Introduction
Depression has a lifetime prevalence of 19% in industrialized nations and affects approximately 322 million people worldwide (World Health Organization, 2008, 2017). In middle- and high-income countries, depression and cardiovascular disease (CVD) are the leading causes for impairments in quality of life and CVD is also a primary cause for mortality (Wittchen et al., Reference Wittchen, Jacobi, Klose and Ryl2010; Christopher and Murray, Reference Christopher and Murray2016).
Depression and CVD are interrelated (Shaffer et al., Reference Shaffer, Whang, Shimbo, Burg, Schwartz and Davidson2012). For example, meta-analyses of longitudinal prospective studies strengthen the assumption that depressive symptoms are an independent risk factor for the development of CVDs, such as hypertension (Meng et al., Reference Meng, Chen, Yang, Zheng and Hui2012), myocardial infarction (van der Kooy et al., Reference van der Kooy, van Hout, Marwijk, Marten, Stehouwer and Beekman2007; Gan et al., Reference Gan, Gong, Tong, Sun, Cong, Dong, Wang, Xu, Yin, Deng, Li, Cao and Lu2014; Wu and Kling, Reference Wu and Kling2016) and coronary heart disease (Rugulies, Reference Rugulies2002; Nicholson et al., Reference Nicholson, Kuper and Hemingway2006a, Reference Nicholson, Kuper and Hemingway2006b; van der Kooy et al., Reference van der Kooy, van Hout, Marwijk, Marten, Stehouwer and Beekman2007; Gan et al., Reference Gan, Gong, Tong, Sun, Cong, Dong, Wang, Xu, Yin, Deng, Li, Cao and Lu2014; Wu and Kling, Reference Wu and Kling2016). In addition, depressive symptoms are frequently observed in patients with CVD: in survivors of acute myocardial infarction, major depression (MD) was prevalent in nearly 20% shortly after the acute medical event (Thombs et al., Reference Thombs, Eric, Bass, Ford, Stewart, Tsilidis, Patel, Fauerbach, Bush and Ziegelstein2014). Clinically relevant depressive symptoms occur in around one-third of patients after stroke (Hackett and Pickles, Reference Hackett and Pickles2014). Depressive symptoms are also predictive for morbidity and mortality in coronary heart disease (Goldston and Baillie, Reference Goldston and Baillie2008).
Heart rate variability (HRV) refers to variations between two successive heartbeats which ensures optimal adaption to environmental challenges. HRV is influenced by parasympathetic autonomic activation including the vagus nerve (which slows down heart rate) and via sympathetic activation (which accelerates heart rate). HRV is frequently quantified using time-domain measures such as the standard deviation of NN intervals (SDNN) and the root mean square of successive differences between normal heartbeats (RMSSD), which is more influenced by vagal activity than SDNN. HRV is also often described in terms of frequency-domain measures. High-frequency (HF)-HRV primarily reflects parasympathetic vagal activity. Low-frequency (LF)-HRV is more complex and may include both sympathetic and parasympathetic influences. Very-low-frequency (VLF)-HRV might reflect long-term regulation mechanisms (e.g. thermoregulation or hormonal factors). A third category of HRV is respiratory sinus arrhythmia (RSA), which reflects heart rate variations via the vagus nerve related to the respiratory cycle (Task Force of The European Society of Cardiology and The North American & Society of Pacing and Electrophysiology, 1996; Shaffer et al., Reference Shaffer, McCraty and Zerr2014; Shaffer and Ginsberg, Reference Shaffer and Ginsberg2017).
Chronically reduced HRV indicates an autonomic imbalance. A substantial body of research suggests that reductions in HRV predict poor cardiovascular health outcomes in both populations without baseline CVD and clinical samples (Buccelletti et al., Reference Buccelletti, Gilardi, Scaini, Galiuto, Persiani, Biondi, Basile and Silveri2009; Hillebrand et al., Reference Hillebrand, Gast, de Mutsert, Swenne, Jukema, Middeldorp, Rosendaal and Dekkers2013; Kubota et al., Reference Kubota, Chen, Whitsel and Folsom2017). Previous meta-analyses suggest that HRV is lower in patients with MD than in healthy controls across all age groups (Kemp et al., Reference Kemp, Quintana, Gray, Felmingham, Brown and Gatt2010; Rottenberg, Reference Rottenberg2007; Koenig et al., Reference Koenig, Kemp, Beauchaine, Thayer and Kaess2016; Brown et al., Reference Brown, Karmakar, Grey, Jindal, Lim and Bryant2018). Importantly, reduced HRV is not a specific feature of MD but rather a transdiagnostic factor which relates to several stress-related states, conditions and behavioral factors, as well as to several medical conditions and medications (Gidron et al., Reference Gidron, Deschepper, De Couck, Thayer and Velkeniers2018). Nevertheless, HRV might be an important mediator between depression and CVD (Sgoifo et al., Reference Sgoifo, Carnevali, Alfonso and Amore2015; Shaffer and Ginsberg, Reference Shaffer and Ginsberg2017).
