Introduction
The Sentence Structure sub-test of the Clinical Evaluation of Language Fundamentals (CELF-4-UK – Semel, Wiig, & Secord, Reference Semel, Wiig and Secord2006) aims to “measure the acquisition of grammatical (structural) rules at the sentence level” (CELF manual p.88). CELF scaled scores are widely used to assess the language development of native speakers of English. CELF raw scores have also been used to estimate the English proficiency of bilingual children (see e.g., Barac & Bialystok, Reference Barac and Bialystok2012; Iluz-Cohen & Armon-Lotem, Reference Iluz-Cohen and Armon-Lotem2013; Paradis, Crago, Genesee, & Rice, Reference Paradis, Crago, Genesee and Rice2003). Although originally designed for clinical practice, components of the CELF are often used to inform psycholinguistic research. The SST in particular is commonly used as a stand-alone measure of receptive syntax, both in monolinguals (e.g., Foorman, Herrera, Petscher, Mitchell, & Truckenmiller, Reference Foorman, Herrera, Petscher, Mitchell and Truckenmiller2015; Justice, Petscher, Schatschneider, & Mashburn, Reference Justice, Petscher, Schatschneider and Mashburn2011; Fricke, Bowyer-Crane, Haley, Hulme, & Snowling, Reference Fricke, Bowyer-Crane, Haley, Hulme and Snowling2013; Tager-Flusberg & Sullivan, Reference Tager-Flusberg and Sullivan1994) and in bilinguals (e.g., Bowyer-Crane, Fricke, Schaefer, Lervåg, & Hulme, Reference Bowyer-Crane, Fricke, Schaefer, Lervåg and Hulme2017; Chondrogianni & John, Reference Chondrogianni and John2018).
While it would be unrealistic to expect that any test might be able to yield a “pure measure” of the aspect of language it is designed to assess, test reliability requires that performance scores be robustly associated with properties of the language aspect targeted, and that the influence of other factors (whether linguistic or cognitive) does not interfere significantly. The impact of structural complexity on sentence comprehension is particularly challenging to assess, as it requires the use of complex visual prompts (Frizelle, Thompson, Duta, & Bishop, Reference Frizelle, Thompson, Duta and Bishop2019). This study explores the extent to which children's performance in the SST is predicted by structural complexity. As a first step, this requires clarifying how structural complexity is operationalised.
Structural complexity
The SST is presented in the manual as a subtest of language comprehension “which focuses specifically on syntax at the spoken sentence level” (manual, p.89). This subtest is designed to present the child with structures of varying degrees of complexity: “Individuals with language disorders… have problems with processing and interpreting spoken sentences when the language increases in structural complexity (sentence transformations) and in syntactic compression (idea density). Studies of these individuals suggest that they may have problems integrating the surface sentence structure and deep sentence structure…. This seems especially evident for spoken sentences that contain subordinate or relative clauses.” (CELF manual pp.88–89). No complexity ranking is provided in the manual, so expectations regarding the relative difficulty of items can only be reconstructed based on the description above. What constitutes sentence structure complexity will of course vary to an extent depending on the theoretical framework. In this study, we try to embrace different theoretical perspectives by operatonalizing structural complexity in different ways, based on (i) a rigorous interpretation of the brief description available in the manual, (ii) an index of clausal structure complexity, (iii) predictions from the language acquisition literature (focusing on the syntactic structures featured in the SST), (iv) structural complexity rankings provided by independent language acquisition experts. Next, we turn to the cognitive demands of the task, as a potential confounding factor.
Cognitive demands
In terms of task demands, each trial of the CELF SST presents the child with four pictures and requires choosing the one that matches the prompt sentence. To inform their choice, children need to evaluate differences between the four pictures in relation with the prompt sentence, and then to identify the one that best fits the prompt. Inferencing and reasoning are therefore key components of the task.
Inferencing is required in this task because the child needs to understand more than the individual words and sentences (Cain, Oakhill, Barnes, & Bryant, Reference Cain, Oakhill, Barnes and Bryant2001; Oakhill & Cain, Reference Oakhill and Cain2018). S/he needs to assemble a mental model of the situation described by each sentence, which requires going beyond the literal meaning. This might require drawing local cohesion inferences (which establish the required connections between parts of the sentence and are triggered by textual elements) and global coherence inferences (which are required to achieve an understanding of the sentence as a whole, within its context). In this task, the mental model required to interpret each sentence will be rather minimal, as there is no contextual build-up from one test item to the next. Most of the inferences required are therefore more likely to relate to the global coherence that needs to be established between the sentence and its best-matching picture. Such inferences are based on knowledge of the world (e.g., What does feeding a cat involve? From what point does the actual feeding begin?) and visual literacy (e.g., Can a verb in future tense be depicted by an image showing an action that is already under way?).Footnote 1 Local linking inferences are, however, also required in some cases: to establish an anaphoric link between a pronoun and its antecedent in the same sentence (as in (1-a)) or a particular character in the pictures (he vs. she in (1-b)).
(1)
a. The girl is wearing her new raincoat although she doesn't need it.
b. She is climbing and he is swinging.
Once the inferences have been drawn, reasoning is required to assess whether the information gathered from each picture (whether simply descriptive, or inference-based) matches the information gathered from the sentence.
It is reasonable to expect the cognitive demands of the SST to have some impact on children's performance. Indeed, all language comprehension tests are cognitively demanding to some extent. However, if the main purpose of a test is to assess language abilities, cognitive demands should be sufficiently controlled so as not to confound the language ability that the test aims to measure.
Finally, we need to consider the extent to which the SST can provide a reliable measure of bilingual children's ability to deal with structural complexity in sentence comprehension.
Language exposure and language proficiency in bilinguals
In bilinguals, language proficiency is affected by a broader range of factors than in monolinguals, including language exposure (in terms of quantity and age of onset) and cross-linguistic influence (Armon-Lotem, de Jong, & Meir, Reference Armon-Lotem, de Jong and Meir2015). Comparatively lower amounts of language exposure in bilinguals imply that they cannot be expected to perform according to monolingual age-based norms until a sufficient threshold of exposure has been reached (Cattani et al., Reference Cattani, Abbot-Smith, Farag, Krott, Arreckx, Dennis and Floccia2014). Convergence with monolingual norms has been shown to vary by linguistic sub-domain and by task difficulty (Paradis & Jia, Reference Paradis and Jia2017; Paradis, Reference Paradis2019; Schulz & Grimm, Reference Schulz and Grimm2019). In heritage speakers, it is not uncommon to observe a discrepancy between receptive and productive skills, even within the same domain (Montrul, Reference Montrul2016), but such a discrepancy is not expected to be as pronounced in the school language, as children interact in that language for many hours a day and benefit from literacy training.
The present study
The reliability of the SST depends on its ability to detect the impact of structural complexity on children's performance, in spite of the cognitive demands of the task. In other words, the impact of cognitive demands should not obscure the effect of structural complexity.
In bilingual children schooled in English, language exposure is expected to predict performance in English proficiency tests until a sufficient threshold of exposure has been reached. While some variability is expected across tests and language domains, aspects of proficiency that are still developing can be expected to be sensitive to the amount of language exposure experienced.
In light of the above, our hypotheses are as follows:
(2)
a. Structural complexity should predict performance on the CELF-SST;
b. Cognitive factors are likely to have an impact on performance in the SST, but this should not obscure the impact of structural complexity.
c. If language exposure is predictive of performance in alternative tests probing structural complexity, it should also correlate with performance in the SST.
This study is based on the secondary analysis of existing data, in order to assess whether these hypotheses are verified. In the next section, we present the population sample and the linguistic and cognitive measures of the original study which will be exploited in the current study. Then we explain how we derived the alternative indices of structural complexity and cognitive complexity of SST items. This yields four alternative rankings of item difficulty in terms of structural complexity, and two alternative rankings in terms of cognitive complexity. In the results section, we start by exploring visually whether the complexity indices correlate with children's response accuracy in the SST. For those that do, we then model the statistical significance of the effect, in light of other predictors (aiming to capture profile effects, i.e., individual differences between participants). Next, focusing on bilingual children, we compare predictors of performance across proficiency tests. In the last section, we discuss the interpretation of our results and their implications for psycholinguistic research.
Methods
The data to be discussed below was collected as part of an investigation of the relationship between executive function skills (cognitive flexibility, inhibitory control and working memory) and language experience in young bilingual children with unbalanced exposure to two languages, investigating these children's ability to make referential choices appropriate to their listener's information needs (see Serratrice & De Cat, Reference Serratrice and De Cat2020). In this section, we describe the population sample and the linguistic and cognitive measures from the original study that are relevant for our present purpose.
Participants
Our population sample includes 174 children (including 87 monolinguals) between the ages of 5 and 7 from schools in the North of England. Ethical approval was obtained from the University of Leeds (Ref. PVAR 12-007), and parental consent was obtained prior to data collection.
All the children were in English monolingual education. The bilingual children also experienced various degrees of exposure to a different language at home (henceforth the Home Language). There was a total of 28 Home Languages in our sampleFootnote 2: bilingual and monolingual children were recruited from the same schools for maximum comparability. None of the children were excluded from the study. All were reported by the school to be developing typically and not to have any known hearing deficit.
Table 1 summarizes the distribution of the two groups in gender and age. For ease of reference, we will use the term “bilinguals” to refer to children with any amount of exposure to a Home Language. The extent to which these children are bilingual was estimated more precisely on the basis of the amount of language exposure experienced.Footnote 3
Table 1. Participant distribution in gender and age (in months)

