Introduction
A recent case-control genome-wide association study (GWAS) identified, for the first time, 12 independent loci significantly associated with attention-deficit/hyperactivity disorder (ADHD) (Demontis et al., Reference Demontis, Walters, Martin, Mattheisen, Als, Agerbo and Neale2019). This GWAS enables further genetic investigations using polygenic risk scores (PRS), which are calculated for each individual by computing the sum of their risk alleles across the genome, weighted by effect sizes (Choi, Mak, & O'Reilly, Reference Choi, Mak and O'Reilly2018). PRS provide an estimate of the genetic propensity to ADHD at the individual level that can be used to investigate shared genetic etiology between ADHD and other phenotypes.
Previous studies on general population samples show that ADHD PRS are associated with a wide range of psychiatric and somatic disorders and traits, such as depression, anxiety, neuroticism, irritability, childhood internalizing and externalizing symptoms, obesity-related phenotypes and smoking (Brikell et al., Reference Brikell, Larsson, Lu, Pettersson, Chen, Kuja-Halkola and Martin2018; Du Rietz et al., Reference Du Rietz, Coleman, Glanville, Choi, O'Reilly and Kuntsi2018; Riglin et al., Reference Riglin, Eyre, Cooper, Collishaw, Martin, Langley and Thapar2017). Only a few of these population-based studies explored the cognitive phenotypes associated with ADHD using polygenic approaches, but have provided initial evidence for an association between PRS for ADHD and lower general cognitive ability (Du Rietz et al., Reference Du Rietz, Coleman, Glanville, Choi, O'Reilly and Kuntsi2018; Martin, Hamshere, Stergiakouli, O'Donovan, & Thapar, Reference Martin, Hamshere, Stergiakouli, O'Donovan and Thapar2015a), educational attainment (Stergiakouli et al., Reference Stergiakouli, Martin, Hamshere, Heron, St Pourcain, Timpson and Davey Smith2017) and working memory, but not inhibition impairments (measured with the Opposite Words Task; Martin et al., Reference Martin, Hamshere, Stergiakouli, O'Donovan and Thapar2015a). Evidence from clinically diagnosed samples with ADHD remains even more limited. The findings reported to date indicate an association of ADHD PRS with low academic achievement (Vuijk et al., Reference Vuijk, Martin, Braaten, Genovese, Capawana, O'Keefe and Doyle2019) and poor working memory and arousal-alertness, measured with latent variables (Nigg et al., Reference Nigg, Gustafsson, Karalunas, Ryabinin, McWeeney, Faraone and Wilmot2018). In contrast, no significant associations emerged between PRS for ADHD and latent variables capturing inhibition or speed of responses (Nigg et al., Reference Nigg, Gustafsson, Karalunas, Ryabinin, McWeeney, Faraone and Wilmot2018). A recent study found that PRS for ADHD were associated with a measure of interference, the ‘variance of word interference time’ in the Stroop test (Chang, Yang, Wang, & Faraone, Reference Chang, Yang, Wang and Faraone2020).
We now extend, in a sample of 845 people with ADHD, the previous PRS investigations of ADHD-related cognitive phenotypes to two cognitive measures that have extensive evidence from phenotypic studies of a strong association with ADHD, but have not yet been investigated using PRS: increased reaction time variability (RTV) and commission errors (CE) (Kuntsi et al., Reference Kuntsi, Wood, Rijsdijk, Johnson, Andreou, Albrecht and Asherson2010; Loo et al., Reference Loo, Hale, Macion, Hanada, McGough, McCracken and Smalley2009; Schachar et al., Reference Schachar, Logan, Robaey, Chen, Ickowicz and Barr2007; van Rooij et al., Reference van Rooij, Hartman, Mennes, Oosterlaan, Franke, Rom-melse and Hoekstra2015). RTV captures the highly variable speed of responding that is strongly characteristic of people with ADHD across a variety of cognitive tasks requiring a fast response (Kofler et al., Reference Kofler, Rapport, Sarver, Raiker, Orban, Friedman and Kolomeyer2013; Kuntsi et al., Reference Kuntsi, Frazier-Wood, Banaschewski, Gill, Miranda, Oades and Rijsdijk2013), and has been linked in EEG and skin conductance studies to attention allocation and peripheral hypo-arousal (Cheung et al., Reference Cheung, McLoughlin, Brandeis, Banaschewski, Asherson and Kuntsi2017; James, Cheung, Rijsdijk, Asherson, & Kuntsi, Reference James, Cheung, Rijsdijk, Asherson and Kuntsi2016). CE, which represent the responses to non-target stimuli on inhibitory tasks such as the Go/No-Go task, capture failures to withhold responding.
