “funding gap method,” which is often used as the base for the grant calculation. This approach is based on the idea that the EU grant should only co-finance the portion of investments which is not covered by future net revenues (Florio & Vignetti, Reference Florio and Vignetti2012) and is also used to create incentives for attracting private capital, besides the EC contribution (Mairate, Reference Mairate2010). The pro-rata application of the discounted net revenues is calculated as follows:

where
$\text{Inv}$
is the net present value of the investment costs and
$\text{NetRev}$
is the net present value of the difference between revenues and operating costs plus the residual value of assets (all values discounted using the FDR). A limitation is that usually investment costs incur in the early years of the projects and are not much affected by high FDR, while revenues accrue later; this may translate into inflated funding gap rates increasing the possibility to request higher grants to what is actually needed (Florio & Vignetti, Reference Florio and Vignetti2012). Another drawback is that applicants may have an incentive to overestimate costs and underestimate revenues in order to require higher grants. In this perspective, according to the currently adopted “grant decision framework,” the EU should not cover the whole financial gap and the EU grant should be calculated as follows:

where
$\text{EligibleCost}$
is the cost remaining after deducting ineligible costs from the total costs of the major project (European Commission, 2016b
);
$\text{MaxCR}$
is the maximum co-funding rate fixed for each priority activities. This mechanism meant to ensure that the EU grant does not cover the full financial gap as it was happening before 2006, but only co-finances it (Mairate, Reference Mairate2010).Footnote
15
3.2 Economic analysis
After conducting the financial analysis, the subsequent economic analysis consists of checking whether the project is desirable from a social point of view.Footnote 16 As for the financial analysis, social costs and benefits should also be net of potential avoided costs and occurred benefits in a counterfactuals scenario. However, with respect to the financial analysis, the economic analysis introduces important corrections which include the use of shadow prices the consideration of externalities and nonmarket effects, as explained later in this section.
First, to discount social costs and benefits the economic analysis uses the SDR that “reflects the social view on how future benefits and costs should be valued against present ones” (European Commission, 2008; European Commission, 2014, p. 55). In perfectly competitive markets the SDR and FDR coincide; however this does not happen in real life as markets are often inefficient (Florio, Reference Florio2006). To calculate the SDR, the Social Rate of Time Preference (SRTP) is the method adopted by the Guide (HM Treasury, 2003; Hepburn, Reference Hepburn2007; Hagen et al., Reference Hagen, Berntsem, Bye, Hultkrantz, Nyborg, Pedersen, Sandsmark, Holst Volden and Åvitsland2012); this reflects the rate at which a certain society is willing to postpone its consumption today in exchange of higher level of consumption in the future (Arrow, Reference Arrow1999). Although the SDR can be estimated with different methods, the Guide suggests the adoption of the Ramsey (Reference Ramsey1928)’s formula of economic growth and which defines SDR as follows:

where
$g$
is the expected growth rate of a macroeconomic variable used as a proxy of welfare, usually consumption per capita; the idea is that whether future generations will be richer than present ones, the SDR will increase, thus giving more importance to present (and poorer) generations.
$e$
is the elasticity of marginal social welfare with respect to consumption and measures how much worthy is to transfer income from future richer generations to present poorer ones.Footnote
17
ptp is the rate of a pure time preference which includes two components: the first captures the fact that individuals usually prefer consuming today rather than tomorrow; the second takes in consideration the risk of death of human beings and may be captured by the mortality rate. When ptp is positive, the welfare of current generations is preferred to future ones. While estimates for
$g$
are easily available, applied economic research is needed to estimate the other parameters.