Language experience
The amount of English exposure for each child was estimated on the basis of information gathered via parental questionnaires, using a simplified version of the BiLEC (Unsworth, Reference Unsworth2013). Current exposure to English was calculated as the cumulative proportion of total English exposure experienced at school and at home,Footnote 4 divided by the total number of hours of interaction. Cumulative exposure to English was estimated from the number of months of bilingual exposure multiplied by the proportion of current exposure to (or use of) English. The cumulative measures thus correspond to the total number of months equivalent to full-time exposure to English. Cumulative exposure to English was found to be the best predictor of English proficiency in this population sample (see De Cat, Reference De Cat2020) and will therefore be used in as predictor in the current study.
Figure 1 shows that the bilingual children in our sample varied greatly in terms of their cumulative exposure to English. Some children's English exposure was close to monolingual levels (having experienced relatively little exposure to another language at home over their lifetime); some children had just started being exposed to English at the beginning of the current school year. The correlation between age and cumulative exposure to English was nonetheless significant (Pearson's product-moment correlation: t = 2.38, p = 0.02).

Figure 1. Bilingual children's cumulative exposure to English according to age. The line indicates what would be 100% English exposure (i.e., monolingual levels)
Socio-economic profiles
The schools targeted were in areas of varying degrees of affluence, so as to recruit children from as broad as possible a socio-economic spectrum. The socio-economic status of the children's families was estimated on the basis of information gathered via a parental questionnaire. Parental occupation data were scored using the reduced method of the National Statistics Socio-economic Classification (simplified NS- SEC, which is based on the Goldthorpe Scheme of sociological classification – Goldthorpe, Reference Goldthorpe1980). The distribution of scores by group is shown in Figure 2. In spite of our attempt to recruit balanced samples, the bilinguals were at a slight but significant disadvantage (as a group) compared with the monolinguals (Welch Two Sample t-test: t = 2.45, p = 0.02).