Family and twin studies suggest a significant degree of familial/genetic sharing between ADHD and both RTV and CE (Kuntsi et al., Reference Kuntsi, Wood, Rijsdijk, Johnson, Andreou, Albrecht and Asherson2010, Reference Kuntsi, Pinto, Price, van der Meere, Frazier-Wood and Asherson2014). For example, in a large study of 1265 children and adolescents, including 464 participants with ADHD, we observed a familial correlation of 0.74 between ADHD and RTV, and 0.45 between ADHD and CE (Kuntsi et al., Reference Kuntsi, Wood, Rijsdijk, Johnson, Andreou, Albrecht and Asherson2010). The analyses further indicated a significant degree of etiological separation in the association of ADHD with RTV and CE (Kuntsi et al., Reference Kuntsi, Wood, Rijsdijk, Johnson, Andreou, Albrecht and Asherson2010), with a similar conclusion emerging also from model fitting analyses in a population twin sample of 1312 children (Kuntsi et al., Reference Kuntsi, Pinto, Price, van der Meere, Frazier-Wood and Asherson2014). Family model fitting analyses also showed a high familial correlation between RTV obtained from two different tasks (a four-choice reaction time task, the Fast task, and a Go/No-Go task; rf = 0.75) (Kuntsi et al., Reference Kuntsi, Wood, Rijsdijk, Johnson, Andreou, Albrecht and Asherson2010), suggesting RTV can be combined across such tasks for further genetic investigations.
Using a polygenic approach, we can move beyond the inferred etiological sharing between ADHD and RTV or CE that rely on comparisons of related individuals (in twin and family designs), to test the associations using molecular genetic data in unrelated individuals. Specifically, in this collaborative study using ADHD samples from several international sites, we derive PRS for ADHD from the recent GWAS (Demontis et al., Reference Demontis, Walters, Martin, Mattheisen, Als, Agerbo and Neale2019) to test whether genetic variants that contribute to ADHD also influence the cognitive impairments captured by RTV and CE in people with ADHD.
Methods
Discovery sample
As the discovery dataset, we used the Psychiatric Genomics Consortium (PGC) and iPSYCH Danish data analyzed in the recently published GWAS of ADHD (Demontis et al., Reference Demontis, Walters, Martin, Mattheisen, Als, Agerbo and Neale2019). This GWAS consists of 11 studies, with a total of 19 099 ADHD cases and 34 194 control subjects of European ancestry (full sample sizes are given in online Supplementary Table S1).
Target samples and cognitive assessments
From the above discovery sample, four sub-samples from different sites were used as target samples applying a leave-one-study-out approach: International Multisite ADHD Genetics Project (IMAGE-I, subdivided here to IMAGE-8 and IMAGE-Dutch that had different cognitive test batteries), University of California Los Angeles (UCLA), Toronto and Barcelona. All participants for each site completed a comprehensive protocol of cognitive tasks, which differed for each site. Participants from IMAGE-8 performed a four-choice reaction time task (Fast task) and a version of the Go/No-Go task with fast and slow conditions, whereas IMAGE-Dutch participants performed the Stop-Signal Task (SST). At UCLA and Barcelona, participants performed the Continuous Performance Test II (CPT-II), whereas the Go/No-Go task was administered in Toronto. Descriptive statistics for each sample are shown in Table 1. Based on previous publications, cognitive variables were selected from the tasks that showed a significant ADHD case-control difference (effect sizes ranging from 0.32 to 0.95 for RTV, and from 0.38 to 0.42 for CE; Alemany et al., Reference Alemany, Ribasés, Vilor-Tejedor, Bustamante, Sánchez-Mora, Bosch and Sunyer2015; Hale et al., Reference Hale, Kane, Tung, Kaminsky, McGough, Hanada and Loo2014; Kuntsi et al., Reference Kuntsi, Wood, Rijsdijk, Johnson, Andreou, Albrecht and Asherson2010; Schachar et al., Reference Schachar, Logan, Robaey, Chen, Ickowicz and Barr2007; van Rooij et al., Reference van Rooij, Hartman, Mennes, Oosterlaan, Franke, Rom-melse and Hoekstra2015). RTV [standard deviation (s.d.) of reaction times] was obtained from each of the tasks. Evidence for comparability between tasks was previously obtained from model fitting analyses on the fast task and Go/No-Go task, which indicated a high familial correlation (rf = 0.75) between RTVs obtained from each task, suggesting they are measuring largely the same liability (Kuntsi et al., Reference Kuntsi, Wood, Rijsdijk, Johnson, Andreou, Albrecht and Asherson2010). CE was obtained from the CPT-II and Go/No-Go tasks only. The high rates of Go-stimuli in the CPT-II task make this task comparable to a Go/No-Go task.
Table 1. Descriptive statistics for all samples

IMAGE, International Multisite ADHD Genetics Project; UCLA, University of California Los Angeles.