With respect to the Ramsey’s formula it is important to highlight that consumption growth depends on GDP growth which considerably varies across different EU countries and regions, in particular when looking at the current composition of EU28, which encompasses highly heterogeneous countries. In fact, growth rates of New Member States are much higher compared to EU15 countries and this is the main reason why the last two versions of the Guide, following the methodology proposed by Florio (Reference Florio2006), suggest adopting two different SDRs: 5% for cohesion countries and 3% for the other Member States. Hence, whilst the Guide recommends a single FDR for all EU28, the use of a higher SDR to discount project’s costs and benefits in cohesion countries compared to EU15, reflects the higher importance given by the Cohesion Policy to the welfare of present generations in most deprived areas.Footnote 18
As previously introduced, another important difference between the economic and financial analyses is that whilst the former evaluates cash flows at market prices, the latter uses shadow prices (Drèze & Stern, Reference Drèze and Stern1987, Reference Drèze and Stern1990; Londero, Reference Londero2003). Shadow prices capture the opportunity costs of goods and services as market prices are often distorted due to inefficiencies (e.g., situation of monopoly, subsidies, etc.) particularly in EU lagging regions (OECD, 2015).
To calculate shadow prices the Guide suggests different methods based on the standard literature. With respect to project’s inputs, if these are tradable (e.g., raw materials), the Guide recommends the application of “border price,” thus excluding custom duties or other taxes applied after these goods cross the national border (Little & Mirrlees, Reference Little and Mirrlees1974; Saerbeck, Reference Saerbeck1990; European Commission, 2008). If inputs are not tradable, a standard conversion factor is used for minor items such as administrative costs whereas for other major items (e.g., land) long-run marginal cost is adopted.
With respect to labor, regional shadow wages are calculated following the methodology suggested by Del Bo, Fiorio and Florio (Reference Del Bo, Fiorio and Florio2011). This methodology takes into account heterogeneous labor markets across EU regions where market wages do not often reflect the real opportunity cost of labor due to wages rigidities, the existence of legal minimum wages and other structural reasons. The general formula used to calculate the shadow wage rate (SWR), under the assumption that all workers’ income is spent on consumption is:

where
$r$
is a certain EU region,
$m_{1}$
is the marginal productivity of the worker which will be displaced by the project from one sector to another,
$w_{2}$
is a proxy of wage in a competitive market where the worker will be employed thanks to the project and
$\unicode[STIX]{x1D6FD}$
is a regional welfare weight. The idea behind this formula is that, when a worker is displaced from an activity to another one, the opportunity costs which capture what the economy has lost, is given by the worker’s output in his previous activity. However, this formula takes into account that every time a worker is displaced from his/her previous work there could be social costs such as transport, training and other opportunity costs that are not entirely captured by market wages. Thus SWR considers the net social cost of labor of a region as “a welfare-weighted linear combination of the previous (ex ante) and of the current (postproject) social value of the new job opportunity” (European Commission, 2014, p. 314).
According to the methodological approach suggested by the Guide, this general formula is then re-adapted to reflect different regional specificities of the EU. More specifically, Del Bo et al. (Reference Del Bo, Fiorio and Florio2011) identify four clusters of regions in terms of employment, wage rigidities, migration flows, urban–rural dualism and so on. The use of regional shadow wage constitutes a significant methodological advancement of the Guide and it is in line with the overall objectives of the Cohesion Policy of achieving growth, convergence and reducing unemployment. Unfortunately we do not have systematic data about the values of shadow wages used in the applications, but visual inspection suggests that shadow wages are mostly adopted using shortcuts based on unemployment rate (see European Commission, 2014; p. 59 for further info).
With respect to the project’s outputs, these are here calculated through the willingness to pay (WTP) principle, which measures the maximum amount that people are keen to pay for a desirable outcome. WTP can be estimated through different techniques (Johansson & Bengt, Reference Johansson and Bengt2015), for example, by calculating the saved costs that would have incurred if users had bought the same good from an alternative source.
Apart from the estimation of the shadow prices, another important characteristic of the economic analysis is that it should include externalities and nonmarket effects. These comprise, for example, the impact on the quality of life, the production of project’s externalities such as noise, soil contamination, deterioration of landscapes and GHG emissions among others (Johansson, Reference Johansson1987). Environmental aspects are particularly important since Europe is becoming more and more interested in supporting environmentally friendly activities and in promoting higher efficiency in resource management (Dijkstra, Reference Dijkstra2014). Considering the environmental impact of the project on landscape, pollution, waste production and so on, it is essential to reveal the real economic benefit of the project for the society. Due to their nature, positive or negative externalities and nonmarket effects need to be assessed separately often using the WTP approach. Although, in recent years, significant progress has been made to give credit to costs and benefits that spillover from the project without monetary compensation, additional theoretical and empirical efforts are still needed (European Commission, 2008, 2014).