The latest meta-analysis investigating HRV in MD in (apart from late-life depression, see Brown et al., Reference Brown, Karmakar, Grey, Jindal, Lim and Bryant2018) was conducted by Kemp and colleagues in 2010 and included 11 studies (published until July 2009) (Kemp et al., Reference Kemp, Quintana, Gray, Felmingham, Brown and Gatt2010). Results indicated that several HRV measures are lower in MD compared to controls by medium to large effect sizes. In the last decade, many studies have investigated HRV in MD. While some of them have also reported reductions in HRV (e.g. Berger et al., Reference Berger, Schulz, Kletta, Voss and Bär2011; Berger et al., Reference Berger, Kliem, Yeragani and Bär2012; Kemp et al., Reference Kemp, Quintana, Felmingham, Matthews and Jelinek2012; Brunoni et al., Reference Brunoni, Kemp, Dantas, Goulart, Nunes, Boggio, Mill, Lotufo, Fregni and Benseñor2013; Kemp and Quintana, Reference Kemp and Quintana2013) others did not find significant differences or have attributed alterations in HRV to antidepressant treatment (Licht et al., Reference Licht, de Geus, Zitman, Hoogendijk, van Dyck and Penninx2008; O'Regan et al., Reference O'Regan, Kenny, Cronin, Finucane and Kearney2015).
The purpose of this study is to provide up-to-date random-effects meta-analyses of studies that compare resting-state measures of HRV between unmedicated adults with MD (as defined by DSM-III-R, DSM-IV, DSM-IV-TR or DSM-5) and controls. Effects of antidepressants on HRV have been the subject of controversy and HRV alterations in MD may result in part from antidepressants, in particular tricyclic antidepressants (Kemp et al., Reference Kemp, Quintana, Gray, Felmingham, Brown and Gatt2010, Reference Kemp, Quintana and Malhi2011, Reference Kemp, Fráguas, Brunoni, Bittencourt, Nunes, Dantas, Andreão, Mill, Ribeiro, Koenig, Thayer, Benseñor and Lotufo2016; Licht et al., Reference Licht, Penninx and de Geus2011; Huang et al., Reference Huang, Liao, Kuo, Chang, Chen, Chen and Yang2016). To avoid confounded or overestimated results, we therefore exclusively focus on studies that include participants without antidepressants, and also without cardiac drugs and CVD. Different from an earlier meta-analysis in this field, which has combined interrelated measures of HRV (Kemp et al., Reference Kemp, Quintana, Gray, Felmingham, Brown and Gatt2010), our meta-analysis provides separate results for specific measures of HRV. This approach was facilitated by the increasing availability of HRV data from samples with MD and may enable a more differentiated understanding of HRV disturbances in MD. Further, the literature is inconclusive regarding equivalence and the approach of treating interrelated HRV measures equivalent, in particular when measures derived from short-term recordings (Task Force of The European Society of Cardiology and The North American & Society of Pacing and Electrophysiology, 1996; Berntson et al., Reference Berntson, Lozano and Chen2005; Kemp et al., Reference Kemp, Quintana, Gray, Felmingham, Brown and Gatt2010; Shaffer and Ginsberg, Reference Shaffer and Ginsberg2017). Finally, this study considers appropriate methods to control for publication bias and investigates if ECG recording length and study quality moderate magnitudes of effect sizes.
Methods
Literature search and inclusion criteria
This meta-analysis was conducted according to the ‘Preferred Reporting Items for Systematic Reviews and Meta-Analysis’ (PRISMA) guidelines (Moher et al., Reference Moher, Liberati, Tetzlaff and Altman2009). Online Supplement 1 includes the PRISMA-Checklist. Because this meta-analysis did not aim to compare intervention effects, it was not preregistered and no review protocol exists.
A systematic literature search was performed up to 10 July 2018. Two investigators (CK & FE) independently searched on PubMed and PsychINFO for publications using the terms [(depress*) AND (heart rate variability) OR HRV OR (cycle length variability) OR (RR variability) OR (heart period variability) OR vagal OR (autonomic nervous system) OR (ANS)]. Studies published since 1 January 1987 (the year of publication of DSM-III-R) were considered with no filters applied. Email alerts notified the investigators of potentially relevant studies published during the process of study selection. The ClinicalTrials.gov database was searched for unpublished studies. Disagreements between the investigators were solved by discussion. The search in PubMed and PsychINFO yielded 6121 and 1779 results, respectively. After removal of duplicates, 7104 titles and abstracts were screened. The search on ClinicalTrials.gov yielded 29 unpublished studies on depressive patients with HRV assessment. Reviews, meta-analyses, abstracts from conference proceedings and single-case studies were excluded. In particular, studies including patients with CVD, cardiac medication and antidepressants were excluded. Also, studies with samples of patients with diabetes and neurological disorders were excluded. Exclusion criteria for each study are outlined in online Supplement 2 (Table S1).
To be eligible for full-test screening, abstracts had to report a comparison of HRV in adults (⩾18 years) in MD to healthy controls.
Studies were included if they:
(1) reported a resting state time-or frequency domain measure of HRV in both (i) unmedicated adults with MD (as defined by DSM-III-R, DSM-IV, DSM-IV-TR or DSM-5) and (ii) age-matched healthy controls.
(2) were published in a peer-reviewed journal
(3) were written in English or German.
Data extraction
A data extraction sheet was developed based on inclusion criteria, previous meta-analyses (e.g. Tak et al., Reference Tak, Riese, de Bock, Manoharan, Kok and Rosmalen2009; Kemp et al., Reference Kemp, Quintana, Gray, Felmingham, Brown and Gatt2010; Koenig et al., Reference Koenig, Kemp, Beauchaine, Thayer and Kaess2016) and common study characteristics usually extracted in meta-analyses (e.g. year and country of publication). During the process of study extraction, the sheet was continually adapted. Information on the year and country of publication, matching, inclusion and exclusion criteria, sample size, mean age of participants, diagnostics (i.e. diagnosis assessment tool, medical and psychiatric comorbidities), as well as ECG recording length was extracted from all included studies. If data were not extractable (i.e. only provided in graphs), authors were contacted and asked for additional information. If the data could not be provided on time (Bär et al., Reference Bär, Greiner, Jochum, Friedrich, Wagner and Sauer2004; Chang et al., Reference Chang, Chang, Kuo and Huang2015) a plot digitizer (Rohatgi, Reference Rohatgi2012, Web Plot Digitizer, available at https://automeris.io/WebPlotDigitizer/) was used to estimate the mean and standard deviation (s.d.). When standard errors were reported instead of standard deviations, the standard deviation was estimated in accordance to an earlier meta-analysis, by using the following formula: ${\rm SD}\, = \,{\rm SE}\; \times \,\sqrt n $ (Higgins and Green, Reference Higgins and Green2011; Koenig et al., Reference Koenig, Kemp, Beauchaine, Thayer and Kaess2016). Absolute values as well as logarithmically transformed values but not normalized values were included in the meta-analysis (Rottenberg, Reference Rottenberg2007; Tak et al., Reference Tak, Riese, de Bock, Manoharan, Kok and Rosmalen2009).