IMAGE-I
Sample: IMAGE-I is a European project on ADHD familiality using a common protocol of centralized training and data management. IMAGE-I includes data from different European sites and Israel, recruited from specialist clinics in Tel-Aviv, Essen, Gottingen, Brussels, Dublin, Valencia, Zurich, London, Nijmegen and Amsterdam (Kuntsi, Neale, Chen, Faraone, & Asherson, Reference Kuntsi, Neale, Chen, Faraone and Asherson2006a; Müller et al., Reference Müller, Asherson, Banaschewski, Buitelaar, Ebstein, Eisenberg and Steinhausen2011a; Müller et al., Reference Müller, Asherson, Banaschewski, Buitelaar, Ebstein, Eisenberg and Steinhausen2011b). The full IMAGE-I sample consisted of 782 individuals with DSM-IV ADHD combined type (680 ADHD combined type probands including 102 of their siblings who also met criteria for ADHD) and 808 additional unaffected siblings aged 6–19 years (Kuntsi et al., Reference Kuntsi, Neale, Chen, Faraone and Asherson2006a). All participants were recruited from specialist clinics. In IMAGE-I, parents of children were interviewed by trained researchers with the Parental Account of Childhood Symptom (PACS), a semi-structured, standardized, investigator-based interview developed as an instrument to provide an objective measure of child behavior. Both parents and teachers completed the respective versions of the Conners' ADHD rating scales and the Strengths and Difficulties Questionnaire (SDQ). Exclusion criteria were autism, epilepsy, IQ < 70, brain disorders and any genetic or medical disorder associated with externalizing behaviors that might mimic ADHD. Wherever possible, families withdrew stimulant medication for 1 week prior to research assessments to allow for more accurate ascertainment of the current level of ADHD symptoms and behaviors. Alternatively, clinical interviews were based on medication-free periods. A minimum of a 48-h medication-free period was required for cognitive testing. All data were collected with informed consent of the parents and with the approval of the site's Institutional Review Board (IRB) or Ethical Committee.
Due to differences in the protocol of the cognitive tasks, IMAGE-I can be subdivided into two subsamples: IMAGE-8 (including participants from Tel-Aviv, Essen, Gottingen, Brussels, Dublin, Valencia, Zurich and London) and IMAGE-Dutch (including participants from Nijmegen and Amsterdam). In the current study, we included only participants with an ADHD diagnosis who had both cognitive and genetic data available. The final sample consisted of 143 ADHD participants from the IMAGE-8 study and 226 ADHD participants from the IMAGE-Dutch study.
Tasks: Fast-Task, Go/No-Go and SST: The Fast task is a computerized four-choice reaction time (RT) task which measures performance under a baseline (slow-unrewarded) and a fast-incentive condition (Andreou et al., Reference Andreou, Neale, Chen, Christiansen, Gabriels, Heise and Kuntsi2007; Kuntsi et al., Reference Kuntsi, Rogers, Swinard, Börger, van der Meere, Rijsdijk and Ash-erson2006b). In the current study, only data from the baseline condition was included as this condition is more sensitive to ADHD (Kuntsi et al., Reference Kuntsi, Frazier-Wood, Banaschewski, Gill, Miranda, Oades and Rijsdijk2013). The baseline condition consisted of 72 trials. Four empty circles (warning signals, arranged horizontally) first appeared for 8 s, after which one of them (the target) was colored in. Participants were asked to press the response key that corresponded to the position of the target. Following a response, the stimuli disappeared from the screen and a fixed inter-trial interval of 2.5 s followed. Speed and accuracy were emphasized equally.
The Go/No-Go task is a computerized test used to assess inhibitory control (Börger & van der Meere, Reference Börger and van der Meere2000; Kuntsi, Andreou, Ma, Börger, & van der Meere, Reference Kuntsi, Andreou, Ma, Börger and van der Meere2005; van der Meere, Stemerdink, & Gunning, Reference van der Meere, Stemerdink and Gunning1995). On each trial of the Go/No-Go task, one of two possible stimuli appeared for 300 ms in the middle of the computer screen. The child was instructed to respond only to the Go stimuli and to withhold their response to No-Go stimuli. Participants were asked to react as quickly as possible while maintaining a high level of accuracy. The proportion of Go stimuli to No-Go stimuli was 4:1. This version of the Go/No-Go task consisted of three conditions (slow, fast and incentive). Here, we use data only from the slow condition, which show a strong association with ADHD (Andreou et al., Reference Andreou, Neale, Chen, Christiansen, Gabriels, Heise and Kuntsi2007; Kuntsi, Wood, Van Der Meere, & Asherson, Reference Kuntsi, Wood, Van Der Meere and Asherson2009; Uebel et al., Reference Uebel, Albrecht, Asherson, Börger, Butler, Chen and Banaschewski2010). The slow condition consisted of 72 trials and were presented with a fixed inter-stimulus interval of 8 s.
The SST is a response inhibition task, where participants had to respond as quickly as possible to a Go stimulus by left or right button press, unless shortly after presentation it was followed by a Stop signal, in which case they were to withhold their response (25% of trials) (Logan, Cowan, & Davis, Reference Logan, Cowan and Davis1984). The task difficulty was adaptive, meaning delays between the Go and Stop stimulus were adjusted by 50 ms after every failed or successful response, leading to an approximate 50% success rate on the Stop-trials for all participants. The task consisted of two practice blocks and four test blocks, each consisting of 60 trials.
