1 Introduction
‘Shall I apply for a new job?’. ‘Shall I move house?’. ‘Shall I have a baby?’. Everyday decisions are not trivial decisions. They are often complex, multiattribute decisions with multiple risks and uncertainties. For the decision maker they may be critical, even life changing choices. Or they may be smaller, daily decisions – but smaller decisions made repeatedly add up over time to major differences in life outcomes in health and wellbeing, relationships, finance, career etc. The aim of everyday decision making is to choose the option that leads to a successful outcome. How do people make decisions to achieve this outcome?
Many approaches to decision making recommend selecting an option that maximises expected utility in order to achieve the best decision outcome (Edwards & Fasolo, Reference Edwards and Fasolo2001; Milkman, Chugh & Bazermann, Reference Milkman, Chugh and Bazerman2009). However, these methods are difficult to apply to complex, everyday decisions and have rarely been evaluated to test if they predict choices that lead to successful and satisfying outcomes. In contrast, associative knowledge is typically linked to biased decision outcomes (Morewedge & Kahneman, Reference Morewedge and Kahneman2010). But it may be adaptive to draw upon associative knowledge in everyday decision making because associations between choices and their outcomes are grounded in an individuals’ experience, and they may be valid predictors of successful outcomes in personally meaningful decisions (Banks, Reference Banks2021). In this paper we investigate attribute-based and associative methods of decision making and compare how well they predict everyday decision outcomes. Across three experiments we find that attribute-based decision processes are not more successful than simpler heuristics or decisions using associative knowledge. The most successful everyday decisions arise when attribute-based and associative knowledge is combined.
1.1 Analytic approaches to everyday decision making
Several lines of research suggest that the best approach to decision making is to make choice that maximises expected utility in order to achieve the best decision outcome (e.g., Bruine de Bruin, Parker & Fischoff, Reference Bruine de Bruin, Parker and Fischhoff2007; Edwards & Fasolo, Reference Edwards and Fasolo2001; Milkman, Chugh, & Bazermann, Reference Milkman, Chugh and Bazerman2009). Thinking can be changed to follow rational principles more closely through training (e.g., Morewedge, Yoon, Scopelitti, Symborski, Korris & Hassam, Reference Morewedge, Yoon, Scopelliti, Symborski, Korris and Kassam2015) or the deliberate application of cognitive strategies such as taking an outside view (e.g., Flyvbjerg, Reference Flyvbjerg2013). Decision making competence, the ability to follow the principles of rational choice derived from normative models, has been found to be associated with scores on the Decision Outcome Inventory, a measure of negative life events (Bruine de Bruin, Parker & Fischoff, Reference Bruine de Bruin, Parker and Fischhoff2007; Parker, Bruine De Bruin & Fischhoff, Reference Parker, Bruine de Bruin and Fischhoff2015). Common methods of decision analysis such as multiattribute utility analysis follow analytic processes explicitly in order to find the optional that maximises expected utility (e.g., Edwards & Fasolo, Reference Edwards and Fasolo2001; Hammond, Keeney & Raiffa, Reference Hammond, Keeney and Raiffa1999; Keeney & Raiffa, Reference Keeney and Raiffa1993). However, these studies do not provide direct evidence that analytic choices lead to better outcomes in everyday decision making.
There are several reasons why these approaches have so far not provided this evidence. First, the most common experimental paradigms for testing the ability of participants to make an optimal decision or resist a bias judge success against the standard of a statistical principle that is taken as normative (Gigerenzer, Reference Edwards and Barron1996). This leaves open the question of whether the chosen normative principle is in fact the most appropriate for everyday decision making and therefore whether the effort to comply with it is beneficial in everyday situations (Arkes, Gigerenzer, & Hertig, Reference Arkes, Gigerenzer and Hertwig2016; Weiss & Shanteau, Reference Weiss and Shanteau2021). Second, most decision research relies on hypothetical decisions that do not closely resemble everyday decisions. As a result, theories developed to explain decisions in common experimental paradigms may not fully explain or predict successful everyday decision making (Fischoff, Reference Fischhoff1996). Third, decision analysis enables decision makers to simulate an analytic decision process, but studies often do not follow up the decision to discover if the outcome was successful. This is because they are often applied to large, uncertain, one-shot decisions in which chance could lead to a poor outcome (Wallenius, Dyer, Fishburn, Steuer, Zionts, Deb, Reference Wallenius, Dyer, Fishburn, Steuer, Zionts and Deb2008). However, the consequence is that there is little empirical evaluation of whether the most successful choice was in fact made. Overall, there is surprisingly little evidence examining whether deliberately applying an analytic decision process leads to successful decision outcomes in personally meaningful everyday decisions, or indeed many real-world decisions.
In order to test whether an analytic, attribute-based approach to everyday decision making leads to a successful and satisfying outcome, we applied multiattribute utility (MAU) to participants’ everyday decisions to predict the most successful choice (Keeney & Raiffa, Reference Keeney and Raiffa1993). However, a limitation of this approach is the difficulty people have in applying this method in practice to a complex decision (Simon, Reference Simon1955). We therefore tested simpler heuristics as well. These are efficient cognitive processes that ignore information (Gigerenzer & Brighton, Reference Gigerenzer, Brighton, Green and Armstrong2009) and can therefore be applied in practice to everyday decisions. Early work demonstrated that simpler models were as effective as the complex models (Lovie & Lovie, Reference Lovie and Lovie1986). For example the Equal Weights rule (EW) in which each attribute is given the same weighting (Dawes & Corrgian, Reference Dawes and Corrigan1974) and Tallying in which each attribute has a binary value (Russo & Dosher, Reference Russo and Dosher1983) have been found to predict outcomes effectively in real-world decisions (Dawes, Reference Dawes1979). Similarly, Makridakis and Hibon (Reference Makridakis and Hibon1979) found simpler models to be as effective as more complex models. Simplifications such as using expected values and using quantiles to represent probability distributions approximate the more complex results from MAU (Durbach & Stewart, Reference Durbach and Stewart2009; Durbach & Stewart, Reference Durbach and Stewart2012). More recently, Green and Armstrong (2015) reviewed a range of models and did not find that model complexity increases forecasting accuracy.