Statistical analyses
The Comprehensive Meta-Analysis (CMA; Version 3) statistical software package (Borenstein et al., Reference Borenstein, Hedges, Higgins and Rothstein2014) was used to aggregate individual studies. Since sample sizes varied between studies and between samples with MD and controls within the same study, the adjusted mean difference (Hedges' g) was chosen as the primary summary measure and 95% confidence intervals were computed. Effect sizes of 0.2, 0.5 and 0.8 were considered as low, moderate and large effects (Cohen, Reference Cohen1988). Several study characteristics varied between studies (e.g. sample size, mean age and gender distribution). Therefore, non-random-variance in effect-sizes was assumed and random-effects models were chosen to compute the overall effect sizes for HRV-measures (Borenstein et al., Reference Borenstein, Hedges, Higgins and Rothstein2009). Heterogeneity was assessed using the I 2 index (Higgins and Thompson, Reference Higgins and Thompson2002), which quantifies the amount of variation between studies that can be attributed to true variation in effect sizes (e.g. an I 2 of 23% indicates that 23% of the variation in effect sizes between studies can be explained by true variation in effect sizes and not by sampling error). An I 2 of 25, 50 and 75% is considered as low, moderate and high heterogeneity, respectively. I 2 does not depend on the number of studies included in the meta-analysis or the metric of the effect size (Higgins et al., Reference Higgins, Thompson, Deeks and Altman2003). To assess publication bias, funnel plots were visually inspected to discover possible asymmetry that might occur due to the selective publication of smaller studies reporting large effect sizes (Higgins and Green, Reference Higgins and Green2008). Additionally, the trim and fill method was used as a statistical procedure as an estimate of the unbiased effect size (Duval and Tweedie, Reference Duval and Tweedie2000). Meta-regression was performed on each of the HRV measures to test if the ECG recording length and study quality moderated the effect size. Two researchers (MW and SS) independently rated study quality using a slightly modified rating scale adapted from Tak et al. (Reference Tak, Riese, de Bock, Manoharan, Kok and Rosmalen2009). Interrater reliability for independent ratings was in the range of almost perfect agreement (κ = 0.823). As the next step, any disagreements were resolved through discussion to obtain consistent values. Rating criteria and results are shown in online Supplement 4.
Results
The database search yielded 197 articles to be full-text screened. The gray literature search on ClinicalTrials.gov yielded no additional results since all potentially relevant studies were still recruiting. After full-text screening, 21 studies remained to be included in the meta-analysis, with N = 2250 patients and N = 1982 controls (N = 4235). Figure 1 illustrates results of the selection procedure. Characteristics of the included studies and HRV recording lengths for each study (M = 10.68 min, S.D. = 9.66 min, range = 28.33 min) are shown in online Supplementary Table S1. Separate meta-analyses were conducted for HRV frequency- and time-domains. We provide no meta-analysis for RSA, because only two studies were extracted, which differ in RSA calculation (Lehofer et al., Reference Lehofer, Moser, Hoehn-Saric, McLeod, Liebmann, Drnovsek, Egner, Hildebrandt and Zapotoczky1997; Berger et al., Reference Berger, Kliem, Yeragani and Bär2012).