Figure 2. Pirate splot of the socio-economic occupational data by group (based on the simplified National Statistics Socio-Economic Classification reversed scores), showing means and confidence intervals.
Proficiency measures
Several measures of English language proficiency were collected to assess different aspects of language competence.
The LITMUS sentence repetition task (Marinis, Chiat, Armon-Lotem, Gibbons, & Gipps, Reference Marinis, Chiat, Armon-Lotem, Gibbons and Gipps2010; Marinis & Armon-Lotem, Reference Marinis, Armon-Lotem, Armon-Lotem, de Jong and Meir2015) was used as a measure of language processing at all levels of representation (phonological, morpho-syntactic and semantic). Although a production measure, it taps into comprehension to the extent that it is necessary for correct repetition. The test was designed for bilingual populations. It comprises 30 sentences with three levels of structural difficulty (see Appendix for a full list of items, by difficulty level). As our focus here is on structural complexity, accuracy was scored as the correct repetition of the target structure, as per the LITMUS manual.Footnote 5 Utterances containing unintelligible material were excluded (total: 208, i.e., 4% of the data).
We included four lexical-semantic tests of the Diagnostic Evaluation of Language Variation (DELV – Seymour, Roeper, & de Villiers, Reference Seymour, Roeper and de Villiers2005)Footnote 6. Three of them (the verb and preposition contrasts, real verb mapping, and novel verb mapping tasks) tap into lexical semantics. In addition, the DELV articles task taps into discourse semantics.
The Sentence Structure subtest of the CELF was chosen to provide a measure of receptive syntax.
Baseline cognitive measures
From the set of cognitive measures collected for the original study, two are relevant here to inform our appraisal of the cognitive demands of the language proficiency tasks.
Measures of short-term and working memory were obtained from the Digit Span tasks (Wechsler Intelligence Scale for Children III – Wechsler, Reference Wechsler1991). The Forward Digit Span measure was used as a proxy for children's short term memory capacity, a key component in Baddeley's (Reference Baddeley2000) Multicomponent Working Memory Model, which has been argued to represent a constraint in language processing (Boyle, Lindell, & Kidd, Reference Boyle, Lindell and Kidd2013). The Backward Digit Span measure was used as a proxy for children's working memory capacity, which has been shown to correlate with spoken sentence comprehension (Montgomery, Magimairaj, & O'Malley, Reference Montgomery, Magimairaj and O'Malley2008; Magimairaj & Montgomery, Reference Magimairaj and Montgomery2012). The raw results on the memory tests are summarized in Tables 2 and 3.
Table 2. Forward Digit Recall (number of digits correctly recalled)

Table 3. Backward Digit Recall (number of digits correctly reversed)

To assess children's cognitive flexibility, we administered the Dimensional Change Card Sort task, following the protocol described in Zelazo (Reference Zelazo2006). Performance was scored based on a pass-fail criterion on each trial block. The distribution of scores is shown in Table 4.
Table 4. Distribution of overall DCCS scores (based on block pass-fail)

Operationalization of structural complexity in the CELF SST
Semel et al. (Reference Semel, Wiig and Secord2006: 88–89) states that the SST includes items that vary in “structural complexity (sentence transformations) and in syntactic compression (idea density). Studies of these individuals suggest that they may have problems integrating the surface sentence structure and deep sentence structure…. This seems especially evident for spoken sentences that contain subordinate or relative clauses”. No grading of items is provided, however. In the following four subsections, we explore four alternative operationalizations of structural complexity that are compatible with the definition provided in the CELF manual (quoted above, in the introduction). Subsequently, the resulting structural complexity rankings of items will be presented in a comparative table (Table 8).
Movement and Embedding
In current linguistic terminology, one could interpret the CELF definition of complexity provided in the manual as follows: (i) structures that involve syntactic movement should be more complex (i.e., passives, wh-questions, relative clauses), (ii) sentences with a subordinate clause should be more complex than monoclausal sentences, and (iii) relative clauses should be particularly complex as they are embedded clauses involving syntactic movement. How to interpret “syntactic compression (idea density)” (and which test items it maps onto) is unclear.
Based on this operatonalization of structural complexity, the easiest items should be those that do not feature movement nor embedded clauses. Items featuring embedded clauses should be comparatively more difficult. Items featuring movement (such as passives or wh-questions) should also be more difficult than the ‘baseline’ items, and possibly more difficult than biclausal sentences without NP movement. The hardest items should presumably be those that combine movement and an embedded clause. This operatonalization of structural complexity is in line with the principles that define difficulty level in the LIT- MUS sentence repetition (SRep) test, in which Movement and Embedding are manipulated factorially. An important difference between the two tests is that the wh-structures in the SST include only one (adjunct) wh-question and the three relative clauses all involve subject dependencies, whereas in the SRep the wh-structures are all object dependencies (6 object questions and 5 object relative clauses). The SRep test also manipulates the presence of a by-phrase in passives (absent from Level 1 (N = 3), present at Level 2 (N = 3)); in the SST, the two passive structures both include a by-phrase.
According to this operationalization, four levels of structural complexity could be defined, as follows:
(3)
a. Level 1: sentences featuring no syntactic movement and no embedded clause (but possibly including coordinated clauses)
b. Level 2: biclausal sentences featuring an embedded clause (complement clause, direct quotation, or adverbial clause)
c. Level 3: monoclausal sentences featuring syntactic movement (i.e., a passive or a wh-quesion)
d. Level 4: biclausal sentences featuring syntactic movement and an embedded clause
Clause types
Another notable difference between the SRep and the SST is that only the latter features a broad range of clause types, as shown in Table 5. To capture this variety, we propose to use clause type as an alternative operatonalization of structural complexity, as per the order of rows in that table: a sentence with conjoined clauses is structurally more complex than a monoclausal sentence; direct quotation could be argued not to involve as much syntactic structure as embedded clause; adverbial clauses could be argued to be structurally more complex than complement clauses as their syntactic position can vary; relative clauses would be considered the most complex as they involve a syntactic dependency as well as embedding.
Table 5. Distribution of clause types in the CELF Sentence Structure test (SST) and in the LITMUS Sentence Repetition task (SRep)