In conclusion, projects eligible for EU funding, apart from being in need of co-financing, should present a positive economic net present value (ENPV) meaning that the project’s overall benefits for the society are expected to exceed the social costs, all values discounted with the SDR. Another indicator measuring the project economic performance is the ERR which is defined as the rate that produces a zero ENPV. Like the FRR, it is a scale-invariant pure number; when the ERR is higher than the SDR and the ENPV is positive the project is worthy to be implemented (European Commission, 2008, 2014).
4 Framework of the empirical analysis and descriptive statistics
As previously mentioned whilst FNPV and FRR are based on market prices, ENPV and ERR are based on shadow prices and take into account externalities (European Commission, 2008, 2014). This lead to the following equation valid for each project
$i$
:

where
$p$
is a vector of market prices,
$v$
is a vector of shadow prices,
$q_{i}$
is a project-specific vector of quantities of inputs and outputs for
$n$
goods, while
$t$
is the time horizon of the analysis. Externalities are simply the case of inputs or outputs valued zero by markets whilst different from zero values when using shadow prices. By definition
$\text{ENPV}=0$
when the internal rate of return ERR is entered in the formula, and similarly
$\text{FNPV}=0$
when the internal FRR is used, because internal rates of return are defined as those rates that lead to zero NPV (Boardman, Greenberg, Vining & Weimer, Reference Boardman, Greenberg, Vining and Weimer2001; European Commission, 2008, 2014). Hence, the difference of ENPV of FNPV must also be zero when, respectively, the ERR and FRR are used to compute them. From (5) it follows that a condition for
$\text{ERR}_{i}=\text{FRR}_{i}$
to hold, it is that market prices are equal to shadow prices for each of the
$n$
goods and there are no externalities
$(p_{n}=v_{n})$
. In this perspective, the divergence between ERR and FRR for each project can be considered as a proxy indicator of the CBA role in taking into account market distortions.Footnote
19
In general, given the objectives of the EU Cohesion Policy, we expect that selected projects are those where FRRs are lower than FDRs; in fact such projects would not have been financed by capital markets although beneficial for the whole society. However, this does not imply that FRRs and ERRs are inversely correlated. In fact, with the exception of externalities where
$p=0$
, in general
$v=cp$
, where
$c$
is a conversion factor (Little & Mirrlees, Reference Little and Mirrlees1974; Londero, Reference Londero2003), hence
$v-p=v(1-\frac{1}{c})$
. The correlation between ERR and FRR given in equation (5) is tested empirically later.
CBA, as conceived in the framework of the Cohesion Policy, is useful to determine whether the project is actually in need of co-financing and desirable from a socio-economic perspective. If the FNPV is negative (the FRR is relatively low) whilst the ENPV is positive (the ERR is relatively high), the project is not attractive for capital markets although beneficial for the society and therefore worthy to be financed and implemented. The combination of the financial and economic analyses represents an important advantage for a fairer allocation of the grants. First, an important strength of this combined approach is given by the fact that applicants who may tend to overestimate project’s market benefits are likely to present higher financial revenues and therefore receive smaller grants.Footnote 20 Second, by carrying out the economic analysis following the principles suggested by the Guide, corrections of market failures are introduced in the portfolio of major projects which are selected for funding. These corrections mainly operate thanks to the mechanism of converting market prices into shadow prices and considering projects’ externalities and other nonmarket effects. The double assessment, financial and economic, highlights the importance of empirically studying the relation existing between FRR and ERR.