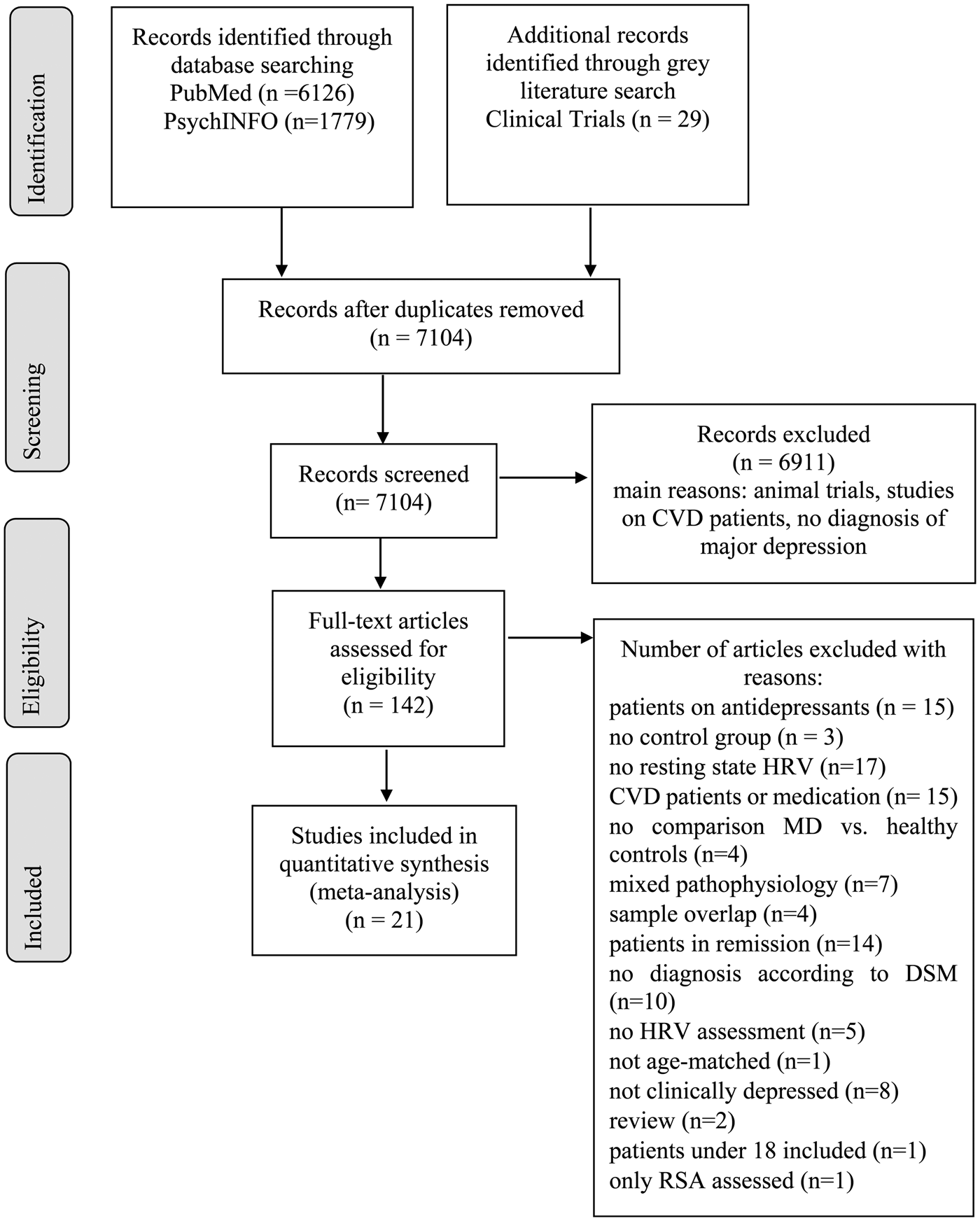
Fig. 1. PRISMA flow diagram.
Meta-analysis 1 – frequency domain: high-frequency heart rate variability (HF-HRV)
Compared to control groups (N = 1724), depressed samples (N = 1977) showed a significant reduction in HF-HRV [Z = −8.860, p < 0.001; Hedges' g = −0.318; 95% CI (−0.388 to −0.247); k = 13, N = 3701], as illustrated in Table 1. There was no evidence for heterogeneity across studies (τ 2 = 0.00, χ2(13, N = 3701) = 12.513, p = 0.405; I 2 = 4.103%). The visual inspection of the funnel plot indicated slight asymmetry (online Supplement 3, Fig. S2). Using trim and fill did not change the effect size Hedges' g = −0.318; 95% CI (−0.388 to −0. 247).
Table 1. Random-effects meta-analysis forest plot for HF-HRV: Comparison between patients with Major Depression (MD) and healthy controls (HC).
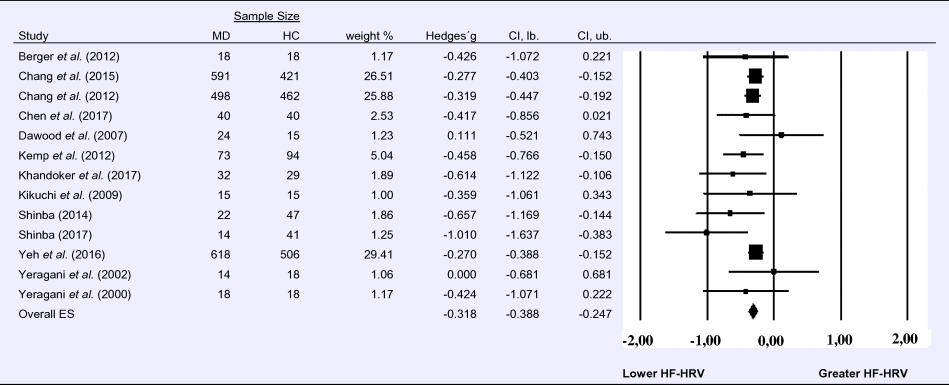
Meta-analysis 2 – frequency domain: low-frequency heart rate variability (LF-HRV)
Compared to control groups (N = 1640), depressed samples (N = 1939) showed a significant reduction in LF-HRV (Z = −2.803, p = 0.005; Hedges' g = −0.195; 95% CI (−0.332 to −0.059); k = 12, N = 3579), as illustrated in Table 2. Heterogeneity across studies was low (τ 2 = 0.02, χ2(11, N = 3579) = 26.07, p = 0.009; I 2 = 26.12%). The visual inspection of the funnel plot indicated slight asymmetry (Supplement 3, Fig. S3). Using trim and fill attenuated the effect size to Hedges’ g = −0.158; 95% CI (−0.297 to −0.019).
Table 2. Random-effects meta-analysis forest plot for LF-HRV: Comparison between patients with Major Depression (MD) and healthy controls (HC).
