1. Introduction
Even though spoken languages contain thousands of words and unique segment combinations, some sounds and gestures just seem to fit certain meanings. This iconic resemblance between aspects of form and aspects of meaning (Winter & Perlman, Reference Winter and Perlman2021) is well-documented across both natural spoken and signed languages (Dingemanse et al., Reference Dingemanse, Blasi, Lupyan, Christiansen and Monaghan2015; Perniss & Vigliocco, Reference Perniss and Vigliocco2014). Semantic domains in which vocal iconicity is often present also constitute much of the semantic basis for nominal classification systems, that is, the way languages categorize nouns grammatically. In this explorative study, we investigate whether overrepresentations of specific sound types could be found for semantic categories that are common in nominal classification systems using a cross-linguistic approach.
1.1 Lexical vocal iconicity
For almost 100 years, experiments have been conducted showing preferences between certain sounds and meanings. This started with Sapir’s (Reference Sapir1929) experimental study where a majority of participants preferred to associate a small table with a form such as /mil/ and a large table with a form such as /mal/. Contemporaneously, Köhler (Reference Köhler1929) found that when asking participants to match an amoeba-like shape and a star-like shape with either /takete/ or /baluma/ (later replaced by /maluma/), most of them thought that the best-fitting name for the round shape was the word containing voiced sounds and rounded vowels, while the pointy shape was paired with the word containing unvoiced sounds and unrounded vowels. These studies have later been reconfirmed and expanded upon (Styles & Gawne, Reference Styles and Gawne2017) and new types of experiments have shown, for example, that iconicity can naturally emerge through communication between participants (Erben Johansson et al., Reference Erben Johansson, Carr and Kirby2021; Perlman et al., Reference Perlman, Dale and Lupyan2015) and that human vocalization intended to iconically imitate various concepts can be understood cross-culturally (Ćwiek et al., Reference Ćwiek, Fuchs, Draxler, Asu, Dediu, Hiovain, Kawahara, Koutalidis, Krifka, Lippus, Lupyan, Oh, Paul, Petrone, Ridouane, Reiter, Schümchen, Szalontai, Ünal-Logacev and Perlman2021).
More recently, the increased accessibility of language descriptions from lesser-known language families, together with digitization and new statistical models, has enabled a number of large-scale cross-linguistic studies to find consistent overrepresentations of sounds and phonetic features in specific meanings (Blasi et al., Reference Blasi, Wichmann, Hammarström, Stadler and Christiansen2016; Erben Johansson et al., Reference Erben Johansson, Anikin, Carling and Holmer2020; Joo, Reference Joo2020; Wichmann et al., Reference Wichmann, Holman and Brown2010). These studies usually involve lexical data consisting of what are considered basic vocabulary words, that is, concepts that are assumed to be culturally neutral, in order to produce consistent comparisons. Recent studies also investigated iconicity within the general vocabulary of individual languages, showing that size and shape iconicity is found throughout at least the English lexicon (Sidhu et al., Reference Sidhu, Westbury, Hollis and Pexman2021; Winter & Perlman, Reference Winter and Perlman2021) and since the associated sounds and meanings correspond to previously established experimental and cross-linguistic findings, it is likely that other languages incorporate iconicity in a similar manner. Many languages around the world also possess large sets of structurally marked words used to depict sensory imagery, usually referred to as ideophones, whose inventories are typically on par with nouns and verbs (Dingemanse, Reference Dingemanse2018).
Altogether, lexical vocal iconicity appears to be common in the sensory domain (Sidhu & Pexman, Reference Sidhu and Pexman2018; Winter et al., Reference Winter, Perlman, Perry and Lupyan2017), but in cross-linguistically comparable concepts, body parts (nose, tongue), bodily functions (to bite, to yawn), deictic words (including personal pronouns), kinship terms (mother, father), descriptive words (small, round), and natural entities (leaf, ash) seem to be particularly prominent. These findings could partially be dependent on the concepts included in basic vocabulary lists and in forced choice experimental setups, for example, small vs. big or pointy vs. round, with the notable exception of iconicity in color words (Anikin & Johansson, Reference Anikin and Johansson2019). Nevertheless, these studies point to the semantic domains which commonly involve iconic associations.
1.2 Grammaticalized vocal iconicity
Iconicity has also been proposed to influence the general structure of language (Croft, Reference Croft2003, p. 102; Haiman, Reference Haiman1983; Haspelmath, Reference Haspelmath2008), but the presence of more concrete and direct mappings between specific sounds and meanings in morphology is less studied. Since morphemes are the smallest meaning-bearing unit of language, they allow for vocal iconicity, whereby phonetic material is directly connected to semantics via iconicity. A related phenomenon is phonesthemes, phonemes or phoneme clusters corresponding to specific meanings (Kwon & Round, Reference Kwon and Round2015), which are very similar to “regular” vocal iconicity. Like ideophones, phonesthemes can be language- and family branch-specific, such as English initial /gl-/ in glitter and gleam being associated with light or vision, but others also involve cross-linguistic vocal iconicity, such as word-final /-nk/ being associated with resonant sounds that are cut short (Kwon, Reference Kwon2016; Mompean et al., Reference Mompean, Fregier and Valenzuela2020). Evaluative morphology, such as diminutives and augmentatives, has also been investigated from the perspective of vocal iconicity, but the results have been inconclusive. There seems to be a tendency for diminutives to contain high-frequency vowels and consonants, and augmentatives to contain low-frequency vowels, backed up by some cross-linguistic evidence (Ultan, Reference Ultan and Greenberg1978). Some studies suggest that no reliable associations can be found (Bauer, Reference Bauer1996; Gregová et al., Reference Gregová, Körtvélyessy and Zimmermann2010; Körtvélyessy, Reference Körtvélyessy2011), but the language samples used in these are rather small, including only a few language families, and the studies were aimed at testing a rather restrictive hypothesis of contrastive associations between high front vowels (and post-alveolar and palatal consonants) in diminutives on one side, and high back vowels in augmentatives on the other.
Nominal classification, that is, grammatical systems that classify nominal referents using nominal classification devices, involves similarly fundamental semantic categories. These systems are very heterogeneous and can include everything from categories based on biological sex (feminine/masculine), humanness (human/non-human), and animacy (animate/inanimate) to physical properties (e.g., size, shape) and functional properties (e.g., container, tool). Thus, many of the categories which were found to be iconic in previous studies are grammatically encoded in nominal classification systems. While some nominal classification systems include no phonological cues for distinguishing between nominal classes (Kraaikamp, Reference Kraaikamp2012; Vos & Vogelaer, Reference Vos and Vogelaer2011), Nastase and Popescu (Reference Nastase and Popescu2009) showed that German and Romanian masculine, feminine, and neuter inflections could be deduced from their word forms and Sidhu and Pexman (Reference Sidhu and Pexman2015) found gender iconicity in English first names. Arguably, nominal classification provides a fertile ground for investigating vocal iconicity.
1.3 Nominal classification: an overview
Nominal classification covers phenomena as diverse as grammatical gender in German [stan1295] (brackets following language names indicate Glottolog codes used for languoid identification), noun classes in Swahili [swah1253], and numeral classifiers in Mandarin Chinese [mand1415]. Seifart (Reference Seifart2010, p. 719) defines nominal classification systems in the following way:
-
1. Nouns collocate in well-defined grammatical environments with classificatory elements (these may be free forms, clitics, affixes, etc., and these may also occur elsewhere).
-
2. The number of classificatory elements is larger than 1, but significantly smaller than the number of nouns.
-
3. Classificatory elements show different patterns of collocation with nouns, that is, they impose a classification (some overlap is allowed; prototypically, there is a relatively equal division of the nominal lexicon by classificatory elements).
-
4. At least a substantial subpart of nouns is classified in this way.
With the recent increase in descriptions of nominal classification systems of previously less well-described languages, it has become evident that there are reasons for treating all nominal classification systems as a unified phenomenon (Aikhenvald, Reference Aikhenvald2000; Corbett & Fedden, Reference Corbett and Fedden2016, p. 496; Grinevald, Reference Grinevald and Senft2000). For example, many Amazonian languages have nominal classification systems that share properties with both (prototypical) noun class and numeral classifiers systems (Grinevald & Seifart, Reference Grinevald and Seifart2004). Viewing nominal classification as a continuum avoids forcing such “intermediary” systems into either one or the other type. However, we can identify certain clusters along the continuum and it can be meaningful to draw lines between these clusters. In the literature on nominal classification, a distinction is commonly made based on the presence or absence of agreement, that is, marking of grammatical category values across multiple words (Aikhenvald, Reference Aikhenvald2000, p. 20; Corbett, Reference Corbett1991; Kilarski, Reference Kilarski2013, p. 10), which is the distinction criterion we have adopted in this study.
Agreement correlates with a high degree of grammaticalization, that is, mechanisms causing lexical sources to change into more grammatical linguistic units through semantic bleaching, loss of morphosyntactic properties, and phonetic erosion (Heine, Reference Heine, Joseph and Janda2004). For nominal classification systems specifically, Lehmann (Reference Lehmann2015, p. 63ff) mentions the following as signs of more grammaticalized systems: obligatory marking, morphosyntactic bondedness, reduction of the number of distinctions, phonetic reduction and sandhi phenomena, paradigmatic irregularity (allomorphy, cumulative expression, suppletion), semantic opaqueness, and decreasing assignment flexibility. It has been attested that nouns can develop into nominal class markers, and it has been hypothesized that less grammaticalized nominal classification systems may develop into more grammaticalized types (Franjieh et al., Reference Franjieh, Corbett and Grandison2020; Grinevald & Seifart, Reference Grinevald and Seifart2004; Passer, Reference Passer2016). In general terms, on a grammaticalization cline of nominal classification systems, we would place the prototypical agreeing system closer to the grammatical end and the prototypical non-agreeing system closer to the lexical end.