Our original dataset included over 1000 project applications, representing the entire population of project applications for ERDF and CF during the period 2007–2013.Footnote 21 In this perspective, the issue of sample selection is a minor one (although, as mentioned in Section 1, we could not consider projects funded outside the EU grant mechanism). Before starting the analysis, we had to carefully clean the database from outliers and inconsistencies in data. For each relevant variable, such as total investment cost, ERR, FRR, FNPV and ENPV, we divided the relative distributions in percentiles, dropping observations if the corresponding value belonged to the 1st or 99th percentile. The dataset was therefore reduced to 945 projects; however due to the presence of missing data in some of the main variables of interest, estimations could be based on 762 observations only. We checked that the final sample was still representative of the original dataset in terms of the main variables of interests. The following descriptive statistics refer to the restricted sample.
Table 2 Number of projects by sector (2007–2013).

Notes: Sector classification taken and re-elaborated from the Commission Implementing Regulation (EU) No. 215/2014 of 7 March 2014 (European Union, 2014c ). “Other Transport” includes ports and inland waterways, local transport, air transport, multimodal transport, sustainable transport. “ICT” includes both demand stimulation, applications, services and infrastructure. “Other environmental services” includes also cultural heritage and cultural infrastructure. “Others” includes: Business development, Education & Education Infrastructure and projects for urban and rural regeneration. Source: Own elaboration on data provided by Directorate General for Regional and Urban Policy (2017).
Projects are located in 22 European countries and three macrosectors; as Chart 1 shows, around 66% of these projects are located in New Member States,Footnote 22 with Poland (185) and Romania (94) showing the highest number of applications.Appendix shows the sector composition at the country level.

Chart 1 Project applications by country (2007–2013). Source: Own elaboration on data provided by Directorate General for Regional and Urban Policy (2017).
At the sector level, Table 2 shows that the highest number of projects is concentrated in the transport sector (343) and environment (244). The number of projects in R&D, energy, ICT, and industry is in the range 30–40 for each sector whilst the remaining projects are in health, cultural heritage and other sectors such as education and urban regeneration.
The highest EU contribution requested by the applicants out of total investment is for environmental projects (around 60%) while the lowest for Productive Investment (around 13%). In terms of geographical distribution, New Member States require a much higher contribution (60%) compared to EU15 countries which require only 30% of the total expected investment.
Tables 3 and 4 present summary statistics for investment costs, FRR, ERR at country and sector levels. The total amount of investment costs is about €180 billion whilst the average investment cost is €190 million with a large standard deviation among countries and sectors. For example, the average investment cost in the Czech Republic is about seven times higher compared to Malta (Table 3) whilst the average investment in the transport sector is more than three times higher compared to the health sectorFootnote 23 (Table 4).
Table 3 Investment and returns by country.

Source: Own elaboration on data provided by Directorate General for Regional and Urban Policy (2017).
Table 4 Investment and returns by sector.

Source: Own elaboration on data provided by Directorate General for Regional and Urban Policy (2017).
In terms of sector share of investments at the country level, calculated as the ratio between total investment in each country out of total investment for each sector in the considered country, transport and environment projects have the largest share, both in EU15 and New Member States.
With respect to FRR the average value is
$-2.9$
% with a standard deviation of 6.3. As already observed by Florio and Vignetti (Reference Florio and Vignetti2005) a negative FRR is not surprising as these projects usually have low financial returns and for this reason they need support by the EU. For example, Table 3 shows how the average FRR in Lithuania is
$-11.8$
% meaning that, in this country, projects are likely to be much more in need of financial assistance compared to the EU15 average (
$-1.8$
%). At industry level, sectors with closer exposure to market are, not surprisingly, reporting positive FRRs, such as productive investment (6.6%) and energy infrastructures (3.5%). One sector which does not follow this pattern is ICT (
$-6.4$
%) and this can be explained by robust application of State Aid rules, as investments are often concentrated in rural and peripheral areas, with evident market failure in terms of broadband services.Footnote
24
Finally looking at the ERR, the average value is 16.2% with the highest return in the United Kingdom (24.9%), Italy (24.5%) and Germany (21.5%) and the lowest in Czech Republic (10.1%). This result suggests that economic benefits of these projects are higher in EU15 compared to New Member States; this could be partially explained by sector composition effect and the relatively small number of projects in the EU15. In fact, the proportion of projects in traditional infrastructure which presents a lower ERR is much higher in New Member States than in EU15 (for example, transport projects present lower ERR (14.4%) compared to other sectors such as ICT (33.8%)).