Age of acquisition
On the assumption that simpler structures are acquired first, we surmise that age of acquisition by typically-developing monolinguals could be a reliable indicator of processing difficulty, and that this could have an impact on sentence comprehension. The predicted level of difficulty based on this criterion was informed by a review of the literature on the acquisition of the structures featured in the SST. As our starting point, we used the D-Level Scale of structural complexity from Covington, He, Brown, Naci, and Brown (Reference Covington, He, Brown, Naci and Brown2006) (which is itself a revision of the scale proposed by Rosenberg & Abbeduto, Reference Rosenberg and Abbeduto1987). The original scale was based on the order of acquisition in typically developing children; the revision was based on psycholinguistic evidence. The revised D-Level Scale comprises 7 levels (as shown in (4)), with the highest complexity level resulting from the combination of structures from lower levels.
(4) The revised D-Level Scale (Covington et al., Reference Covington, He, Brown, Naci and Brown2006)
Level 0:Simple sentence
Level 1:Non-finite clause as object without overt subject
Level 2:Coordinated structure
Level 3:Finite clause as object with overt subject
Level 4:Non-finite clause as object with overt subject
Level 5:Finite or non-finite adjunct clause
Level 6:Complex subject
Level 7:More than one structure from Levels 1–6
Not all the structures in (4) are instantiated in the SST, and not all the relevant features of the SST are captured by the revised D-Level Scale. To evaluate the level of difficulty of the structural features absent from the revised D-Level Scale, we reviewed the relevant acquisition literature. To obtain a sufficient number of items per level, we also condensed the scale into 4 levels.
(5) Our proposed scale of structural complexity, based on Age of Acquisition:
Level 1:Monoclausal, affirmative sentences in active voice, which might contain a PP, an auxiliary or modal verb, a direct object, or NP coordination.
Level 2:Monoclausal negative, active sentences or biclausal sentences featuring co- ordinated clauses or an infinitival complement.
Level 3:Monoclausal passive sentences, complex sentences containing an affirmative direct quotation or an affirmative adverbial clause.
Level 4:Complex sentences containing a relative clause, a negative adverbial clause, or a direct quotation featuring either a negation or a wh-question.
Level 1 represents the baseline, featuring the least complex structures (all in monoclausal sentences). Level 2 includes sentential negation, as it appears relatively early in spontaneous speech production but takes a long time to mature (Klima & Bellugi, Reference Klima, Bellugi, Lyons and Wales1966; Thornton & Tesan, Reference Thornton and Tesan2013). Coordinated clauses and infinitival complement clauses appear well before the age of 3 (Van Valin Jr, Reference Van Valin2001) and do not present comprehension difficulties in 4 year-olds (Friedmann & Costa, Reference Friedmann and Costa2010). It is not clear whether coordinated structures appear before infinitival complements (Vasilyeva, Waterfall, & Huttenlocher, Reference Vasilyeva, Waterfall and Huttenlocher2008) so we allowed them to coexist in the same level. Level 3 includes direct quotations, as these have been shown to pose comprehension difficulties for children up to the age of 4 (Hollebrandse, Reference Hollebrandse2007). It also includes adverbial clauses, as these appear later than complement and coordinated clauses (Vasilyeva et al., Reference Vasilyeva, Waterfall and Huttenlocher2008) and remain difficult to interpret for 4- to 5-year-olds (de Ruiter, Theakston, Brandt, & Lieven, Reference de Ruiter, Theakston, Brandt and Lieven2017). Children's comprehension of passive sentences is in place in typically-developing 5- to 6-year-olds (van der Lely, Reference van der Lely1996). Level 4 includes relative clauses, as these appear later than adverbial clauses in spontaneous speech (Vasilyeva et al., Reference Vasilyeva, Waterfall and Huttenlocher2008). It also includes negative complex sentences, as these combine two complexity features from lower levels.
Expert ratings
Finally, we conducted a survey of language acquisition specialists from different theoretical persuasions, to inform the creation of a 4-level structural complexity ranking of SST items. Our aim was to elicit relative complexity rankings for the following structures:
(6)
a. coordinated clauses
b. infinitival clauses
c. sentential negation
d. direct quotations
e. passives
f. relative clauses
g. adverbial clauses
We created seven sets of sentences from the SST, with each set including two of the structures of interest. Each set also included a simple baseline (featuring none of the structures in (6)) and an item featuring a relative clause, as these were presumed to be the most complex. In that way, each set comprised two structures of interest and two anchoring points (targeting low and high structural complexity respectively). Apart from (6-a) and (6-g), each structure was included in more than one set, so it could be compared with several other structures. The composition of the seven sets is summarized in Table 6. Twenty-one out of the 26 SST items were included in the survey. The 5 excluded items were all of the simplest type (as in the Baseline).
Table 6. Structures of interest featuring in the comparison sets in the expert survey on structural complexity

Each set included 6 items: two anchoring points (a simple sentence and a relative clause), and two sentences per structure of interest.Footnote 7 A brief description of the structural properties of the baseline items is given in (7). For the high-level anchoring points we used the same two sentences (alternating from one set to the next).
(7) All baseline items were monoclausal, affirmative and active sentences featuring one of the following:
a. A subject NP and a PP modifying the V (sets 2, 3, 5, 6)
b. A subject NP and an object NP (set 4)
c. A subject NP, a direct object NP and an indirect PP complement (set 7)
Five SST items were rated more than once (i.e., they were repeated across sets), as there were not enough representatives of each type of structure to allow for different items in each set.
Participants were asked to rate each sentence on a 4-point scale reflecting (low to high) structural complexity. The sentences were presented on their own, without the set of pictures associated with them in the SST. Participants were recruited via convenience sampling (through the researchers’ contacts) and advertised on the CHILDES Google Group. Thirty-one participants completed the survey.
The results are displayed by set in Figure 3. The only set in which the baseline item is rated higher than 2 is Set 1 (which compared coordinated clauses with infinitival clauses): in that case, the baseline item was itself a coordinated structure (2 NPs).