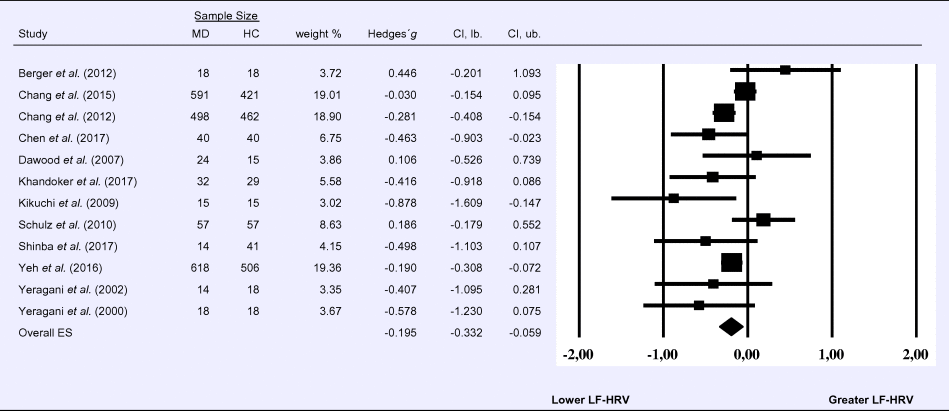
Meta-analysis 3 – frequency domain: very-low frequency heart-rate variability (VLF-HRV)
Compared to control groups (N = 992), depressed samples (N = 1273) showed a significant reduction in VLF-HRV [Z = −2.263, p = 0.024; Hedges’ g = −0.096; 95% CI (−0.179 to −0.013); k = 5, N = 2265], as illustrated in Table 3. There was no evidence for heterogeneity across studies (τ 2 = 0.00, χ2(4, N = 2265) = 2.05, p = 0.726; I 2 = 0.00%). The visual inspection of the funnel plot indicated slight asymmetry (online Supplement 3, Fig. S4), but using trim and fill did not change the magnitude of the effect size.
Table 3. Random-effects meta-analysis forest plot for VLF-HRV: Comparison between patients with Major Depression (MD) and healthy controls (HC).
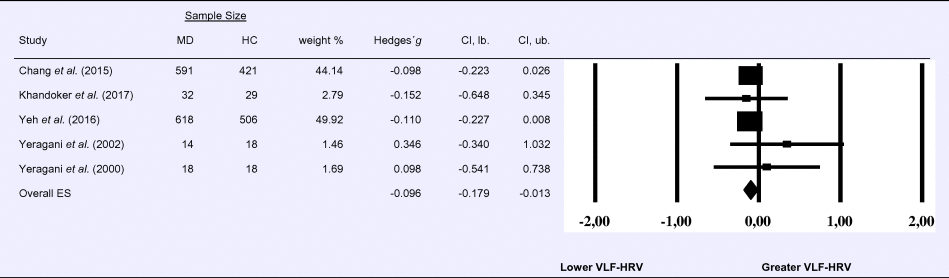
Meta-analysis 4 – frequency domain: LF/HF ratio
Compared to control groups (N = 2189), depressed samples (N = 1929) exhibited a significantly higher LF/HF ratio (Z = 3.525, p < 0.001; Hedges’ g = 0.195; 95% CI (0.086; 0.303); k = 19, N = 4118), as illustrated in Table 4. Heterogeneity across studies was low to moderate (τ 2 = 0.02, χ2(18, N = 4118) = 32.232, p = 0.021; I 2 = 44.16%). The visual inspection of the funnel plot indicated slight asymmetry (online Supplement 3, Fig. S5), but using trim and fill did not change the magnitude of the effect size.
Table 4. Random-effects meta-analysis forest plot for LF/HF Ratio: Comparison between patients with Major Depression (MD) and healthy controls (HC).
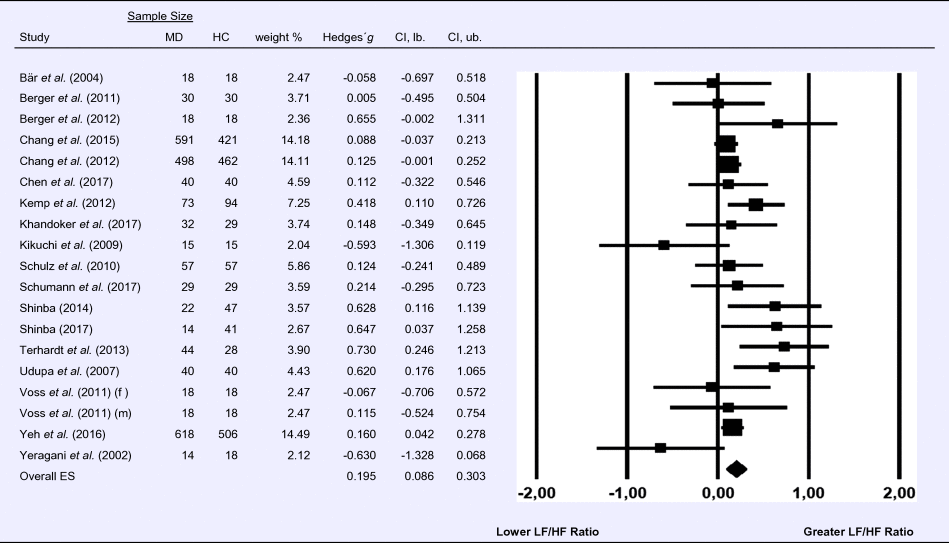
Meta-analysis 5 – time domain: root mean square of successive differences between normal heartbeats (RMSSD)
Compared to control groups (N = 337), depressed samples (N = 356) showed a significant reduction in RMSSD [Z = −6.037, p < 0.001; Hedges’ g = −0.462; 95% CI (−0.612 to −0.312); k = 9, N = 692], as illustrated in Table 5. There was no evidence for heterogeneity across studies [τ 2 = 0.00, χ2(8, N = 692) = 2.67, p = 0.954; I 2 = 0.00%]. The visual inspection of the funnel plot indicated slight asymmetry (online Supplement 3, Fig. S6). Using trim and fill changed the effect size to Hedges' g =−0.480; 95% CI (−0.623 to −0.339).