From summary statistics in Tables 3 and 4, it is also possible to notice that the standard deviation of ERR (12.8%) is much higher of the FRR (6.3%). According to Florio and Vignetti (Reference Florio and Vignetti2005) on the analysis of 240 projects in 11 ISPA countries, the high variability of FRR and ERR is not just driven by countries or sectors specificities but it depends also on project-specific characteristics as well as possible inconsistencies in applying the principles of CBA across different teams of experts preparing applications. It may be a safe assumption that certain inconsistencies continued during the 2007–2013 period, especially between countries assisted and not assisted by JASPERS which reviewed applications and CBAs for over 500 major projects in countries that accessed the EU after 2003.
We want to address two main questions: to what extent has the CBA introduced corrections to the financial analysis? What are the main drivers of such corrections? Whilst only a project by project review could provide detailed information about the way in which shadow prices, externalities and other nonmarket effects have been considered in the project appraisal, we are interested here to see the aggregate effect of CBA in respect to a financial appraisal.
While we cannot observe market and shadow prices in our data, inspection of the project applications suggests that these vary across industries and countries. For example, the marginal social value of time savings in transport projects is correlated to users’ income which widely differs across Member States (Bickel et al., Reference Bickel, Friedrich, Burgess, Fagiani, Hunt, De Jong and Navrud2006). Shadow wages, as previously explained, should be correlated to regional unemployment and specific labor market regimes. Moreover, the extent of externalities is certainly different across diverse industries. In order to address this variability we turn to a simple regression analysis in the next Section. However, before presenting our analysis, it may be interesting to mention some examples of these project applications to provide an intuition of the CBA role in this context. For example, in the road transport sector, projects with the highest economic impact are typically ring roads of cities that divert heavy traffic from the congested existing roads; these projects allow high savings in value of time, vehicle operating costs and show high environmental benefits. Moreover the more developed and congested is the region (and the city) in which this type of project is located, the better is their economic viability. For instance, the internal ring road of Wroclaw (Poland, Dolnoslaskie) achieves impressive ERR (68%); whereas in smaller cities, construction of ring roads on national roads brings smaller yet impressive returns: ring road of Serock (Mazowieckie) (37%), Jedrzejow (Swietokrzyskie) (31%), whereas sections in Eastern Polish regions score much lower: Jaroslaw (Podkarpackie) (15%), Hrubieszow (10%) and Barglow (Podlaskie) (6%). At the same time, all these roads are toll-free so the financial analysis is generally negative. The same pattern can be found in other countries such as Spain where the most economically viable project is the new eastern ring road of Malaga (46% ERR), whilst national motorways score much worse because of more limited traffic.
Productive investments analyzes are indeed a place for applicants to maneuver between financial and economic viabilities (e.g., certain UK technology transfer center has 6% of FRR while 38% of ERR). A productive investment in France has FRR of 13% while ERR of 30%. There are also productive investments with good financial scores while bringing less for society: one application in Poland has an impressive FRR of 38%, while ERR was less impressive (nearly 17%). Another productive investment in Spain has FRR of 60% while ERR is only 21%. Some projects were not approved because their FRR suggested they do not need financial assistance by the EU or they did not provide sufficient explanation of incentive effect in the region.
5 Results
The following Equation (6) is used as an empirical benchmark model to test the predictive power of financial returns on economic returns, after controlling for some project characteristics:

where,
$\text{err}$
and
$\text{frr}$
are, respectively, the economic and financial rates of return of a major project
$i$
. The remaining covariates are controls:
$\text{time}\_\text{horizon}$
represents the temporal horizon used for CBA analysis;
$\text{lninv}$
is the natural logarithm of the investment cost,
$\text{sector}$
and
$\text{country}$
are dummy variables to control for composition effects that may arise from sector heterogeneity and geographical location of the project, and
$u_{i}$
is the error term.