Figure 3. Results of the Structural Complexity rating survey, showing SST items’ mean ratings by structure, in each comparison set.
The average ratings for each structure were highly consistent across items and across sets: the difference between item scores was never greater than .33 of a point (on the 4-point scale), with an average difference of .2. The average ratings by structure are shown in Table 7.
Table 7. Average rating for structural complexity, by structure

Four levels of structural complexity were derived from the distribution of the average scores of the structures of interest (8).
(8) Level 1:Monoclausal, active sentences (including sentential negation); biclausal sentences featuring an infinitival clause.
Level 2:Biclausal sentences featuring coordinated clauses or a direct quotation.
Level 3:Monoclausal sentences in passive voice.
Table 8 shows the structural complexity levels for each SST item, according to the four alternative operationalizations. In spite of a certain level of incongruence between the four predictors of structural complexity (as seen in Table 8), the expectation is that there will be a negative correlation between children's accuracy scores and the SST items’ structural complexity.
Table 8. Structural complexity rankings, according to four different operatonalizations: Movement + embedding, Clausal structure, Age of acquisition, Expert ratings. The items are listed in the order in which they are presented in the test.
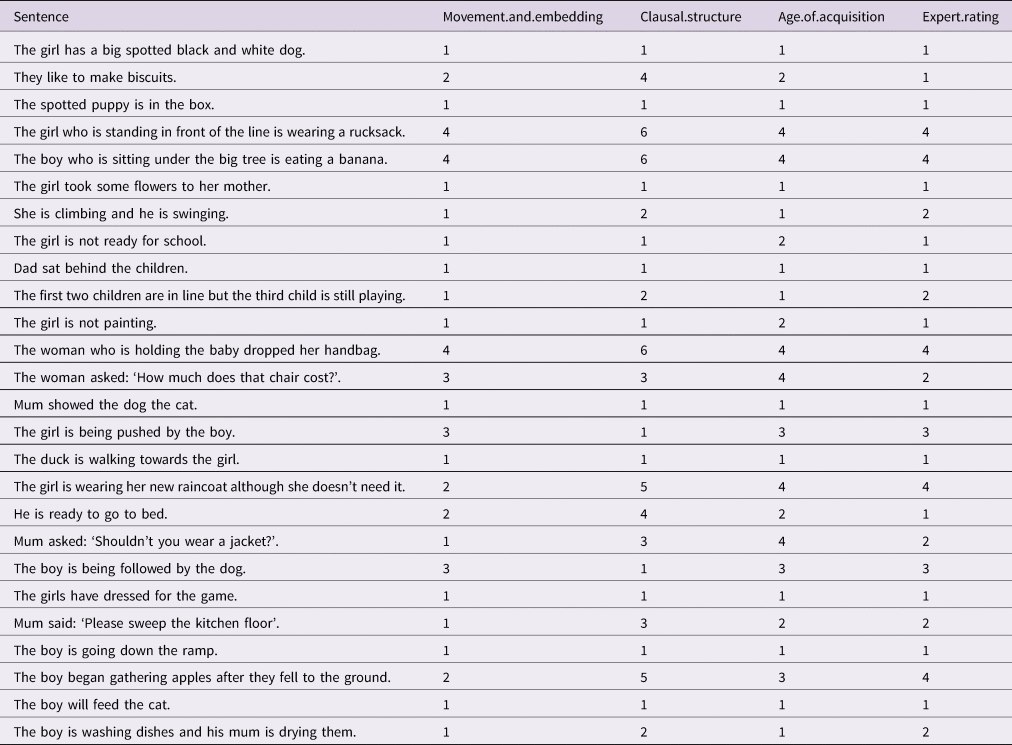
Operationalisation of the cognitive demands of the CELF SST
To allow the investigation of the impact of global inferences on children's performance in the SST, we propose three hypothetical sources of inference difficulties (9), based on our analysis of the test items in the context of their respective set of pictures. For each test item, this analysis consisted in unveiling the global inference that best captured the relationship between the sentence and a key semantic aspect manipulated across the set of pictures relevant to that item. We illustrate each of them in turn below. Note that permission to reproduce the visual prompts was not granted by Pearson, so we provide brief descriptions of the pictures instead in the figures below.
(9) Global inferences requiring to resolve
a. the relative timing of events
b. counterfactuals
c. aspectual implications
Figure 4 describes the picture prompts for items where the child needs to draw inferences that take into account the relative timing of events: in the item depicted on the left, there needs to be apples on the ground indicating that their falling had started before the harvesting; in the item depicted on the right, future tense implies that the event has not started

Figure 4. Picture descriptions for: “The boy began gathering apples after they fell to the ground.” (left) and “He will feed the cat.” (right)
Figure 5 describes the picture prompts for items where the child needs to compute counterfactuals to draw the required inference: not being ready for school implies not being at school or on the way to school; being asked if one should wear a jacket implies one isn't already wearing one.

Figure 5. Picture descriptions for “The girl is not ready for school.” (left) and “Mum asked: ‘Shouldn't you wear a jacket?’” (right)
Figure 6 describes the picture prompts for items where the child needs to take aspectual distinctions into account to draw the required inference: (1) having dressed for the match implies one is completely dressed; (2) to be going down a ramp implies that one has initiated the motion but not reached the end of the ramp.