Research on fast and frugal heuristics has explored “ecologically rational” simple heuristics. For example, Take the Best uses only the most valid cue that discriminates between options (Gigerenzer & Goldstein, Reference Edwards and Barron1996). This can make predictions as accurately as a linear regression model (Czerlinski, Gigerenzer & Goldstein, Reference Czerlinski, Gigerenzer, Goldstein, Gigerenzer and Todd1999). Fast and frugal trees link several cues together (Martignon, Katsikopoulos & Woike, Reference Martignon, Katsikopoulos and Woike2008). These explain decision making in applied settings well. For example, a fast and frugal heuristic of military decision making explained 80% of decisions with three cues and was as effective as standard military decision making methods (Banks, Gamblin & Hutchinson, Reference Banks, Gamblin and Hutchinson2020). Simple heuristics are effective at classifying objects in a range of real-world situations (Katsikopoulos, Simsek, Buckman & Gigerenzer, Reference Katsikopoulos, Simsek, Buckmann and Gigerenzer2020).
In this paper, we tested heuristics that are systematic simplifications of analytic models. Shah and Oppenheimer (Reference Shah and Oppenheimer2008) propose a framework for heuristics that simplifies different aspects of an analytic model, such as simplifying the weighting of cues and examining fewer cues. In particular we tested the Equal Weights rule (EW) and Tallying that simplify the weighting of cues. We also tested a rule using only the first reported attribute, thus simplifying the number of cues. Finally, we tested the simplest heuristic, Take the First (TTF), in which the first option considered for a decision is chosen (Johnson & Raab, Reference Johnson and Raab2003).
1.2 Associative knowledge and everyday decision making
The analytic approaches discussed so far rely on deliberate analysis of the decision to identify the optimal choice. In contrast, associative processes have been linked to biases (Morewedge & Kahneman, Reference Morewedge and Kahneman2010), for example anchoring effects (Mussweiler, Strack & Pfeiffer, Reference Mussweiler, Strack and Pfeiffer2000) and preference reversals (Bhatia, Reference Banks2013). Dual process theories highlight the benefits of associative processes as fast and simple heuristic responses to problems that may be effective (System 1). But when they conflict with the responses generated by attribute-based processes (System 2), the attribute-based process will override the associative response (Evans & Stanovich, Reference Evans and Stanovich2013; Kahneman & Frederick, Reference Kahneman, Frederick, Gilovich, Griffin and Kahneman2002; Sloman, Reference Sloman1996; Stanovich & West, Reference Stanovich and West2000). This theory implies that analytic, attribute-based thought is optimal and intervenes to prevent biased, heuristic responses.
However, more recent hybrid dual process theories have suggested intuitions may be logical (De Neys, Reference De Neys2012) and there is often no need for a deliberate, attribute-based process to correct faulty initial responses (Bago & De Neys, Reference Bago and De Neys2019). The effectiveness of intuitions may be learnt through experience (Raoelison, Boissin, Borst & De Neys, Reference Raoelison, Boissin, Borst and De Neys2021). As associative knowledge is learnt from prior experience, it is most likely to be valid information in domains of personal relevance to the individual and applying it will be an adaptive strategy (Banks, Reference Banks2021). For example, applied studies show that experts can make reliable intuitive judgements based on learnt associations between cues and outcomes, given a high-validity environment, the opportunity to learn the association, and a real rather than hypothetical decision (Crandall & Getchell-Reiter, Reference Crandall and Getchell-Reiter1993; Kahneman & Klein, Reference Kahneman and Klein2009). Overall, this suggests that associative knowledge is not inherently biased. But its effectiveness has not been tested on meaningful decisions with experimental methods.
In order to test whether associative knowledge about everyday decisions predicts a successful and satisfying outcome, we used a process of free association to elicit the thoughts and images associated with each decision outcome (Szalay and Deese, Reference Szalay and Deese1978). Participants rated the utility of each of these associates and we tested rules that were equivalent to those applied to the attribute-based knowledge. We calculated total rating of all of the associates to test the Free Association Utility (FAU). We calculated the binary values of the associates as good and bad and tallied these (FAUtal). We also tested the rating of the first associate only (FAUFAO).
1.3 Individual and Decision Characteristics
The ability to make successful decisions has been linked with characteristics of both the individual and the decision. The willingness to override intuitive responses, measured using the Cognitive Reflection Test (Frederick, Reference Frederick2005), is associated with, for example, skepticism about paranormal phenomena and less credulity for fake news (Pennycook, Fugelsang & Koehler, Reference Pennycook, Fugelsang and Koehler2015; Pennycook & Rand, Reference Pennycook and Rand2019). The Rational-Experiential Inventory also measures the tendency to engage in rational thought and has been linked to optimal choices on common decision tasks, but the association with everyday decision making has not been tested (Epstein, Pacini, Denes-Raj & Heier, Reference Epstein, Pacini, Denes-Raj and Heier1996; Pacini & Epsten, Reference Pacini and Epstein1999). High levels of numeracy are associated with higher expected utility of choices on decision tasks, but again the association with everyday decision making has not been tested (Cokely, Galesic, Schulz, Ghazal & Garcia-Retamero, Reference Cokely, Galesic, Schulz, Ghazal and Garcia-Retamero2012; Cokely & Kelly; Reference Cokely and Kelley2009). We asked whether the tendency and ability to engage in attribute-based thought increases the success of everyday decision making.
Everyday decisions are likely to vary in characteristics and this may influence the process and success of the decision (e.g., Hogarth, Reference Hogarth, Betsch and Haberstroh2005). Frequently encountered decisions may enable associations to be learnt with decision outcomes, increasing the validity of associative knowledge, whereas more complex decisions may make valid associations harder to acquire (Banks, Reference Banks2021; Kahneman & Klein, Reference Kahneman and Klein2009). We also investigate whether the importance of the decision and knowledge about the decision influences the success of the decision.