Table 5. Random-effects meta-analysis forest plot for RMSSD: Comparison between patients with Major Depression (MD) and healthy controls (HC).
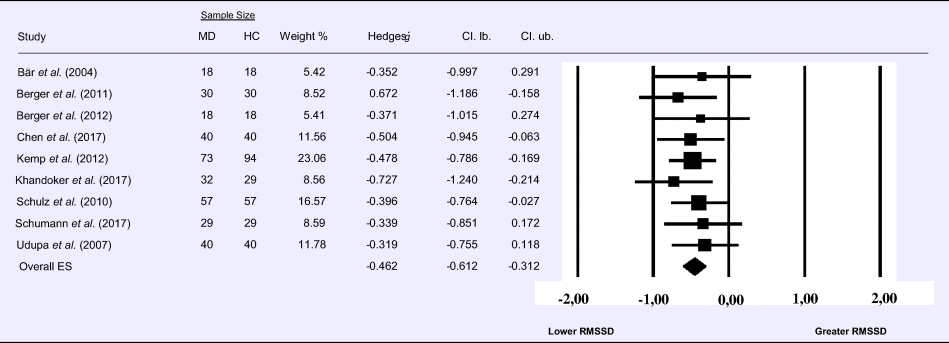
Meta-analysis 6 – time domain: standard deviation of the intervals between normal beats (SDNN)
Compared to control groups (N = 271), depressed samples (N = 289) presented a significant reduction of small effect size in SDNN [Z = −3.142, p = 0.002; Hedges' g = −0.266; 95% CI (−0.431 to −0.100); k = 9, N = 560], as illustrated in Table 6. There was no evidence for heterogeneity across studies [τ 2 = 0.00, χ2(5, N = 560) = 4.469, p = 0.484; I 2 = 0.00%]. The visual inspection of the funnel plot (online Supplement 3, Fig. S7) indicated no asymmetry and using trim and fill did not change the magnitude of the effect size.
Table 6. Random-effects meta-analysis forest plot for SDNN: Comparison between patients with Major Depression (MD) and healthy controls (HC).
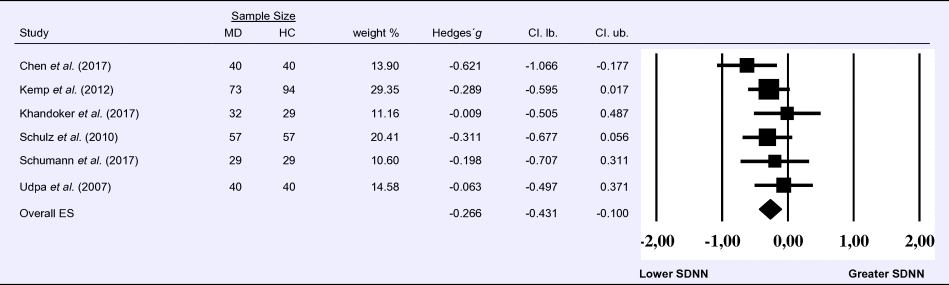
Meta-analysis 7 – time domain: interbeat interval (IBI)
Compared to control groups (N = 1855), depressed samples (N = 1535) showed a significant reduction of small effect size in IBI [Z = −2.267, p = 0.023; Hedges' g = −0.163; 95% CI (−0.304 to −0.022); k = 7, N = 3390], as illustrated in Table 7. Heterogeneity across studies was moderate [τ 2 = 0.02, χ2(6, N = 3390) = 16.629, p = 0.011; I 2 = 63.92%]. The visual inspection of the funnel plot indicated slight asymmetry (online Supplement 3, Fig. S8). Using trim and fill attenuated the effect size to Hedges' g = −0.141; 95% CI (−0.275 to −0.007).
Table 7. Random-effects meta-analysis forest plot for IBI: Comparison between patients with Major Depression (MD) and healthy controls (HC).
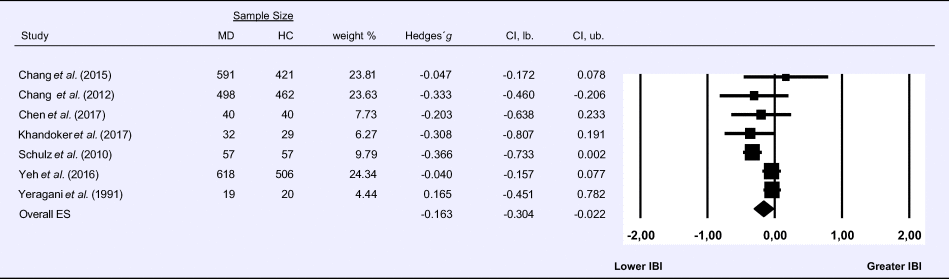
Meta-regression 1 – impact of ECG recording length
Recording length did not moderate the differences between depressed samples and controls in HRV measures, except for the interbeat interval [β = −0.0172, 95% CI (−0.026 to−0.008)]. Here, greater recording length resulted in lower effect sizes. Results of the other meta-regressions are shown in online Supplement 5 (Table S2).
Meta-regression 2 – impact of study quality
Study quality did not moderate the differences between depressed samples and controls in any of the HRV measures. Results of meta-regressions are shown in online Supplement 6 (Table S3).
