1. Introduction
To what extent decades of family planning (FP) interventions have helped economic development? This paper answers this question in the context of the Indian economy. Since Malthus (Reference Malthus1798), population policies have been at the center of long-debated economic questions, and the recent eco-demographic modeling continues to highlight this [Bloom and Williamson (Reference Bloom and Williamson1998), Galor and Weil (Reference Galor and Weil2000), Lee et al. (Reference Lee, Mason and Miller2000), Kelley and Schmidt (Reference Kelley and Schmidt2005), Cavalcanti et al. (Reference Cavalcanti, Kocharkov and Santos2020)]. Economic support for FP produces substantial health, economic, and societal benefits for individuals, families, and nations. Investments in FP are both impactful and cost-effective [Frost et al. (Reference Frost, Sonfield, Zolna and Finer2014), Barot (Reference Barot2017), Cavalcanti et al. (Reference Cavalcanti, Kocharkov and Santos2020)]. At the same time, cutting FP aid would weaken the global commitments to achieving sustainable development goals (SDGs). There is a growing agreement that SDGs cannot be reached without universal access to FP and reproductive health care. The goal of FP program is not just stabilizing the populations of countries to optimize the balance between population and resources but also to improve reproductive, maternal and sexual health, nutrition, and reduce abortions [Rana et al. (Reference Rana, Gautam, Goli, Reja, Nanda, Datta and Verma2019)]. FP also contributes to a lower investment of resources on life course events, such as costs associated with pregnancy, miscarriage, abortion, and delivery. FP helps in economic progress through multiple channels. Thus, historically, an array of studies makes a robust economic case for FP programs [Schultz (Reference Schultz1969), Miller (Reference Miller2010), Canning and Schultz (Reference Canning and Paul Schultz2012), Kohler and Behrman (Reference Kohler and Behrman2014), Cavalcanti et al. (Reference Cavalcanti, Kocharkov and Santos2020)].
Knowing its multi-dimensional return on investment (ROI) and one of the highest cost–revenue ratio, FP programs have been recognized as the second-best “buy” for global development after liberalizing trade [Kohler and Behrman (Reference Kohler and Behrman2014)]. A consequent outcome of FP programs, planning of births, and fertility decline is also often associated with an increase in women's paid labor force participation and a reduction in additional expenditure for children's education and health care, thus allowing families to boost their income and reduce poverty [Campbell (Reference Campbell1968), Biggs et al. (Reference Biggs, Foster, Hulett and Brindis2010), Darroch and Singh (Reference Darroch and Singh2011), Sonfield et al. (Reference Sonfield, Hasstedt, Kavanaugh and Anderson2013)]. The economic returns of the FP program and fertility decline are so multiplicative that every single unit investment in the FP program confers more returns than its cost. Evidence from Bangladesh suggests that families who received FP and maternal and child health (MCH) services through the program experienced greater health benefits in addition to larger incomes, higher levels of education, and greater accumulation of wealth. The evidence from Kenya suggests that with a small government budget of 0.5% of the gross domestic product (GDP), FP interventions can yield more cost-effective benefits by improving long-term living standards than policies that subside basic education [Cavalcanti et al. (Reference Cavalcanti, Kocharkov and Santos2020)]. Thus, investment in FP can be a useful tool in curtailing expenditure incurred in the costs related to adversities that arise out of childbearing and rearing as well as in the provisions for treating MCH morbidities for the households and the state [Joshi and Schultz (Reference Joshi and Schultz2007), Schultz (Reference Schultz2009), Frost et al. (Reference Frost, Sonfield, Zolna and Finer2014)].
Despite a long history (about seven decades) of FP programs in India, assessment of the ROI of the program is virtually non-existent. This relationship between FP and economic returns has no surprising elements, but there is no systematic documentation of empirical evidence to show FP benefits to policy-makers. The recent reluctance of the Government of India to increase the investment in the FP program is a consequence of the lack of strong advocacy for its benefits [Goli et al. (Reference Goli, Moradhvaj, Singh and Srinivasan2020)]. A lack of adequate information on earned and expected returns of FP programs and consequent fertility decline has led population policy analysts to fail in strongly advocating increased investments by the states in FP programs. This is an important time to highlight the economic returns of FP programs and fertility decline in India for its refurbishment.
In this context, this study makes four key contributions: (1) With an integrated approach of using three existing Spectrum 5.27 computer simulation program modules, viz., DemProj (Demographic Projection), FamPlan (Family Planning), and RAPID (Resources for the Awareness of Population Impacts on Development), the study estimates for the first time in India the economic returns of decades of investment in FP programs and consequent fertility decline. (2) The study presents a robust framework and methodological approach in estimating various components involved in the simulation exercise in all three modules, which can be immensely useful for practical applications in future studies in this area. (3) The study provides projected scenarios of ROI on FP programs based on attainable future fertility scenarios. (4) In addition to the economic returns of fertility decline, this study estimates the educational and health infrastructure required for the next few decades in order to reap the demographic dividend. This assessment assumes importance in the context where savings as results of fertility decline leads to the investment in human capital, particularly in education and reproductive, maternal and child health and nutrition (RMNCHN), and may increase the country's capacity to grab opportunities for demographic advantage [Schultz (Reference Schultz2009), Prettner et al. (Reference Prettner, Bloom and Strulik2013), Frost et al. (Reference Frost, Sonfield, Zolna and Finer2014)]. Thus, it gives a strong policy message for continued investment regarding FP and other associated components and thereby fertility stabilization (to an optimal level), and enhance the quality of its human capital so that India can reap and also maximize its demographic dividend (i.e., economic returns as a result of demographic changes).
2. Background and related literature
The interplay between population growth and economic development has long been investigated both at global and regional scales [Lewis (Reference Lewis1954), Kuznets (Reference Kuznets1967), Campbell (Reference Campbell1968), Kelley (Reference Kelley1988), Lee et al. (Reference Lee, Arthur, Kelley, Rodgers and Srinivasan1988)]. During 1960–1990, the performance of economic growth in Asian countries, particularly the East Asian countries, was largely influenced by demographic factors [Bloom and Williamson (Reference Bloom and Williamson1998)]. The evidence suggests that FP has been one of the most successful development interventions in the past 60 years. It not only reduces the level of fertility at an aggregate level in a country but also supports the development of healthier and wealthier families in the long term [Bloom et al. (Reference Bloom, Canning and Sevilla2001), Ashraf et al. (Reference Ashraf, Weil and Wilde2013)]. Furthermore, fertility decline and resulting demographic changes influence economic growth through labor supply, accumulation of human capital, women's empowerment, and savings [Mammen and Paxson (Reference Mammen and Paxson2000), Doepke (Reference Doepke2004), Mason et al. (Reference Mason, Lee, Lee, Ito and Rose2010)]. An increase in the working-age population raises the income per capita because the productivity of the workers is constant and the proportion of youth dependants is reduced [Lee et al. (Reference Lee, Mason and Miller2000), Bloom et al. (Reference Bloom, Canning, Hu, Liu, Mahal and Yip2010, Reference Bloom, Canning, Fink and Finlay2007), Mason et al. (Reference Mason, Lee, Lee, Ito and Rose2010)]. Fertility decline is also associated with reduction in additional expenditures for children's education and health care, thus allowing families to boost their savings, which results in increased investments and income and reduced poverty [Darroch and Singh (Reference Darroch and Singh2011)].
The evidence with respect to the returns of FP programs and fertility decline has been rather weak in developing countries. A lack of evidence on the cost-effectiveness of the FP program has also led to decreasing political interest to invest in the core FP program in developing countries, especially since the late 1990s [Goli et al. (Reference Goli, Moradhvaj, Singh and Srinivasan2020)]. In particular, countries that have achieved or that are about to achieve replacement level fertility are gradually reducing funds for their FP programs, even though a large share of the population in the reproductive age group have an unmet need for FP to avoid unplanned pregnancies, abortions, and reproductive morbidities [Barot (Reference Barot2017), Rana and Goli (Reference Rana and Goli2018)]. Thus, this is high time to highlight the multi-sectoral impacts of FP programs and measure their socioeconomic and health returns in countries that are close to reaching replacement level fertility. Like the East Asian Tigers, India has a tremendous potential to reap the demographic dividend in the next few decades, provided that a favorable environment is created [Bloom et al. (Reference Bloom, Canning, Hu, Liu, Mahal and Yip2010)]. In the following section, we briefly present features of the unique story of India's FP program and the trajectory of fertility decline.
2.1 FP and fertility decline in India
Since its initiation in 1951, the official FP program in India has been considered both unique and the first of its kind in the world. Although its implementation has been criticized, concerted FP program efforts have yielded tremendous returns in the form of faster fertility decline, [James and Goli (Reference James and Goli2016)]. India has achieved a rapid fertility decline, which is unconventional compared to the pattern of fertility decline in developed and other developing countries, where replacement level fertility has typically taken much longer than what has been observed in India. The total fertility rate (TFR) in the country declined from 6 per woman in the 1950s to 2.1 per woman in 2019 [Arokiasamy and Goli (Reference Arokiasamy and Goli2012), James and Goli (Reference James and Goli2016), Office of RGI (2018)]. Thus, India and most of its states are close to reaching replacement level fertility by 2020, while its core FP expenditure is already at a record low. The contraception prevalence rate is either stalling or decreasing [James et al. (Reference James, Rajan and Goli2020)]. Studies have shown that the main window of opportunity for the country began in 2015 and will continue till 2045, but fringe opportunities will be observed until 2061 [Goli and Pandey (Reference Goli and Pandey2010), James and Goli (Reference James and Goli2016)].
Because high fertility is not an issue in most of the states in India, FP is not receiving the same attention it did in the past [James et al. (Reference James, Rajan and Goli2020)]. Budget allocations for core FP programs have been shrinking over the years [Goli et al. (Reference Goli, Moradhvaj, Singh and Srinivasan2020)]. Along with the decline in fertility, if researchers can communicate the socioeconomical and health-related positive impacts of FP to policy-makers, then the government's interest in investments in FP can be rejuvenated. In particular, both national governments and international organizations are concerned about evidence in support of returns from past and future investments back into FP program [Horton and Peterson (Reference Horton and Peterson2012), Kohler and Behrman (Reference Kohler and Behrman2014), Barot (Reference Barot2017)].
3. FP, fertility decline and economic returns: pathways
Based on an extensive literature review we present in this section, a framework showing the pathways through which investment in FP leads to multi-sectoral benefits, with a focus on economic returns. Figure A1 illustrates the relationship between fertility decline and economic outcomes through micro and macroeconomic pathways. FP is directly associated with the planning of birth regarding timing, spacing, and limiting of children, which results in the overall fertility decline and improving quality of human capital at the macro level. With the help of FP, women can plan timing for marriage and childbearing, investing more years in their education and obtain better job opportunities and higher income [Bailey et al. (Reference Bailey, Malkova and Norling2014), Bailey (Reference Bailey2006), Darroch and Singh (Reference Darroch and Singh2011), Bailey et al. (Reference Bailey, Hershbein and Miller2012), Longwe and Smits (Reference Longwe and Smits2013)]. FP directly reduces the burden of household out-of-pocket health care and other expenditure due to the planned number of children and minimizing the chances of illness. In turn, this increases household savings [Bailey (Reference Bailey2006), Canning and Schultz (Reference Canning and Paul Schultz2012), WHO (2013)]. In the long run, the educational status of children whose parents family-planned tends to be higher than that of those whose parents did not, which also led to higher income and wealth of the households [Campbell (Reference Campbell1968), Cleland et al. (Reference Cleland, Bernstein, Ezeh, Faundes, Glasier and Innis2006), Sonfield et al. (Reference Sonfield, Hasstedt, Kavanaugh and Anderson2013)].
At the macro level, as the fertility level declines, the share of the working-age population increases and the number of child dependents decreases over time. Even if productivity remains the same, the per capita income increases because of reduced child dependency [Mason et al. (Reference Mason, Lee, Lee, Ito and Rose2010)]. Due to this, expenditure on dependants in terms of welfare schemes can be saved at the national level. National savings and the savings of the working-age population are later converted into capital investment, which increases the GDP per capita [Lee et al. (Reference Lee, Mason and Miller2000), Mason et al. (Reference Mason, Lee, Lee, Ito and Rose2010)].