1.4 The Current Research
To test how effective attribute-based and associative processes are in everyday decision making, we developed the Everyday Decision Making Task. The aim of this task is to enable the systematic study of participants’ own, meaningful, everyday decisions and the options that they consider for each decision. Rather than being given a decision to make, participants report an everyday decision that they are currently facing. They then identify two options that they will choose between for this decision. We then elicit information from them about the options, either the relevant attributes or associates, and they rate this information. Finally, participants rate the options and select which one they will choose. We use the information elicited from them about the options to calculate the decision rules: multiattribute utility, equal weights, tallying, take the first, first attribute only, free association utility, free association tallying, and first associate only. We can then test which rule most accurately explains the choice they made, and which rule best predicts the most successful and satisfying outcome for them. The key feature of the everyday decision making task is that each participant is making their own unique decision, but the structure of the task means that they can be analysed quantitatively across conditions and participants.
We do not operationalise successful decision making as complying to an explicit normative model. Instead, we are interested in how each decision maker rates the outcome of their decision (Weiss & Shanteau, Reference Weiss and Shanteau2021). As the everyday decision making task elicits genuine decisions that participants are currently facing, their assessment of the outcome of those decisions is grounded in their experience of the decision and its outcome. This measure therefore directly assesses the aim of everyday decision making, which is to make a choice that leads to the most successful and satisfying outcome for the decision maker.
Experiment 1 tested how well the decision rules explained the decision made and predicted how satisfying and successful the decision was. Experiment 2 replicated this using a within subjects design to directly compare the alternative decision rules. Experiment 3 again used a within subjects design but within a longitudinal study to test how well different decision rules predicted future satisfaction and success, after the outcome of the decision was known.
2 Experiment 1
The aim of the first experiment was to test how well different decision methods predicted decision satisfaction and success on the everyday decision making task. Participants were allocated to one of three conditions. In the Attributes condition participants completed the everyday decision making task and reported up to six attributes and then rated the value and likelihood of each attribute for each decision option. This is based on the multiattribute utility method developed for everyday decision making by Weiss, Edwards and Mouttapa (Reference Weiss, Edwards, Mouttapa, Weiss and Weiss2009). However, whilst Weiss et al. presented each participant with the same decision and set of attributes, we allowed each participant to generate their own decision and set of attributes. In the Associative condition participants completed the everyday decision making task and rated the value of the associative knowledge linked with each option. Associative knowledge was elicited using the method developed by Szalay and Deese (Reference Szalay and Deese1978) and used to assess the affect heuristic by Slovic et al. (Reference Slovic, Layman, Kraus, Flynn, Chalmers and Gesell1991). In our ‘free association’ adaptation of the method, participants listed up to six thoughts or images that came to mind when they thought of each option, then they rated how good or bad they felt each of these associates were. In the control condition participants completed the everyday decision making task and rated the options without any further intervention.
2.1 Method
2.1.1 Participants
A sample of three hundred and three participants were recruited online using the Prolific Academic participant pool (http://www.prolific.co). Twenty-five participants were removed for failing an attention check or failing to provide a coherent everyday decision. A sample two hundred and seventy-eight participants (104 male, 174 female) remained. Their mean age was 36.63 (SD = 12.67). In order to take part, participants were required to have English as a first language. We compensated participants for their time at a rate of £6 per hour.
2.1.2 Design
A between subjects design with three conditions was used. Participants were randomly allocated to either the attributes condition, the associative condition, or the control condition. The dependent variable was the decision satisfaction scale.
2.1.3 Materials
Everyday decision making task. The everyday decision making task first asks participants to report a personal decision that they are about to make and are currently thinking about but have not yet made. They are asked to think of a decision with two options and then asked to report those options. They are then asked a series of questions about the decision, depending on the condition.
In the attributes condition, participants were asked to think of the most important attributes that might cause them to prefer one option or the other. They were asked to take a moment to think through the decision carefully and thoroughly and list factors that were independent of each other. They reported up to six attributes. Next, participants rated the value of each of their attributes for each option in turn on a scale from -3 (extremely bad) to +3 (extremely good). Finally, participants rated how likely each attribute was to occur for each option in turn on a seven point scale (extremely unlikely, moderately unlikely, slightly unlikely, equal chance, slightly likely, moderately likely, extremely likely).
In the associative condition, participants reported up to six thoughts or images that they associated with each option in turn. They were asked to write down the first thoughts or images that came to mind. Participants then rated how good or bad each thought and image was for each option in turn on a scale from -3 (extremely bad) to +3 (extremely good).
In the control condition, participants were asked to reflect on their decision without any specific questions.
Decision Satisfaction Scale. This scale was created to assess overall satisfaction with the decision. It is comprised of six items: ‘I am satisfied with my decision’; ‘This decision will be successful’; ‘I am confident this is the best decision’; ‘I have thought carefully about the decision’; and ‘I am fully informed about the decision’. Within this scale the item ‘It is important that you pay attention to this study. Please select ’Strongly disagree’.’ was used as an attention check. Participants rated how much they agreed with these statements on a seven point scale from ‘strongly disagree’ to ‘strongly agree’.
Individual and decision characteristics measures. Participants rated the characteristics of the decision: frequency; knowledge; complexity; and importance. Participants also completed the Cognitive Reflection Test (Frederick, Reference Frederick2005), the Berlin Numeracy Test (Cokely et al., Reference Cokely, Galesic, Schulz, Ghazal and Garcia-Retamero2012), and the Rational Experiential Inventory (Epstein et al., Reference Epstein, Pacini, Denes-Raj and Heier1996).
2.2 Results and Discussion
De-identified data are available at https://osf.io/xrg86/?view_only=fb8fa59161674f7586f2399fc3605e2b.