Agreeing systems include most systems traditionally labelled as “gender” or “noun class” systems. Even though the sole criterion of agreement can motivate inclusion in this group, these systems typically have other properties in common: they generally contain a fairly small number of distinctions (from two to a couple of dozen), each noun typically belongs to exactly one class, and the nominal classification devices are typically morphosyntactically bound, realized as, for example, affixes or clitics (Seifart, Reference Seifart2010, p. 720). An example of an agreeing system is the Spanish grammatical gender system [stan1288], including a two-way distinction (masculine/feminine) marked on, for example, articles and adjectives: la casa blanca ‘the white house’ vs. el libro blanco ‘the white book’.
Non-agreeing systems include most systems traditionally labelled ‘classifier’ systems. This group comprises a fairly diverse set of systems, which are often divided into a typology based on the morphosyntactic locus of the classifier morphemes: numeral classifiers, noun classifiers, and verbal classifiers, as well as further semantically- and syntactically-based distinctions such as sortal and mensural classifiers (Aikhenvald, Reference Aikhenvald and Brown2006, Reference Aikhenvald, Aikhenvald and Dixon2017; Grinevald, Reference Grinevald and Senft2000). What non-agreeing systems have in common is that the number of distinctions typically is higher (from a couple of dozen to a couple of hundred), and that nominal classification devices tend to be assigned to nouns on a more flexible and context-dependent basis (Seifart, Reference Seifart2010, p. 72ff). An example of a non-agreeing system is the numeral classifier system of Iu Mien [iumi1238], comprising around a hundred nominal classification devices which primarily encode physical properties of nominal referents (Arisawa, Reference Arisawa2016). In example (1), we see that the same noun can be used with different classifiers, resulting in different meanings.

Except for some agreeing systems that, to a great extent, are centered around phonological or morphological cues, nominal classification is primarily constructed around some sort of semantic basis (Aikhenvald, Reference Aikhenvald2000, p. 22ff, Reference Aikhenvald, Aikhenvald and Dixon2017). This basis consists of a set of ‘core parameters’ (Aikhenvald, Reference Aikhenvald and Brown2006, p. 468; Denny, Reference Denny1976), namely (a) animacy, (b) physical properties (e.g., shape, dimensionality, direction, size, consistency, material), (c) functional properties (including social status), and (d) arrangement (i.e., configuration). The universal relevance of these parameters can be explained by the fact that they have a high cue validity, that is, that they serve as good predictors of other features (Rosch et al., Reference Rosch, Gray, Johnson, Boyes-Braem and Mervis1976; Seifart, Reference Seifart2010, pp. 725–726). Certain semantic categories are also more likely to show up in some nominal classification systems than others. For example, animacy, humanness, and biological sex are preferred by agreeing systems, whereas physical properties, such as shape, seem to be more dominant in the non-agreeing systems. However, one crucial difference between agreeing and non-agreeing systems is that class assignment in the latter tends to be more semantically transparent than in the former (Kilarski, Reference Kilarski2013, p. 12). For example, the ‘book’ and ‘flat’ classifiers in the non-agreeing Iu Mien system are consistent with observable properties of the referents in question, example (1), whereas there is nothing observably ‘feminine’ about houses or ‘masculine’ about books, as in the agreeing Spanish grammatical gender system.
1.4 Research questions
Although nominal classification is not universally present in languages, it is a rather widespread phenomenon which is also based on semantics. The semantic basis of these categories, however, tends to become less transparent the more a system is grammaticalized. Since it is generally agreed that vocal iconicity is present in words that belong to similar semantic categories, nominal classification devices are promising subjects for vocal iconicity research. If iconicity can be detected in nominal classification systems, it would be reasonable to assume that it affects less grammaticalized systems to a higher degree, as the nominal classes of such systems remain more transparent and semantically meaningful. The following research questions were formulated for the present study:
-
1. Can the presence of cross-linguistic vocal iconicity be established in nominal classification devices?
-
2. If yes, is the vocal iconicity found in both non-agreeing and agreeing systems?
2. Method
2.1 Language sampling
Languages were selected to represent as much linguistic diversity as possible by including at least one representative from each language family with relevant and attainable data. According to Glottolog (Hammarström et al., Reference Hammarström, Forkel, Haspelmath and Bank2021), there are 425 identified language families. Languages classified as “artificial”, “bookkeeping”, “unattested”, “mixed”, “pidgins”, “speech register”, and “unclassifiable” had to be immediately excluded because they were either constructed, administrative artifacts, unattested, or difficult to classify. Despite the considerable amount of iconicity found in signed languages, we also had to exclude these given our focus on specifically vocal iconicity. Out of the remaining families, 212 were found to have at least one language with a nominal classification system. Naturally, not all of the languages from the remaining 205 language families necessarily lack nominal classification systems, but not enough comprehensive data were found for them at the time of data collection.
Although it would be preferable to control for genetic bias completely by only including one language per language family, including more than 212 languages was deemed necessary to capture a greater share of the semantic diversity encoded across nominal classification systems. Therefore, large, diachronically well-understood language families could be represented by one language from each primary branch as long as the selected languages possessed nominal classification devices which were obviously phonologically and structurally distinct from the nominal classification devices in the other selected languages from the same family. For example, Indo-European could include Spanish [stan1288] masculine /el/ and feminine /la/, Eastern Armenian [nucl1235] generic /haṭ/ and human /hokʿi/ classifiers, Hittite [hitt1242] animate /-aš/, /-š/ and inanimate /-an/, /-∅/, and Swedish [swed1254] common /-en/, /-n/ and neuter /-et/, /-t/.
Several large language families had one dominating primary branch which included most of the families’ languages, for example, the Volta-Congo branch of the Atlantic-Congo family includes 1,338 of the family’s 1,403 languages. For these language families, each primary branch, as well as each primary subbranch of the dominating primary branch, was represented. In the present sample, these include Atlantic-Congo (Volta-Congo), Austronesian (Malayo-Polynesian), Angan (Nuclear Angan), North Halmahera (Northern North Halmahera), Sino-Tibetan (Burmo-Qiangic), Tai-Kadai (Kam-Tai), Timor-Alor-Pantar (Alor-Pantar), Tucanoan (Eastern Tucanoan), and Khoe-Kwadi (Khoe). In three cases, the internal language family structure of one dominating branch is also found one level further down in the primary subbranches. For example, the Algic language family includes 46 languages, one of its primary branches includes 44 languages (Algonquian-Blackfoot) and its primary subbranch includes 43 languages (Algonquian). All subbranches from all of these levels could be included, just as the Athabaskan subbranch of the Athabaskan-Eyak branch in the Athabaskan-Eyak-Tlingit family and the Indo-Iranian subbranch of the Classical Indo-European branch in the Indo-European language family. In two language families, there were only two primary branches which, in turn, were binarily split among the subbranches. In these cases, both of the subbranches from each primary branch were included when data were available. The affected families were Otomanguean (Eastern Otomanguean and Western Otomanguean) and Uto-Aztecan (Northern Uto-Aztecan and Southern Uto-Aztecan).
The final sample resulted in 344 languages, of which 210 (61.1%) had non-agreeing systems (126 language families) and 151 (43.9%) had agreeing systems (123 language families), aligning rather closely with global distribution between the two main system types: 57.9% non-agreeing and 49.5% agreeing (Allassonnière-Tang et al., Reference Allassonnière-Tang, Lundgren, Robbers, Cronhamn, Larsson, Her, Hammarström and Carling2021). The same language could be included in both the agreeing nominal classification device and non-agreeing nominal classification device samples if it had clearly parallel but unrelated systems. For example, Ayoreo [ayor1240] combines a system of classifiers which contrasts plants, property, pets, and vehicles and haul with a typical grammatical gender system which contrasts masculine and feminine. This accounts for the discrepancy between the 210 non-agreeing and 151 agreeing systems adding up to more than the 344 sampled languages.
2.2 Data collection
The data from the sampled languages were directly gathered from primary sources consisting of language descriptions in the shape of grammar and journal articles. The presence or absence of agreement in the nominal classification systems was for the most part clearly stated in the source material, but in the few cases where this was unclear, additional language descriptions and glossed text material was consulted.
The agreeing nominal classification devices occurred in a number of different grammatical environments. Most of these were directly attached to nouns, but a lot of the data also includes morphemes marked on other types of words. We wanted to avoid including entire agreement paradigms for single nominal classes from languages which had particularly rich systems of agreement markers since these could blur out potential sound-meaning associations. Therefore, only the most overtly marked agreeing nominal classification devices for each language were included, that is, affixes/clitics directly connected to the noun, followed by articles (which modify the noun but are not unbound morphemes), and subject-related morphemes marked on the verb (which corresponds to, e.g., nominative). Markings on independent pronouns, adjectives, demonstratives, and object-related morphemes were neither included, and nor were in plural forms. Non-agreeing nominal classification devices included a wide range of classifiers, such as general noun and verbal classifiers, as well as numeral, measure, possessive, deictic, and locative classifiers. Only singular versions were collected, except for nominal classification devices which denoted groups of entities rather than plurality.