Discussion
The purpose of this study was to provide up-to-date meta-analyses of studies that compare resting-state measures of HRV between unmedicated adults with MD and controls. Results suggest that patients with MD are likely to display small reductions in several measures of HRV such as HF-HRV, LF-HRV, SDNN and IBI and an increase in LF/HF ratio. The largest effect size was found for RMSSD, a time domain measure of HRV, suggesting that reductions in patients with MD in this measure are of small to moderate magnitude. The reduction in VLF-HRV was minimal but still statistically significant. Our findings thus strengthen evidence that MD is not associated with alteration in specific indicators of HRV but rather with abnormalities in several time- and frequency-domain measures, although the effect sizes for these alterations differ. In this context, it is noteworthy that several time- and frequency-domain measures, rather than specific indicators, have predictive value for poor cardiovascular health outcomes in populations without known baseline CVD (Hillebrand et al., Reference Hillebrand, Gast, de Mutsert, Swenne, Jukema, Middeldorp, Rosendaal and Dekkers2013). A further important feature of our meta-analyses is that we found no evidence for a moderating role of study quality. This negative finding strengthens robustness of the observed HRV alterations in MD.
Given the controversies about unfavorable effects of antidepressants on HRV (Kemp et al., Reference Kemp, Quintana, Gray, Felmingham, Brown and Gatt2010, Reference Kemp, Quintana and Malhi2011, Reference Kemp, Fráguas, Brunoni, Bittencourt, Nunes, Dantas, Andreão, Mill, Ribeiro, Koenig, Thayer, Benseñor and Lotufo2016; Licht et al., Reference Licht, de Geus, van Dyck and Penninx2010, Reference Licht, Penninx and de Geus2011; Huang et al., Reference Huang, Liao, Kuo, Chang, Chen, Chen and Yang2016), this meta-analysis with unmedicated samples clearly demonstrates that reductions in HRV are prevalent in depressed patients without antidepressants. However, effect sizes for differences in HRV measures between depressed patients and controls are substantially smaller than effects sizes for the reduction of HRV when starting the use of tricyclic or noradrenergic antidepressants (Licht et al., Reference Licht, de Geus, van Dyck and Penninx2010). These observations strengthen the assumption that HRV alterations in MD may be overestimated when studying patients who use antidepressants. In addition, although our findings result from cross-sectional analyses, the small to moderate effect sizes observed in this work may suggest that lower HRV does not completely explain the risk of CVD associated with MD. This is in line with previous research indicating that no single biological or behavioral factor accounts for more than a fraction of the total risk of CVD associated with depression (Carney and Freedland, Reference Carney and Freedland2017).
Our findings mostly support the results of a previous meta-analysis by Kemp et al. (Reference Kemp, Quintana, Gray, Felmingham, Brown and Gatt2010), although these authors report a larger elevation in LF/HF ratio in MD than in our analysis. A further difference is that our study indicates a significant reduction in LF-HRV in MD while Kemp et al. (Reference Kemp, Quintana, Gray, Felmingham, Brown and Gatt2010) found no evidence for differences in LF-HRV between patients and controls. Importantly, a recent meta-analysis focusing on late-life depression (Brown et al., Reference Brown, Karmakar, Grey, Jindal, Lim and Bryant2018) did not observe alterations in HF-HRV in MD but, consistent with our findings, a significant reduction in LF-HRV in MD (Brown et al., Reference Brown, Karmakar, Grey, Jindal, Lim and Bryant2018). Another recent meta-analysis of HRV alterations in childhood and adolescent depression suggests that HF-HRV was lower in children with depression than in controls, but the authors did not analyze LF-HRV alterations (Koenig et al., Reference Koenig, Kemp, Beauchaine, Thayer and Kaess2016). A possible reason why the present and previous meta-analyses (Kemp et al., Reference Kemp, Quintana, Gray, Felmingham, Brown and Gatt2010; Koenig et al., Reference Koenig, Kemp, Beauchaine, Thayer and Kaess2016) found differences in HF-HRV between MD and nondepressed controls while the meta-analysis including patients with late-life depression and older nondepressed participants did not (Brown et al., Reference Brown, Karmakar, Grey, Jindal, Lim and Bryant2018), may be because HF-HRV declines with aging (Jandackova et al., Reference Jandackova, Scholes, Britton and Steptoe2016). Further reasons for partly differing results are speculative but it is important to note that our work considers a substantially larger number of patients with MD for analyses than previous publications. Our findings might thus provide more robust results.