FP allows couples or women to have the desired number of children with healthy timing and spacing between births. Indirectly, with the adoption of FP, women can spend more years in their own education, obtaining better opportunities in the labor market and save resources by spending less on children. In turn, the economic status of the household improves over time. On the other hand, the government may save additional resources on welfare schemes due to the reduced number of children, which increases national savings. Due to declining fertility over a longer time, the age structure of the population changes, thus the working-age population rises. This increase in the working-age population has the potential to contribute to the GDP in a country [Bloom et al. (Reference Bloom, Canning, Hu, Liu, Mahal and Yip2010, Reference Bloom, Canning, Fink and Finlay2007)]. In the following subsections, we provide a detailed description of the key pathways that create a favorable environment to reap the demographic dividend from the demographic transition fostered by FP.
3.1 Women's education and employment
Previous studies suggest that with FP, women can improve their level of education and reduce the risk of unintentional pregnancy, thus reducing the number of births and facilitating a more favorable timing of childbearing, which further contributes to greater participation of women in the workforce and higher-quality jobs as their age at marriage tends to go up [Birdsall and Chester (Reference Birdsall and Chester1987), Darroch and Singh (Reference Darroch and Singh2011), Longwe and Smits (Reference Longwe and Smits2013)]. Spending more hours on their work further reduces fertility, which results in reduced child dependency and increased earnings, savings, and assets among women [Bailey (Reference Bailey2006), Canning and Schultz (Reference Canning and Paul Schultz2012), WHO (2013)]. According to Goldin and Katz (Reference Goldin and Katz2000, Reference Goldin and Katz2002), use of oral pills among unmarried women enables them to progress in their career, avoiding childbearing before marriage, and decreasing the incidence of shotgun marriages. Edlund and Machado (Reference Edlund and Machado2009) found evidence that delaying pregnancy within marriage increases educational and professional advancement among women. It has also been found that the gender gap in income decreases with increasing contraceptive use through the empowerment of women [Bailey (Reference Bailey2012), Bailey et al. (Reference Bailey, Hershbein and Miller2012)].
3.2 Child education, employment, and economic status
Time-series and longitudinal studies at both macro and micro levels have documented that short birth spacing and large family size are linked with lower parental investment in their children. This, consequently, influences the children's mental and behavioral development and educational achievement. Whereas, FP increases children's schooling, promotes their college completion and, finally, their participation in the labor force. As a result, parental adoption of FP for a longer time reduces poverty and enables better use of their income for their children [Cleland et al. (Reference Cleland, Bernstein, Ezeh, Faundes, Glasier and Innis2006), Canning and Schultz (Reference Canning and Paul Schultz2012), Sonfield et al. (Reference Sonfield, Hasstedt, Kavanaugh and Anderson2013)].
3.3 Economic savings
FP benefits are not restricted at the individual or the household level; they are evident even at the macro level. Early evidence from eco-demographic studies reveals that the ratio between cost and benefit of FP programs is 1:26. Thus, a parent can save the cost of unwanted childcare and other expenses [Campbell (Reference Campbell1968)]. Similarly, major public expenditure can be saved by reducing expenditure on MCH care and other welfare and social services. Some estimates suggest that ~$1.5 billion per year can be saved if the current unmet need for FP in the United States is addressed [Biggs et al. (Reference Biggs, Foster, Hulett and Brindis2010), Darroch and Singh (Reference Darroch and Singh2011)]. Another estimate suggests that the benefit of every dollar spent on universal access to FP and sexual and reproductive health services is annually $120 [Kohler and Behrman (Reference Kohler and Behrman2014)].
3.4 The demographic window of opportunity
Change in the age structure of the population has the potential to contribute to the economy of a country in a demographic transition. The decline in fertility level results in a decrease in child dependency and increase in the working-age population. As said earlier, this transition phase is considered the “demographic window of opportunity” for a country. The decline in fertility also decreases the dependency ratio, which, in turn, increases the per capita income and savings for an improved lifestyle. The economic bonus of the demographic change is known as the “first demographic dividend”. When the cohort in the working-age group reaches the older-age group with more capital, the rising capital–labor ratio is maintained through capital investment, which is considered as the “second demographic dividend” [Mason et al. (Reference Mason, Lee, Lee, Ito and Rose2010)].
4. Previous approaches for estimating the ROI of FP programs
Population growth and economic development are unfinished, long-debated issues in human history. In the previous section, our conceptual framework showed that FP has direct and indirect effects on economic growth at the individual, household, and national levels through savings, better education, and increasing economic engagement of women. In the macroeconomic model, the role of rising fertility rates and increasing population growth in the depletion of the earth's physical resources has long been a concern [Malthus (Reference Malthus1798)]. Previous studies have assessed the relationship between fertility decline or population growth and economic development from the perspectives of macroeconomic, microeconomic, and simulation approaches. Among the macroeconomic studies that have contributed to the literature on the relationship between population growth and economic development, Bloom and Williamson (Reference Bloom and Williamson1998), Livi-Bacci and De Santis (Reference Livi-Bacci and De Santis1998), Lucas (Reference Lucas, Rosenzweig and Stark1997), Lee et al. (Reference Lee, Arthur, Kelley, Rodgers and Srinivasan1988), Lewis (Reference Lewis1954), Kuznets (Reference Kuznets1967), Leff (Reference Leff1969), and Malthus (Reference Malthus1798) have made novel contributions. The pioneering study by Kuznets (Reference Kuznets1967) showed a positive association between population growth and income growth by a broad grouping of countries around the world. Contrary to this view, Kelley (Reference Kelley1988) suggested a spurious relationship between population growth and growth in income per capita. Using cross-country analysis, he reported that there is no clear unidirectional relationship between the growth of the population and the growth in the rate of savings. Furthermore, he argued that evidence for a negative association is rather weak. The attention of scholars turned more towards examining this relationship after growth regression models were proposed by Lucas (Reference Lucas2000), Mankiw et al. (Reference Mankiw, Romer and Weil1992), Barro (Reference Barro1991), and Romer (Reference Romer1990). The classical growth regression models addressed population growth, labor force growth or changes in the dependency ratios and growth of income per capita or economic growth (savings rates, etc.) with the former as right-hand side variables and the latter as left-hand side variables. As per the study by Kelley and Schmidt (Reference Kelley and Schmidt2005), the regression of income growth rate on population growth rate (Solow effect) and growth rate of the working-age population (dependency effect) revealed that the combined impact of the parameters of demographic change (i.e., change in fertility and life expectancy and age-structural transition) on per capita output growth accounted for 20% of the total economic growth. Similarly, using a panel of cross-country regression models, Bloom and Canning (Reference Bloom and Canning2004) reported a positive and significant coefficient by regressing income per capita growth on working-age population growth. Here, we could say that FP and fertility decline contribute to a decrease in population growth, which decreases child dependency ratios and increases the working-age population and offers a window of opportunity to reap the demographic dividend.
The methodological issues encountered in macro models require extra caution in the interpreting results. Hence, for a more policy-inclined view, one needs to capture the interrelationship at the household level to affect the outreach of the benefits of a particular program and policy intervention. Some pioneering studies addressing the long-term effects of contraception in randomized control trials designs in Matlab, Bangladesh, have found that reduction in fertility levels (preventing childbearing through contraception) improves the health, economic earnings, and household assets of women as well as their existing children [Joshi and Schultz (Reference Joshi and Schultz2007), Schultz (Reference Schultz2009)]. Similarly, a study evaluating the Profamilia FP program in Colombia documented greater postponement of marriage and first birth, lower fertility, and higher education in women in households that followed the program than in women in control households [Miller (Reference Miller2010)]. Rosenzweig and Zhang (Reference Rosenzweig and Zhang2009) found a negative relationship between fertility level and educational attainment at the individual level. In contrast, studies have also shown that there is no effect of fertility (number of children) on the health and education of children while controlling for possible alterations arising because of twins and mixed-sex compositions of the children in a household [Angrist et al. (Reference Angrist, Lavy and Schlosser2010)].
The third and final set of studies that examined the implication of FP and fertility decline on economic growth used a simulation approach. Coale and Hoover (Reference Coale and Hoover1958), who are considered the intellectual ancestors of modern eco-demographic models, analyzed the effect of fertility on income per capita in India. While reporting the significance of their study, they made three alternative population projections (high, medium, and low) and projected per capita income in all scenarios, finding a strong negative correlation between change in fertility level and change in income per capita. Enke (Reference Enke1971) proposed the more modern production model by considering cases of high fertility (constant gross reproduction rate) and low fertility (fall in the gross reproduction rate) and suggested an adverse impact of high population growth on the income per capita. The study by Mason et al. (Reference Mason, Lee, Lee, Ito and Rose2010) has shown a drop in fertility level with increasing years of schooling per child. Finally, in the more dynamic simulation model proposed by Ashraf et al. (Reference Ashraf, Weil and Wilde2013), the authors considered different paths of population growth (i.e., physical capital accumulation, effective labor, returns on schooling and experience, childcare effects on labor supply) and economic outcomes were estimated. A recent study included more channels such as child health outcomes, savings due to changes in age structure and FP programs in estimating the economic returns of fertility decline [Karra et al. (Reference Karra, Canning and Wilde2017)].
5. Data inputs
The data inputs for the select indicators have been collected and compiled from different sources for the period 1991–2016 and the details are presented in section 6. The major data sources used for analyses are (i) successive rounds of National Family Health Survey (NFHS) [IIPS (1995), IIPS and ORC Macro (2000), IIPS and Macro International (2007), IIPS and ICF (2017)], (ii) Sample Registration System [Office of RGI (2016)], (iii) Census of India [Office of RGI and Commissioner (1991), Office of RGI and Commissioner (2001), Office of RGI and Commissioner (2011)], (iv) Information from Government of India reports [GOI (2016), NHSRC (2016), World Bank (2016), NHSRC (2019), RBI (2020)], and (v) other published papers [Singh et al. (Reference Singh, Hussain, Shekhar, Acharya, Moore and Stillman2018)]. Some of the input indicators were interpolated wherever they are not available for any years from this period. Standard assumptions have been made for the projection of estimates up to 2061. The DemProj, RAPID, and FamPlan modules of the Spectrum Suite (5.72) computer simulation program were used to estimate and project the economic returns to progress in FP and fertility decline. For the projection period (2016–2061), four different scenarios of fertility decline were assumed following the Gompertz curve: TFR 2.1 (replacement level), TFR 1.8 (high variant) TFR 1.6 (medium variant), and TFR 1.4 (low variant). In 2061, the fertility level is assumed to be one of these four. Thus, separate estimates have been made for the different variants of progress in fertility decline. We explain the simulation procedure and data input assumptions in detail for the indicators considered in each module below.
6. The model estimations and assumptions
6.1 Demproj module
6.1.1 Population projections
Population projection is a scientific tool that integrates various components of change to project future population and composition. For the projection of population of India from 1991 to 2061, the DemProj modules of the Spectrum projection function have been used Stover and Kirmeyer (Reference Stover and S.2008). The population of 1991 has been used as the base year for the projection. This year was chosen for two important reasons. First, it allows evaluating the validity and suitability of the model by comparing the projected and actual populations for the period 1991–2011. Second, after the International Conference on Population and Development (ICPD) 1994, the FP agenda shifted from a target-based approach to the rights-based approach. The agenda was expanded to encompass the welfare of women rather than focusing solely on FP targets. The ICPD has brought a multitude of changes in reproductive health outcomes, which have an undeniable impact on the future growth of the population. Thus, 1991 can be considered the foundation of both demographic and health perspectives in India as well. We discuss the compilation of input indicators for the base year.
6.1.2 Age–sex distribution of population
The age–sex distribution of the population for the base year was compiled from the 1991 census of India. For a high-quality base year input, the age–sex-disaggregated data obtained for the base year has been adjusted for non-sampling errors and those who did not state their correct age have been distributed proportionately across other age groups.
6.1.3 Fertility levels
The trajectory of fertility in India is the most contested component in its population projections. Earlier projections were concerned with the attainment of replacement level fertility (TFR = 2.1). However, considering the unexpected fertility decline in the recent past across states, the goalpost (the end year) assumptions in the projections have been shifted to fertility levels lower than the replacement level of 2.1. Different fertility assumptions made by different projections listed in Table 1 indicate that the TFR projection for the end year is much lower than 2.1.
Table 1. Fertility goalpost assumptions in previous projections exercise for India