We estimate an ordinary least squares (OLS) regression. Table 5 shows the first set of results. Columns (1) and (2) consider ERR as dependent variable whilst FRR, investment cost and time horizon are the regressors; operating sectors and country dummies (in column 2 only) are additional controls; Column (3) disregards individual country dummies while it includes a simple dummy for EU15 and New Member States. Columns (4)–(6) add the natural logarithm of the average real GDP per capita at PPP in the period 2007–2013 for each country in which the project is located as an additional control.
Table 5 The relation between ERR and FRR of major investment projects (2007–2013).

Robust standard errors in parentheses ***
$p<0.01$
, **
$p<0.05$
, *
$p<0.1$
. Omitted Sector: Energy Infrastructure.
In all these specifications the coefficient of frr is positive and significant. This suggests the existence of a positive correlation between projects which are marginally favorable from a commercial point of view and those which are also worthy for the society. The fact, that FRR is a predictor of ERR suggests that, on average, bad projects in financial terms are not per se particularly good in economic terms.Footnote 25 This result may also be useful to stress the value of combining a financial and an economic analysis as proposed by the CBA Guide for a fairer allocation of the grants. In fact, applicants who tend to underestimate their financial revenues to maximize the expected grant risk to present economic benefits that are too low and will therefore have fewer chances to receive the grant.
The coefficient of time horizon used to discount cash flows is significant and negative in all specifications suggesting that when benefits are spread over a long period the impact on the ERR is negative, after controlling for the other variables. The coefficient of total investment cost is also negative and significant in all specifications. In fact, the ERR by construction is not an absolute indicator as it is the ENPV, and while it is likely that bigger projects in terms of investment cost have higher ENPVs compared to the smaller ones, in relative terms these may be less efficient.
The coefficients for sectors show the importance of the composition effect, confirming that even after considering the FRR, the aggregate ERR is influenced by the portfolio composition in terms of type of the investment supported. In this respect it seems that projects in ICT, road and motorways, and productive investment are relatively more socially efficient than projects in energy infrastructure, used a benchmark. This suggests that after controlling for projects’ scale and duration, CBA captures some sector specificities in terms of externalities and shadow prices. For example, as seen in Table 4, the average FRR in the road and motorways sector is negative; this possibly reflects the fact that road tolls are either nonexistent or inferior to the recovering costs, therefore these projects, on average, are not favorable from a financial point of view; conversely benefits for users expressed in terms of marginal social value of time savings and other typical ingredients of road infrastructures lead to high ERR showing that these projects are beneficial for the community. With respect to ICT, low FRRs are probably due to the high costs and modest revenues of broadband investments in rural and peripheral areas, whilst a particularly high ERR, reflects the social value of bridging the digital divide, consistently with the EU objectives in this matter. In contrast, energy project (the omitted benchmark sector in the analysis), possibly due to the liberalization of the industry in the EU and the consequent increase of tariffs, shows a positive FRR and while the ERR is higher, the spread is inferior to the spreads of roads, ICT, environment, and R&D. The wide gap between FRR and ERR in the case of productive investment (usually manufacturing) is more difficult to explain. The average FRR, as expected, is the highest across sectors, but its spread ERR–FRR is also high (Table 4). One reason could be that applicants in this sector make optimistic assumptions in estimating benefits (in order to justify the grants although this was not always a successful strategyFootnote 26 ); another possible reason could be related to the use of shadow wages. In fact, most of these projects are implemented in Spain (45%), Portugal (32%) and Greece (6%) where unemployment rates are high. Hence it is also plausible that by using low shadow wages to account for unemployment in Southern Europe, this sector shows a higher ERR.