Given the increased risk of patients with MD for CVD (Rugulies, Reference Rugulies2002; Nicholson et al., Reference Nicholson, Kuper and Hemingway2006a, Reference Nicholson, Kuper and Hemingway2006b; van der Kooy et al., Reference van der Kooy, van Hout, Marwijk, Marten, Stehouwer and Beekman2007; Meng et al., Reference Meng, Chen, Yang, Zheng and Hui2012; Shaffer et al., Reference Shaffer, Whang, Shimbo, Burg, Schwartz and Davidson2012; Gan et al., Reference Gan, Gong, Tong, Sun, Cong, Dong, Wang, Xu, Yin, Deng, Li, Cao and Lu2014; Wu and Kling, Reference Wu and Kling2016), reduced HRV has been considered an indicator of autonomic imbalance and one potential mediator in the relationship of depression and other stress-related states and conditions with poor health outcomes (Kop et al., Reference Kop, Stein, Tracy, Barzilay, Schulz and Gottdiener2010; Kemp et al., Reference Kemp, Koenig and Thayer2017). Reduced HRV and other indicators of autonomic dysfunction interact with peripheral inflammation, a further potential pathway between MD and CVD (Howren et al., Reference Howren, Lamkin and Suls2009; Dowlati et al., Reference Dowlati, Herrmann, Swardfager, Liu, Sham, Reim and Lanctot2010; Rief et al., Reference Rief, Hennings, Riemer and Euteneuer2010; Haarala et al., Reference Haarala, Kähönen, Eklund, Jylhävä, Koskinen, Taittonen, Huupponen, Lehtimäki, Viikari, Raitakari and Hurme2011; Euteneuer et al., Reference Euteneuer, Mills, Rief, Ziegler and Dimsdale2012; Jarczok et al., Reference Jarczok, Koenig, Mauss, Fischer and Thayer2014; Halaris, Reference Halaris, Dantzer and Capuron2016). Reduced HRV is not a specific feature of depression but rather a transdiagnostic marker of health and well-being which can be affected by several medical, psychosocial and behavioral factors (e.g. medical conditions, drugs, nutrition, smoking, physical activity) (Rozanski et al., Reference Rozanski, Blumenthal, Davidson, Saab and Kubzansky2005; Eller et al., Reference Eller, Kristiansen and Hansen2011; Nemeroff and Goldschmidt-Clermont, Reference Nemeroff and Goldschmidt-Clermont2012; Elderon and Whooley, Reference Elderon and Whooley2013; Shaffer et al., Reference Shaffer, McCraty and Zerr2014; Cohen et al., Reference Cohen, Edmondson and Kronish2015; Pieritz et al., Reference Pieritz, Süssenbach, Rief and Euteneuer2016; Kemp et al., Reference Kemp, Koenig and Thayer2017; Gidron et al., Reference Gidron, Deschepper, De Couck, Thayer and Velkeniers2018; Young and Benton, Reference Young and Benton2018).
One important question in the context of HRV and depression is, whether interventions that aim to reduce depressive symptoms can also improve HRV in these patients. There is a large debate on whether antidepressants affect HRV. While tricyclic antidepressants seem to reduce HRV, findings for Serotonin Reuptake Inhibitors (SSRIs) or specific subgroups of SSRIs respectively are not clear or mainly result from cross-sectional studies which allow no causal assumptions (Kemp et al., Reference Kemp, Quintana, Gray, Felmingham, Brown and Gatt2010, Reference Kemp, Fráguas, Brunoni, Bittencourt, Nunes, Dantas, Andreão, Mill, Ribeiro, Koenig, Thayer, Benseñor and Lotufo2016, Reference Kemp, Quintana and Malhi2011; Licht et al., Reference Licht, Penninx and de Geus2011; Huang et al., Reference Huang, Liao, Kuo, Chang, Chen, Chen and Yang2016). The impact of psychological interventions on HRV in patients with MD without CVD is understudied and may be a promising aim for future studies. In an ongoing randomized controlled trial of our research group, we intend to address this issue by examining whether cognitive behavioral therapy, a standard treatment for depression, improve HRV in patients with MD (Euteneuer and Rief, Reference Euteneuer and Rief2016). Previous research indicates that cognitive behavioral therapy has beneficial effects on HRV in depressed patients with CVD and in older patients with manifest cardiovascular risk factors (Carney et al., Reference Carney, Freedland, Stein, Skala, Hoffman and Jaffe2005; Taylor et al., Reference Taylor, Conrad, Wilhelm, Strachowski, Khaylis, Neri, Giese-Davis, Roth, Cooke, Kraemer and Spiegel2009). Moreover, a recent preliminary study with a small sample of female college students with MD suggests that combining HRV biofeedback with psychotherapy reduces not only depressive symptoms but also increases HRV (Caldwell and Steffen, Reference Caldwell and Steffen2018). Therefore, from a translational perspective, future studies should examine (i) which kind of interventions improve HRV in MD and (ii) whether a potential increase in HRV reduces risk for CVD or possibly, reduces other biological risk factors for CVD (e.g. inflammation). In this context, it may be of relevance which mechanisms may underlie a potential increase in HRV during psychological treatments. Although speculative, potential mechanisms may include several interrelated factors such as cognitive-affective mechanisms (e.g. mood changes, better cognitive skills to cope with stressors) and behavioral factors (e.g. increased physical activity, relaxation).
This study has important strengths. To determine HRV alternations in MD this set of meta-analyses considered a total of N = 4220 subjects. We further conducted separate meta-analyses for specific HRV measures providing a more comprehensive picture for HRV alterations in MD. Finally, we examined the moderating role of study quality, which is per se an important contribution to the existing literature. This study also has limitations. A conservative approach was taken in the process of study selection. For example, studies, in which only a very few of the participants did not entirely fulfill our inclusion criteria (e.g. in one study some patients received a low dose of Lorazepam and in another, a low dose of benzodiazepines) were excluded from analyses. On the one hand, this procedure may draw a clear picture of the impact of MD on HRV in the absence of any medication effects. On the other hand, excluding samples with medication may also bias meta-analytic findings in terms of an underestimation of HRV alterations in MD. In addition, our meta-analyses based on cross-sectional studies and do not provide any causal explanation of the relationship between MD and HRV. In this context, it is important to note that we are not able to identify potential mediators between MD and reduced HRV, for example differences between patients and controls in lifestyle factors such as smoking or nutrition.
To conclude, this set of meta-analyses strengthens evidence that MD is associated with alterations in several measures of HRV, a transdiagnostic indicator of stress and cardiovascular health with potential predictive value for poor health outcomes.
Supplementary material
The supplementary material for this article can be found at https://doi.org/10.1017/S0033291719001351.
Acknowledgements
This work was supported, in part, by Grant EU 154/2-1 from the German Research Foundation (F.E.).
Author contributions
C.K. and F.E. conceptualized the study and extracted the data. F.E. and C.K. wrote the original draft. All authors reviewed and revised the original draft. C.K. performed statistical analysis. S.S. and M.W. rated the study quality.
Conflict of interest
None.