In this study, we assume four scenarios for fertility decline in India. The first scenario is the stabilization of the TFR at replacement level fertility (TFR = 2.1) by 2061, whereas the second scenario assumes that the TFR will be 1.8 children per woman by 2061, which is ideally followed by many recent projections including United Nations Population Prospects for India case [United Nation (2015)]. However, the recent estimates of trends in fertility and its trajectory under different socioeconomic inter-sectionality in the country suggest that TFR observed is around 1.6–1.4 for a majority of the intersectional categories [Goli et al. (Reference Goli, Rana and Mishra2018)]. Thus, we have assumed the lowest possible attainable TFR to be 1.4 births per woman by 2061 if TFR across all socioeconomic groups converges to the current lower-fertility clubs Figure 1.
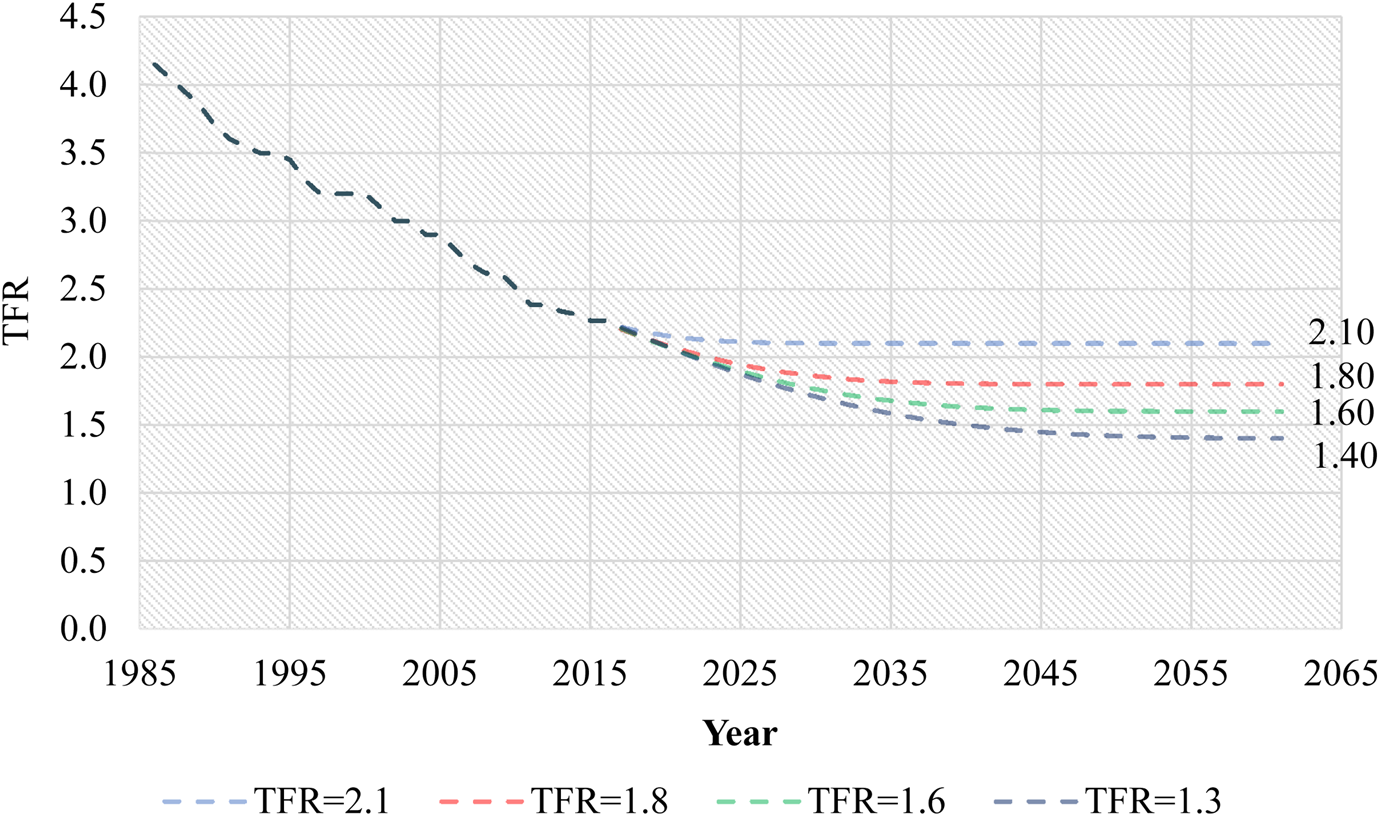
Figure 1. The trajectory of fertility (TFR) under different assumptions.
Source: Authors' estimation based on TFR estimates for 1986–2016 from Office of RGI, Government of India.
Once the goalpost (2061) scenarios of TFR have been determined under different assumptions, the Brass Gompertz model has been exercised to extrapolate the fertility trajectory in India for the different fertility scenarios.
6.1.4 Age distribution of fertility
With significant improvement in FP and consequent fertility decline in the country, a definite shift in the age distribution for births is also observed. We estimate time-series data on age-specific fertility rate at 5 years interval for the women in age-group 15–49 years from 1996 to 2015. We observe an increase in the mean age at birth and a decline in the standard deviation of age at birth [Office of RGI (2007, 2018)]. A large proportion of births was concentrated in the age group of 20–34 years. However, the proportion of births by women younger than 19 and older than 35 has significantly reduced. This suggests that FP has helped in cutting down adolescent pregnancies. DemProj routinely optimizes the distribution of the births as per the imputed assumption of the TFR. Thus, under the given fertility scenarios, the distribution of births post optimization process is mainly concentrated in the age group 24–34 years, whereas a significant decline in births is observed in women aged <20 and >40 years.
6.1.5 Sex ratio at birth (SRB)
The recent trends in the SRB have had conspicuous fluctuations at national and sub-national levels in India. When compared with past trends across major states, the deviation in SRB narrows as the average levels improves. Surprisingly, South Indian states that had secular trends in SRB worsened in the recent five-year period [IIPS and ICF (2017)]. Given the distortion in SRB estimates in India and the discordance between survey and census estimates, it is immensely difficult to assume future scenarios for SRB. Many studies assumed that the SRB estimate would become secular over time. We also believe that with a rise in the level of urbanization, in the affluence of states and improvement in the status of women, SRB will improve and stabilize at 105 boys per 100 girls at birth by 2061, which lies within the recommended global average [United Nation (2019)].
6.1.6 Life expectancy at birth (LEB)
LEB in India has secularly increased over the past years. A significant level of disparity in LEB has been observed across the states of India. Affluent states such as Kerala, Karnataka, and Tamil Nadu have a considerably higher LEB than less-developed states such as Assam, Bihar, and Madhya Pradesh. However, with the progress in the socioeconomic profile of states in India, noteworthy improvements in the LEB can be observed in recent years. It has also been observed that the life expectancy of females has risen more sharply than that of males from 1981 to 2015, creating wider gender differences in mortality. The past trends of LEB have shown an increase of nearly 5 years per decade for women, while for males, it has increased by approximately 3 years per decade [Office of RGI (2018)]. Thus, considering the same trends, the extrapolated results indicate that the LEB for males would be ~81 years by 2061, while for females, it would be about 87 years. The obtained values of LEB are close to the maximum average attainable LEB in developed countries. For instance, the LEB of India in 2061 will be equivalent to LEB of Japan (a country with the highest average LEB (84 years) in the world) in 2016 [United Nation (2017)].
6.1.7 Model life tables
We have adopted the South Asian model life table for population projection. Previous literature suggests that mortality estimates using the South Asian model has been more consistent in comparison with actual data [Stover et al. (Reference Stover, Heaton and Ross2006)].
6.1.8 International migration
Migration is the third-most important component of population projection and is mostly assumed to be constant over time. The report of the expert committee on population projection based on the 2011 census data has assumed constant net migration as per the 1991–2001 census data in the absence of the 2011 census migration tables. The same committee assumed no significant role of international migration on the projected population totals [Office of RGI and Census Commissioner (2011)]. Keeping this in view, we have assumed that the migration will remain the same throughout the period at all-India level, thus will not affect our projected population totals.
5.1.9 Single-year age estimates of population, fertility and mortality
Spectrum simulation procedure also requires inputs of single-year age estimates of population, fertility, and mortality rates and thus necessitating a transformation of estimates from five-year age cohort to single-year age. The base year population in the five-year age group has been converted into single-year age population using Beers' (Reference Beers1945) methodology. Following the same methodology, age-specific mortality rates, age-specific fertility rates and age-specific net migration rate have been split into single-year age distribution. For the subsequent years, the population is projected using the cohort component method as follows:

where P a,s,t, j is the population of particular sex (s) at age a, at time t under the jth scenario. Miga,s,t,j refers to the migrant population of particular sex (s) at age a, at time t under the jth scenario. The Deatha,s,t−1,t,j indicates the number of persons of particular sex (s) died at age a, between mid-year to mid-year under the jth scenario, which is given as follows:

where Survia−1,a,s,t,j indicates age-specific survival rates between age a−1 and a for the person of particular sex (s = male or female). Once the male and female population between two time periods has been estimated, it becomes important to estimate the number of births that occurred between two mid-years. The mathematical formula for the estimation of birth is given as follows:

where Fp a,t,j is the female population at age a and time t and ASFRa,t,j is age-specific fertility rate corresponding to age a at time t in the jth scenario.
On multiplying the number of births by the corresponding SRB, the number of male and female births were estimated. The births were then multiplied by their corresponding survival functions and are placed in the initial age group 0–1 year. So, the population in the age group 0–1 year is given as follows:

The population in the age zero is replaced and projections for the subsequent years are carried out using the same iterative procedure till the final projection year is reached.
6.2 FamPlan module
This module is very useful in the sense that it captures various dimensions of growth in a country. These areas include fertility and use of different FP methods, the impact of FP, demographic events, fertility-related risks, mortality rates, post-abortion care, and associated costs and revenues. The spectrum module DemProj is integrated with other modules such as FamPlan and RAPID [Stine and Schmitz (Reference Stine and Schmitz2013)]. Unlike other modules, FamPlan is an impact assessment module linked with DemProj that undertakes assumptions for reaching the FP and fertility goals in a country. Specifically, it integrates the age–sex disaggregated population obtained from DemProj and applies it to two data types. First, the proximate determinant of fertility and second, program characteristics such as methods and source mix of modern contraception. The approach here is based on achieving desired fertility rates. The estimation in FamPlan is based on the famous proximate determinant of fertility framework [Bongaarts (Reference Bongaarts1978), Bongaarts and Stover (Reference Bongaarts and Stover1986), Bongaarts and Potter (Reference Bongaarts and Potter2013)]. The desired fertility levels and corresponding trajectories are provided in the DemProj and FamPlan modules (as explained in the previous section on DemProj). The goal for each year for identified jth scenario (j = 1 for TFR 2.1; j = 2, TFR = 1.8; j = 3, TFR = 1.6; j = 4, TFR = 1.4) till the final year is estimated as follows:

where TFRg,j is the value of TFR at the end years (2061) in jth scenario and percentage reduction refers to the gap between actual TFR and TFR at goalpost of 2061. So, once the TFR at year t is estimated, the corresponding prevalence of contraception is computed from the proximate determinant frameworks as follows:

where C prev,t = contraceptive prevalence at time t; C m,t index of marriage; C i,t index of insusceptibility; C a,t index of abortion; C s,t index of sterility; TF is total fecundity, and C eff,t the average effectiveness of all contraceptive methods at time t.
6.2.1 Proximate determinants of fertility
The proximate determinants of fertility include a set of behavioral and biological variables that have a direct impact on the fertility outcome. These include the percentages of women who are married or in a union, postpartum insusceptibility, total abortion rate, and sterility. From the base year of the projection to 2016, the values for the percentage of women who are married or in a union and the duration of postpartum insusceptibility have been estimated from the NFHS rounds and the values between the surveys have been interpolated. The percentage of women who will be married or in a union in 2061 is assumed to be lower than the percentage in 2016 because of the increasing median age at marriage. Similarly, postpartum insusceptibility is assumed to be lower than the estimate in 2016 because of a decreasing trend in the duration of breastfeeding practices. The total abortion rate and sterility remain the same as those estimated for India throughout the projection period [Stover et al. (Reference Stover, Heaton and Ross2006)] because these values are less likely to change over time.
6.2.2 Contraceptive method mix and source mix
Contraceptive method mix refers to the percentage of contraceptive users by a method to the total number of users. Thus, the sum of the contraceptive methods is 100%. The method mix has been calculated from contraceptive users as reported in NFHS [IIPS (1995), IIPS and ORC Macro (2000), IIPS and Macro International (2007), IIPS and ICF (2017)]. For the base year, the estimates from the NFHS-1 have been used. During the inter-survey period, the values are interpolated using the linear interpolation method. For the year 2061, a method-mix of contraceptive methods has been taken from the developed countries. Thus, the percentage of limiting methods, particularly female sterilization, has been reduced. Similarly, the spacing methods have been increased because the government of India has introduced a new spacing method, such as injectables into the method-mix basket. Values between 2016 and 2061 were filled with the interpolated estimates obtained for all contraceptive methods separately and the corresponding method mix and users at each method. It can be expressed as follows:

where CPa,m,t is the contraceptive prevalence for a particular method m among users of age a at time t; Method Mixa,m,t refers to the share of all users using specific method m, at time t; and CPa,t contraceptive prevalence among users aged a at period t.
Also, the numbers of users at each method are estimated by multiplying method-specific prevalence CPa,m,t with married women of reproductive age group.

where USERs a,m,t refers to users of a particular method m among users of age a at time t and MWARa,t married women of reproductive age a at time t.
The source mix is the percentage of contraceptive users who receive their services from different sources. The sum of the source mix is 100%. For this study, the sources were defined as public, private and NGOs. The information from 1991 to 2016 on the source mix was collected and estimated from the NFHS reports. For the information during the inter-projection period, the values have been interpolated: various sources “p” for contraceptive method “m” is computed as follows:

where USERs a,m,s,t,j is number of methods users of reproductive age a at time t relying upon source s for specific contraceptive method m under scenario j; Source Mixm,p,t,j refers to the share of users receiving specific contraceptive method m from sources s at time t under jth scenario. For the final year of the projection (2061), the share of public sources has been reduced, and the share of private sectors and NGOs has been increased based on the current ones in developed countries. Thus, over the year, expenditure on FP may be reduced in the public sector and the share may be increased in both the private sector and NGOs in the future. Thus, the source of contraceptive methods in the final year of the projection may be equivalent to that of developed countries.
6.2.3 Child survival
The inputs for child survival are needed for the base year, i.e., 1991. The indicators for child survival include the percentage of births with any risk involved, the infant mortality rate (IMR) and under-five mortality in the survey years, the relation of risky births to contraceptive use, the relation of IMR to risky births and the relation of under-five mortality to risky births. The IMR and under-five mortality rates have been collected from the sample registration system (SRS) [Office of RGI (2007 and 2018)]. The percentage of risky births has been estimated from the first round of the NFHS. All coefficients for the relationships are assumed to be the default [Stover et al. (Reference Stover, Heaton and Ross2006)].
6.2.4 Cost of services and consultation fees
The cost of services on contraceptive methods was taken from the Ministry of Health and Family Welfare (MoHFW) reports for the year 1991. The “regression” option of the FamPlan module allows for the projection of future costs per user assuming a certain relationship [Stover et al., (Reference Stover, Heaton and Ross2006)]. It assumes that the cost of services will decrease with increased number of users or acceptors for a particular method [Stover et al. (Reference Stover, Heaton and Ross2006)]. The consultation fees for the contraceptive methods are varied across the sources of supply. The public sector supply is free of cost. The fees for NGOs are assumed to be half of those of the private sector because NGOs are generally non-profit organizations while health institutions are for-profit. The consultation fees for male and female sterilization may be equivalent to those for a C-section delivery, which is taken from a published paper for the year 2014 [Goli et al. (Reference Goli, Rammohan and Pradhan2016)]. The fees for other modern methods of contraception may be equivalent to the average of the charges for normal medical visits for treatment.
6.2.5 Method attributes
Method attributes refer to the durability of each contraceptive method. For the limiting methods, the average age of female and male sterilization is considered to be 26 years throughout the projection period. For long-acting reversible contraceptive methods, the average durations for Implanon (implant), copper T IUD and LNG-IUD are 2 years 5 months, 4 years 6 months and 3 years 3 months, respectively. The method attributes for the short-term contraceptive methods are defined as the number of units required for one-year protection of a couple. The number of units for condoms, daily pills (one cycle), injectables (Depo-Provera for 3 months) and lactational amenorrhea method (LAM) are 120, 4, 15 and 0.3, respectively.
6.2.6 Lactational amenorrhea method
LAM is a one-time input for the base year (one-time entry). The percentage of women who use LAM by months has been estimated from the first round of the NFHS (1992–1993).
6.2.7 The effectiveness of contraceptive methods, impact rates and miscarriage rate
The information on the effectiveness of contraceptive methods has been taken as standard rates proposed by Stover et al. (Reference Stover, Heaton and Ross2006) based on observation of the data from global experiences. All indicators of impact rates (one-time entry) have been either estimated from the first round of the NFHS or taken from publications/reports [Singh et al. (Reference Singh, Hussain, Shekhar, Acharya, Moore and Stillman2018)]. The miscarriage rate varies between 10% and 20% across the globe [Stover et al. (Reference Stover, Heaton and Ross2006)]. For India, at the base year (one-time entry), this rate is considered to be 15% (0.15).
6.2.8 Post-abortion care
The percentage of legal abortions has been calculated from the sex ratio of each decennial census, assuming that the imbalanced SRB is the result of illegal abortions. The percentage of legal and illegal abortions that require treatment is taken from published reports [Cohen (Reference Cohen2009), Singh et al. (Reference Singh, Hussain, Shekhar, Acharya, Moore and Stillman2018)]. The percentage of maternal deaths due to abortion (5.9%) is taken from a study by Say et al. (Reference Say, Chou, Gemmill, Tunçalp, Moller, Daniels, Metin Gülmezoglu, Temmerman and Alkema2014). The annual expenditure for post-abortion care at the base year of projection (single entry) is assumed to be the same as the cost of postnatal care [Goli et al. (Reference Goli, Rammohan and Pradhan2016)]. The cost per abortion complications treated is assumed to be the same as the delivery cost [Goli et al. (Reference Goli, Rammohan and Pradhan2016)]. The cost of annual FP counseling or service per case has been assumed to be the same as the fees for medical consultation [Goli et al. (Reference Goli, Rammohan and Pradhan2016)]. The cost of abortion complications treated and FP counseling or service fees are adjusted with inflation for the following years and deflated for the previous years during the projection period.
6.2.9 Distribution of fertility-related risk
The distribution of fertility-related risk is represented by the percentage of women at different age groups and birth order and by the percentage of children at groups of birth interval. These percentages have been estimated from the first round of the NFHS (1992–1993).
6.3 RAPID module
The RAPID module of spectrum is an organized tool for estimating the workforce and economic outcomes aiming to meet the desired social and economic goals in a country. At the same time, it also provides the socioeconomic requisites to enable achieving various country-specific targets within the stipulated timeframe. In the RAPID module, we use the projected age–sex-wise population totals and other demographic parameters from the DemProj for different sectors such as economy, health, education agriculture and urbanization. We discuss two major aspects (education and health) of the RAPID module in detail under the four projection scenarios.
6.3.1 Economic input indicators
For the RAPID module, we include an array of economic input indicators and those also recognized by previous eco-demographic studies as critical factors of determining and predicting a country's economy [Coale and Hoover (Reference Coale and Hoover1958), Barro (Reference Barro1991), Lee et al. (Reference Lee, Mason and Miller2000), Bloom et al. (Reference Bloom, Canning and Sevilla2001), Kelley and Schmidt (Reference Kelley and Schmidt2005)]. Our model includes the labor force participation rate (LFPR) for boys 10–14 years old, LFPR for men 15–64 years old, LFPR for girls 10–14 years old, LFPR for women 15–64 years old, GDP at the base year (one-time entry) in Indian rupees and percentage annual growth rate in GDP. The LFPRs for both boys and girls 10–14 years old are obtained from different census rounds, with 1991 as the base year; the estimates of the LFPR obtained from the census rounds have been duplicated for the inter-census period. Among both adolescent children 10–14 years old, we assume LFPR to be 0.01% in 2061 considering child labor may reduce to nearly zero under best scenario as a result of the accelerated effort to eradicate child labor in India. Among the men 15–64 years old, under the best scenario, the LFPR is assumed to be 86% in 2061 despite the current trend shows a decline. This is also equivalent to the LFPR level in developed countries [World Bank (2016)]. The highest LFPR for females worldwide is observed in developing countries such as Tanzania and Zimbabwe, where it has reached ~79%; for the developed countries, the LFPR is ~65% [World Bank (2016)]. Since we always use developed nations as a benchmark, we assume that India will reach an LFPR of 65% in 2061. Assumption concerning GDP growth rate is the most complex and uncertain process considering that it is the most volatile indicator that depends on several economic, political, health elements and related shocks. Considering the past growth trajectory and current growth rate, we have set short-term growth targets. But, the goalpost has been set based on the GDP growth rate of large developed economies. Hence, the annual growth rate in 2061 has been assumed to be 4%, with an initial growth rate of 8% until 2035, 7% until 2045, 6% until 2055 and 4% until 2061. Similarly, the total GDP for the base year is taken from the statistics of the Reserve Bank of India (RBI). The annual growth rate of GDP during 1991–2016 has been estimated from the statistics of the RBI [RBI (2020)].
6.3.2 Education input indicators
The educational input indicators for the RAPID module considers the age of entry into school (one-time entry), the number of years of schooling (one-time entry), the enrolment rates of schools (in percentage), the number of students per teacher, and the number of students per school separately for both primary and secondary schools. The age of entry to primary and secondary schools at the base year (1991) was 6 and 11 years, respectively. The number of years of schooling is 5 years each for both primary and secondary schools. The gross enrolment ratio (GER) is collected from the Unified District Information System for Education (U-DISE) fact sheets [NIEPA (2017)]. For 2061, the GER is assumed to be 100% for both primary and secondary schools, since, after more than four decades, almost all children in India are expected to enrol in schools up to the secondary level. The statistics on the number of students per primary and secondary school teacher are obtained from the U-DISE. The student–teacher ratio is assumed to be 13, as observed among the developed countries.
6.3.3 Health input indicators
The indicators of health for inputs in the RAPID module are population per doctor, population per nurse, population per health center, population per hospital, population per hospital bed, and annual health expenditure. The statistics on the population per doctor, nurse, health center, hospital, and hospital bed during 1991–2016 were taken from the World Development Indicators database [GOI (2016), World Bank (2016)]. For the year 2061, the statistics have been taken from developed countries, as India may achieve an equivalent health infrastructure in the next four decades [World Bank (2016)]. The annual health care expenditure per capita for the year 2014–2015 was taken from the National Health Accounts of India [Government of India (2017)]. The amount includes the expenditure from public and private sectors and donations from international agencies. For the estimates of the next years until 2061, the expenditure has been adjusted with the current rate of inflation.
6.4 Estimation of outcome indicators
6.4.1 FP cost and revenue
Gross cost is the total expenditure of FP services provided, which includes both temporary and permanent methods. The gross cost depends on the potential FP adopters and is the multiplication of cost per adopter and the number of adopters. Revenue on the other hand includes fees for the use of temporary contraceptive methods and acceptance of permanent methods. The net cost is the result of total cost or gross cost subtracted by the revenue [Stover et al. (Reference Stover, J. E., M. N., E. L., H. S., M. and B.2017)]. The statistical representation of the gross cost, revenue and the net cost at time t under the identified projection scenario j is given as follows.