Figure 6. Picture descriptions for “The girls have dressed for the game” (left) and “The boy is going down the ramp” (right).
For validation, 7 independent raters were asked to code the SST items using the categories in (9).
To complement the above, we also used an alternative estimate of cognitive complexity obtained by asking the 7 independent raters to assign each item with an overall score reflecting their own appraisal of the cognitive challenge posed by the item in question (on a 7-point scale). The evaluation required considering the four candidate pictures alongside the SST item, without specific instructions regarding the cognitive aspects to focus on. The intention here was to obtain a measure that would be more broadly encompassing than that based on global inferences.
The consensus ratings are summarized in Table 9. Items were assigned to an inferential category if 70% (5/7) or more of the independent raters had suggested that category. The cognitive complexity score corresponds to the average score across raters. If either of these alternative predictors of cognitive complexity is on the right track, we can expect it to be negatively correlated (or negatively associated) with children's SST accuracy scores.
Table 9. Cognitive complexity and inferential category, based on consensus from seven independent raters. The items are listed in the order in which they are presented in the test.

Results
Structural complexity analysis
Children's accuracy scores are plotted against each of the structural complexity predictors in Figure 7 (showing mean score and confidence interval for each structural complexity level, across the four operationalizations of structural complexity). The plots do not show the expected decline in performance as structural complexity increases, against our hypothesis (2-a). Given the violation of the basic assumption that performance will decline numerically as structural complexity increases, it does not make sense to perform a statistical analysis of the data.

Figure 7. CELF SST score according to four different operatonalizations of the level of structural complexity in monolinguals and bilinguals, showing mean (by structural com- plexity level) and confidence intervals for each group
A closer look at the monolinguals’ performance reveals some surprising results. Items with the most errors were among the least structurally complex. For instance, the mono- clausal and movement-free sentence in (10) (ranked lowest in structural complexity across all relative clauses (11) (which the CELF manual itself singles out as particularly complex) were at ceiling (with 99% accuracy in the monolinguals).
(10) The boy will feed the cat. (63%)
(11) The girl who is standing in front of the line is wearing a rucksack. (99%)
Performance also varied widely between structurally similar sentences, such as the ones in (12), featuring conjoined clauses and similar aspectual properties.
(12) a. The boy is washing dishes and his mum is drying them. (70%)
b. She is climbing and he is swinging. (87%)
Children's performance thus appears to be affected by something other than structural complexity in a way that was not intended in the design, contrary to our second hypothesis (2-a). In the following section, we consider the relationship between cognitive demands and performance on the task.
Figure 8 shows children's performance in the SST in relation with the two alternative predictors of cognitive complexity. The left panel shows a numerical trend for lower accuracy scores in items pertaining to the categories defined above (which we hypothesized involve inferencing difficulties). The right panel of Figure 8 shows a decrease in accuracy as cognitive complexity increases.