2.3 Data transcription and phonetic categorization
Following Erben Johansson et al. (Reference Erben Johansson, Anikin, Carling and Holmer2020), the data were transcribed in a way which was specifically designed to be used for statistical measuring of vocal iconic associations from a cross-linguistic perspective. The resulting segments were then categorized into sound groups to account for the fact that sound-meaning associations are, cross-linguistically speaking, seldom restricted to specific phonemes, but rather based on underlying features. Tables 1 and 2 show the phonological categories for vowels and consonants, respectively. Optional segments in the sampled forms were included, that is, /(pə)suog/ was treated as /pəsuog/. Lexical tones and stress patterns were removed, and syllabic, tense, and creaky consonants were transcribed as regular versions of the same segments. Long segments, diphthongs, and affricates, such as /a:/, /a͡ɪ/ and /d͡ʒ/, were transcribed as two separate segments, such as /aa/, /aɪ/ and /dʒ/, and aspiration, labialization and other secondary articulations, such as /tsʰ/ and /kʷ/ were transcribed as independent segments, such as /thsh/ and /kw/. Devoiced versions of normally voiced consonants, such as /w̥/, /ɡ̊/ and /b̥/, were transcribed as voiceless counterparts, such as /ʍ/, /k/ and /p/, and laminal consonants, such as /s̻/, were transcribed as regular alveolars, such as /s/. In a few instances, this meant that two phonologically distinct forms were rendered identical. For example, Mandarin Chinese [mand1415] /jiā/ and /jià/ were both transcribed as /tɕja/. Vowels were divided according to their main articulatory dimensions and into aggregated combinations of these, and consonants were divided according to manner, place and voicing, as well as combinations.
Table 1. Main articulatory dimensions for vowels
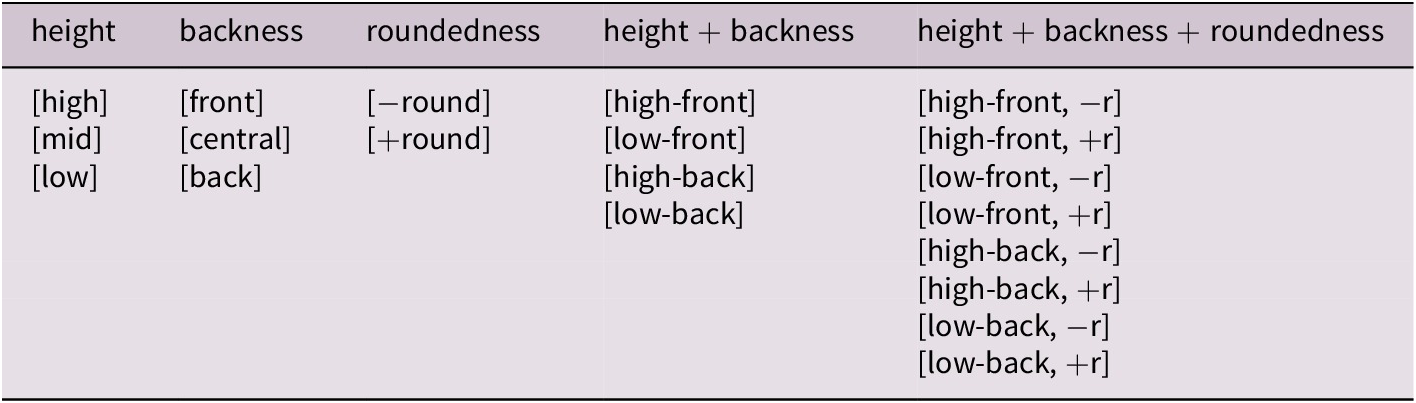
Note: This includes height, backness, roundedness and aggregated combinations of height and backness, and height, backness and roundedness respectively. Unrounded is noted as [−r] and rounded as [+r].
Table 2. Main articulatory dimensions for consonants
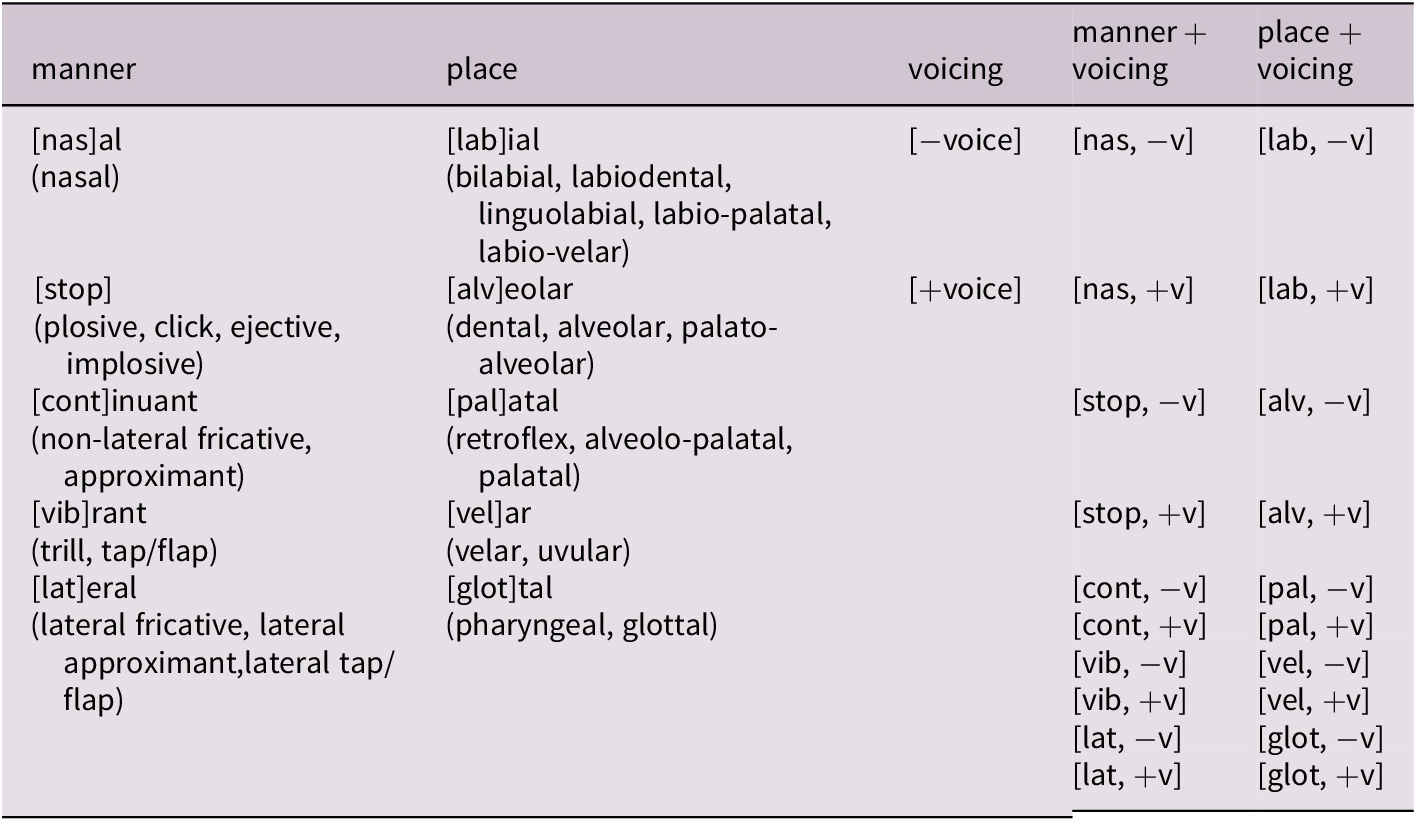
Note: This includes manner, place, voicing and aggregated combinations manner and voicing, and place and voicing respectively. Voiceless is noted as [−v] and voiced as [+v].
2.4 Semantic categorization
Nominal classification categories listed by the nominal classification literature dedicated to semantics were considered (Aikhenvald, Reference Aikhenvald2000, pp. 271–306, Reference Aikhenvald and Brown2006). Semantic domains and categories with global spread or spread across large geographical areas, such as Australia, Oceania, the Amazon, the Caucasus, North America, and Sub-Saharan Africa, were then selected to be used for the analysis, except for cultural-specific categories that usually pertained to social structure (Aikhenvald, Reference Aikhenvald2000, p. 292, Reference Aikhenvald and Brown2006, p. 470). The description of the semantic range of nominal classes can vary considerably depending on language, tradition, and author. Since we were unable to rely on native speakers for obtaining in-depth usage information, the semantic categories used in this study, along with the actual categorization of each form, were based on literal interpretations of the source material.