Furthermore, the GDP per capita has been projected assuming that the growth in GDP is not affected by the growth in population. Based on historical trends and country-specific futuristic goals, the annual rate of GDP growth has been projected. It is a general consensus that with the decline in the population, growth of GDP per capita would increase. Thus, GDP for time t under jth scenario is estimated as

where GDPpercapitat,j is the estimated GDP per capita and $\sum\nolimits_{x = i}^k {P_{x, t, j}}$ is projected population.
6.4.2 Educational infrastructure
School enrolment projections are carried out to determine the potential future needs of educational institutions and resources under the four scenarios. To compute the potential number of primary school-aged children, participation ratio (PR) method has been adopted based on the U-DISE data [NIEPA (2016‒17)]. A fixed ratio that is similar to that of the developed countries has been taken for the year 2061. Then a linear interpolation has been made between 2016 and 2061. The assumptions for other indicators for 2061 are also based on previous literature and the targets set by the Government of India and SDGs. The projected ratios were then applied to the population projection considering age and sex composition.
6.4.3 Children of primary school age
Children of primary school age are calculated as the summation of all children who are at the ages to attend primary school under the jth projection scenario, the mathematical formulation can be written as follows:

where x represents the age; i represents the age at which primary schooling begins and k represents the number of years of primary schooling. Also, Pp x,t refers to the population at primary school age x at year t. Therefore, the potential number of primary students (PPs,t,j) under the jth projection scenario is estimated as

where PER refers to the primary enrolment rate.
6.4.4 Children of secondary school age
Similarly, the number of children of secondary school age (PSst) and the number of secondary students (SSst) under the jth projection scenario are estimated using the PR technique as followed in the previous section:

Therefore, the potential number of primary students (P s,t) under the jth projection scenario is estimated as

where SER refers to the secondary enrolment rate.
6.4.5 Required number of teachers
With the change in the demographics of school-going children, there will be a change in teacher requirements in the future. From the above indicators, the number of school-going children was estimated for each year till 2061. Furthermore, the ratio of students to teachers was computed for the projected scenarios till 2061. The projected ratio of students to teachers has been corroborated from various sources and projected for the future as an input to the data. The number of teachers required in primary (TPj) and secondary schools (TSj) under the jth projection scenario has been computed by employing the projected student to teacher ratio as follows:

where for jth projection scenario SPt,j represents the total number of students in primary school and TPRt,j represents the ratio of students to teachers in primary school.
Similarly, the secondary school teacher required (TSj) is calculated by the formula

where SSt t,j represents the total number of students in secondary school and TSRt,j represents the ratio of students to teachers in secondary school.
6.4.6 Required number of schools
The number of schools required under the jth projection scenario is calculated by projecting the ratio of students to schools (SSR). The formula used to calculate the estimated number of schools (SS) required in India under four different scenarios is

where S t,j is the sum of SPt,j and SSt t,j.
6.4.7 Health infrastructure requirements
With the change in the population, there will be a rising demand in the health infrastructure in India. This section calculates the potential changes required for doctors, nurses, hospitals and expected health expenditures in the future.
Potential number of doctors: Similar to the previous section, the ratio method has been used, where doctors per thousand were obtained from official reports. The doctor to population ratio has been estimated for each projection scenarios. The number of doctors in the future (DRt,j) is projected by taking the ratio of the total projected population (TP) to that of the number of people to doctor ratio (DPRt,j). The mathematical equation can be given as follows:

Potential number of nurses: The potential number of nurses required (NRt,j) in the future is projected by dividing the projected total population at time t in jth projection scenario to number of nurses per person (NPRt,j) in time t as

Potential number of hospitals: Similarly, the potential number of hospitals required (HHt,j) with the changing population is calculated as follows:

where HPt,j represents population per hospital at time t under jth projection scenario.
Annual recurrent health expenditure: The annual recurrent expenditure (ARE) is calculated by multiplying total population (TPt,j) by the recurrent expenditure per person (REPt,j).