UCLA
Sample: At UCLA, 156 participants with ADHD were recruited as part of the PUWMa collaboration [Pfizer-funded study from the University of California, Los Angeles (UCLA), Washington University, and Massachusetts General Hospital (MGH)], which included 540 children and adolescents aged 5–18 years, and 519 of their parents, ascertained from 370 families with ADHD-affected sibling pairs. Children and adolescents were assessed according to DSM-IV-TR criteria. Families were recruited through clinical referrals, schools and responses to advertisements (e.g. newsletters, community newspapers or flyers distributed at parent meetings in the greater Los Angeles area). Respondents without a previous diagnosis of ADHD were screened with the parent and teacher version of the Swanson, Nolan and Pelham Rating Scale, SNAP-IV (Swanson et al., Reference Swanson, Schuck, Porter, Carlson, Hartman, Sergeant and Wigal2012). After initial screening, children and adolescents were assessed by master's level clinical psychologists or highly trained interviewers using the Schedule for Affective Disorders and Schizophrenia for School-Age Children-Present and Lifetime version (K-SADS-PL), as well as a parent-completed Child Behaviour Checklist (CBCL) and Teacher Report Form. Participants were excluded if they were positive for any of the following: neurological disorder, head injury resulting in concussion, lifetime diagnoses of schizophrenia or autism or estimated IQ < 70. Participants on stimulant medication were asked to discontinue use for 24 h prior to their visit. The final sample with both cognitive and genetic data available consists of 55 ADHD cases.
Task: CPT-II: The CPT-II (Conners, Reference Conners2000) is a 14-min computerized task that consisted of six blocks and three sub-blocks. Participants were required to press the space button on the keyboard whenever any letter except the letter ‘X’ appeared on the computer screen. The task consisted of 360 trials, including 36 presentations of the inhibition target (X). Targets (including ‘go’ targets: A, B, C, D, F, I, L, O, T) were presented in randomized order for 250-ms with variable inter-trial interval of 750, 1750 and 3750 ms. The presentation order of the different inter-trial intervals varied between blocks. The Go:No/Go ratio was 9:1.
Barcelona
Sample: The Spanish sample included 607 adults with ADHD (age range 18–40 years), recruited and evaluated at the Hospital Universitari Vall d'Hebron in Barcelona. The diagnosis of ADHD was evaluated by clinicians with the Structured Clinical Interview for DSM-IV Axis I and II Disorders (SCID-I and SCID-II) and the Conner's Adult ADHD Diagnostic Interview for DSM-IV (CAADID Parts I and II). Exclusion criteria were IQ < 70, schizophrenia or other psychotic disorders, ADHD symptoms due to mood, anxiety, dissociative or personality disorders, adoption, sexual or physical abuse, birth weight <1.5 kg and other neurological or systemic disorders that might explain ADHD symptoms. Cognitive and genetic data were available from 367 ADHD participants. More information about this sample can be found elsewhere (Sánchez-Mora et al., Reference Sánchez-Mora, Ramos-Quiroga, Bosch, Corrales, Garcia-Martínez, Nogueira and Ribasés2015).
Task: CPT-II: See UCLA for task description.
Toronto
Sample: The initial Canadian ADHD sample included 248 children aged 6–16 years referred for ADHD, learning and/or behavioral problems to the Hospital for Sick Children, Toronto (Lionel et al., Reference Lionel, Crosbie, Barbosa, Goodale, Thiruvahindrapuram, Rickaby and Scherer2011). ADHD diagnostic information was obtained based on DSM-IV criteria from parents and teachers in semi-structured clinical interviews including the Parent Interview for Child Symptoms (PICS) and the Teacher Telephone Interview (TTI). The assessments were conducted by a social worker, a clinical nurse specialist or a clinical psychologist and supervised by a clinical psychologist or child psychiatrist. Exclusion criteria were an IQ < 80, pervasive developmental disorder, autism or comorbid psychiatric disorder that could better account for the disorder Participants who were treated with stimulant medication had to be unmedicated for a minimum of 24 h before assessment and testing. Cognitive and genetic data for this study were available from 54 children with ADHD.
Task: Go/No-Go task: This version of the Go/No-Go task involved 128 trials of which 32 were No-Go trials and 96 were Go trials. During the Go task, one of two possible letters was presented (an X or an O) on each trial. Participants were required to make a response to the Go task stimuli as quickly and as accurately as possible by pressing one key of a handheld response box for an X and the other for an O (Go stimuli). The No-Go task involved an auditory tone which was presented, at the same time as the stimulus (letters), at random, on 25% of trials. Participants were instructed to withhold their response when they heard the tone. The Go task stimulus was presented for 1000 ms immediately following a fixation point of 500 ms. The task included four blocks, each with 24 Go trials and eight No-Go Trials. The Go:No-Go ratio was 3:1.
Data analyses
Quality control of genetic and cognitive data
Quality control of genetic data was previously performed and was available for analyses (for more information see Demontis et al., Reference Demontis, Walters, Martin, Mattheisen, Als, Agerbo and Neale2019).
To account for positive skewness of the cognitive data, we applied appropriate transformations to all cognitive measures for each variable prior to analyses. Square root transformations were used in all samples for CE. For RTV, we used a logarithm transformation for IMAGE-8 team, Dutch-IMAGE and UCLA, and square root transformation for Barcelona and Toronto. There were no extreme outliers for RTV or CE (>3.5 s.d.).