2.2.1 Topics of Everyday Decision Making
First, we examined the topics that participants report they were currently facing. As the everyday decision making task was used in all three experiments, these data are combined into a single table (Table 1).
Table 1: Frequency of everyday decision topics.

Food & drink and Occupation categories were used as examples in the experimental instructions which likely inflated their frequency. It is interesting to note that the important topics of “Health and Finance” had much lower frequencies than “Purchases & Retail” and “Housing & Living arrangements”. The topics of everyday decision making are as might be expected, but some areas are more salient than others.
2.2.2 Predicting Participant Choice
First, we compared how frequently participants chose either the first option they reported (Option A) or the second option (Option B). Significantly more participants chose Option A (60.4%) than Option B (39.6%; Wald = 11.921, df = 1, p = .001; Table 2).
Table 2: Frequencies of intention to choose Option A or B

The preference for Option A over Option B was significant in the Associative (Wald = 3.993, df = 1, p = .046) and Control (Wald = 10.769, df = 1, p = .001) conditions, but not in the attributes condition (Wald = .391, df = 1, p = .532).
Next, we tested how well each decision rule predicted the participants’ final choice. Table S1 (supplementary materials) shows the means and SDs for participants’ ratings of the options. For each rule, the rule prediction for option B was subtracted from the rule prediction for option A to provide a measure of the strength of rule preference for the alternatives. Positive results suggest that the participant should choose option A, and negative results suggest that the participant should choose option B. This score was used as a predictor in a logistic regression with the participants’ choice as the dependent variable (Table 3).
Table 3: Summary logistic regression statistics for the decision rules used to predict the participants’ choice. MAU = multi-attribute; EW = equal weights; Tallying = each outcome has a binary value; FAO = first associate only; FAU = free-association utility.

In the attributes condition, the MAU, EW, and Tallying rules all predict the decision made but FAO does not. Of these, EW explains the most variance followed by MAU. In the associative condition, the FAU and FAUFAO rules predict the decision made but FAUtal does not. As expected, the rating of the alternatives in the control condition predicts the subsequent choice.
2.2.3 Predicting Decision Satisfaction and Success
To test how well each of the decision rules predicted decision satisfaction and success we recategorised the decision rule predictions into variables for chosen or unchosen options. We then used these as predictors in two separate multiple regressions, with decision satisfaction and decision success as the outcome variables (Table 4).
Table 4: Multiple regressions of decision rules predicting decision satisfaction and success in Experiment 1.

Note: p ≤ .001. for the constant in all models.
In the attributes condition all of the decision rules predicted decision satisfaction and the model prediction for the chosen option was a significant predictor in each. FAO explains the most variance followed by MAU. In the Associative condition, the FAU and FAUFAO rules predicted decision satisfaction and the rule prediction for the chosen option was a significant predictor in each but FAUtal did not predict decision satisfaction. FAUFAO explains the most variance followed by FAU. The rating of the chosen option predicted decision satisfaction in the control condition. The first option (TTF) was significantly more satisfying than the second (Option A: M = 5.66, s.d. = 1.00; Option B: M = 5.19, s.d. = 1.30; t(190.211) = 3.22, p = .002).
For success, in the attributes condition all of the decision rules predicted decision success and the rule prediction for the chosen option was a significant predictor in each. FAO explains the most variance followed by EW. In the Associative condition, the FAU and FAUFAO rules predicted decision success and the rule prediction for the chosen option was a significant predictor in each but FAUtal did not predict decision success. FAUFAO explains the most variance followed by FAU. The rating of the chosen option predicted decision success in the control condition. The first option (TTF) was not significantly more successful than the second (Option A: M = 5.41, s.d. = 1.04, Option B: M = 5.35, s.d. = 1.27; t(276) = .47, p = .64).
2.2.4 Confidence, Care, and Feeling Informed
Correlations were performed for the remaining items of the Decision Satisfaction Scale and are available in the supplementary materials. Confidence was positively associated with the chosen alternative for all of the decision rules, except for FAUtal. Thinking carefully and Feeling informed were not significantly associated with any of the decision rules.
Overall, both attribute-based and associative decision rules were equally successful in predicting choice, satisfaction, and success. The best attribute-based and associative decision rules both correctly predicted approximately 70% of decisions. Similarly, the best attribute-based and associative rules were significant predictors of decision satisfaction and success. Weighting the attributes did not improve decision rule performance – EW was as effective as MAU. However, simply tallying, that is, adding pros and subtracting cons as binary cues, was less effective than using the more fine-grained rating scale. This was the case whether tallying attribute-based or associative knowledge. FAUFAO associate did successfully predict choice, satisfaction and success, whereas the FAO did not predict choice significantly but it did predict satisfaction and success.
3 Experiment 2
Experiment 1 found that decision rules based on both attribute-based and associative knowledge predicted choice, satisfaction, and success. However, given the between subjects design, it is not clear if the different decision rules are explaining similar or different variance in the outcomes. On the one hand, the attribute-based and associative knowledge elicitation procedures were quite different and were intended to elicit different types of knowledge. But on the other hand, participants may have generated attributes in an associative manner so that the two types of knowledge were similar in practice. The aim of the second experiment was to compare the relative contribution of attribute-based and associative knowledge to decision outcomes and discover if they are independent sources of information or not. To do this, Experiment 2 replicated the Experiment 1 method using a within subjects design with the attributes and associative conditions enabling a test of how much shared and unique variance is explained by each decision rule.
3.1 Method
3.1.1 Participants
A sample of two hundred and forty-eight participants were recruited online using the Prolific Academic participant pool (www.prolific.co). Eighteen participants were removed for failing an attention check or failing to provide a coherent everyday decision. A sample two hundred and thirty participants (63 male, 167 female) remained. Their mean age was 35.31 (SD = 13.26). In order to take part, participants were required to have English as a first language. We compensated participants for their time at a rate of £6 per hour.
3.1.2 Design
A within subjects design with two conditions was used, the attributes condition and the associative condition. The dependent variable was the decision satisfaction scale.