7. Results
7.1 Cost and revenues of family planning
In this section, we present the economic cost of the FP program and returns for the period 1991–2016 and 2016–2061. Our findings reveal that the cost of the FP program has increased from 537 million rupees in 1991 to 1631 million rupees in 2016 to reduce the fertility of India from 4 births per woman to 2.3 births per woman during the same period (Figure 2a). In response, the revenues have increased from 2.5 billion rupees in 1991 to 74 billion rupees in 2016 (Figure 2b). During 1991–2016, the total cost for the FP program was 6.7 billion rupees, while the revenue from the investment was 193 billion rupees. In other words, during the same period, the cost-revenue ratio increased from 1:5 in 1991 to 1:45 in 2016 (Figure 2c). The cumulative cost–revenue ratio can be increased to 1:96 in 2030, 1:235 in 2045 and 1:628 in 2061 if fertility declines to the predicted level of TFR 1.8, following the Gompertz curve (Table 2). In 2061, the cost–revenue ratio was projected to be the highest for the high variant of fertility (TFR 1.8). This may be because a fertility level that is lower than just below the replacement level for a long time may increase the burden of the older population and consequently yield lower support ratios, which may result in a lower cost–revenue ratio from the FP program.
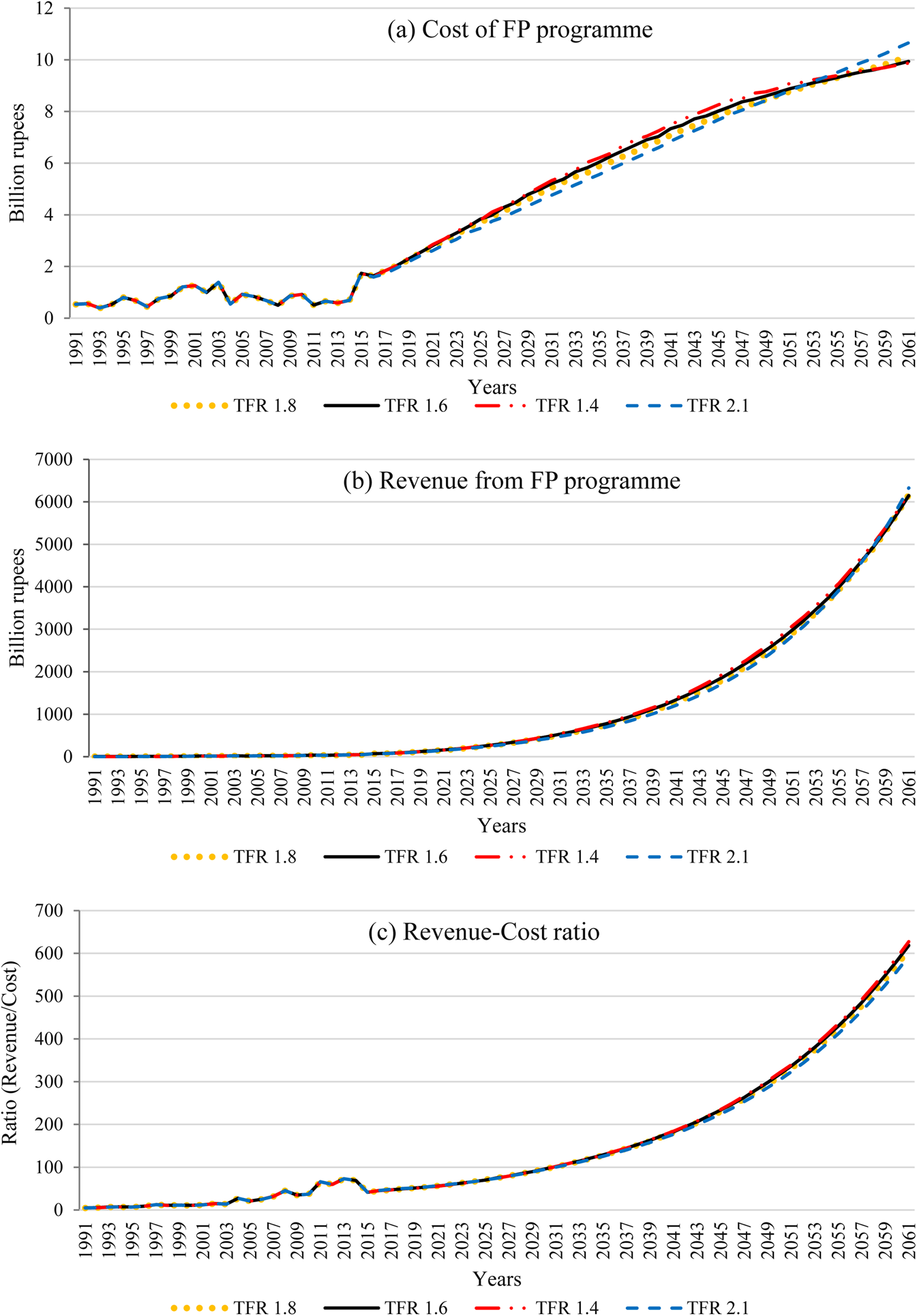
Figure 2. Cost and revenue of family planning services in India, 1991–2061. (a) Cost of FP program. (b) Revenue from FP program. (c) Revenue–Cost ratio.
Table 2. Projected cost–revenue ratio of FP program in India

7.2 Economic returns of FP program
The economic returns from fertility decline are estimated by the GDP in both total and per capita terms. The total GDP increased from 5 trillion rupees in 1991 to 130 trillion rupees in 2016, and is projected to rise to 492 trillion rupees in 2030, 1531 trillion rupees in 2045 and 3906 trillion rupees in 2061 (Figure A2). The GDP per capita steadily increased from 6000 rupees per person to 107,000 rupees per person during 1991–2016 (Figure 3). It can further increase to 2.7 million rupees per person in 2061 if the fertility level declines to TFR 1.6 in the same year (TFR 1.8: 2.6 million rupees per person; TFR 1.4: 2.8 million rupees per person). For the year 2030, the GDP projections for India (492 trillion rupees) falls within the range of pessimistic (455.6 trillion rupees) and optimistic (670 trillion rupees) figures reported by Bloomberg economics [Bloomberg Economics (2019)].

Figure 3. Estimated and projected GDP per capita in India, 1991–2061.
7.3 Requisites for reaping the demographic dividend
In the next few decades, India has the window of opportunity to reap the advantages of a large working-age population. As mentioned earlier, education and health play an important role in taking advantage of the demographic dividend [Prettner et al. (Reference Prettner, Bloom and Strulik2013), James and Goli (Reference James and Goli2016)]. Therefore, we have projected the requisite educational and health infrastructure for the next four decades and beyond.
7.3.1 Education
The number of school-age children and students was estimated and projected to determine the requirements for teachers and schools up to 2061. We have also projected the required educational infrastructure for primary- and secondary-level students, particularly with respect to the number of schools and teachers.
7.3.2 School-age children and students in primary and secondary schools
In this section, we describe the school-age children and students in primary and secondary schools during 1991–2016 and project figures for 2016–2061. The number of primary school-age children increased from 105 million in 1991 to 112 million in 2012 and declined to 109 million in 2016 (Figure 4a). The number may further decline to 66 million in 2061 if fertility is reduced to TFR 1.6 in the same year (TFR 1.8: 76 million; TFR 1.4: 57 million). Similarly, the number of students in primary school increased from 88 million in 1991 to 129 million in 2011, and it may decline to 108 million in 2016 (Figure 4b). We find that the gap between school-age children and students in primary school will be narrowed and eventually be zero in 2061. The school-age children in secondary schools increased from 89 million in 1991 to 110 in 2016 (Figure 4c). The number of children is projected to be highest during 2018–2020; again, it will reduce to 71 million in 2061 if the TFR declines to 1.6 in the same year (TFR 1.8: 80 million; TFR 1.4: 63 million). Similarly, the number of students in secondary school increased from 59 million to 97 million during 1991–2016 (Figure 4d). It is projected that the gap between secondary school-age children and secondary students will be zero in 2061.

Figure 4. Estimated and projected educational infra-structure required in India, 2016–2061. (a) Number of primary school-age children. (b) Number of primary students. (c) Number of secondary school-age children. (d) Number of secondary students. (e) Primary school teachers required. (f) Secondary school teachers required. (g) Primary schools required. (h) Secondary schools required.
7.3.3 Teachers required in primary and secondary schools
The estimated numbers of primary and secondary school teachers during 1991–2061 are presented in Figures 4e and 4f, respectively. The results suggest that the number of primary school teachers has increased from 2 million to 4.7 million during 1991–2016. The estimated number of teachers required can gradually increase to 5.1 million in 2061 in order to maintain the teacher–student ratio comparable to that of developed countries. In 2061, the estimated number of required teachers for primary schools may vary from 5.9 million to 4.4 million for high TFR (1.8) and low TFR (1.4) fertility projections, respectively. The required number of teachers for secondary schools increased from 1.85 million in 1991 to 3.6 million in 2016 (Figure 4f) and is projected to increase further to 5.44 million in 2061 if the TFR were to decline to 1.6 in the same year (TFR 1.8: 6.14 million; TFR 1.4: 4.86 million).
7.3.4 Primary and secondary schools required
The estimated and projected number of schools to cater to primary and secondary students are presented in Figures 4g and 4h. The numbers show an increase from 0.50 million in 1991 to 0.77 million in 2010 but a decrease to 0.70 million in 2016 (Figure 4g). The number of primary schools required may further reduce to 0.4 million in 2061 if the fertility level decreases to TFR 1.6 in that year (TFR 1.8: 0.49 million; TFR 1.4: 0.37 million). Approximately 0.27 million secondary schools were required in 1991, which increased to 0.57 million in 2016 (Figure 4h). The number of secondary schools required is projected to be approximately 0.42 million in 2061 if the fertility rate decreases to TFR 1.6 (TFR 1.8: 0.47 million; TFR 1.4: 0.37 million).
7.4 Health
A few key indicators of health infrastructure are estimated and projected as a prerequisite for the healthy population in India. These indicators include health personnel, health infrastructure and annual health care expenditure, which are described in this section.
7.4.1 Required medical health personnel
The required medical health personnel are estimated in terms of the number of doctors and nurses in hospitals. The required number of doctors was 0.39 million in 1991, which increased to 0.72 million in 2016 (Figure 5a). Furthermore, the number of required doctors may increase to 3.59 million in 2061 if the fertility rate declines to TFR 1.6 in the same year (TFR 1.8: 3.74 million; TFR 1.4: 3.47 million). Similarly, the number of required nurses increased from 0.3 million to 2.5 million during 1991–2016 (Figure 5b). The number of required nurses can further be increased to 14.1 million in 2061 at the medium variant of fertility decline (TFR 1.8: 14.8 million; TFR 1.4: 16.6 million).