PRS analyses
The GWAS summary statistics used as the discovery sample included the four target sub-groups (IMAGE-I, Toronto, Barcelona and UCLA). For this reason, PRS were calculated from the main GWAS each time excluding one of the target samples using four leave-one-out association meta-analyses, to ensure entirely independent discovery and target samples. PRS were estimated for each target sample using PRSice-2 software (Euesden, Lewis, & O'Reilly, Reference Euesden, Lewis and O'Reilly2015) (https://www.prsice.info) and applying standard procedures (imputation quality cut-off using PRSice INFO >0.9, and minor allele frequencies cut-off using PRSice MAF > 0.05) (Choi et al., Reference Choi, Mak and O'Reilly2018). PRSice computes PRS by calculating the sum of trait-associated alleles, weighted by the log odds ratio generated from the discovery GWAS. An R 2 ⩾ 0.1 (250-kb window) including all single-nucleotide polymorphisms (SNPs) (p 1, p 2 = 1) was used for linkage disequilibrium (LD) clumping to keep a set of independent SNPs. Linear regression models were used to estimate associations between PRS and phenotypes in the target samples. PRS were calculated at a number of p value thresholds for SNP inclusion to provide the most predictive PRS. The p value thresholds used were 0.001, 0.05, 0.1, 0.2, 0.3, 0.4, 0.5 and 1. We included age, sex and the first five principal components (PCs) as covariates in all analyses, to control for population stratification. The number of PCs was chosen based on the cohort's sample size (all <1000) in order to avoid overfitting and to reflect the differential power to capture true population structure by principal component analysis, as reported in Demontis et al. (Reference Demontis, Walters, Martin, Mattheisen, Als, Agerbo and Neale2019). The estimated amount of variance explained by PRS (i.e. R 2 values) that we report for each study are adjusted from a baseline model including the covariates; the reported regression coefficients and standard errors (s.e.) were standardized to have mean = 0 and s.d. 1 using the PRSice command (--score std). We performed stringent permutation testing within PRSice-2 using 10 000 permutations to control for type 1 error and to prevent data overfitting across the range of p value thresholds considered (0.001, 0.05, 0.1, 0.2, 0.3, 0.4, 0.5 and 1). The p values are reported before correction (indicated with ‘p’), and after correction (indicated with ‘empirical-p’). Online Supplementary Figs S1–S9 provides plots for the PRS prediction models for RTV and CE across all sites.
Meta-analyses
For the meta-analyses, we used a random effects model using the rma.uni function of the metafor package in R, with the method set to ‘REML’. Meta-analyses for both RTV and CE were performed across all samples at all thresholds to check the consistency of the associations between PRS and these measures (online Supplementary Tables S2 and S3). Combining all samples, the sample size for the meta-analysis consisted of n = 743 ADHD participants for RTV and n = 679 ADHD participants for CE.
Results
PRS in individual datasets
PRS for ADHD were not significantly associated with RTV in any of the individual datasets (R 2 = 0.004, p = 0.771, empirical-p = 0.993, β = 0.024 for IMAGE-8; R 2 = 0.016, p = 0.124, empirical-p = 0.317, β = 0.135 for IMAGE-Dutch; R 2 = 0.008, p = 0.466, empirical-p = 0.823, β = 0.032 for UCLA; R 2 = 0.031, p = 0.362, empirical-p = 0.459, β = 0.112 for Toronto; R 2 = 0.012, p = 0.029, empirical-p = 0.079, β = 0.122 for Barcelona). All associations showed a positive direction. PRS for ADHD were not significantly associated and showed inconsistent direction of association with CE in any of the individual samples (R 2 = 0.011, p = 0.085, empirical-p = 0.217, β = −0.104 for IMAGE-8; R 2 = 0.036, p = 0.188, empirical-p = 0.556, β = 0.101 for UCLA; R 2 = 0.013, p = 0.407, empirical-p = 0.761, β = −0.121 for Toronto; R 2 = 0.006, p = 0.122, empirical-p = 0.301, β = 0.083 for Barcelona).
Meta-analysis of all datasets
Meta-analysis across all thresholds for RTV showed that the best threshold for PRS association with RTV was 0.2 (online Supplementary Table S2). At this threshold, the PRS for ADHD was significantly associated with RTV (R 2 = 0.011 p = 0.022, β = 0.088), with a positive direction. The best threshold for PRS association with CE was 0.001 (online Supplementary Table S3), but the association with CE did not reach significance CE (R 2 = 0.011, p = 0.732, β = 0.013). Heterogeneity tests showed low heterogeneity across studies for both measures (Q = 3.777, p = 0.436, I 2 = 13.513% for RTV; Q = 1.195, p = 0.754, I 2 = 0% for CE). Forest plots for each variable are reported in Figs 1 and 2.