3.1.3 Materials & Procedure
The materials and procedure were the same as in Experiment 1, except participants completed the decision questions for both the attributes and the associative conditions and they were not presented with a summary of their ratings.
3.2 Results and Discussion
3.2.1 Predicting Participant Choice
First, we compared how frequently participants chose either the first option they reported (Option A) or the second option (Option B). Significantly more participants choice Option A (60.9%) than Option B (39.1%; Wald = 10.694, df = 1, p = .001).
Table S1 (supplementary materials) shows the means and SDs for participants’ ratings of the options. We used logistic regression to test how well each decision rule predicted the participants’ final choice by subtracting the rule prediction for Option B from the rule prediction for Option A as in Experiment 1 (Table 5).
Table 5: Summary logistic regression statistics for the decision rules used to predict the participants’ choice.

All of the decision rules significantly predicted choice. FAU explains the most variance, followed by MAU.
Next, we tested whether participant choices could be better predicted by combining attribute-based and associative rules. Using hierarchical logistic regression, we added FAU at step 2 to MAU in one model, and to EW in another model. In both cases, FAU explained a significant amount of additional variance in participant choice. FAU explained an additional 9.1% of variance on MAU and an additional 10.1% of variance on EW.
3.2.2 Predicting Decision Satisfaction and Success
To test how well each of the decision rules predicted decision satisfaction and success we categorized the decision rule predictions into variables for chosen or unchosen options. We then used these as predictors in multiple regression with decision satisfaction and decision success as the outcome variables as in Experiment 1 (Table 6).
Table 6: Multiple regressions of decision rules predicting decision satisfaction and success in Experiment 2.

Note: p ≤ .001. for the constant in all models.
For satisfaction, all of the decision rules significantly predict decision satisfaction except FAUtal. FAU explains the most variance, followed by FAUFAO and EW. The first option (TTF) was not significantly more satisfying than the second (Option A: M = 5.54, s.d. = 1.21, Option B: M = 5.29, s.d. = 1.23; t(228) = 1.50, p = .13). For success, all of the decision rules significantly predict decision success except FAO and FAUtal. FAUFAO explains the most variance, followed by FAU and EW. The first option (TTF) was not significantly more successful than the second (Option A: M = 5.43, s.d. = 1.11, Option B: M = 5.51, s.d. = 1.01; t(228) = –.57, p = .57).
Next, we asked whether the Free association method explained any variance in decision satisfaction and success in addition to the established MAU procedure. Using a hierarchical multiple regression (Table 7), decision rules based on MAU were entered at step 1 and FAU was entered at step 2. This analysis was conducted with both MAU and EW decision rules. In both cases FAU explained a significant amount of additional variance in decision satisfaction. For satisfaction, FAU explained an additional 8% of variance than MAU and an additional 7.9% of variance than EW. For success, FAU explained an additional 7% of variance than MAU and an additional 6.8% of variance than EW.
Table 7: Hierarchical multiple regressions of decision rules predicting decision satisfaction in Experiment 2.

Note: p ≤ .001. for the constant in all models
3.2.3 Confidence, Care, and Feeling Informed
Correlations were performed for the remaining items of the Decision Satisfaction Scale and are available in the supplementary materials. Confidence was positively associated with the chosen alternative for MAU, EW, Tallying, FAO, and FAUFAO. Careful decision making was positively associated with the chosen alternative for MAU, EW, Tallying, and FAU. Feeling informed was positively associated with the chosen alternative for MAU, EW, FAO, and FAU.
Overall, Experiment 2 largely replicated the findings of Experiment 1. Both attribute-based and associative rules equally predict choice, satisfaction and success. There is no advantage to weighting attributes or associates, but simply tallying explains decisions and outcomes less well. In addition to replicating the main findings of Experiment 1, Experiment 2 also enabled a within subjects comparison of attribute-based and associative rules. These explained different variance in success and satisfaction and combining them was a stronger predictor of decision outcome than attribute-based rules alone. This suggests that these are separate and equally important sources of knowledge that should be combined to best predict decision satisfaction and success.
4 Experiment 3
Experiments 1 and 2 explored which decision rules explain how everyday decisions are made and which decision rules predict ratings of decision satisfaction and decision success at the time at which the decision is made. However, they do not test how well the decision rules predict future decision satisfaction and success, after the outcome of the decision is known. To fully meet the aim of identifying which decision rules predict successful decision outcomes, Experiment 3 used a longitudinal design. Participants were contacted one week after the initial decision was made and asked to rate the outcome of the decision. This was used to test the accuracy of the decision rules at predicting future decision satisfaction and success.
4.1 Method
4.1.1 Participants
A sample of two hundred and fifty participants were recruited online for Part 1 of the study 2 using the Prolific Academic participant pool (http://www.prolific.co.) Forty-five participants were removed for failing an attention check, failing to provide a coherent everyday decision, or failing to consent for their data to be used upon completion of the study. A sample two hundred and five participants (55 male, 148 female, 1 other, and 1 prefer not to say) remained. Of these, 192 participants completed Part 2 of the study. Nine were removed for failing an attention check, failing to provide a coherent everyday decision leaving a sample of 183 participants. In order to take part, participants were required to have English as a first language. We compensated participants for their time at a rate of £6 per hour. The study was approved by the University Research Ethics Committee, and participants provided informed consent prior to participation.
4.1.2 Design
A within subjects design with two conditions was used, the attributes condition and the associative condition. The dependent variable was the decision satisfaction scale.
4.1.3 Materials & Procedure
The materials and procedure were the same as in Experiment 1, except participants completed the decision questions for both the attributes and the associative conditions and they were not presented with a summary of their ratings. One week after the decision was made, participants were contacted and completed a second decision satisfaction and outcome measure.
4.2 Results and Discussion
4.2.1 Predicting Participant Choice at Time 1
First, we compared how frequently participants chose either the first option they reported (Option A) or the second option (Option B). Significantly more participants choice Option A (62.4%) than Option B (37.6%) (Wald = 12.418, df = 1, p < .001).