The aim was to categorize each nominal classification device in the sample using as few categories as possible. The most straightforward type were categorical descriptions: ‘classifier for round things’ was classified as round and ‘classifier for flat and flexible things’ was classified as flat and flexible. Many nominal classes, especially those regarding physical characteristics, were described using a set of referent nouns. Consequently, classes could be categorized using obvious common semantic denominators among the referent nouns if the semantic denominators corresponded to the selected semantic categories: ‘classifier for head, taro, garlic’ was classified as round and ‘classifier for all edible roots, tubers, shoots, flowers and fruits’ was classified as consumable. Specific noun referents, often followed by “-like” or similar, that were used as an example for a set of noun referents (Aikhenvald, Reference Aikhenvald and Brown2006, p. 469), were also employed for the categorization. For example, stick-like, pole-like, stem-like, string-like, and rope-like for long and one-dimensional objects, sheet-like, cloth-like, disc-like, and leaf-like for flat and two-dimensional objects, and seed-like for small objects. Descriptions of nominal classes which did not fit any of the categories based on these described criteria, including classes for specific referents, such as classes only used for eggs or fingers, as well as classes that were described through a set of referent nouns which did not share any semantic common denominator that corresponded to the selected semantic categories, were categorized as unclear. Obvious loans were also categorized as unclear.
While the categorization of typical grammatical gender systems (masculine vs. feminine or human vs. non-human) tended to be rather unambiguous, when the semantic descriptions were very broad, some nominal classes had to be categorized using several categories. For example, the so-called “vegetable gender” in Giimbiyu [mang1382] which categorizes plants and their parts, some weapons, some body parts and fire was categorized as flora, fire, consumable, and body. There were instances when semantic features were disregarded. For example, a classifier for ‘small water courses’ was categorized as liquid and not as small because it is unclear whether the size is a salient semantic feature for the classifier, that is, it does not seem to refer to small things other than water courses. This, in combination with variance in the descriptions of the nominal classification devices depending on language and source, lead to a rather restrictive approach to the categorization, which, in turn, also led to some data loss. When several forms were given for the same semantic scope, they were treated as variants of the same category, similarly to how he, she, and it are all versions of third person singular pronouns in English.
The semantic categories, found in Table 3, included contrasts based on sex, animacy, humanness, shape, size, consistency, nature, arrangement, quanta, material, orientation, food, concreteness, the body, as well as a generic category. Common gender was categorized as feminine and masculine, non-masculine as feminine and neuter, and non-feminine as masculine and neuter. Complex massifier categories were categorized as combinations of features, such as ‘groups of people’ resulting in mass and human and ‘bundles of sticks’ in bunch and long. Semantically, similar categories were aggregated in order to create extended categories which also added more data for the statistical analysis.
Table 3. Semantic categories, examples of included nominal classes and corresponding extended semantic categories
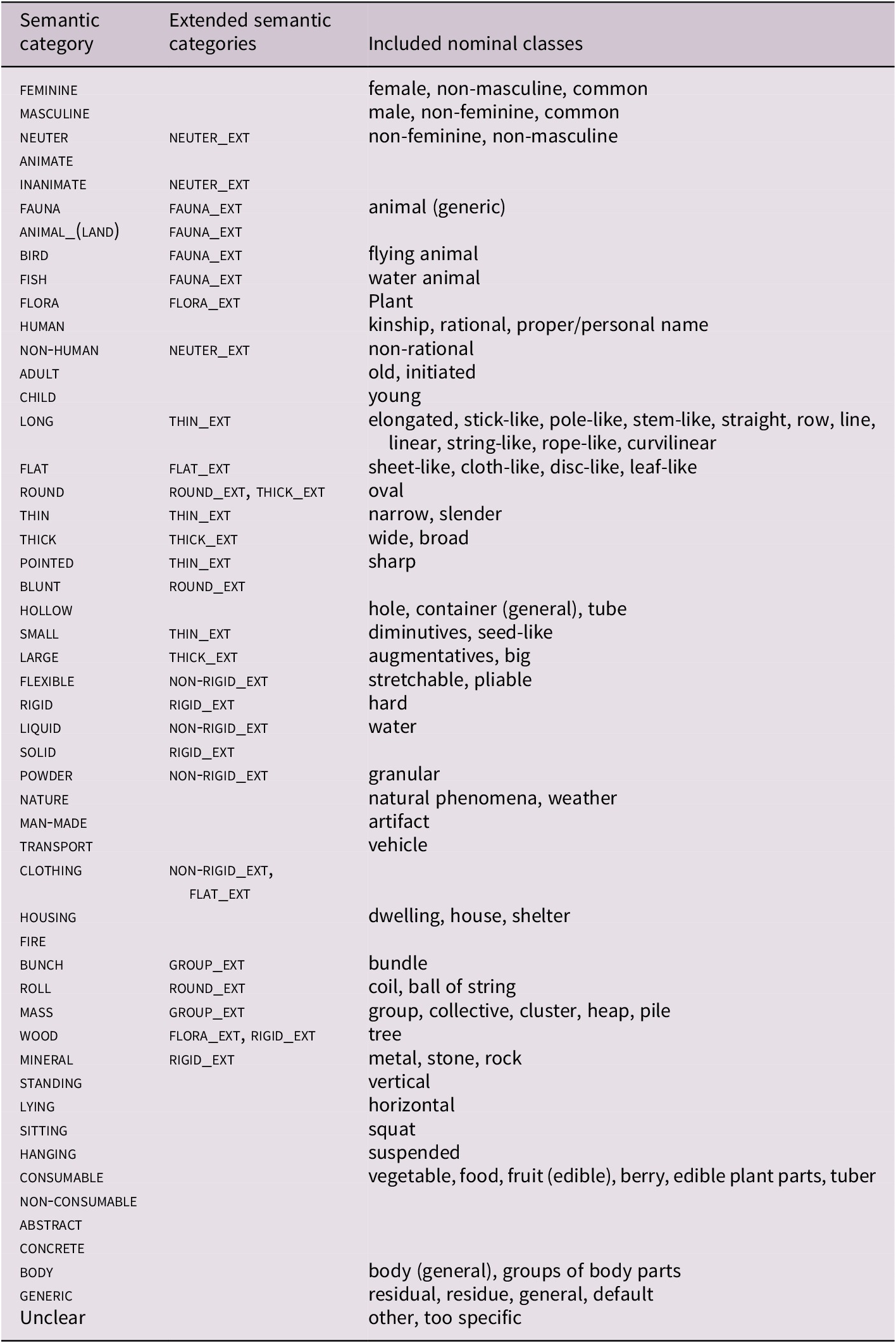
Both authors independently coded the entire dataset using the selected categories and categorization criteria without being able to see the phonological forms of the nominal classification devices. The two sets of categorizations were then compared and only the matching values were used for the data analysis. Out of the 4,037 nominal classification devices, 2,140 were categorized as unclear because there was no overlap between the assigned semantic categories by the raters. Since the nominal classification devices could be assigned to more than one semantic category, the remaining 1,897 nominal classification devices resulted in 4,422 semantic ratings. These 4,422 ratings represented an 81% overlap between the two raters and were used for the analysis. All nominal classification devices deemed as unclear were also added to the analysis, with the expectation that this residual category would not produce any noteworthy results. Category occurrence and semantic clustering of categories are found in Fig. 1.
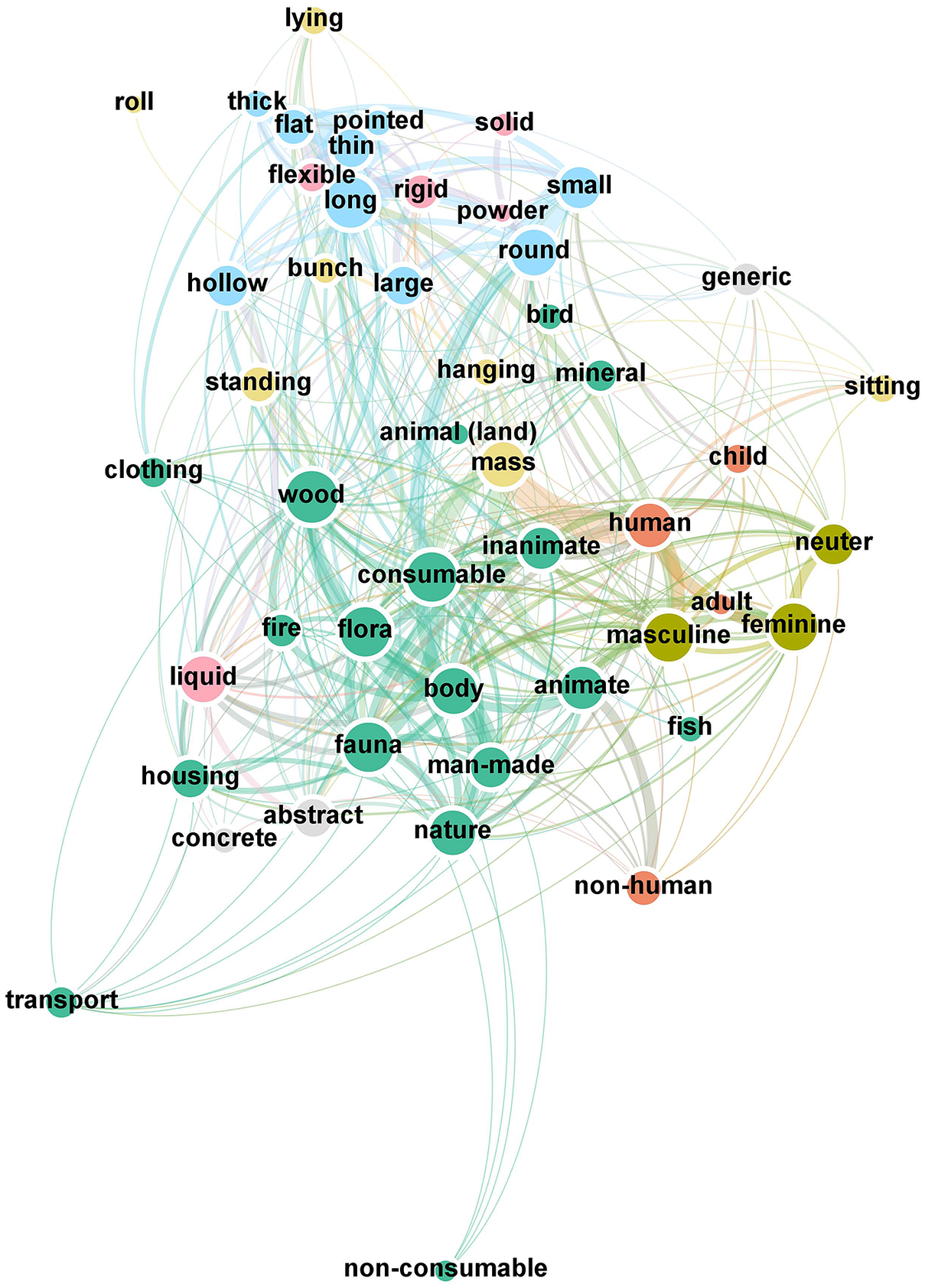
Fig. 1. Network based on category co-occurrence within the same nominal classification device across the overlapping dataset (excluding unclear). Node size represents the occurrence of categories and line thickness represents the occurrence of co-categorizations. Node color represents which semantic domain the categories belong to: light cyan, ~shape/size; pink, ~consistency; light yellow, ~arrangement/orientation; olive, sex; orange, humanness; mint, ~animacy; gray, other. Line colors are mixes of the colors of the connected nodes.