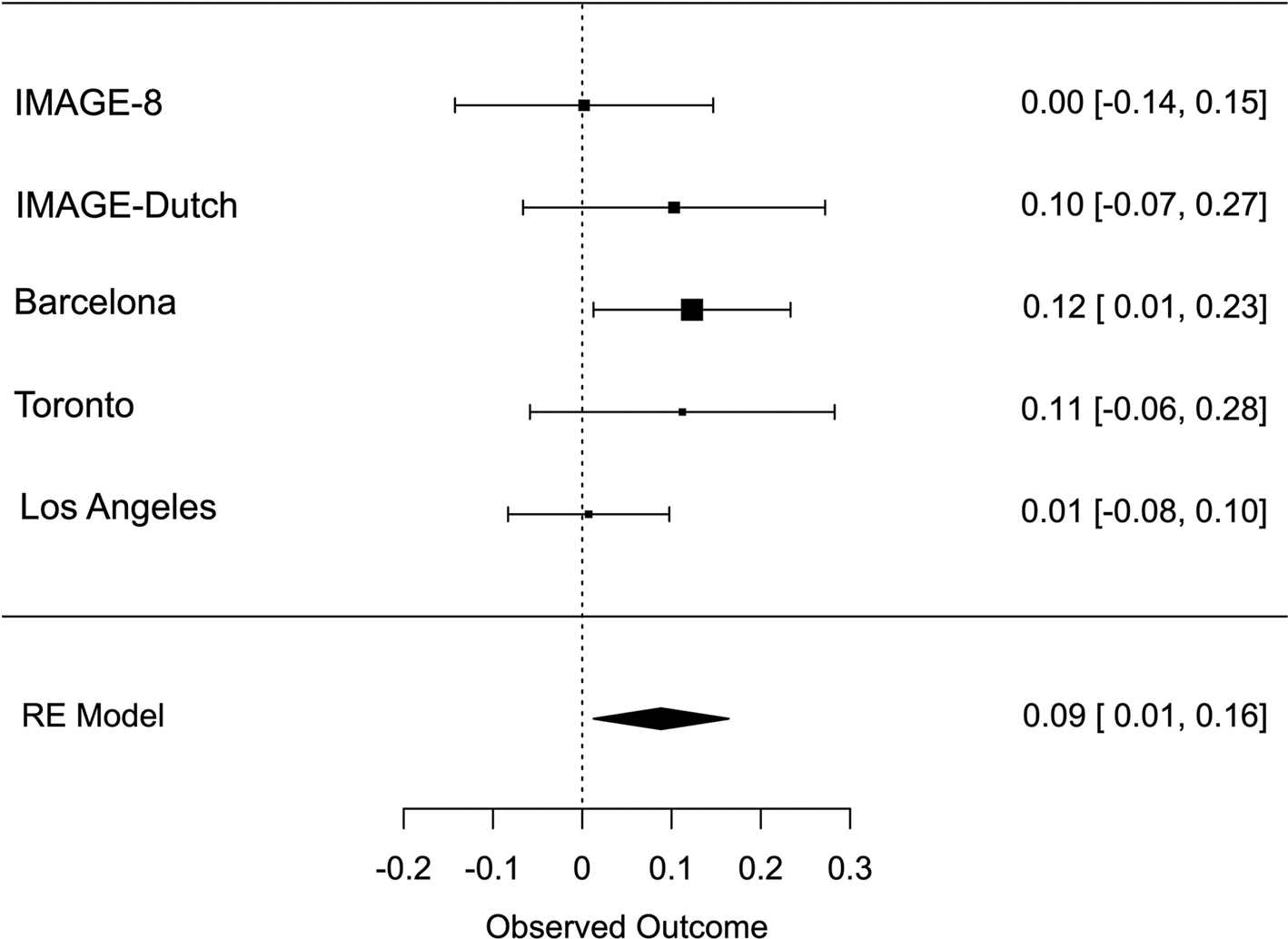
Fig. 1. Forest plot of the meta-analysis of RTV. The overall estimate from random effects model is represented by the diamond below the individual study estimates.

Fig. 2. Forest plot of the meta-analysis of CE. The overall estimate from random effects model is represented by the diamond below the individual study estimates.
Discussion
This is one of the largest studies investigating the association between ADHD PRS and cognitive impairments in individuals diagnosed with ADHD. Combining our samples in meta-analyses, our results show that polygenic risk for clinically diagnosed ADHD is positively associated with higher RTV, but not with CE as measured by Go/No Go tasks. These data suggest that common genetic variation relevant for ADHD influences attention regulation (RTV) but not response inhibition processes (CE) in a clinical ADHD sample. Whether the lack of an association with CE could reflect possible involvement of rare variants not detectable in this analysis or limited power to detect a potentially smaller association, requires further study.
Our results on RTV build on previous evidence from a smaller sample of children with ADHD showing a significant positive association between a latent variable of arousal-alertness and PRS for ADHD (Nigg et al., Reference Nigg, Gustafsson, Karalunas, Ryabinin, McWeeney, Faraone and Wilmot2018). Of note, the association we observed between PRS for ADHD and RTV was mostly consistent across all p value thresholds in the meta-analysis, with only slight fluctuations in results possibly due to low power. Similarly, our results on CE are consistent with a previous population-based study and a clinical study showing no association between polygenic risk for ADHD and other inhibition measures (Martin et al., Reference Martin, Hamshere, Stergiakouli, O'Donovan and Thapar2015a; Nigg et al., Reference Nigg, Gustafsson, Karalunas, Ryabinin, McWeeney, Faraone and Wilmot2018), although a recent study did report an association between PRS for ADHD and interference when measured with the variance of word interference time in the Stroop test (Chang et al., Reference Chang, Yang, Wang and Faraone2020). Previous twin and sibling analyses have indicated a degree of shared genetic/familial influences on ADHD and response inhibition (Kuntsi et al., Reference Kuntsi, Rogers, Swinard, Börger, van der Meere, Rijsdijk and Ash-erson2006b; Kuntsi et al., Reference Kuntsi, Wood, Rijsdijk, Johnson, Andreou, Albrecht and Asherson2010). Further evidence from a sibling study suggested in fact two familial cognitive impairment factors for ADHD: a larger factor (85% of familial variance of ADHD) related to RTV, and a smaller factor (12.5% of familial variance of ADHD) capturing CE and omission errors (an overall measure of task accuracy) (Kuntsi et al., Reference Kuntsi, Wood, Rijsdijk, Johnson, Andreou, Albrecht and Asherson2010). The findings from the sibling and twin studies (Kuntsi et al., Reference Kuntsi, Wood, Rijsdijk, Johnson, Andreou, Albrecht and Asherson2010, Reference Kuntsi, Pinto, Price, van der Meere, Frazier-Wood and Asherson2014) suggested a potential separation, at the genetic level, between attention regulation and response inhibition processes in their association with ADHD. It is possible that our current analyses detected the larger factor accounted for by RTV in the sibling analyses (Kuntsi et al., Reference Kuntsi, Wood, Rijsdijk, Johnson, Andreou, Albrecht and Asherson2010) while the smaller factor (accounting for CE) could not be detected with the current sample size. Future studies should investigate the genetic correlation between ADHD and RTV or CE across the whole genome using LD score regression, when summary statistics from GWAS on the appropriate cognitive traits will be available.