Table S1 (supplementary materials) shows the means and SDs for participants’ ratings of the options. We used logistic regression to test how well each decision rule predicted the participants’ final choice by subtracting the rule prediction for Option B from the rule prediction for Option A as in Experiment 1 (Table 8).
Table 8: Summary logistic regression statistics for the decision rules used to predict the participants’ choice.

All of the decision rules significantly predict the decision made. FAO explains the most variance, followed by EW.
Next, we asked whether participant choices could be better predicted by combining attribute-based and associative models. Using hierarchical logistic regression, we added FAU at step 2 to MAU in one model, and to EW in another model. In both cases, FAU explained a significant amount of additional variance in participant choice. FAU explained an additional 12.7% of variance on MAU and an additional 12.1% of variance on EW.
4.2.2 Predicting Decision Satisfaction and Success at Time 1
To test how well each of the decision rules predicted decision satisfaction we recategorised the decision rule predictions into variables for chosen or unchosen options. We then used these as predictors in multiple regression with decision satisfaction and decision success as the outcome variables as in Experiment 1 (Table 9).
Table 9: Multiple regressions of decision rules predicting decision satisfaction and success at Time 1 in Experiment 3.
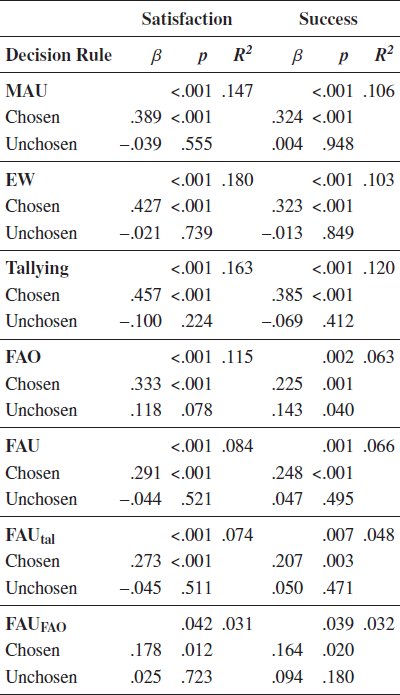
Note: p ≤ .001. for the constant in all models
The first option (TTF) was significantly more successful than the second (Option A: M = 5.52, s.d. = 1.18, Option B M = 5.18, s.d. = 1.13; t(203) = 1.99, p = .048).
Next, we asked whether the Free association method explained any variance in decision satisfaction and success in addition to the established MAU procedure using a hierarchical multiple regression. This analysis was conducted with both MAU and EW decision models. In both cases FAU explained a significant amount of additional variance in decision satisfaction. For satisfaction, FAU explained an additional 5.6% of variance than MAU alone, and an additional 5.8% of variance than EW alone. For success, FAU explained an additional 4.2% of variance than MAU alone, and an additional 4.5% of variance than EW alone.
Table 10: Hierarchical multiple regressions of decision rules predicting decision satisfaction and success at Time 1 in Experiment 3.

Note: p ≤ .001. for the constant in all models
Correlations were performed for the remaining items of the Decision Satisfaction Scale and are available in the supplementary materials. Confidence, Careful decision making, and Feeling informed were all positively associated with the chosen alternatives for the decision rules based on the attribute-based scores (MAU, EW, Tallying, and FAO), but were not associated with those based on the associative scores (FAU, FAUTAL, and FAUFAO).
4.2.3 Predicting choice at Time 2
Overall, 175 participants had made a valid choice at Time 2. This fell from the 205 participants at Time 1: 22 participants were lost to attrition (10.73%) and a further 8 due to reporting choices that were incompatible with their initial scenario. Participants who reported not having made the decision by Time 2 were removed from the Time 2 analysis. There was no longer a significant difference between the number participants who chose Option A (56.0%) and Option B (44.0%) (Wald = 2.537, df = 1, p = .111).
Next, we tested how well each decision rule predicted the participants’ choice at Time 2 (Table 11).
Table 11: Summary logistic regression statistics for the decision rules used to predict the participants’ choice at Time 2, Experiment 3.

Only the associative rules FAU and FAUtal rules significantly predicted the final decision. None of the attribute-based rules – MAU, EW, FAO and tallying – were significant predictors of choice at Time 2.
4.2.4 Predicting Decision Satisfaction and Success at Time 2
Next we tested how well each of the decision rules predicted decision satisfaction and success at Time 2 (Table 12).
Table 12: Multiple regressions of decision rules predicting decision satisfaction and success at Time 2 in Experiment 3.

Note: p ≤ .001. for the constant in all models.
All of the decision rules predicted satisfaction at Time 2 except FAO. FAU predicted the most variance. The first option (TTF) was significantly more satisfying than the second (Option A: M = 6.09, s.d. = 1.14, Option B: M = 5.66, s.d. = 1.36; t(173) = 2.27, p = .03). All of the decision rules significantly predict decision success. EW explains the most variance. The first option (TTF) was not significantly more successful than the second (Option A: M = 5.78, s.d. = 1.25, M = 5.48, s.d. = 1.27; t(173) = 1.59, p = .11).
Next, we asked whether the Free association method explained any variance in decision satisfaction and success in addition to the established MAU procedure. Using a hierarchical multiple regression, decision models based on MAU were entered at step 1 and FAU was entered at step 2. This analysis was conducted with both MAU and EW decision models. Whilst MAU is a significant predictor of Satisfaction at Time 2, it can still be significantly improved upon by including data from the free association task, with FAU explaining an additional 13.3% of variance. EW was similarly significantly improved upon, adding FAU to the model explained an additional 12.1% of variance. For Success, MAU was a significant predictor at Time 2, and can also still be significantly improved upon by including data from the free association task, with FAU explaining and additional 16.7% of variance. EW was similarly significantly improved upon, adding FAU to the model explained an additional 15.4% of variance (Table 13).