Figure 8. CELF SST score according to two different operatonalizations of cognitive complexity in monolinguals and bilinguals, showing mean (for each category) and confidence intervals
Cognitive complexity analysis
To evaluate the significance of our hypothetical predictor of inference complexity, we fitted a generalized linear regression model (with Participant as random effect) taking into account cognitive differences (DCCS, WM) and differences in terms of lexical semantic competence. Lexical semantic competence was measured by the DELV, which was the closest to a vocabulary test in our battery. The models did not converge if Working Memory was included together with our inference complexity variable, or if the DCCS score (of cognitive flexibility) was included alongside the DELV proficiency score as predictor variables. As this could have been due to the attested colinearity between these two variables, we residualized the DELV scores against the DCCS score. The resulting measure (DELV.resid) allowed model convergence.
The summary for the optimal model is presented in Table 10. The three categories of Inference Complexity we postulated are associated with significantly lower performance compared with the baseline category (which includes all other items), over and above the significant effect of English proficiency (indexed by DELV.resid: the residualised lexical semantics score) and cognitive flexibility.
Table 10. Fixed-effect coefficients of the optimal Generalized Linear Mixed-Effect Model predicting the likelihood of a correct response in the CELF SST based on inferencing difficulty. Random effect: Participant
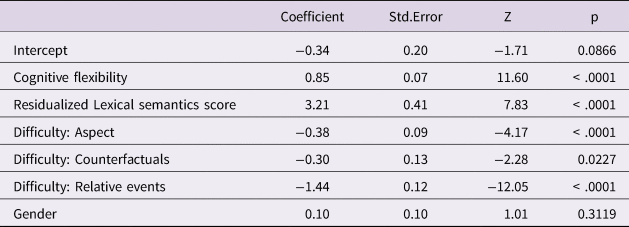
Likelihood ratio tests do not lend robust support for a difference between monolingals and bilinguals when lexical semantic competence is taken into account (Chi-sq. = 3.15, p = 0.08). There was no model convergenge if Inferencing Difficulty was allowed to interact with Bilingualism.
A similar pattern of results obtains using Cognitive Complexity as an alternative predictor (see Table 13 in the Appendix for the model summary).
We now turn to our third hypothesis (2-c), repeated here for ease of reference: “In bilingual children schooled in English, language exposure is expected to predict performance in English proficiency tests until a sufficient threshold of exposure has been reached. Some variability is expected across tests and language domains.”
Predictors of performance across language proficiency tests
We start by reporting the distribution of results across proficiency tests, comparing monolingual and bilingual performance at group level. Then we model performance in each test according to the same set of cognitive and environmental predictors.
The distribution of raw scores across proficiency tests is plotted in Figure 9. The scores express proportional accuracy for each child, with accuracy measured according to the task's protocol. From a purely distributional point of view, the four proficiency measures reveal a consistent pattern. As a group, the monolinguals performed significantly better than the bilinguals in each test: the Sentence Repetition test (Welch Two Sample t-test: t = 4.7, p <.0001), the Lexical Semantics tests (Welch Two Sample t-test: t = 6.08, p < .0001), the Discourse Semantics test (Welch Two Sample t-test: t = 5.07, p <.0001) and the CELF SST (Welch Two Sample t-test: t = 5.67, p <.0001).
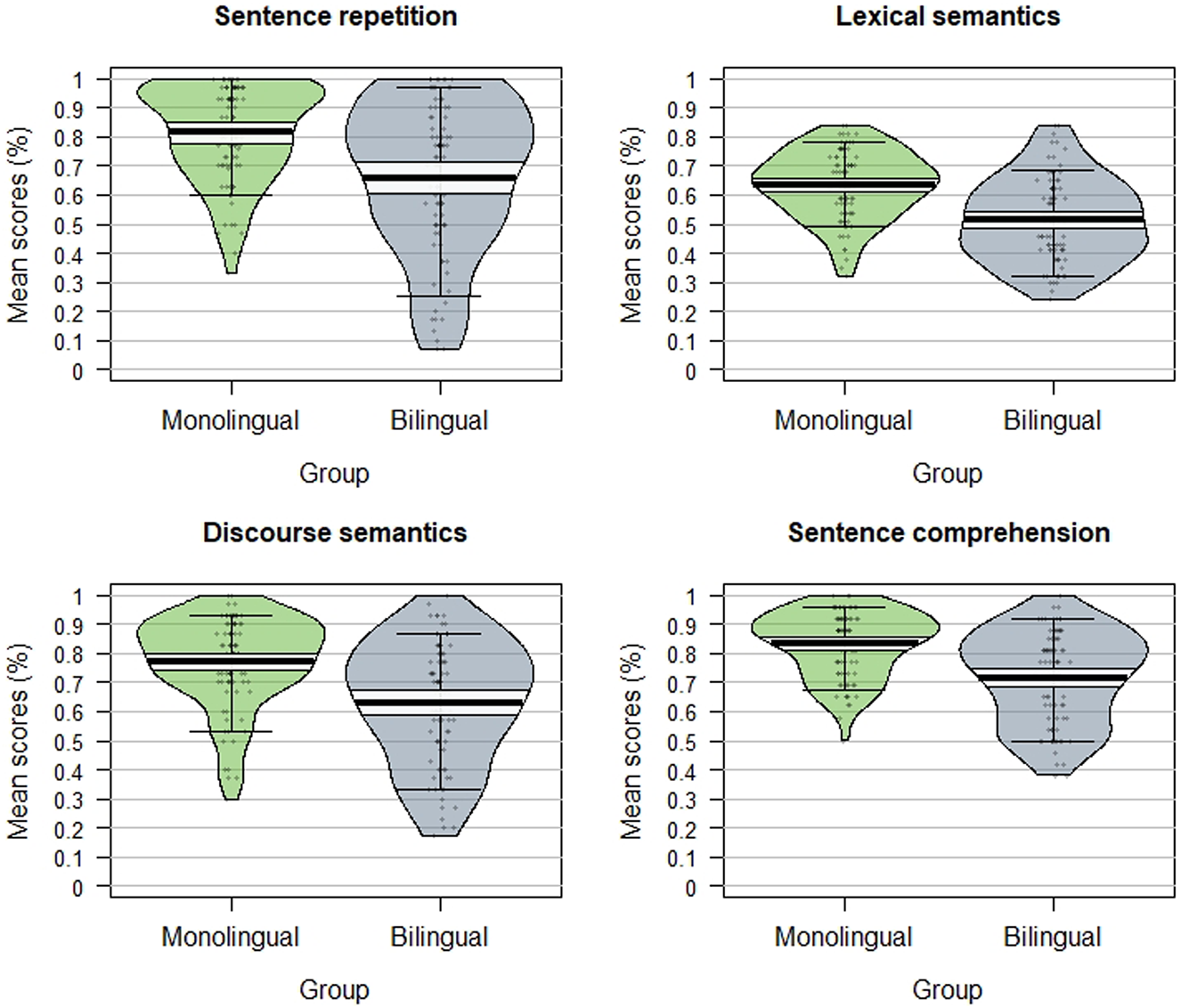
Figure 9. Pirate plots for individual mean scores across proficiency tests in monolinguals and bilinguals: target structure accuracy in the Sentence Repetition (top left), and response accuracy in the four Lexical Semantics tests (top right), the Discourse Semantics test (bot- tom left) and the CELF Sentence Structure test (bottom right). Each plot shows group mean (thick line), confidence intervals (lighter area around the mean) and 10% and 90% quantiles (whiskers).
To identify the significant predictors of proficiency as assessed by each test, we performed linear regression analyses on children's overall score in each test, using the ‘lme4’ package (version 1.1.17) in R (version 3.5.0). The models were built by adding predictors incrementally, starting from a model with Gender only (as a control variable). A predictor was retained only if it improved the fit of the model, yielding a significant reduction in AICFootnote 8 and a significant R-squared value for the model.Footnote 9 The model residuals (i.e., the observations not accounted for by the model) were checked for normality of distribution.
In all analyses, we tested whether the following variables were significant predictors: cumulative exposure to English, SES, short-term memory, working memory, cognitive flexibility, age and gender. All continuous variables were standardized so that the impact of the predictors can be compared within each model.Footnote 10
Table 11 summarizes the results of the linear regression model for each proficiency test. The predictors from the optimal models are in bold. The statistics for non-significant predictors (not bolded in the table) were computed by adding each predictor in turn to the optimal model.
Table 11. Effect of the (scaled) predictors of English proficiency scores in the bilingual children across domains. T-values represent the coefficients divided by their Standard Error. Results from the optimal models are in bold face (except for Gender, which was included as a control variable in all models).