Figure 5. Estimated and projected health infra-structure required, 2016–2061. (a) Doctors required. (b) Nurse required. (c) Hospitals required. (d) Hospital beds required.
7.4.2 Required medical health infrastructure
The requisite medical infrastructure is estimated in terms of the number of hospitals and hospital beds during 1991–2016 and the projection of the same for 2016–2061. The number of hospitals increased from 0.012 million in 1991 to 0.013 million in 2016 (Figure 5c). Furthermore, the required number of hospitals is projected to reach 0.025 million in 2061 if fertility declines to TFR 1.6 in that year (TFR 1.8: 0.026 million; TFR 1.4: 0.025 million). The number of hospital beds also decreased from 0.80 million in 1991 to 0.59 million in 2016 (Figure 5d). The projected figures suggest that the required number of hospital beds will increase to 0.78 million in 2061 for the medium variant of fertility decline (TFR 1.8: 0.88 million; TFR 1.4: 0.75 million).
7.4.3 Required annual recurrent health care expenditure
The necessary annual recurrent health care expenditure is estimated from 1991 to 2016 and projected to 2016–2061. It increased from 0.1 trillion rupees to 1.7 trillion rupees during 1991–2016 (Figure 6). The public health expenditure in India for 2016 is roughly accounted for around 1.3% of estimated GDP, which is almost equivalent to reported health budgets by Government of India (Reserve Bank of India, 2020). It may further increase to 42.5 trillion rupees in 2061 if the fertility level declines to TFR 1.6 in the same year (TFR 1.8: 44.3 trillion rupees; TFR 1.4: 41.1 trillion rupees). However, as a result of the tremendous reduction in avoidable expenditure on reproductive, MCH care following FP and fertility decline, the percentage share of total public health expenditure in GDP remains slightly above 1% of the GDP. These findings are in line with the life cycle savings as results of FP and fertility decline reported by Lee et al. (Reference Lee, Mason and Miller2000), Canning and Schultz (Reference Canning and Paul Schultz2012) and Kohler and Behrman (Reference Kohler and Behrman2014).
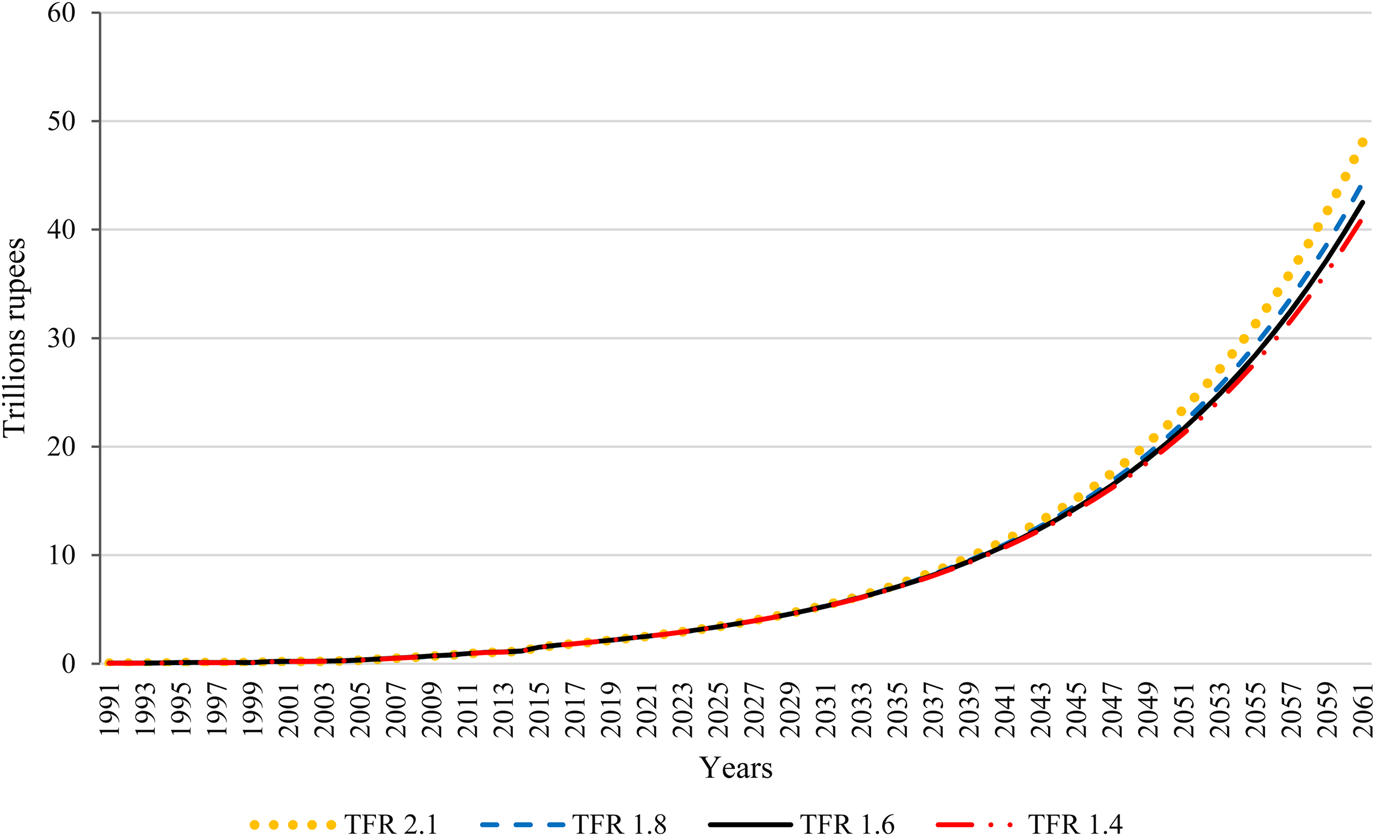
Figure 6. Estimated and projected annual recurrent health expenditure in India, 2016–2061.
8. Conclusions and policy implications
The economic rationale for FP investments has been receiving considerable attention in the global demographic, public health, and economic literature. While governments and international organizations are interested in the returns on decades of investments in FP, so far there has not been any study that estimates ROI of FP programs for India. Thus, for the first time in the Indian context, this study estimates economic returns for the investment in the FP program and consequent TFR decline. Our findings suggest that India will have greater benefits of FP investments to lifetime economic returns compared to the world average (cost–revenue ratio of 1:120) by 2045 (1:235) and will maximize it further by 2061 (1:628) [Kohler and Behrman (Reference Kohler and Behrman2014)]. Although different scenarios of TFR levels at the goalpost (the year 2061) show various levels of lifetime returns of FP, TFR <1.8 will be counterproductive and will reduce the potential benefits. Although our findings in the context of India are straightaway not comparable to similar studies in the context of African countries, especially considering differences in methodologies adopted and circumstantial variations, the qualitative nature of our results and broader conclusions are in line with findings reported by Cavalcanti et al. (Reference Cavalcanti, Kocharkov and Santos2020), Karra et al. (Reference Karra, Canning and Wilde2017) and Kohler and Behrman (Reference Kohler and Behrman2014).
Like any other studies using a simulation approach, our study suffers from some limitations. For our model, we rather conservatively treat children as “commodities” or “economic goods and services”. The fertility scenarios are markedly determined by developmental factors and also social values (e.g., the quality–quantity trade-off of fertility reduction, old-age insecurities of parents, and opportunity costs of mothers). Cost and benefits have not always been quantified in economic terms as social values are also attached to it [Galor and Weil (Reference Galor and Weil2000), Doepke (Reference Doepke2004), Singh et al. (Reference Singh, Darroch, Ashford and Vlassoff2009)]. Our model does not consider the value judgement of fertility behaviors, thus fail to capture the spill-over effects (emotional well-being and broader welfare outcomes). Furthermore, in our interpretation of the relationship between fertility decline and economic growth, the endogeneity of fertility decline due to economic growth is not taken into consideration. However, this is less likely to affect the qualitative interpretation of projected ROI estimates of an FP program. Although our model considers several parameters and is more inclusive compared to other models [Karra et al. (Reference Karra, Canning and Wilde2017), Cavalcanti et al. (Reference Cavalcanti, Kocharkov and Santos2020)], we have excluded a few institutional factors such as the efficiency of governance and investment in basic infrastructure.
Nevertheless, the findings of this study would help Indian policy-makers to emphasize on the FP program and thereby achieve the SDGs. With a comprehensive approach, if the country focuses more on improving the quality of FP services and reducing the unmet need for FP to enhance reproductive health and expand opportunities for education and employment for both women and men, then it can improve its potential to reap more benefits than those reported here. The study draws the special attention of the policy-makers towards spending on education and health infrastructure required to reap the demographic dividend for the country. The estimates of the required educational and health infrastructure and expenditure can be set as benchmarks of supplies and infrastructural development for the country. Finally, the study suggests that reducing health care spending on avoidable maternal and child morbidities and rising household savings through quality FP services can contribute substantially to economic growth in India. A similar approach can be adopted in other developing countries as well.
For the research and practice purpose, this study argues that Spectrum-based simulation is a more robust analytical approach to determine the ROI of not only FP programs but also other reproductive MCH programs. Especially, the vast inclusiveness of the indicators in the model makes it a more reliable approach to estimate the ROI of FP. We have also provided several optimal procedures to estimate the input indicator and set the goalpost assumptions.
Supplementary material
The supplementary material for this article can be found at https://doi.org/10.1017/dem.2021.3.
Acknowledgement
The authors acknowledge UNFPA, India office for funding the study. The authors benefited immensely from the suggestions of the national level technical advisory board members (viz. Prof. P.M. Kulkarni, Prof. Arvind Pandey and Dr. Sanjay Kumar) appointed by UNFPA, India and others who participated in the findings dissemination seminar on 3rd May 2019. They thank Prof. David Canning, Prof. Jocelyn E. Finlay (Harvard University), and other participants for their comments during the presentation of the earlier version of this paper at the first IUSSP Population, Poverty, and Inequality Research Conference, Ann Arbor (MI), United States, 27–29 June 2019. However, the errors remaining are the sole responsibility of the authors.