Table 13: Hierarchical multiple regressions of decision rules predicting decision satisfaction and success at Time 2 in Experiment 3.

Note: p ≤ the constant in all models.
Overall, the findings at Time 1 largely replicated Experiments 1 and 2. Attribute-based and associative rules were both equally effective at predicting choice and decision outcomes. Weighting attributes did not improve the rule beyond using equal weights. In this experiment tallying was not a notably less effective rule. The main aim of Experiment 3 though was to test the decision rules’ predictions longitudinally. Unlike Time 1, the choices made at Time 2 were significantly predicted by the associative rules but not the attribute-based rules. However, both attribute-based and associative rules were predictors of decision satisfaction and success at Time 2. Combining attribute-based and associative rules demonstrated that they explained different variance in the decision outcomes, and together they were better predictors of satisfaction and success than either individually.
Individual and Decision Characteristics
Combining data from all three experiments, we calculated the correlations between decision characteristics (frequency; knowledge; complexity; and importance) and participant characteristics derived from the Cognitive Reflection Task (Frederick, Reference Frederick2005), the Berlin Numeracy Test (Cokely et al., Reference Cokely, Galesic, Schulz, Ghazal and Garcia-Retamero2012), and the Rational Experiential Inventory (Epstein et al., Reference Epstein, Pacini, Denes-Raj and Heier1996) – which comprised of Need for Cognition (NFC) and Faith in Intuition (FII) (Table 14).
Table 14: Correlation of decision characteristics, participant characteristics, and decision outcomes for Experiments 1-3.

* p<.05
** = p<.01
Decision knowledge and decision frequency were positively correlated, and both were associated with increased satisfaction and success at both Time 1 and at Time 2, confidence, and feelings of being informed. Decision complexity and decision importance were positively correlated, and both were associated with lower decision knowledge and frequency. Both complexity and importance were positively correlated with care in making the decision and negatively correlated with feelings of being informed. Decision complexity was also associated with decreased satisfaction and success at Time 1 and Time 2, and confidence.
Of the participant characteristics, FII was positively correlated with satisfaction and success at Time 1 only, whilst the others (CRT, BNT, NFC) were not associated with these outcomes. Participants scoring higher on CRT and BNT felt less confident with their decisions, whilst those scoring higher on FII felt more confident. FII was positively associated with feelings of being informed, and both FII and CRT were positive associated with careful decision making.
Satisfaction and success were significantly associated with a medium correlation. This indicates that whilst they were positively associated – a successful decision is likely to be satisfying – they were not entirely overlapping constructs.
General Discussion
The aim of this study was to compare attribute-based and associative methods of decision making and investigate which processes best explains everyday decision making and best predicts the most successful outcome. We developed an everyday decision making task to elicit current, meaningful decisions, examine how these decisions are made, and predict which processes lead to the best outcomes. We compared decision rules based on an analysis of the attributes of the decision against decision rules based on associative knowledge elicited through a free association procedure. The attribute-based process was not better at explaining everyday decision making and predicting decision success than other decision rules. We also found that a tendency and ability to use an analytic thinking style did not lead to better decision outcomes. Decision outcomes were better predicted by characteristics of the decisions; namely the frequency, simplicity, and knowledge about the decision. Associative knowledge was as effective as rational, attribute-based decision rules. However, the best approach to everyday decision making was to combine attribute-based and associative cognition.
These findings challenge the view that the analytic, attribute-based approach is the best strategy to apply when making everyday decisions. Decisions with a higher multiattribute utility (MAU) did not predict choices or outcomes better than other, simpler attribute-based decision rules or the associative rules. This conflicts with research on naturalistic choice that found the weighted-additive decision rule (equivalent to MAU) to be more effective at predicting decisions than simpler rules (Bhatia & Stewart, Reference Bhatia and Stewart2018). However it is consistent with older research in which the simpler equal weights rule (EW) was as effective, suggesting that there is no additional benefit from the full analysis of the likelihood of attributes (Dawes & Corrigan, Reference Dawes and Corrigan1974; Dawes, Reference Dawes1979). This may be because many more high likelihood outcomes were reported than low likelihood (Table S1, supplementary materials) and the resulting narrow range means that the weighting of attributes did not greatly affect relative utility scores. In this case, focusing on high likelihood outcomes is an adaptive strategy as low likelihood items have little weight in MAU and omitting them simplifies the decision process.
A second line of evidence that challenges the view that the analytic approach is the best strategy for everyday decision making is the lack of association between the CRT, numeracy, need for cognition, and decision outcomes. This is surprising as the CRT in particular is positively associated with a wide range of measures of decision making and resistance to errors (e.g., Toplak, West & Stanovich, Reference Toplak, West and Stanovich2011). However, decisions in prior research are often compared against a normative standard that is based upon logical or mathematical principles, and so it is reasonable that the tendency and ability to engage logical and mathematical thinking will be beneficial in those situations. But the aim of this study of everyday decision making was to identify a successful outcome rather than a mathematical conclusion. The lack of association with analytic thinking suggests that a successful approach to everyday decision making does not involve a tendency to apply typical analytic frameworks.
The findings also challenge the view that associative knowledge leads to bias and poor decision making. Consistent with the logical intuitions dual process theory in which System 1 associative knowledge is effective and often logical (De Neys Reference De Neys2012; De Neys & Pennycook, Reference De Neys and Pennycook2019), we found that the associative rules performed at least as well as the best attribute-based decision rules both in explaining choice and predicting decision outcomes. Free association utility (FAU) was particularly successful in all experiments in both explaining choice and predicting outcomes. As the associative rules do not rely on explicit probabilities, they are well suited for decisions under uncertainty that is a characteristic of many everyday decisions. The decision characteristics correlated with decision outcomes – frequency and simplicity of the decision and knowledge about the decision – are also consistent with the effective use of associative knowledge as adaptive associations are more likely to be developed in predictable environments such as these (Kahneman & Klein, Reference Kahneman and Klein2009).