While results for productive investment may be correlated to optimistic assumption of applicants or to the location of such projects in high unemployment regions, it is interesting that findings for ICT and roads are consistent with previous empirical results by Del Bo, Florio and Manzi (Reference Del Bo, Florio and Manzi2010), Del Bo and Florio (Reference Del Bo and Florio2012) and based on an entirely different approach and set of data. In this earlier literature a correlation between growth and the endowment of infrastructure is studied by empirically estimating an aggregate production function in a spatial econometrics context, using EU data at regional level. The main finding is that GDP growth is more strongly correlated to the availability of telecommunication and transport infrastructure. The fact that the same sectors emerge from a project-level analysis as particularly correlated to the spread between economic and financial returns seems interesting and is worthy of further research, where data at country/region and project level are compared more in-depth. In fact this is also consistent with other studies that highlight the strong socio-economic impact of EU funds when implementing large ICT infrastructure in peripheral areas (Catalano & Florio, Reference Catalano and Florio2017).
Table 6 The relation between ERR and FRR of major investment projects (2007–2013).

Robust standard errors in parentheses ***
$p<0.01$
, **
$p<0.05$
, *
$p<0.1$
. Notes: (1)(2)(3) Omitted Sector: Other Sectors (5)(6)(7) Omitted Sector: Other Sectors 2
$=$
Other Sectors
$+$
Environment.
In columns (4) and (5), it is also worth noting that when introducing both country dummies and GDP per capita we do not find any effect for GDP; however when controlling for New Member States versus EU15 countries in column (6) the effect becomes significant and negative. This indicates, as expected, that all being equal, countries with lower GDP per capita should be able to obtain higher social benefits from the project implementation. However the negative and significant coefficient for New Member States, compared to EU15, indicates that despite this catching up effect in terms of GDP, New Member States are still not capable of pushing their ERR compared to FRR, maybe because of lack of institutional capacity or skills in applying CBA techniques.
In order to further investigate the sector effect we group all sectors into three main categories (i) Transport; (ii) Environment and (iii) All other sectors, including R&D, ICT, health infrastructure and so on. Results are shown in Table 6, columns (1)–(3). In column (1) it is worth noting that, all other things being equal, projects in environment have a lower ERR compared to other sectors (used as the benchmark). This result could possibly highlight the difficulties for CBA of environmental projects in showing their economic benefits compared to their financial returns whilst compared to other sectors. In columns (2) and (3) when including interactions between investment and these three groups of sector dummies we notice that transport is the sector which shows higher economic benefits compared to the financial ones, after controlling for investment costs. Finally, in columns (4)–(6) as an additional robustness check, we group sectors into two main groups (1) transport and (2) all other sectors including the category environment. Results confirm again that projects in transport have higher ERR compared to all other sectors, all things being equal.
6 Concluding remarks
This paper contributes to the literature on CBA practice in three different ways. First it highlights the development of the CBA approach, from the first edition of the EC Guide in 1994 to the current one (2014), in response to the changing EU regional policy. In this context, CBA has been instrumental in creating a common evaluation framework among 28 Member States of the EU, in spite of considerable variability of national socio-economic conditions, institutional capacity, administrative and legal specificities. The evolution of the role of CBA for major projects appraisal under the EU Structural Funds, from an initially timid approach to a mandatory framework shows, at the same time, its flexibility and success in increasing the homogeneity in evaluation mechanisms. In fact, the EC Guide has developed consistently over its five editions through an increasing number of case studies and technical refinements. We suggest that the Guide represents a true European intellectual project and shows the value added of adopting a common project evaluation framework in regional policy in an otherwise highly fragmented panorama.
Second, we discuss a specific distinctive feature of the CBA Guide approach, which is common to all the five editions: the requirement that applications for funding must integrate the financial and economic analyses of projects. While this approach is not new, it is relatively unusual as a systematic screening mechanism. For example, most CBA manuals and operative procedures in public administration tend to focus exclusively on economic appraisal (Boardman et al., Reference Boardman, Greenberg, Vining and Weimer2001; De Rus, Reference De Rus2010; Warner, Reference Warner2010). However, there is an important advantage in the integration of these two perspectives; optimism bias in economic appraisal may lead to a self-defeating strategy of presenting projects with high financial returns, which usually may not be funded under the current EU Cohesion Policy regulations. On the other side, in public investment policies more emphasis is given to capital expenditure, while operational expenditure tends to be downplayed; in this context the important role of the financial analysis is to ensure that investment projects are financially sustainable (do not run out of cash in the reference period) while offering goods and services that are not captured by market prices.