Cumulative exposure to English is a significant predictor of proficiency in all but the CELF SST (where it does not even approach significance: p = 0.86). In all tests, one or more cognitive factors account for a significant amount of variability in the data (reflecting the cognitive demands of the task). In the CELF SST, only the cognitive factors are significant predictors of children's accuracy, i.e., Working Memory and Cognitive Flexibility. This is at odds with all the other proficiency tests in our battery, against hypothesis (2-c).
Discussion
Our item analysis of the CELF SST aimed to disentangle the effect of structural complexity from the cognitive demands of the task. Structural complexity is not clearly defined in the manual, so we proposed four alternative operationalizations, based on (i) syntactic properties, (ii) age of acquisition, (iii) clausal structure and (iv) expert ratings. None of them was found to correlate with SST accuracy scores in our study. Instead, it seems that the cognitive complexity of the task masked children's syntactic abilities. The task requires the child to compare four pictures and draw inferences from the information presented visually to choose the picture that best matches the verbal prompt. It appears the complexity associated with that process was not sufficiently controlled across items, and resulted in a confounding factor. Our attempt at identifying sources of difficulties in visual inferencing was clearly tentative, and we do not want to claim that our hypothetical categories define the source of inferencing difficulty with enough precision. For instance, the salience of key pictorial information was not taken into account in our exploration. However, our hypothetical categories of sources of inferencing difficulty did correlate significantly with SST accuracy scores – an effect not intended by design.
Unsurprisingly, these inferencing difficulty categories do overlap with structure complexity to some extent. For instance, some items requiring difficult inferences include adjunct clauses (as in (1-a) or (13)), which have been shown to be difficult to interpret by children in the age range considered here (Blything & Cain, Reference Blything and Cain2019; de Ruiter et al., Reference de Ruiter, Theakston, Brandt and Lieven2017).
(13) The boy began gathering apples after they fell to the ground.
Importantly, however, the overlap between structural and inferential complexity is only partial: the Aspect category features the structurally simplest items; and items with relative clauses (which should be among the most complex from a structural point of view) are part of the baseline in terms of inferential complexity. Indeed, in the relative clause examples, it seems possible for the child to identify the correct picture without having to compute the relative clause, and just by combining the properties of the head noun and the lexical items in the relative clause. This is likely to explain the very high scores in the items featuring that structure.
Language exposure does not predict bilingual children's performance in the SST. This is at odds with the other English proficiency tests, where environmental factors are significant determinants: both cumulative exposure to English and socio-economic status have an impact on response accuracy, in exactly the same group of children. In principle, as pointed out by a reviewer, this could be explained by the fact that the effect of language exposure varies across linguistic domains and modalities (Paradis & Jia, Reference Paradis and Jia2017; Schulz & Grimm, Reference Schulz and Grimm2019) and by the importance of other factors such as socio-economic disadvantage (see e.g., Andersson et al., Reference Andersson, Hansson, Rosqvist, Lyberg Åhlander, Sahlén and Sandgren2019). If that interpretation is on the right track, the lack of impact of language exposure on this measure of receptive syntax might be considered a potentially positive result for the assessment of bilinguals: it would be a sign that bias has been avoided in this standardized test, in spite of it having been normed with monolinguals. Two observations guard us against that interpretation, however. First, the exact same children's performance on the sentence repetition test manipulating structural complexity was very strongly predicted by their language exposure.Footnote 11 While discrepancies between receptive and productive measures are not uncommon, a difference of this magnitude is surprising. Second, if we interpret the disproportionate magnitude of the impact of cognitive predictors as an indication that the SST measures verbal reasoning more than the mastery of structural aspects of language, the lack of impact of cumulative English exposure becomes entirely unsurprising. Indeed, the cumulative amount of English experience is not negatively correlated with cognitive performance in this group of bilingual children.Footnote 12 And it is children's cognitive abilities, dissociated from their language exposure profiles, which best predict their performance in the SST. Poorer SST performance of bilingual children compared with monolinguals at group level is not explained by lower cognitive abilities (at least not working memory, cognitive flexibility or inhibition), but by their lexical competence (as indexed by their residual DELV scores, which proved to be a significant predictor).
In light of the above, we attribute the discrepancy between children's performance in the sentence repetition task (SRep) and the sentence comprehension task (SST) to the impact of cognitive confounds in the CELF SST task: the children's SST score reflects their inferential abilities more than their ability to comprehend complex structures. This in turn explains the lack of predictability of English language exposure of the bilinguals’ SST score. Notably, inferential complexity did not affect bilinguals significantly more than monolinguals, and the overall difference between the two groups became non-significant once lexical semantic scores were included as a predictor (see also Oller, Pearson, & Cobo-Lewis, Reference Oller, Pearson and Cobo-Lewis2007).
Our findings are in line with Frizelle et al. (Reference Frizelle, Thompson, Duta and Bishop2019), who demonstrate that the picture- choice method to test complex sentence comprehension under-estimates children's linguistic competence and reveals a different order of difficulty compared with a less cognitively demanding test. They conclude that “the multiple-choice picture-matching assessment method… tests skills beyond those of linguistic competence” (p. 277).
We conclude from this investigation that the CELF SST does not sufficiently dissociate the impact of structural complexity from the impact of complex reasoning on children's sentence comprehension, and should therefore not be considered a reliable independent measure of receptive syntax in psycholinguistic research.
A final caveat is required, however: for assessment of language disorders, the heavy cognitive demands in the SST, even if they mask structural complexity, might still make this a reasonable choice for assessment with bilinguals. This is because children with language disorders (particularly DLD) show cognitive deficits, especially in performance on language tasks (Tomas & Vissers, Reference Tomas and Vissers2019).
Acknowledgements
The original data were collected as part of a project funded by the Leverhulme Trust (RPG-2012-633), which is gratefully acknowledged. Thanks to the researchers who participated in the two rating surveys to inform our measures. Special thanks to the reviewers for their insightful and challenging comments, and for making us consider the clinical point of view.
The code and data are accessible via the Open Science Foundation https://osf.io/qaw3b/
Appendix
Table 12. LITMUS Sentence Repetition Items, by difficulty level

Table 13. Fixed-effect coefficients of a Generalized Linear Mixed-Effect Model predicting the likelihood of a correct response in the CELF SST based on cognitive complexity. Random effect: Participant. Statistical significance obtains from an absolute t-value of 2.