The best fitting model was neither the best attribute-based nor the best associative model independently. We found that associative and attribute-based rules explained different variance in the decision outcomes (perhaps only because the two predictors are subject to different sources of error). The best explanation of the choice made and the best predictor of decision outcomes was a combination of associative and attribute-based rules. However, what this study does not explain is how these two processes are integrated.
Associative and attribute-based processes could both be mapped onto a dual process account with associative knowledge related to a System 1, intuitive process, and the attribute-based knowledge related to a System 2, analytic process. Our instructions support this possibility. For the associative condition we ask participants to ‘write down the first thoughts or images that come to mind’ and our instructions for the attributes condition ask participants to ‘take a moment to think through the decision carefully and thoroughly’. Instructions such as these have been used to elicit intuitive and analytic thought respectively (e.g., Thompson, Turner & Pennycook, Reference Thompson, Turner and Pennycook2011). If associative and attribute-based processes correspond to System 1 and System 2 processes, respectively, their use can be explored by comparing alternative dual process theory explanations of everyday decision making. Alternatively, a decision maker may switch between them to find the optimal mix (Katsikopoulos et al., 2022).
We are also interested, practically, in which decision rules are most effective for everyday decision making. As discussed above, EW was an effective rule confirming research suggesting that is a robust rule in environments when the decision weights cannot be estimated with precision such as everyday decision making and is less complex to elicit than MAU (Dawes & Corrigan, Reference Dawes and Corrigan1974; Dawes, Reference Dawes1979). FAU is equally effective, although the free association method elicits different information. Tallying, similar to the everyday decision strategy of adding up the pros and cons of a decision, does predict decision satisfaction and success albeit less effectively that MAU, EW, and FAU. Examining only the first associate reported (FAUFAO) predicts decision outcomes whereas examining only the first attribute reported (FAUFAU) does not. The first attribute may be useful because associative knowledge is acquired through experience of repeated associations and so the strongest associate is likely to be the most frequently encountered and valid cue. The simplest strategy of taking the first option reported (TTF) accounted for 60% of choices. Although this was a significant trend towards choosing the first option reported, the 40% that chose the alternative indicates that many participants were not simply reporting a pre-formed or routine decision and generating an alternative without serious consideration. TTF resulted in higher satisfaction but not significantly higher success. It is a useful strategy for satisfaction in the choice but not to identify the most successful outcome. Using the sum of EW and FAU is the best fitting model.
Some aspects of the method limit the conclusions that can be drawn. First, in this task participants generate their own options to consider. How these options are generated is in itself an interesting process that is likely to influence the success of the decision outcome (Johnson & Raab, Reference Johnson and Raab2003). It is possible that option generation is an associative process, and this may or may not be an effective way of identifying options. Options generated associatively may be evaluated more positively when using associative knowledge. This is not inherently a bias as associative knowledge may cue options that are more likely to be successful for the individual. We used this feature of the task to test the Take the First heuristic. We found that there was a preference for the first option generated, as predicted by the heuristic, but at Time 2, whilst this option was more satisfying it was not significantly more successful, contrary to the heuristic predictions. This mixed support for Take the First suggests further research is required to understand the process of successful option generation. However, our experimental paradigm is different to the case where options are presented to decision makers, for example choosing a car from a showroom or a dish from a menu. In these cases heuristics such as Take the First cannot be used and associations cannot be used to cue personally meaningful options. In these decisions, the evaluation of the options may still draw on attribute-based and associative knowledge, but the overall decision process may be different. Future research could investigate these two types of everyday decisions separately to discover where differences lie.
Second, ratings of decision satisfaction and success were used as outcome measures. These are subjective and the rating of an option may have been influenced by the act of choosing it, e.g., inflating ratings to reduce cognitive dissonance (Brehm, Reference Brehm1956; Hornsby & Love, Reference Hornsby and Love2020). It may be that the consequence of this effect is to restrict the range in the outcome measure and lead to an underestimate of predictive value of the decision models so that the true effect may be larger than reported here. Also, the nature of the decision will influence how accurately the decision outcome can be judged after one week. Smaller decisions, e.g. choosing a meal, will be easier to judge with certainty than larger decisions, e.g. whether to move house, that have longer term ramifications. In some cases an objective measure of decision success would be preferable. In many everyday decisions though the satisfaction and perceived success of an option is an inherently subjective construct as many everyday decisions reported (Shall I get married? Shall I have a baby?) do not have an objectively correct solution. Future research will benefit from improving methods to measure the success of everyday decision outcomes.
Third, we have not examined all possible models that could be applied to everyday decision making. Alternative multi-criteria analyses could be conducted along with alternative methods for eliciting and combining attributes (e.g., Edwards & Barron, Reference Edwards and Barron1994; Durbach & Stewart, Reference Durbach and Stewart2009). A wider range of simple heuristics, e.g., Take the Best, could be tested (Gigerenzer & Goldstein, Reference Edwards and Barron1996). Applying these models to the everyday decision making task will provide an informative test of their effectiveness in real-world decisions. Future research could test if they explain everyday choices better than the models tested here and if they predict successful outcomes more effectively.
The everyday decision making task is a fruitful approach to studying decision making. Rather than experimenter-led decisions, it is interesting to note the areas that the general population choose to report as meaningful, everyday decisions that they are facing, summarised in Table 1. The general topics cover the range of everyday scenarios, but some areas are clearly more frequently considered than others and some important topics such as health and finance and comparatively infrequent. The decisions reported varied in their importance, complexity, and frequency of repeating the decision and knowledge about it. Nonetheless, it seems that similar strategies are used across these different characteristics although more research is required on these moderating factors.
The aim of everyday decision making is to choose an option that is satisfying and successful. The present study demonstrates that the best approach for doing this is neither to apply an analytic strategy nor to simply rely on experience-based associations. The best explanation of everyday decisions and the best predictor of decision outcomes may depend on the integration of both sources.