Although PRSs capture the common risk alleles that contribute to clinically diagnosed ADHD, they do not incorporate contributions from other genetic factors, such as copy number variants (CNVs) and single-nucleotide variants (SNVs) that may underlie the association of ADHD with RTV or CE. Several studies indicate a role for CNVs and SNVs in contributing to ADHD risk (Martin, O'Donovan, Thapar, Langley, & Williams, Reference Martin, O'Donovan, Thapar, Langley and Williams2015b; Satterstrom et al., Reference Satterstrom, Walters, Singh, Wigdor, Lescai, Demontis and Daly2018; Thapar et al., Reference Thapar, Martin, Mick, Arias Vásquez, Langley, Scherer and Holmans2016; Williams et al., Reference Williams, Franke, Mick, Anney, Freitag, Gill and Faraone2012; Williams et al., Reference Williams, Zaharieva, Martin, Langley, Mantripragada, Fossdal and Thapar2010; Yang et al., Reference Yang, Neale, Liu, Lee, Wray, Ji and Subgroup2013). CNVs were shown to be associated with cognitive features in the general population such as general cognitive ability (MacLeod et al., Reference MacLeod, Davies, Payton, Tenesa, Harris, Liewald and Deary2012), educational and occupational attainment (Kendall et al., Reference Kendall, Rees, Escott-Price, Einon, Thomas, Hewitt and Kirov2017; Männik et al., Reference Männik, Mägi, Macé, Cole, Guyatt, Shihab and Reymond2015), and other cognitive phenotypes such as working memory, episodic memory, speed processing, visual attention and fluid intelligence (Kendall et al., Reference Kendall, Rees, Escott-Price, Einon, Thomas, Hewitt and Kirov2017). Similarly, SNVs have been implicated in intellectual disability (Satterstrom et al., Reference Satterstrom, Walters, Singh, Wigdor, Lescai, Demontis and Daly2018). Yet, the extent to which CNVs and other genetic variants may contribute to cognitive impairments in individuals with ADHD is poorly understood and is an important direction for future research.
Although this is the largest study to date to investigate RTV and CE with a cutting-edge PRS method in a sample of individuals with clinically diagnosed ADHD, certain limitations need to be considered. First, our individual study analyses were underpowered due to the small sample sizes available in each single study. To increase statistical power, we analyzed the target studies with meta-analyses, reaching a combined sample size of n = 743 ADHD participants for RTV and n = 679 ADHD participants for CE; yet future studies, ideally with larger samples, are needed to replicate these results. Second, the age range of our participants was wide (8–45 years old). It would be informative in future larger studies to explore results separately for participants of different age groups (children, young adults and older adults). Third, our study included only participants of European ancestry; the generalizability of our findings to non-European populations requires further investigation. Fourth, the use of different tasks to reflect the two constructs of interest at different sites could have introduced heterogeneity in our data; however, we used random effects in the meta-analyses to account for between-study variation across sites. A further direction for future research is to widen the PRS investigation to additional cognitive impairments associated with ADHD.
Overall, polygenic risk associated with clinical ADHD diagnosis was associated with higher RTV in individuals with clinically diagnosed ADHD. Our results provide molecular genetic evidence that attention regulation and ADHD share common genetic factors. In other words, ADHD common variants not only contribute to risk of ADHD diagnosis, but are also a marker of poorer RTV performance in the context of having such a diagnosis. Further investigation, with bigger sample sizes, is needed to replicate these findings and to further determine the neurobiological mechanisms underlying this association. Furthermore, it is unknown whether the findings reported here are specific to ADHD or generalize to other disorders where increased RTV is also observed (such as bipolar disorder, schizophrenia and autism) (Brotman, Rooney, Skup, Pine, & Leibenluft, Reference Brotman, Rooney, Skup, Pine and Leibenluft2009; Kaiser et al., Reference Kaiser, Roth, Rentrop, Friederich, Bender and Weisbrod2008; Karalunas, Geurts, Konrad, Bender, & Nigg, Reference Karalunas, Geurts, Konrad, Bender and Nigg2014).