2.5 Statistical analysis
The data analysis was aimed at identifying overrepresented sound groups among the analyzed semantic categories across the sampled languages. Just as Erben Johansson et al. (Reference Erben Johansson, Anikin, Carling and Holmer2020), we interpreted markedly higher proportions of a specific sound group in any of the semantic categories as evidence for vocal iconicity. This was achieved by means of modeling the class of each phoneme – for example, the type of each vowel in a particular word as front / central / back – with multi-logistic regression in the framework of Bayesian mixed models as implemented in R 4.2.1 (R Core Team, 2022) and the package brms version 2.17.0 (Bürkner, Reference Bürkner2017). Supplementary Material and Appendices with R scripts are available at https://osf.io/7vhc8/.
The model included a population-level intercept corresponding to the overall distribution of sounds from the modelled category (fitted with a mildly conservative normal(0, 10) prior) and group-level (random) intercepts per language, language family, region, and semantic category. This random intercept per semantic category was the measure of interest, as it showed deviations from the typical distribution.
Fitted values from this model provided a list of fitted proportions of sound groups for each semantic category, and cases of over- or underrepresentations were identified by extracting fitted average proportions of each sound group (e.g., high vowels) across all semantic categories and then comparing estimates per semantic category to these average values. This comparison was performed for each step in the Markov chain Monte Carlo, resulting in a posterior distribution of deviation from typical distribution of sound groups. Lastly, since directly taking simple differences of proportions between sound groups does not scale well when the proportions are close to 0% or 100%, we instead compared odds ratios (OR): an increase from 5% to 10% corresponds to OR = 1:9/1:19 = 2.1, while an increase from 50% to 55% gives OR = 11:9/1:1 = 1.2. We then defined a region of practical equivalence (ROPE) (Kruschke & Liddell, Reference Kruschke and Liddell2018), symmetric on a logarithmic scale, around the null effect of no overrepresentation as a threshold for sound group overrepresentation (log-odds ratio = 0 or, equivalently, OR = 1). The width of the ROPE corresponded to a change of OR by a factor of 1.25, 1 * 1.25 = 1.25, or +25%; 1/1.25 = 0.8, or −20%). The results were divided as follows:
-
1. If the 95% credible interval (CI) for the OR fell completely outside the ROPE, this was considered a “strong association”.
-
2. If the 95% CI was completely contained inside the ROPE, this was considered “no association”.
-
3. If the 95% CI partly overlapped with the ROPE, the result was treated as ambiguous, this was considered a “weak association”, but potentially interesting.
3. Results
A full account of over- and underrepresentations broken down by sound parameter is found in Fig. 2. The analysis of the full dataset resulted in 20 strong and 72 weak associations (overrepresentations). The non-agreeing subset generated 10 strong and 34 weak associations, while the agreeing subset only produced 1 weak association, Table 4. Taken together, the full dataset and the non-agreeing subset produced similar associations which suggest that the non-agreeing languages were the main driving force behind the results. Most associations were found in the vowel sound groups. Since most consonant sound groups belong to sound parameters containing more levels, for example manner combined with voicing has 10 levels, this is likely a result of shrinkage imposed by the statistical model. It is therefore possible that more consonant associations are present in the data, but not detected.
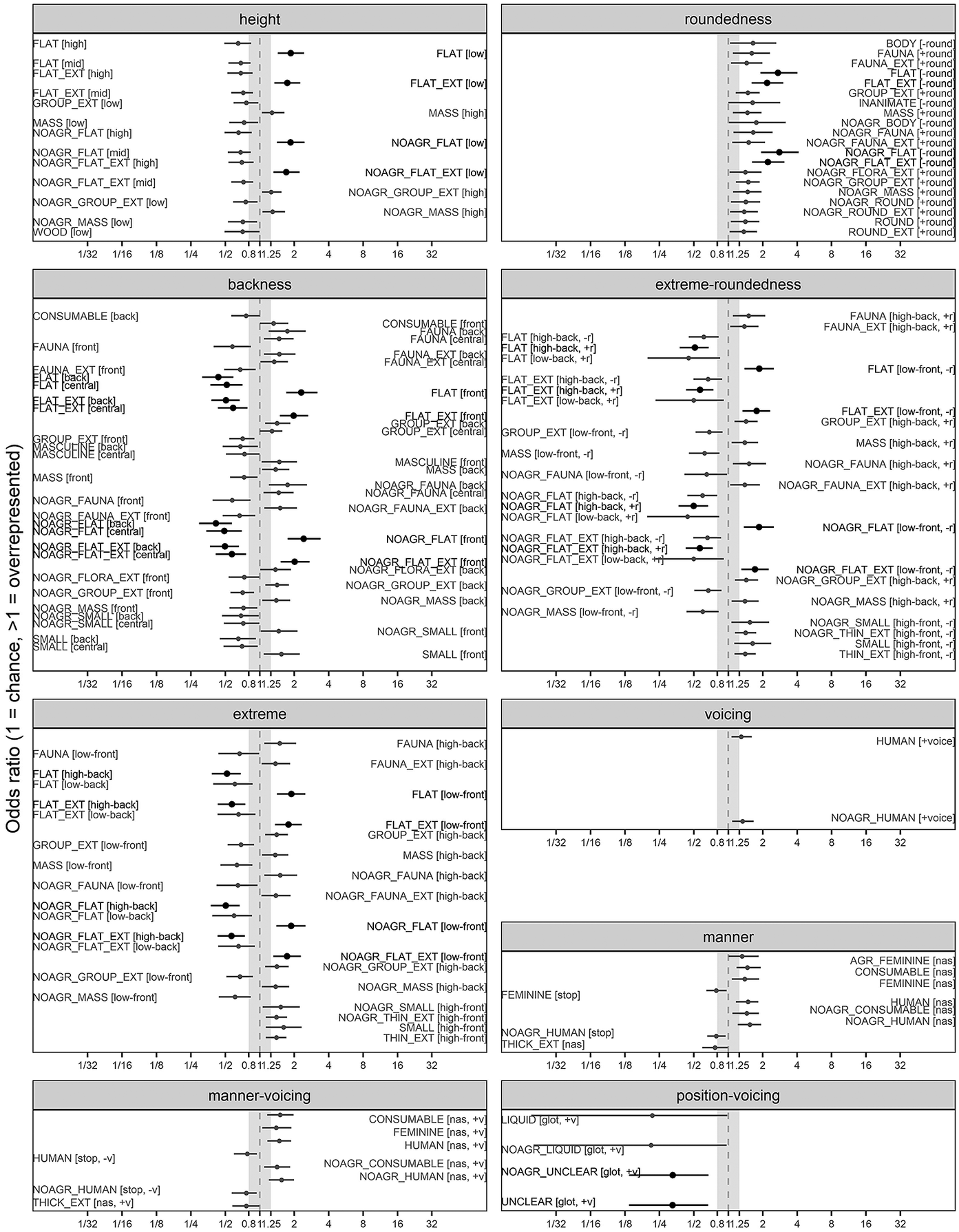
Fig. 2. Over- and underrepresented sound groups in the investigated semantic categories: strong (black) and weak (gray) associations. “[NOAGR]” stands for the non-agreeing subset categories and “[AGR]” for the agreeing subset categories. Each point shows the median of posterior distribution of the ratio of observed to expected odds, with 95% CI. Text labels show the semantic category, associated sound group. The marked region of practical equivalence (ROPE) of [0.8, 1.25] was used to select substantively relevant findings.