Optimism bias and incentives for applicants to exaggerate benefits and underestimate costs are a constant feature of project evaluation, see the discussion of these issues by Florio (Reference Florio2007), De Rus and Socorro (Reference De Rus and Socorro2010), Flyvbjerg (Reference Flyvbjerg2013). It is not easy to contain this bias. In the case of the EU regional policy project applications are reviewed by various institutions: the EC, JASPERS, the European Investment Bank, and also by external consultants. This initial screening counteracts to a certain extent the problem. Moreover, while not in a systematic way, ex post evaluation of major projects has been launched by the EC and is ongoing (Kelly et al., Reference Kelly, Laird, Costantini, Richards, Carbajo and Nellthorp2015). The way in which the co-funding mechanism by the EU is now designed, as described in Section 3 (Equation (2)), somehow constrains the optimism bias in terms of costs and revenues against the amount of the grant that can be obtained.
Third, taking advantage of the above-mentioned feature, we analyze the relationship between the economic and financial rates of return of a large sample of projects as a statistical proxy of the role of CBA in correcting market prices by shadow prices and in including externalities. After controlling in simple empirical models for project scale, duration, and country fixed effects, there are two main findings. Financial and economic returns are positively correlated, showing that, on average, proposed projects, which are expected to be beneficial for the society, are not the most loss makers, although these would have not been financed by private investors. In other words, the CBA captures the expected socio-economic impact of the project by shifting its profitability after the EU grants, but this does not imply that CBA gives an advantage to the worst projects in financial terms (which would be the case if the FRR–ERR correlation were negative). Second, there is variability across sectors of such result.
Further research is needed to understand the determinants of the positive correlation between economic and financial returns that we have detected. In the framework of Equation (5) this calls for a fine grain analysis of the correlation between shadow prices and observed prices, including the issue of how externalities have been included in the analysis. In this perspective, it is interesting that the ERR compared to FRR is higher in some sectors, such as roads, ICT and productive investment. This suggests that on one side, sectors actually differ in terms of the extent of externalities and other market failures, after controlling for costs and duration, but on the other side it may be the case that CBA is still applied in different ways, according to different traditions and assumptions across sectors. Roads are a clear example, given the difference between modest-toll or no-toll policy, on one side, and the marginal social value of saving time for users on the other side. It is widely recognized that CBA is most advanced and established in the transport sector, and this allows to better account for nonmarket effects. The CBA practice in the environment sector is more recent compared with transport. For example, the health benefits of solid waste management and of water treatment may have not been estimated as an externality, beyond the WTP for the service, or its price. ICT seems to be an example of a low FRR, probably because of the high costs of broadband investment in rural or peripheral areas, and a particularly high ERR, reflecting the social value of bridging the digital divide, consistently with the EU objectives in this area.
Another issue left for further research is the difference across sectors of the time horizon of the analysis (the EC Guide suggests from a minimum of 10 years to a maximum of 30 years depending on the sector of intervention) but it should be interesting to observe project by project what time horizon was assumed for the appraisal. Intersectoral comparisons of ERRs are also affected by the legal framework that requires projects to present ERR higher than the SDR, but there is no legal requirement to maximize the ERR in the overall investment portfolio. In this perspective, further research should study clusters of projects by sector and countries to detect more in-depth the drivers of the divergence between market and shadow prices, for instance looking at samples of road projects in different countries and so on.
Finally, in this paper we have not discussed the evidence arising from the ex post evaluation of the approved projects. As mentioned some of the projects in our sample may have been withdrawn, modified or have experienced delays, even if the large majority have been eventually approved. Retrospective evaluation on small samples is available, and it would be interesting to study more systematically the relation between ex ante and ex post CBA, as suggested by Boardman, Mallery and Vining (Reference Boardman, Mallery and Vining1994), Florio (Reference Florio2014), and Kelly et al. (Reference Kelly, Laird, Costantini, Richards, Carbajo and Nellthorp2015).
Appendix. Project applications by country and sector (2007–2013)