Supplementary material
The supplementary material for this article can be found at https://doi.org/10.1017/S0033291720005218
Acknowledgements
Isabella Vainieri is supported by a 3-year Ph.D. studentship awarded by the Social, Genetic and Developmental Psychiatry Centre, Institute of Psychiatry, Psychology and Neuroscience, King's College London. This research has been conducted using PGC ADHD Resources. We acknowledge the following grant support: UK Medical Research Council grant G03001896 (to J. Kuntsi), NIH grants R01MH62873 and R01MH081803 (to S. Faraone), R01MH116037 (to A. Doyle) and RO1NS054124 (to S. Loo), Ministerio de Ciencia e Innovacion, Spain, RTI2018-100968-B-100, AGAUR, Generalitat de Catalunya, 2017-SGR-738, the Canadian Institute of Health Research MOP-93696 (to R. Schachar), and European Commission H2020 grants 667302 (CoCA), 643051 and 728018 (to B. Cormand), 847181 (to J. Buitelaar). Over the course of this investigation, Marta Ribasés was a recipient of a Miguel de Servet contract from the Instituto de Salud Carlos III, Spain (CP09/00119 and CPII15/00023). The research leading to these results has received funding from the Instituto de Salud Carlos III (PI16/01505, PI17/00289, PI18/01788, PI19/00721 and PI19/01224), and co-financed by the European Regional Development Fund (ERDF), the Agència de Gestió d'Ajuts Universitaris i de Recerca-AGAUR (2017SGR1461) and the Health Research and Innovation Strategy Plan (PERIS-SLT006/17/287), Generalitat de Catalunya, Spain. Finally, we would also like to acknowledge the ADHD Working Group of the Psychiatric Genomics Consortium. Correspondence and requests for materials should be addressed to Prof Jonna Kuntsi.
Conflict of interest
Professor Jonna Kuntsi has given talks at educational events sponsored by Medice; all funds are received by King's College London and used for studies of ADHD. Professor Philip Asherson has received funding for research by Vifor Pharma and has given sponsored talks and been an advisor for Shire, Janssen-Cilag, Eli-Lilly, Flynn Pharma and Pfizer, regarding the diagnosis and treatment of ADHD. All funds are received by King's College London and used for studies of ADHD. Professor Iris Manor has received funding for research by Alcobra Ltd., and from Enzymotec Ltd., and all funds were received by Geha MHC for research. She has also given sponsored talks and been an advisor for Shire, Janssen-Cilag, Teva Israel, Medison and Novartis Israel regarding the diagnosis and treatment of ADHD. In the last 36 months, Professor Hans-Christoph Steinhausen has worked as a speaker for an educational event sponsored by Medice and has received book royalties from Cambridge University Press, Elsevier, Hogrefe, Huber, Klett and Kohlhammer publishers. Professor Banaschewski served in an advisory or consultancy role for Lundbeck, Medice, Neurim Pharmaceuticals, Oberberg GmbH, Shire and Infectopharm. He received conference support or speaker's fee by Lilly, Medice and Shire. He received royalties from Hogrefe, Kohlhammer, CIP Medien, Oxford University Press; the present work is unrelated to these relationships. Professor Jan K Buitelaar has been in the past 3 years a consultant to/member of advisory board of/and/or speaker for Takeda/Shire, Roche, Medice, Angelini, Janssen and Servier. He is not an employee of any of these companies, and not a stock shareholder of any of these companies. He has no other financial or material support, including expert testimony, patents, and royalties. In the past year, Professor Faraone received income, potential income, travel expenses continuing education support and/or research support from Takeda, OnDosis, Tris, Otsuka, Arbor, Ironshore, Rhodes, Akili Interactive Labs, Enzymotec, Sunovion, Supernus and Genomind. With his institution, he has US patent US20130217707 A1 for the use of sodium–hydrogen exchange inhibitors in the treatment of ADHD. He also receives royalties from books published by Guilford Press: Straight Talk about Your Child's Mental Health, Oxford University Press: Schizophrenia: The Facts and Elsevier: ADHD: Non-Pharmacologic Interventions. He is Program Director of www.adhdinadults.com. J. Antoni Ramos-Quiroga was on the speakers' bureau and/or acted as consultant for Eli-Lilly, Janssen-Cilag, Novartis, Shire, Takeda, Bial, Shionogui, Lundbeck, Almirall, Braingaze, Sincrolab, Medice and Rubió in the last 5 years. He also received travel awards (air tickets + hotel) for taking part in psychiatric meetings from Janssen-Cilag, Rubió, Shire, Takeda, Shionogui, Bial, Medice and Eli-Lilly. The Department of Psychiatry chaired by him received unrestricted educational and research support from the following companies in the last 5 years: Eli-Lilly, Lundbeck, Janssen-Cilag, Actelion, Shire, Ferrer, Oryzon, Roche, Psious and Rubió. Professor Barbara Franke received educational speaking fees from Medice. Dr Crosbie has received unrestricted funds from DNAgenotek. All funds were received by the Hospital for Sick Children for studies related to ADHD and neurodevelopmental disorders. Dr Schachar has consulted for Highland Therapeutics, Purdue Pharma, E Lilly Corp and Ehave. The other authors report no conflicts of interest.