Table 4. Summary of found associations

Note: Color indicates association strength and overrepresentation/underrepresentation, and numbers indicate which of the dataset(s) the association was found in. Light blue, weak overrepresentations; dark blue, strong overrepresentations; light red, weak underrepresentations; dark red, strong underrepresentations; 1, only full dataset; 2, only non-agreeing subset; 3, full dataset and non-agreeing subset; 4, full dataset and agreeing subset.
It is evident that most underrepresentations were mirror images of the overrepresentations. The roundedness and voicing parameters only contained two levels which means that, for example, an overrepresentation of rounded vowels automatically resulted in an equally strong underrepresentation of unrounded vowels. This also applies to the other vowel parameters despite the fact that they contained three levels or more. For example, overrepresentations of front vowels were coupled with corresponding underrepresentations of back and/or central vowels, and overrepresentations of low, front, and unrounded vowels with underrepresentations of high, back, and rounded vowels. The overrepresented consonant sound groups were solely represented by nasals, and these were, in some cases, paired with underrepresentations of stop and voiceless stop consonants. The extended semantic category for thick nouns was also found to contain underrepresentations of nasal consonants. It is possible that these consonant contrasts also represent ends of phonological parameters, since nasals and (voiceless) stops are both typologically common, but nasals are continuous and highly sonorous, while (voiceless) stops are abrupt and have very low sonority.
The strong associations were all found in nominal classification devices used for flat referents and involved low, front, unrounded vowels, typically /a/, as seen in the Baniwa do Içana [bani1255] classifier maka which is used for ‘stretchable thin extended objects’ and the Bandial [band1340] noun class ga-, used for ‘flatness, thinness and width’.
Weak associations were found for a range of diverse semantic categories, but since large sections of the CIs overlapped with the ROPE, these results should be viewed with a bit more caution. Among these, we find that nominal classification devices for rolls and round nouns, unsurprisingly, tended to contain rounded vowels, whereas high, front, unrounded vowels, typically /i/, were common in nominal classification devices for small, long, thin, and pointed nouns. These associations can be found in the Lakkia [lakk1238] classifier system in which kjo:k24 classifies rounds and scoops, and mi24 classifies long, thin objects.
Nominal classification devices relating to quantity, used for groups, masses, and bunches, seem to attract high, back, rounded vowels, typically /u/, such as in but, the Abun [abun1252] classifier for ‘bundles of things’ and in ñu, the Jamiltepec Mixtec [jami1235] ‘collective’ grammatical gender/noun class, while nasal consonants and front vowels, typically /a/ or /i/, were common in nominal classification devices used for consumable nouns. For example, the Panare [enap1235] system includes ë’ma ‘classifier for edible: egg, rice, tobacco paste, soup’ and Yawuru [yawu1244] mayi ‘generic term for edible plants including seeds, nuts, berries, fruits, vegetables, tubers and sugarbag’.
Semantic categories more typical for agreeing systems were also found to generate some weak associations. Again, nasal consonants were common in nominal classification devices used for feminine nouns, both in the full dataset and the agreeing subset, while front vowels were common in those used for masculine nouns. Nominal classification devices used for human nouns also included nasal consonants, along with voiced consonants in general, and unrounded vowels were common in those for inanimate nouns. These associations can all be found in the Marithiel [mari1424] noun class system in which ngungku- is used for ‘human, female’ nouns, wati- for ‘human, male’ nouns, and a-, mi-, tha(rr)- and yeli/yeri for various non-human, lower animate and inanimate nouns. Other notable overrepresentations for animacy-related semantic categories included high, back, central, rounded vowels, typically /u/, in nominal classification devices used for flora and fauna including nominal classification devices for land animals, birds, fish, wood, and trees), and unrounded vowels occurred frequently in nominal classification devices for the body and body parts. The Lakkia classifier system again provides illustrative examples; tu231 classifies animals, children, and adults (with derogatory overtones), while nam55 classifies concrete objects, including body parts, and abstract concepts such as skills.
Lastly, some categories were found to contain underrepresentations of certain sound groups but no overrepresentations. This included underrepresentations of voiced glottal consonants in nominal classification devices used for liquid nouns and those judged as unclear, but since this sound group is cross-linguistically rare and sparsely represented in the data, these associations can be disregarded. Nominal classification devices used for tree-, wood- or forest-related nouns, on the other hand, contained underrepresentations of low vowels, while nominal classification devices used for and thick, round and large nouns disfavored nasal consonants.
In addition to the stark differences in results between the non-agreeing system and agreeing system subsets, there were also obvious structural differences between the two system types. Across the sampled data, there was an average of 11.74 nominal classes per system. Languages using non-agreeing systems had an average of 17.14 classes, while those using agreeing systems had an average of 5.32 classes. Non-agreeing nominal classification devices were longer than their agreeing counterparts. The overall average of the nominal classification device length per each semantic category and language (which often had multiple forms) while excluding null morphemes (∅), was 3.17 segments. For example, a semantic category with the forms /ba/, /ti/, and /vu/ in one language, and /sam/ and /ta/ in another language would be calculated as 2 + 2 + 2 = 6 and 6/3 = 2 for the former and as 3 + 2 = 5 and 5/2 = 2.5 for the latter, which results in an average of 2 + 2.5 = 4.5 and thus 4.5/2 = 2.25. However, the average nominal classification device length in non-agreeing systems was 3.5 segments, almost twice as long as the agreeing nominal classification devices which had an average length of 1.82 segments. A possible effect of this length disparity could be seen in the ratio between vowels and consonants. The non-agreeing nominal classification devices were more consonant-heavy (40% vowels and 60% consonants) than the agreeing nominal classification devices (61% vowels and 39% consonants).
4. Discussion
While the presence of iconicity in the lexicon is subject to an increasingly broad accumulation of studies, its presence in more grammaticalized linguistic units has been comparably understudied, with the notable exception of sign language grammar (Padden et al., Reference Padden, Meir, Hwang, Lepic, Seegers and Sampson2013). The present study shows that iconicity generally plays a part in nominal classification, albeit restricted to a limited set of rather diverse semantic categories. The strong associations (overrepresentations) only pertained to nominal classification devices for flat nouns such as boards, sheets, discs, and leaves. The weak associations (overrepresentations) also included several size and shape categories, such as small, round, and thin, as well as categories based on group nouns such as masses, collective, clusters, and piles, consumable nouns, such as staple food, meat, and vegetables, and biological sex or animacy such as feminine, masculine, human, inanimate, fauna, flora, and body. Most of these associations are more typical for non-agreeing systems, and despite the fact that sex- and animacy-based distinctions are common in agreeing systems too, only one overrepresentation was found when the agreeing systems were analyzed separately, which indicates that the results were mainly driven by the data from the non-agreeing systems. The data also showed that the analyzed non-agreeing and agreeing systems were structurally different. The non-agreeing systems included larger inventories of nominal classes and nominal classification devices consisting of longer segment lengths and more consonants than agreeing systems.
The following subsections discuss why vocal iconicity seems to be functional in nominal classification systems, as well as how the found sound-meaning associations can be motivated through cross-linguistic and experimental data. The impact of organization, grammaticalization and presence of agreement on the possible emergence, maintenance, and decay of vocal iconicity in the two system types is also addressed.
4.1 Grounding of vocal iconicity in nominal classification systems
Iconicity is linked to a range of functional properties, including increased linguistic learnability related to sensory imagery and affective meanings (Imai & Kita, Reference Imai and Kita2014; Massaro & Perlman, Reference Massaro and Perlman2017; Nielsen & Dingemanse, Reference Nielsen and Dingemanse2021). The types of sounds involved in vocal iconic associations are diachronically stable, which means that the iconic mappings are more likely to survive sound change (Dellert et al., Reference Dellert, Erben Johansson, Frid and Carling2021), but when such sounds do decay, they tend to re-emerge (Erben Johansson et al., Reference Erben Johansson, Carr and Kirby2021; Flaksman, Reference Flaksman, Zirker, Bauer, Fischer and Ljungberg2017; Johansson & Carling, Reference Johansson and Carling2015; Jones et al., Reference Jones, Vinson, Clostre, Zhu, Santiago and Vigliocco2014; Tamariz & Kirby, Reference Tamariz and Kirby2016; Vinson et al., Reference Vinson, Jones, Sidhu, Lau-Zhu, Santiago and Vigliocco2021). Iconicity, thus, provides an intuitive link between language and the human experience by aiding our ability to refer beyond what is immediately present and to map linguistic labels to objects and events (Perniss & Vigliocco, Reference Perniss and Vigliocco2014).
There also seems to exist a relation between nominal classification systems and the way in which speakers of classifying languages perceive and categorize referents (Seifart, Reference Seifart2010). For example, plant-centric classification systems tend to be more common in rainforest environments (Epps, Reference Epps2008, p. 183). Reversely, languages that classify inherently inanimate nouns to masculine and feminine classes also tend to ascribe male or female characteristics to them (Phillips & Boroditsky, Reference Phillips and Boroditsky2003), and shape-based classification systems cause speakers to pay more attention to shape (Perniss et al., Reference Perniss, Vinson, Seifart and Vigliocco2012; Saalbach & Imai, Reference Saalbach and Imai2007). Many of the semantic categories encoded in nominal classification also have high cue validity (Rosch et al., Reference Rosch, Gray, Johnson, Boyes-Braem and Mervis1976; Seifart, Reference Seifart2010, p. 725). The general idea is therefore that in a communicative setting, an iconic word could efficiently narrow down the selection of possible referents and thereby the intended meaning could be conveyed quicker than if a completely arbitrary word was used. This means that intuitively understanding the semantic scope of a nominal classification device could also lead to a more rapid retrieval of the approximate meaning of the classified referent.
Some of the iconic mappings found in the present study are also corroborated by previous cross-linguistic and experimental data. The overrepresentation of /a/-like vowels in nominal classification devices for flat nouns provided the strongest results among the semantic categories. This association can be placed in contrast with the categories used for round and thick nouns on the one hand, and the categories used for small and thin nouns on the other. All these categories clearly align with previously established iconic associations. Overrepresentations of /a/-like vowels in words for ‘flat’ and ‘leaf’, /u/-like vowels in words for ‘round’, ‘blunt’, ‘navel’, and so forth, and high-frequency sounds, such as /i/-like vowels, in words for ‘small’ and ‘thin’ are cross-linguistically common (Blasi et al., Reference Blasi, Wichmann, Hammarström, Stadler and Christiansen2016; Erben Johansson et al., Reference Erben Johansson, Anikin, Carling and Holmer2020; Joo, Reference Joo2020; Wichmann et al., Reference Wichmann, Holman and Brown2010). The associations with rounded concepts are also backed up by experimental evidence (Jones et al., Reference Jones, Vinson, Clostre, Zhu, Santiago and Vigliocco2014; Köhler, Reference Köhler1929; Styles & Gawne, Reference Styles and Gawne2017), and the underlying mechanism responsible for the associations with small concepts could be explained by a generally perceived correlation between body size, resonance chamber size, and fundamental frequency of vocalizations (Ohala, Reference Ohala, Nichols, Ohala and Hinton1994; Rendall et al., Reference Rendall, Kollias, Ney and Lloyd2005; Winter et al., Reference Winter, Oh, Hübscher, Idemaru, Brown, Prieto and Grawunder2021). Overrepresentations of /u/-like vowels in the nominal classification devices used for groups and masses, that is, large quantities of entities, align well with findings indicating that back vowels are perceived as larger than front vowels (Preziosi & Coane, Reference Preziosi and Coane2017). These associations also involved sound groups that correspond to the three most extreme points of the vowel space (Lockwood & Dingemanse, Reference Lockwood and Dingemanse2015), and concepts such as ‘flat’, ‘round’, ‘small’, and ‘large/many’ are among the most basic concepts used to describe general physical properties of objects (Strickland, Reference Strickland2017). This provides corresponding acoustic, articulatory, and semantic distinctiveness, which could help explain why vocal iconicity is found in these size- and shape-related categories.
The association between the consumable category and nasal consonants and front vowels is supported by previous evidence suggesting that words for gaping, yawning, and tasting contain /a/-like vowels across languages (Erben Johansson et al., Reference Erben Johansson, Anikin, Carling and Holmer2020), and words for staple food, as well as baby talk words for food or eating contain /a/-like vowels, as well as (nasal) labial consonants, such as Vietnamese [viet1252] măm (Joo, Reference Joo2022).
Finally, most of the remaining associations, including underrepresentations, are harder to explain, primarily because the presence or absence of vocal iconicity in these concepts has not previously been investigated. However, the association between the feminine category and nasal consonants does align with cross-linguistic evidence for similar associations involving female-related concepts, such as mother, breast, and milk (Erben Johansson et al., Reference Erben Johansson, Anikin, Carling and Holmer2020). It can thus be assumed that sound-meaning associations can be inherited from more lexical stages to more grammaticalized stages, such as female-related lexemes being extended to a more general feminine category.
4.2 Vocal iconicity and grammaticalization
The results mainly involved semantic categories which are more typical for non-agreeing systems, suggesting that the non-agreeing languages were the driving force behind the noteworthy overrepresentations. One interpretation of this could be that the semantic categories encoded by agreeing systems simply are not as iconic, but this could also, at least in part, be attributed to differences in the organization and function of the systems. For example, the often highly grammaticalized agreeing systems tend to have a rigid class assignment where each noun is typically assigned to exactly one class (Aikhenvald, Reference Aikhenvald2000, p. 21), whereas the often semantically transparent non-agreeing systems tend to allow the use of multiple classifiers with the same noun, evoking different meanings (Contini-Morava & Kilarski, Reference Contini-Morava and Kilarski2013, p. 266).
Another reason for these differences may be found in the diachronic pathways of the system. It is quite clear that non-agreeing nominal classification devices very often arise from lexical nouns (Seifart, Reference Seifart2010, p. 727), evident from the lack of substantial phonetic erosion, for example, Mam [mamm1241] q’a ‘young man’ (classifier) < q’aa ‘young man’ (noun) (Aikhenvald, Reference Aikhenvald2000, p. 357). The case for the emergence of agreeing nominal classification devices is less clear, and a more varied set of sources has been proposed, for example, nouns, classifiers, demonstratives, definite articles, and third person pronouns (Aikhenvald, Reference Aikhenvald2000, p. 367ff).
The fact that the agreeing nominal classification devices contained fewer segments than the non-agreeing nominal classification devices provides evidence for phonetic erosion. Fewer available segments would, in turn, lead to fewer possible sound-meaning associations since many segments are iconically restricted to specific meanings. Shorter nominal classification devices could also be increasingly susceptible to further sound change and convergence, such as the merging of homophonous nominal classification devices into larger classes. Relatedly, many languages use a mix of phonological and semantic cues to classify nouns, but semantic cues are considerably more cross-linguistically consistent. Interestingly, child learners rely on more phonological cues when assigning nominal classes to nouns even when there are competing highly salient, reliable semantic cues (Culbertson et al., Reference Culbertson, Gagliardi and Smith2017). This uneven reliance on sounds and meanings should affect the development of agreeing systems by propelling phonologically derived processes, such as analogy, which would further break down iconic associations.
On the semantic side, agreeing systems tend to have a much smaller set of nominal classes, which results in a situation where a very large number of diverse referents are conflated into semantically vague classes. This means that even if iconic associations would be present in an agreeing nominal class, the iconicity would probably be only relevant for a subset of the nouns belonging to that class, Fig. 3. For example, an association between feminine and nasal consonants could only be intuitively understood for nouns with semantic features that would somehow semantically evoke the meaning of ‘femininity’. The other meanings belonging to the same nominal class would either not be applicable for this association, or include semantic features perceived to stand in contrast to ‘femininity’. One such example would be that German Mädchen, which means ‘girl’, belongs to the neuter nominal class.
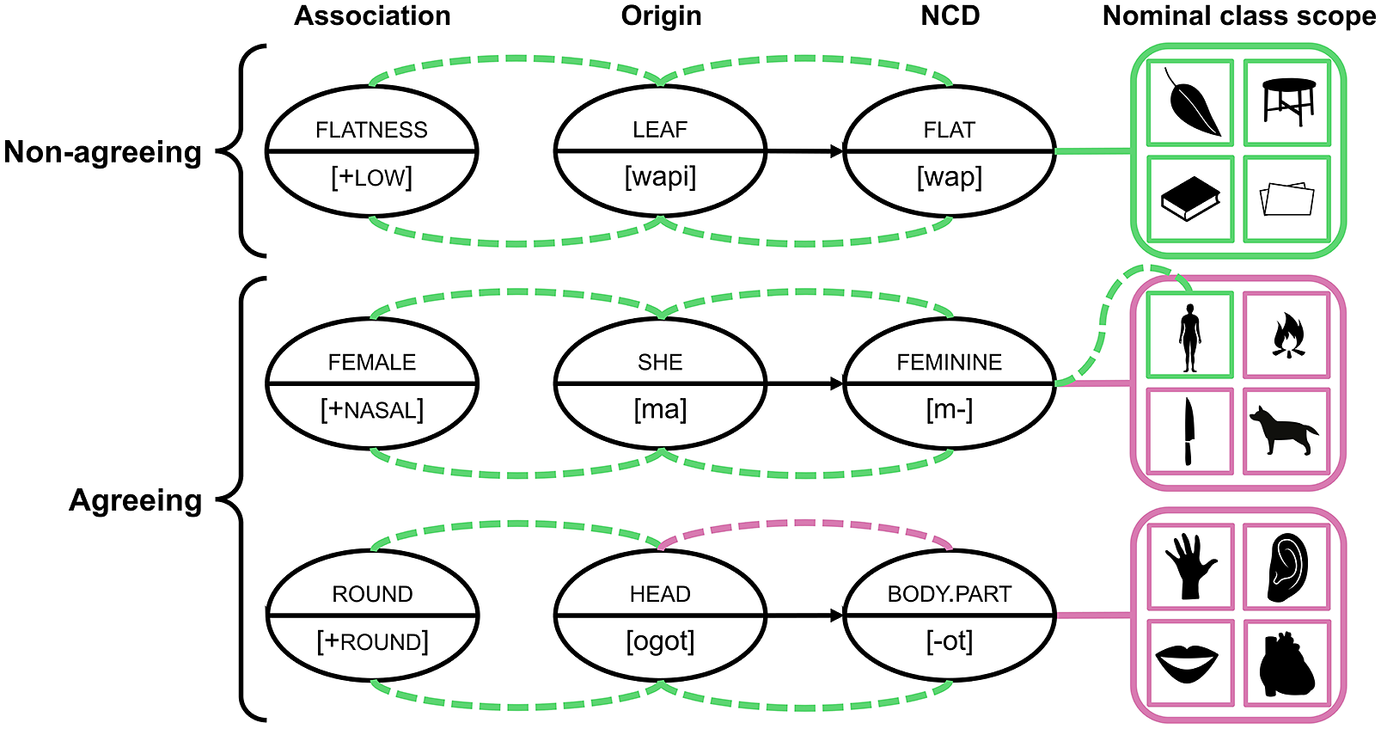
Fig. 3. Hypothetical examples of how well iconicity can be upheld in different nominal classification systems. Iconicity can be fully upheld in non-agreeing systems by nominal classes containing nouns that all share the same relevant semantic feature(s), such as flat (top). Iconicity can be upheld for certain nouns belonging to the nominal class by sharing the same relevant semantic feature(s), such as female, which causes the entire nominal class to be, at best, only partially iconic (middle). Iconicity breaks down completely when a nominal class contain nouns, in this case a broad assortment of different body parts, that cannot be iconically mapped to the relevant sounds, in this case a rounded vowel (bottom).
4.3 Semantic transparency and agreement: competing benefits
Considering the present results, what is known about the functionality of iconicity and the overall distribution of different types of nominal classification systems, there is a notable paradox. What would be the benefit of having a system consisting of general, semantically untransparent nominal classes which ought to be harder to learn rather than a well-functioning, semantically transparent system in which iconicity could aid meaning retrieval (Franjieh et al., Reference Franjieh, Corbett and Grandison2020)? One functional gain would be that agreement makes it easier to predict which words belong to the same noun phrase, but this does not necessarily need to entail semantic bleaching. It is possible that as the formalization of a system becomes stronger, there is a shift from non-agreeing nominal classification devices semantically classifying referent nouns to the nouns being more tightly associated with specific nominal classification devices, which restricts assignment flexibility and lessens the need for semantic transparency, Fig. 4. With the presumably higher functional workload of a less semantically transparent system, it would be expected that many distinctions collapse into a small number of classes, since speakers must be able to keep track of them. This would also mean that most of the encoding of semantic properties cannot be upheld, but the gain of formal predictability via agreement seems to be substantial enough to outcompete the semantic distinctions.
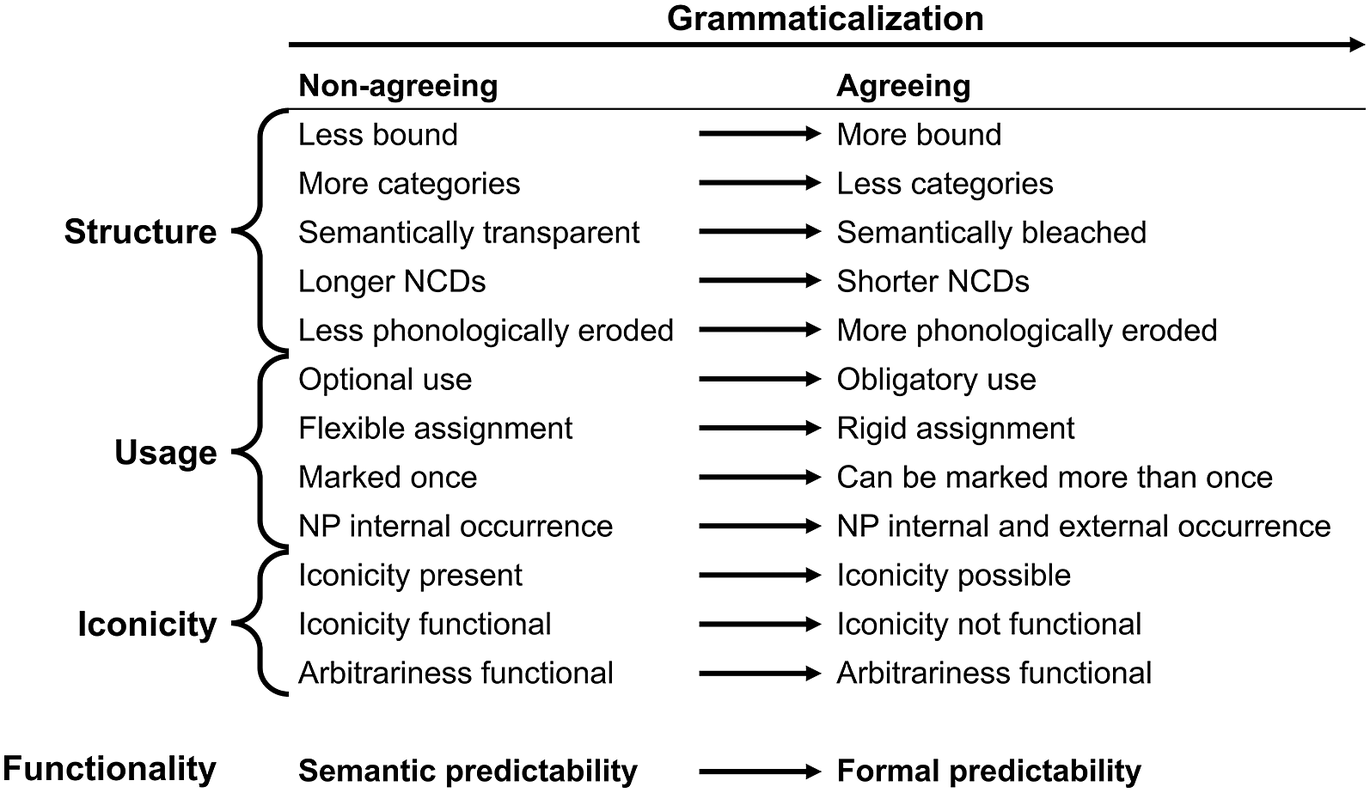
Fig. 4. Summary of differences between non-agreeing and agreeing systems in terms of structure, usage, iconicity and functionally (Lehmann, Reference Lehmann2015, p. 174; Passer, Reference Passer2016, p. 103; Seifart, Reference Seifart2005, p. 102).
This aligns with the evidence suggesting that natural languages require different parallel pathways for connecting signals to meanings as described by Dingemanse et al. (Reference Dingemanse, Blasi, Lupyan, Christiansen and Monaghan2015). While iconicity eases learnability, there are not enough unique linguistic signals to account for all the meanings language users need to express. This means that iconic signals are very useful for a subset of the linguistic system, but other, non-iconic, mappings between signals and meanings are required for providing the remainder of the lexicon with enough labels. Lastly, it should be mentioned that a sizable share of the world’s languages completely lacks nominal classification – 46.6%, according to the largest available database (Allassonnière-Tang et al., Reference Allassonnière-Tang, Lundgren, Robbers, Cronhamn, Larsson, Her, Hammarström and Carling2021), suggesting that the categorization of nouns does not seem to have any intrinsic value. However, if a language uses a nominal classification system, it ought to be equally communicatively efficient to either rely on semantic transparency, through iconicity, or formal predictability, through agreement.
5. Conclusion
We have investigated the presence of vocal iconicity in nominal classification systems by using a large and genetically diverse dataset of spoken languages which was distributed evenly across the two main types of nominal classification systems: non-agreeing and agreeing systems. By collecting the nominal classification devices used for the nominal classes occurring in the sampled languages, transcribing these with the use of a coherent and comparable phonetic system, grouping them according to comparable semantic categories and analyzing them through Bayesian mixed models, we were able to assess whether certain types of sounds were overrepresented in certain class meanings. The results revealed that the strongest overrepresentations were found in nominal classification devices used for flat referents, along with a range of interesting albeit weaker associations relating to shape/size/quantity, function, humanness/animacy, and biological sex. Most of these associations correspond to sound-meaning associations which have been found in the lexicon through large-scale cross-linguistic and experimental studies.
There were considerable structural differences between the two types of nominal classification systems. For example, the non-agreeing systems included in the dataset contained, on average, more than three times as many nominal classes and twice as many segments per nominal classification device as the agreeing systems. These differences could be attributed to a number of intertwined processes linked to language change, such as grammaticalization, including more substantial phonetic erosion and semantic bleaching of agreeing nominal classification devices as nominal classification systems become increasingly formalized. This means that if a non-agreeing system changes into a more agreement-based system, it loses semantic transparency but gains formal predictability. While this does not seem to have any effect on the efficiency or functionality of the system as a whole, the lack of semantic transparency dismantles one of the two key components of vocal iconicity and causes sound-meaning mappings to break down. In other words, when a nominal classification system changes from one type to another, prerequisites which are needed for vocal iconic associations to be operational also change.
The general conclusion that can be drawn from the results is that iconicity is present in nominal classification systems but seemingly primarily in non-agreeing systems. It is possible that these sound overrepresentations exist solely because of inherited iconic effects present in the lexemes that the nominal classification devices are derived from. However, considering the demonstrated benefits iconicity can bring to the acquisition of language, it is reasonable to assume that vocal iconicity also accelerates the retrieval of noun semantics if primed with iconically congruent nominal classification devices.
Future studies could include an even larger sample of languages to increase the statistical reliability of the semantic categories which were represented by very few data points. Categorizing nominal classification systems using a gradual grammaticalization scale could also be beneficial, even if the binary distinction used in the present study ought to be a relatively consistent way of dividing these types of systems considering the varying state of grammatical descriptions.
Acknowledgments
This study was conducted thanks to funding granted by The Swedish Research Council (2020-06398).
Data Availability Statement
Data and code is available at: https://osf.io/7vhc8/.